Have a language expert improve your writing
Run a free plagiarism check in 10 minutes, generate accurate citations for free.
- Knowledge Base
Methodology
- Guide to Experimental Design | Overview, Steps, & Examples

Guide to Experimental Design | Overview, 5 steps & Examples
Published on December 3, 2019 by Rebecca Bevans . Revised on June 21, 2023.
Experiments are used to study causal relationships . You manipulate one or more independent variables and measure their effect on one or more dependent variables.
Experimental design create a set of procedures to systematically test a hypothesis . A good experimental design requires a strong understanding of the system you are studying.
There are five key steps in designing an experiment:
- Consider your variables and how they are related
- Write a specific, testable hypothesis
- Design experimental treatments to manipulate your independent variable
- Assign subjects to groups, either between-subjects or within-subjects
- Plan how you will measure your dependent variable
For valid conclusions, you also need to select a representative sample and control any extraneous variables that might influence your results. If random assignment of participants to control and treatment groups is impossible, unethical, or highly difficult, consider an observational study instead. This minimizes several types of research bias, particularly sampling bias , survivorship bias , and attrition bias as time passes.
Table of contents
Step 1: define your variables, step 2: write your hypothesis, step 3: design your experimental treatments, step 4: assign your subjects to treatment groups, step 5: measure your dependent variable, other interesting articles, frequently asked questions about experiments.
You should begin with a specific research question . We will work with two research question examples, one from health sciences and one from ecology:
To translate your research question into an experimental hypothesis, you need to define the main variables and make predictions about how they are related.
Start by simply listing the independent and dependent variables .
Research question | Independent variable | Dependent variable |
---|---|---|
Phone use and sleep | Minutes of phone use before sleep | Hours of sleep per night |
Temperature and soil respiration | Air temperature just above the soil surface | CO2 respired from soil |
Then you need to think about possible extraneous and confounding variables and consider how you might control them in your experiment.
Extraneous variable | How to control | |
---|---|---|
Phone use and sleep | in sleep patterns among individuals. | measure the average difference between sleep with phone use and sleep without phone use rather than the average amount of sleep per treatment group. |
Temperature and soil respiration | also affects respiration, and moisture can decrease with increasing temperature. | monitor soil moisture and add water to make sure that soil moisture is consistent across all treatment plots. |
Finally, you can put these variables together into a diagram. Use arrows to show the possible relationships between variables and include signs to show the expected direction of the relationships.
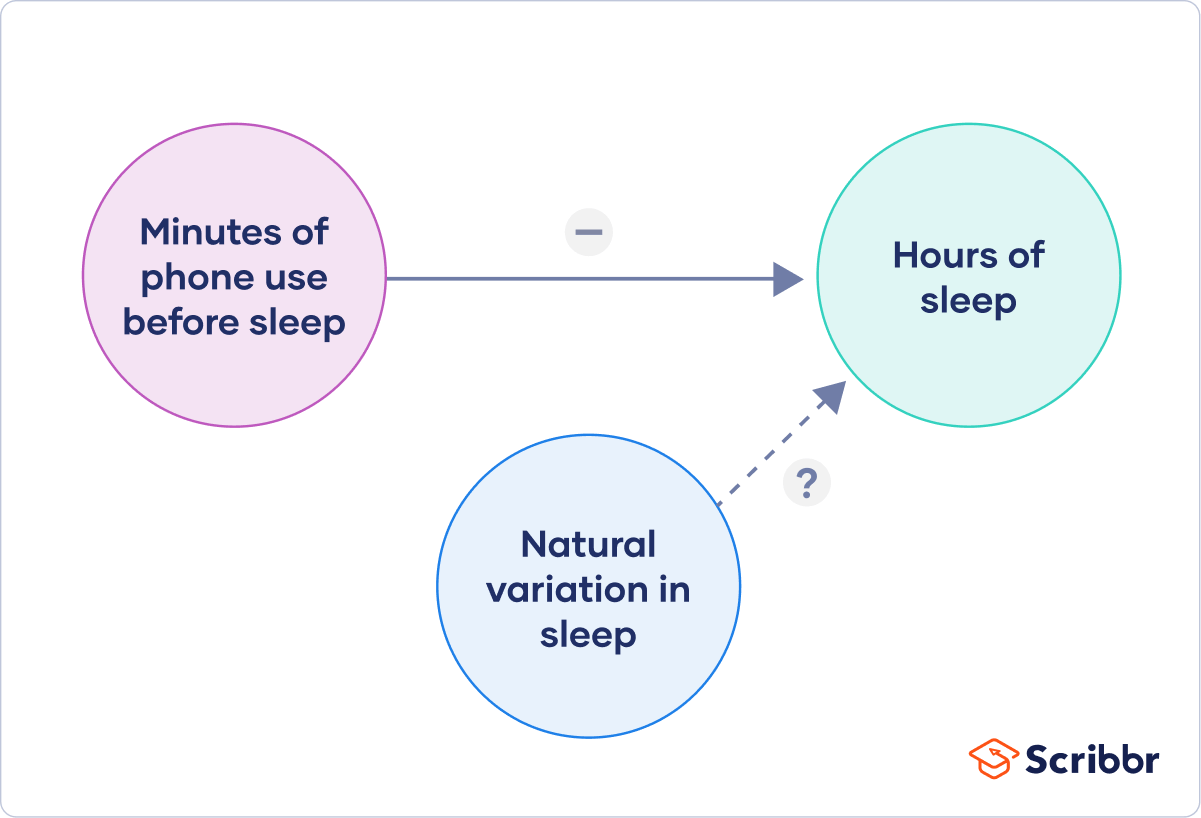
Here we predict that increasing temperature will increase soil respiration and decrease soil moisture, while decreasing soil moisture will lead to decreased soil respiration.
Here's why students love Scribbr's proofreading services
Discover proofreading & editing
Now that you have a strong conceptual understanding of the system you are studying, you should be able to write a specific, testable hypothesis that addresses your research question.
Null hypothesis (H ) | Alternate hypothesis (H ) | |
---|---|---|
Phone use and sleep | Phone use before sleep does not correlate with the amount of sleep a person gets. | Increasing phone use before sleep leads to a decrease in sleep. |
Temperature and soil respiration | Air temperature does not correlate with soil respiration. | Increased air temperature leads to increased soil respiration. |
The next steps will describe how to design a controlled experiment . In a controlled experiment, you must be able to:
- Systematically and precisely manipulate the independent variable(s).
- Precisely measure the dependent variable(s).
- Control any potential confounding variables.
If your study system doesn’t match these criteria, there are other types of research you can use to answer your research question.
How you manipulate the independent variable can affect the experiment’s external validity – that is, the extent to which the results can be generalized and applied to the broader world.
First, you may need to decide how widely to vary your independent variable.
- just slightly above the natural range for your study region.
- over a wider range of temperatures to mimic future warming.
- over an extreme range that is beyond any possible natural variation.
Second, you may need to choose how finely to vary your independent variable. Sometimes this choice is made for you by your experimental system, but often you will need to decide, and this will affect how much you can infer from your results.
- a categorical variable : either as binary (yes/no) or as levels of a factor (no phone use, low phone use, high phone use).
- a continuous variable (minutes of phone use measured every night).
How you apply your experimental treatments to your test subjects is crucial for obtaining valid and reliable results.
First, you need to consider the study size : how many individuals will be included in the experiment? In general, the more subjects you include, the greater your experiment’s statistical power , which determines how much confidence you can have in your results.
Then you need to randomly assign your subjects to treatment groups . Each group receives a different level of the treatment (e.g. no phone use, low phone use, high phone use).
You should also include a control group , which receives no treatment. The control group tells us what would have happened to your test subjects without any experimental intervention.
When assigning your subjects to groups, there are two main choices you need to make:
- A completely randomized design vs a randomized block design .
- A between-subjects design vs a within-subjects design .
Randomization
An experiment can be completely randomized or randomized within blocks (aka strata):
- In a completely randomized design , every subject is assigned to a treatment group at random.
- In a randomized block design (aka stratified random design), subjects are first grouped according to a characteristic they share, and then randomly assigned to treatments within those groups.
Completely randomized design | Randomized block design | |
---|---|---|
Phone use and sleep | Subjects are all randomly assigned a level of phone use using a random number generator. | Subjects are first grouped by age, and then phone use treatments are randomly assigned within these groups. |
Temperature and soil respiration | Warming treatments are assigned to soil plots at random by using a number generator to generate map coordinates within the study area. | Soils are first grouped by average rainfall, and then treatment plots are randomly assigned within these groups. |
Sometimes randomization isn’t practical or ethical , so researchers create partially-random or even non-random designs. An experimental design where treatments aren’t randomly assigned is called a quasi-experimental design .
Between-subjects vs. within-subjects
In a between-subjects design (also known as an independent measures design or classic ANOVA design), individuals receive only one of the possible levels of an experimental treatment.
In medical or social research, you might also use matched pairs within your between-subjects design to make sure that each treatment group contains the same variety of test subjects in the same proportions.
In a within-subjects design (also known as a repeated measures design), every individual receives each of the experimental treatments consecutively, and their responses to each treatment are measured.
Within-subjects or repeated measures can also refer to an experimental design where an effect emerges over time, and individual responses are measured over time in order to measure this effect as it emerges.
Counterbalancing (randomizing or reversing the order of treatments among subjects) is often used in within-subjects designs to ensure that the order of treatment application doesn’t influence the results of the experiment.
Between-subjects (independent measures) design | Within-subjects (repeated measures) design | |
---|---|---|
Phone use and sleep | Subjects are randomly assigned a level of phone use (none, low, or high) and follow that level of phone use throughout the experiment. | Subjects are assigned consecutively to zero, low, and high levels of phone use throughout the experiment, and the order in which they follow these treatments is randomized. |
Temperature and soil respiration | Warming treatments are assigned to soil plots at random and the soils are kept at this temperature throughout the experiment. | Every plot receives each warming treatment (1, 3, 5, 8, and 10C above ambient temperatures) consecutively over the course of the experiment, and the order in which they receive these treatments is randomized. |
Finally, you need to decide how you’ll collect data on your dependent variable outcomes. You should aim for reliable and valid measurements that minimize research bias or error.
Some variables, like temperature, can be objectively measured with scientific instruments. Others may need to be operationalized to turn them into measurable observations.
- Ask participants to record what time they go to sleep and get up each day.
- Ask participants to wear a sleep tracker.
How precisely you measure your dependent variable also affects the kinds of statistical analysis you can use on your data.
Experiments are always context-dependent, and a good experimental design will take into account all of the unique considerations of your study system to produce information that is both valid and relevant to your research question.
If you want to know more about statistics , methodology , or research bias , make sure to check out some of our other articles with explanations and examples.
- Student’s t -distribution
- Normal distribution
- Null and Alternative Hypotheses
- Chi square tests
- Confidence interval
- Cluster sampling
- Stratified sampling
- Data cleansing
- Reproducibility vs Replicability
- Peer review
- Likert scale
Research bias
- Implicit bias
- Framing effect
- Cognitive bias
- Placebo effect
- Hawthorne effect
- Hindsight bias
- Affect heuristic
Experimental design means planning a set of procedures to investigate a relationship between variables . To design a controlled experiment, you need:
- A testable hypothesis
- At least one independent variable that can be precisely manipulated
- At least one dependent variable that can be precisely measured
When designing the experiment, you decide:
- How you will manipulate the variable(s)
- How you will control for any potential confounding variables
- How many subjects or samples will be included in the study
- How subjects will be assigned to treatment levels
Experimental design is essential to the internal and external validity of your experiment.
The key difference between observational studies and experimental designs is that a well-done observational study does not influence the responses of participants, while experiments do have some sort of treatment condition applied to at least some participants by random assignment .
A confounding variable , also called a confounder or confounding factor, is a third variable in a study examining a potential cause-and-effect relationship.
A confounding variable is related to both the supposed cause and the supposed effect of the study. It can be difficult to separate the true effect of the independent variable from the effect of the confounding variable.
In your research design , it’s important to identify potential confounding variables and plan how you will reduce their impact.
In a between-subjects design , every participant experiences only one condition, and researchers assess group differences between participants in various conditions.
In a within-subjects design , each participant experiences all conditions, and researchers test the same participants repeatedly for differences between conditions.
The word “between” means that you’re comparing different conditions between groups, while the word “within” means you’re comparing different conditions within the same group.
An experimental group, also known as a treatment group, receives the treatment whose effect researchers wish to study, whereas a control group does not. They should be identical in all other ways.
Cite this Scribbr article
If you want to cite this source, you can copy and paste the citation or click the “Cite this Scribbr article” button to automatically add the citation to our free Citation Generator.
Bevans, R. (2023, June 21). Guide to Experimental Design | Overview, 5 steps & Examples. Scribbr. Retrieved September 26, 2024, from https://www.scribbr.com/methodology/experimental-design/
Is this article helpful?
Rebecca Bevans
Other students also liked, random assignment in experiments | introduction & examples, quasi-experimental design | definition, types & examples, how to write a lab report, "i thought ai proofreading was useless but..".
I've been using Scribbr for years now and I know it's a service that won't disappoint. It does a good job spotting mistakes”
Reference.com
What's Your Question?
- History & Geography
- Science & Technology
- Business & Finance
- Pets & Animals
What Is an Experimental Setup in Science?
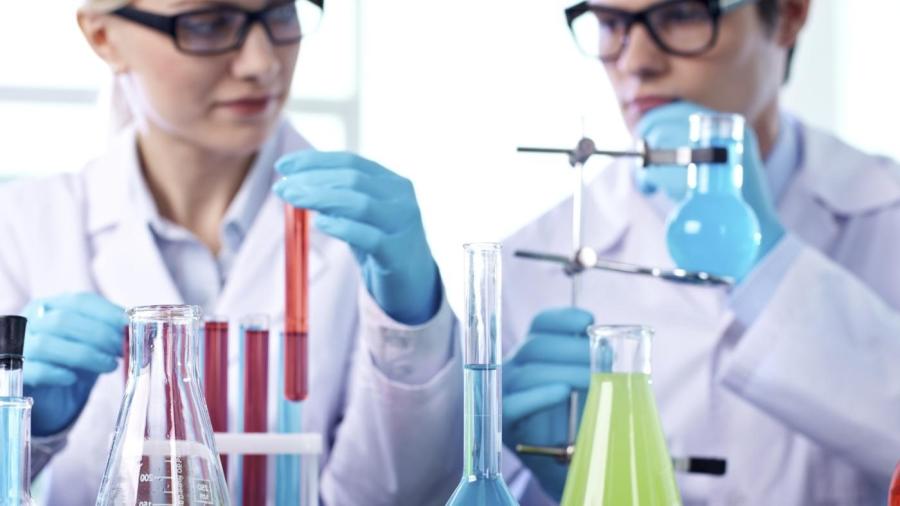
In science, the experimental setup is the part of research in which the experimenter analyzes the effect of a specific variable. This setup is quite similar to the control setup; ideally, the only difference involves the variable that the experimenter wants to test in the current project.
Consider a scenario in which a researcher wants to determine whether scuffing a baseball with an emery board provides more distortion to the baseball’s flight than a dab or two of Vaseline. Both of these methods were used, primarily in the 1970s and 1980s, to help pitchers gain an advantage over batters. The researcher would engage the services of a pitcher, and after a warm-up period, would have the pitcher throw a set number of pitches, such as 10 fastballs and 10 curve balls with no doctoring to the baseball at all. This is the control setup.
Next, the pitcher would use an emery board to scuff the surface of the ball. It would be important for these pitches to take place at the same time and place as the control pitches to keep the environmental factors the same. The experimental setup would involve 10 fastballs and 10 curve balls with the doctored baseball. Continuing with 10 fastballs and 10 curve balls with a ball that has some Vaseline on it and comparing observations of the flight of the baseball would constitute the experimental setup. The observer could be a potential batter or someone standing behind the catcher — or the catcher himself.
MORE FROM REFERENCE.COM
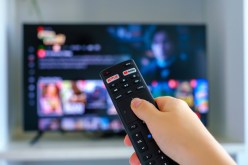
Have a language expert improve your writing
Run a free plagiarism check in 10 minutes, automatically generate references for free.
- Knowledge Base
- Methodology
- A Quick Guide to Experimental Design | 5 Steps & Examples
A Quick Guide to Experimental Design | 5 Steps & Examples
Published on 11 April 2022 by Rebecca Bevans . Revised on 5 December 2022.
Experiments are used to study causal relationships . You manipulate one or more independent variables and measure their effect on one or more dependent variables.
Experimental design means creating a set of procedures to systematically test a hypothesis . A good experimental design requires a strong understanding of the system you are studying.
There are five key steps in designing an experiment:
- Consider your variables and how they are related
- Write a specific, testable hypothesis
- Design experimental treatments to manipulate your independent variable
- Assign subjects to groups, either between-subjects or within-subjects
- Plan how you will measure your dependent variable
For valid conclusions, you also need to select a representative sample and control any extraneous variables that might influence your results. If if random assignment of participants to control and treatment groups is impossible, unethical, or highly difficult, consider an observational study instead.
Table of contents
Step 1: define your variables, step 2: write your hypothesis, step 3: design your experimental treatments, step 4: assign your subjects to treatment groups, step 5: measure your dependent variable, frequently asked questions about experimental design.
You should begin with a specific research question . We will work with two research question examples, one from health sciences and one from ecology:
To translate your research question into an experimental hypothesis, you need to define the main variables and make predictions about how they are related.
Start by simply listing the independent and dependent variables .
Research question | Independent variable | Dependent variable |
---|---|---|
Phone use and sleep | Minutes of phone use before sleep | Hours of sleep per night |
Temperature and soil respiration | Air temperature just above the soil surface | CO2 respired from soil |
Then you need to think about possible extraneous and confounding variables and consider how you might control them in your experiment.
Extraneous variable | How to control | |
---|---|---|
Phone use and sleep | in sleep patterns among individuals. | measure the average difference between sleep with phone use and sleep without phone use rather than the average amount of sleep per treatment group. |
Temperature and soil respiration | also affects respiration, and moisture can decrease with increasing temperature. | monitor soil moisture and add water to make sure that soil moisture is consistent across all treatment plots. |
Finally, you can put these variables together into a diagram. Use arrows to show the possible relationships between variables and include signs to show the expected direction of the relationships.
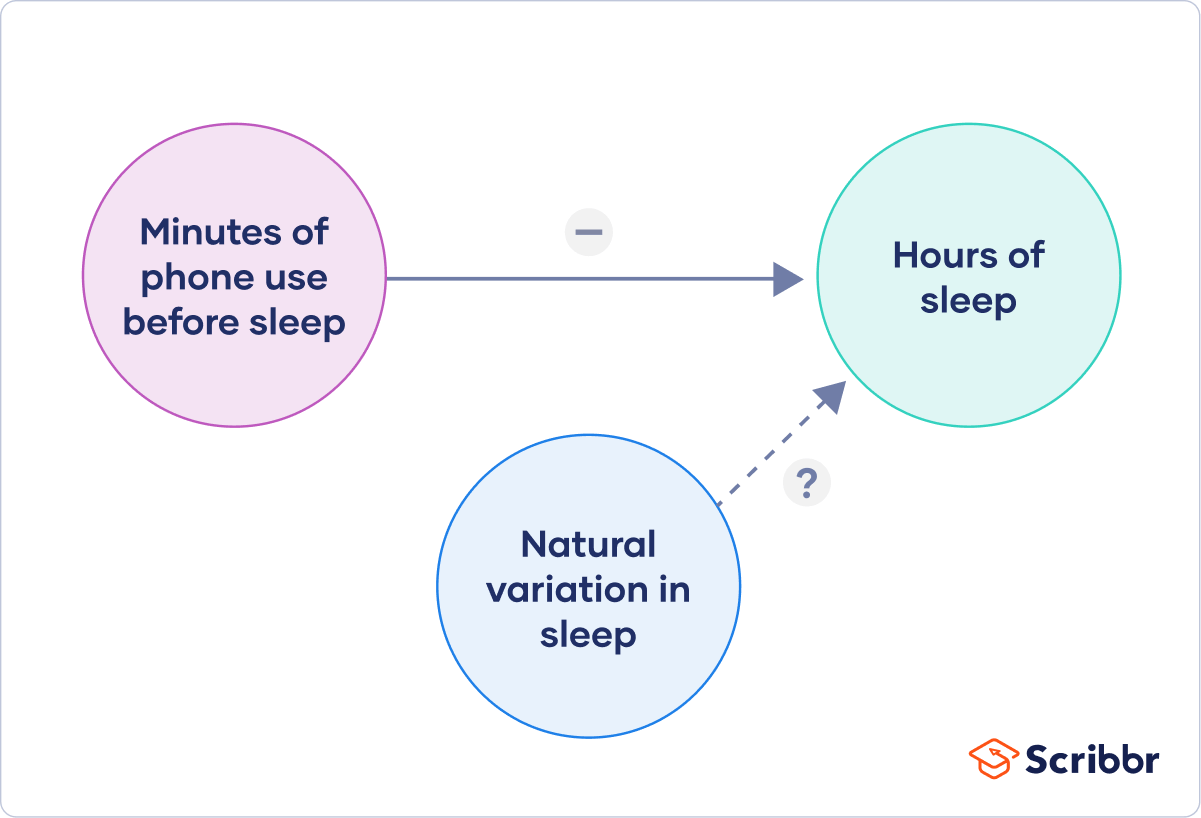
Here we predict that increasing temperature will increase soil respiration and decrease soil moisture, while decreasing soil moisture will lead to decreased soil respiration.
Prevent plagiarism, run a free check.
Now that you have a strong conceptual understanding of the system you are studying, you should be able to write a specific, testable hypothesis that addresses your research question.
Null hypothesis (H ) | Alternate hypothesis (H ) | |
---|---|---|
Phone use and sleep | Phone use before sleep does not correlate with the amount of sleep a person gets. | Increasing phone use before sleep leads to a decrease in sleep. |
Temperature and soil respiration | Air temperature does not correlate with soil respiration. | Increased air temperature leads to increased soil respiration. |
The next steps will describe how to design a controlled experiment . In a controlled experiment, you must be able to:
- Systematically and precisely manipulate the independent variable(s).
- Precisely measure the dependent variable(s).
- Control any potential confounding variables.
If your study system doesn’t match these criteria, there are other types of research you can use to answer your research question.
How you manipulate the independent variable can affect the experiment’s external validity – that is, the extent to which the results can be generalised and applied to the broader world.
First, you may need to decide how widely to vary your independent variable.
- just slightly above the natural range for your study region.
- over a wider range of temperatures to mimic future warming.
- over an extreme range that is beyond any possible natural variation.
Second, you may need to choose how finely to vary your independent variable. Sometimes this choice is made for you by your experimental system, but often you will need to decide, and this will affect how much you can infer from your results.
- a categorical variable : either as binary (yes/no) or as levels of a factor (no phone use, low phone use, high phone use).
- a continuous variable (minutes of phone use measured every night).
How you apply your experimental treatments to your test subjects is crucial for obtaining valid and reliable results.
First, you need to consider the study size : how many individuals will be included in the experiment? In general, the more subjects you include, the greater your experiment’s statistical power , which determines how much confidence you can have in your results.
Then you need to randomly assign your subjects to treatment groups . Each group receives a different level of the treatment (e.g. no phone use, low phone use, high phone use).
You should also include a control group , which receives no treatment. The control group tells us what would have happened to your test subjects without any experimental intervention.
When assigning your subjects to groups, there are two main choices you need to make:
- A completely randomised design vs a randomised block design .
- A between-subjects design vs a within-subjects design .
Randomisation
An experiment can be completely randomised or randomised within blocks (aka strata):
- In a completely randomised design , every subject is assigned to a treatment group at random.
- In a randomised block design (aka stratified random design), subjects are first grouped according to a characteristic they share, and then randomly assigned to treatments within those groups.
Completely randomised design | Randomised block design | |
---|---|---|
Phone use and sleep | Subjects are all randomly assigned a level of phone use using a random number generator. | Subjects are first grouped by age, and then phone use treatments are randomly assigned within these groups. |
Temperature and soil respiration | Warming treatments are assigned to soil plots at random by using a number generator to generate map coordinates within the study area. | Soils are first grouped by average rainfall, and then treatment plots are randomly assigned within these groups. |
Sometimes randomisation isn’t practical or ethical , so researchers create partially-random or even non-random designs. An experimental design where treatments aren’t randomly assigned is called a quasi-experimental design .
Between-subjects vs within-subjects
In a between-subjects design (also known as an independent measures design or classic ANOVA design), individuals receive only one of the possible levels of an experimental treatment.
In medical or social research, you might also use matched pairs within your between-subjects design to make sure that each treatment group contains the same variety of test subjects in the same proportions.
In a within-subjects design (also known as a repeated measures design), every individual receives each of the experimental treatments consecutively, and their responses to each treatment are measured.
Within-subjects or repeated measures can also refer to an experimental design where an effect emerges over time, and individual responses are measured over time in order to measure this effect as it emerges.
Counterbalancing (randomising or reversing the order of treatments among subjects) is often used in within-subjects designs to ensure that the order of treatment application doesn’t influence the results of the experiment.
Between-subjects (independent measures) design | Within-subjects (repeated measures) design | |
---|---|---|
Phone use and sleep | Subjects are randomly assigned a level of phone use (none, low, or high) and follow that level of phone use throughout the experiment. | Subjects are assigned consecutively to zero, low, and high levels of phone use throughout the experiment, and the order in which they follow these treatments is randomised. |
Temperature and soil respiration | Warming treatments are assigned to soil plots at random and the soils are kept at this temperature throughout the experiment. | Every plot receives each warming treatment (1, 3, 5, 8, and 10C above ambient temperatures) consecutively over the course of the experiment, and the order in which they receive these treatments is randomised. |
Finally, you need to decide how you’ll collect data on your dependent variable outcomes. You should aim for reliable and valid measurements that minimise bias or error.
Some variables, like temperature, can be objectively measured with scientific instruments. Others may need to be operationalised to turn them into measurable observations.
- Ask participants to record what time they go to sleep and get up each day.
- Ask participants to wear a sleep tracker.
How precisely you measure your dependent variable also affects the kinds of statistical analysis you can use on your data.
Experiments are always context-dependent, and a good experimental design will take into account all of the unique considerations of your study system to produce information that is both valid and relevant to your research question.
Experimental designs are a set of procedures that you plan in order to examine the relationship between variables that interest you.
To design a successful experiment, first identify:
- A testable hypothesis
- One or more independent variables that you will manipulate
- One or more dependent variables that you will measure
When designing the experiment, first decide:
- How your variable(s) will be manipulated
- How you will control for any potential confounding or lurking variables
- How many subjects you will include
- How you will assign treatments to your subjects
The key difference between observational studies and experiments is that, done correctly, an observational study will never influence the responses or behaviours of participants. Experimental designs will have a treatment condition applied to at least a portion of participants.
A confounding variable , also called a confounder or confounding factor, is a third variable in a study examining a potential cause-and-effect relationship.
A confounding variable is related to both the supposed cause and the supposed effect of the study. It can be difficult to separate the true effect of the independent variable from the effect of the confounding variable.
In your research design , it’s important to identify potential confounding variables and plan how you will reduce their impact.
In a between-subjects design , every participant experiences only one condition, and researchers assess group differences between participants in various conditions.
In a within-subjects design , each participant experiences all conditions, and researchers test the same participants repeatedly for differences between conditions.
The word ‘between’ means that you’re comparing different conditions between groups, while the word ‘within’ means you’re comparing different conditions within the same group.
Cite this Scribbr article
If you want to cite this source, you can copy and paste the citation or click the ‘Cite this Scribbr article’ button to automatically add the citation to our free Reference Generator.
Bevans, R. (2022, December 05). A Quick Guide to Experimental Design | 5 Steps & Examples. Scribbr. Retrieved 23 September 2024, from https://www.scribbr.co.uk/research-methods/guide-to-experimental-design/
Is this article helpful?
Rebecca Bevans
19+ Experimental Design Examples (Methods + Types)

Ever wondered how scientists discover new medicines, psychologists learn about behavior, or even how marketers figure out what kind of ads you like? Well, they all have something in common: they use a special plan or recipe called an "experimental design."
Imagine you're baking cookies. You can't just throw random amounts of flour, sugar, and chocolate chips into a bowl and hope for the best. You follow a recipe, right? Scientists and researchers do something similar. They follow a "recipe" called an experimental design to make sure their experiments are set up in a way that the answers they find are meaningful and reliable.
Experimental design is the roadmap researchers use to answer questions. It's a set of rules and steps that researchers follow to collect information, or "data," in a way that is fair, accurate, and makes sense.
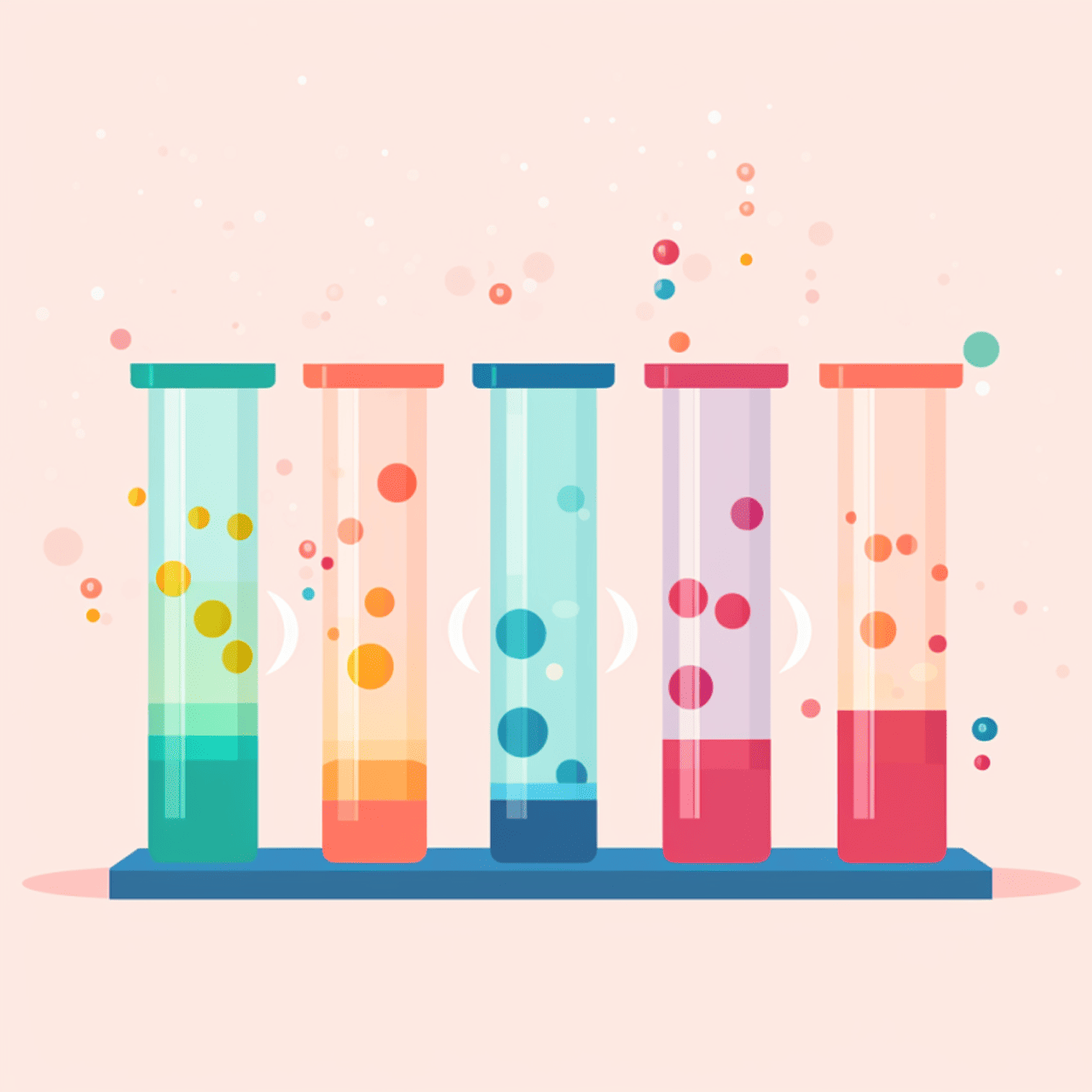
Long ago, people didn't have detailed game plans for experiments. They often just tried things out and saw what happened. But over time, people got smarter about this. They started creating structured plans—what we now call experimental designs—to get clearer, more trustworthy answers to their questions.
In this article, we'll take you on a journey through the world of experimental designs. We'll talk about the different types, or "flavors," of experimental designs, where they're used, and even give you a peek into how they came to be.
What Is Experimental Design?
Alright, before we dive into the different types of experimental designs, let's get crystal clear on what experimental design actually is.
Imagine you're a detective trying to solve a mystery. You need clues, right? Well, in the world of research, experimental design is like the roadmap that helps you find those clues. It's like the game plan in sports or the blueprint when you're building a house. Just like you wouldn't start building without a good blueprint, researchers won't start their studies without a strong experimental design.
So, why do we need experimental design? Think about baking a cake. If you toss ingredients into a bowl without measuring, you'll end up with a mess instead of a tasty dessert.
Similarly, in research, if you don't have a solid plan, you might get confusing or incorrect results. A good experimental design helps you ask the right questions ( think critically ), decide what to measure ( come up with an idea ), and figure out how to measure it (test it). It also helps you consider things that might mess up your results, like outside influences you hadn't thought of.
For example, let's say you want to find out if listening to music helps people focus better. Your experimental design would help you decide things like: Who are you going to test? What kind of music will you use? How will you measure focus? And, importantly, how will you make sure that it's really the music affecting focus and not something else, like the time of day or whether someone had a good breakfast?
In short, experimental design is the master plan that guides researchers through the process of collecting data, so they can answer questions in the most reliable way possible. It's like the GPS for the journey of discovery!
History of Experimental Design
Around 350 BCE, people like Aristotle were trying to figure out how the world works, but they mostly just thought really hard about things. They didn't test their ideas much. So while they were super smart, their methods weren't always the best for finding out the truth.
Fast forward to the Renaissance (14th to 17th centuries), a time of big changes and lots of curiosity. People like Galileo started to experiment by actually doing tests, like rolling balls down inclined planes to study motion. Galileo's work was cool because he combined thinking with doing. He'd have an idea, test it, look at the results, and then think some more. This approach was a lot more reliable than just sitting around and thinking.
Now, let's zoom ahead to the 18th and 19th centuries. This is when people like Francis Galton, an English polymath, started to get really systematic about experimentation. Galton was obsessed with measuring things. Seriously, he even tried to measure how good-looking people were ! His work helped create the foundations for a more organized approach to experiments.
Next stop: the early 20th century. Enter Ronald A. Fisher , a brilliant British statistician. Fisher was a game-changer. He came up with ideas that are like the bread and butter of modern experimental design.
Fisher invented the concept of the " control group "—that's a group of people or things that don't get the treatment you're testing, so you can compare them to those who do. He also stressed the importance of " randomization ," which means assigning people or things to different groups by chance, like drawing names out of a hat. This makes sure the experiment is fair and the results are trustworthy.
Around the same time, American psychologists like John B. Watson and B.F. Skinner were developing " behaviorism ." They focused on studying things that they could directly observe and measure, like actions and reactions.
Skinner even built boxes—called Skinner Boxes —to test how animals like pigeons and rats learn. Their work helped shape how psychologists design experiments today. Watson performed a very controversial experiment called The Little Albert experiment that helped describe behaviour through conditioning—in other words, how people learn to behave the way they do.
In the later part of the 20th century and into our time, computers have totally shaken things up. Researchers now use super powerful software to help design their experiments and crunch the numbers.
With computers, they can simulate complex experiments before they even start, which helps them predict what might happen. This is especially helpful in fields like medicine, where getting things right can be a matter of life and death.
Also, did you know that experimental designs aren't just for scientists in labs? They're used by people in all sorts of jobs, like marketing, education, and even video game design! Yes, someone probably ran an experiment to figure out what makes a game super fun to play.
So there you have it—a quick tour through the history of experimental design, from Aristotle's deep thoughts to Fisher's groundbreaking ideas, and all the way to today's computer-powered research. These designs are the recipes that help people from all walks of life find answers to their big questions.
Key Terms in Experimental Design
Before we dig into the different types of experimental designs, let's get comfy with some key terms. Understanding these terms will make it easier for us to explore the various types of experimental designs that researchers use to answer their big questions.
Independent Variable : This is what you change or control in your experiment to see what effect it has. Think of it as the "cause" in a cause-and-effect relationship. For example, if you're studying whether different types of music help people focus, the kind of music is the independent variable.
Dependent Variable : This is what you're measuring to see the effect of your independent variable. In our music and focus experiment, how well people focus is the dependent variable—it's what "depends" on the kind of music played.
Control Group : This is a group of people who don't get the special treatment or change you're testing. They help you see what happens when the independent variable is not applied. If you're testing whether a new medicine works, the control group would take a fake pill, called a placebo , instead of the real medicine.
Experimental Group : This is the group that gets the special treatment or change you're interested in. Going back to our medicine example, this group would get the actual medicine to see if it has any effect.
Randomization : This is like shaking things up in a fair way. You randomly put people into the control or experimental group so that each group is a good mix of different kinds of people. This helps make the results more reliable.
Sample : This is the group of people you're studying. They're a "sample" of a larger group that you're interested in. For instance, if you want to know how teenagers feel about a new video game, you might study a sample of 100 teenagers.
Bias : This is anything that might tilt your experiment one way or another without you realizing it. Like if you're testing a new kind of dog food and you only test it on poodles, that could create a bias because maybe poodles just really like that food and other breeds don't.
Data : This is the information you collect during the experiment. It's like the treasure you find on your journey of discovery!
Replication : This means doing the experiment more than once to make sure your findings hold up. It's like double-checking your answers on a test.
Hypothesis : This is your educated guess about what will happen in the experiment. It's like predicting the end of a movie based on the first half.
Steps of Experimental Design
Alright, let's say you're all fired up and ready to run your own experiment. Cool! But where do you start? Well, designing an experiment is a bit like planning a road trip. There are some key steps you've got to take to make sure you reach your destination. Let's break it down:
- Ask a Question : Before you hit the road, you've got to know where you're going. Same with experiments. You start with a question you want to answer, like "Does eating breakfast really make you do better in school?"
- Do Some Homework : Before you pack your bags, you look up the best places to visit, right? In science, this means reading up on what other people have already discovered about your topic.
- Form a Hypothesis : This is your educated guess about what you think will happen. It's like saying, "I bet this route will get us there faster."
- Plan the Details : Now you decide what kind of car you're driving (your experimental design), who's coming with you (your sample), and what snacks to bring (your variables).
- Randomization : Remember, this is like shuffling a deck of cards. You want to mix up who goes into your control and experimental groups to make sure it's a fair test.
- Run the Experiment : Finally, the rubber hits the road! You carry out your plan, making sure to collect your data carefully.
- Analyze the Data : Once the trip's over, you look at your photos and decide which ones are keepers. In science, this means looking at your data to see what it tells you.
- Draw Conclusions : Based on your data, did you find an answer to your question? This is like saying, "Yep, that route was faster," or "Nope, we hit a ton of traffic."
- Share Your Findings : After a great trip, you want to tell everyone about it, right? Scientists do the same by publishing their results so others can learn from them.
- Do It Again? : Sometimes one road trip just isn't enough. In the same way, scientists often repeat their experiments to make sure their findings are solid.
So there you have it! Those are the basic steps you need to follow when you're designing an experiment. Each step helps make sure that you're setting up a fair and reliable way to find answers to your big questions.
Let's get into examples of experimental designs.
1) True Experimental Design
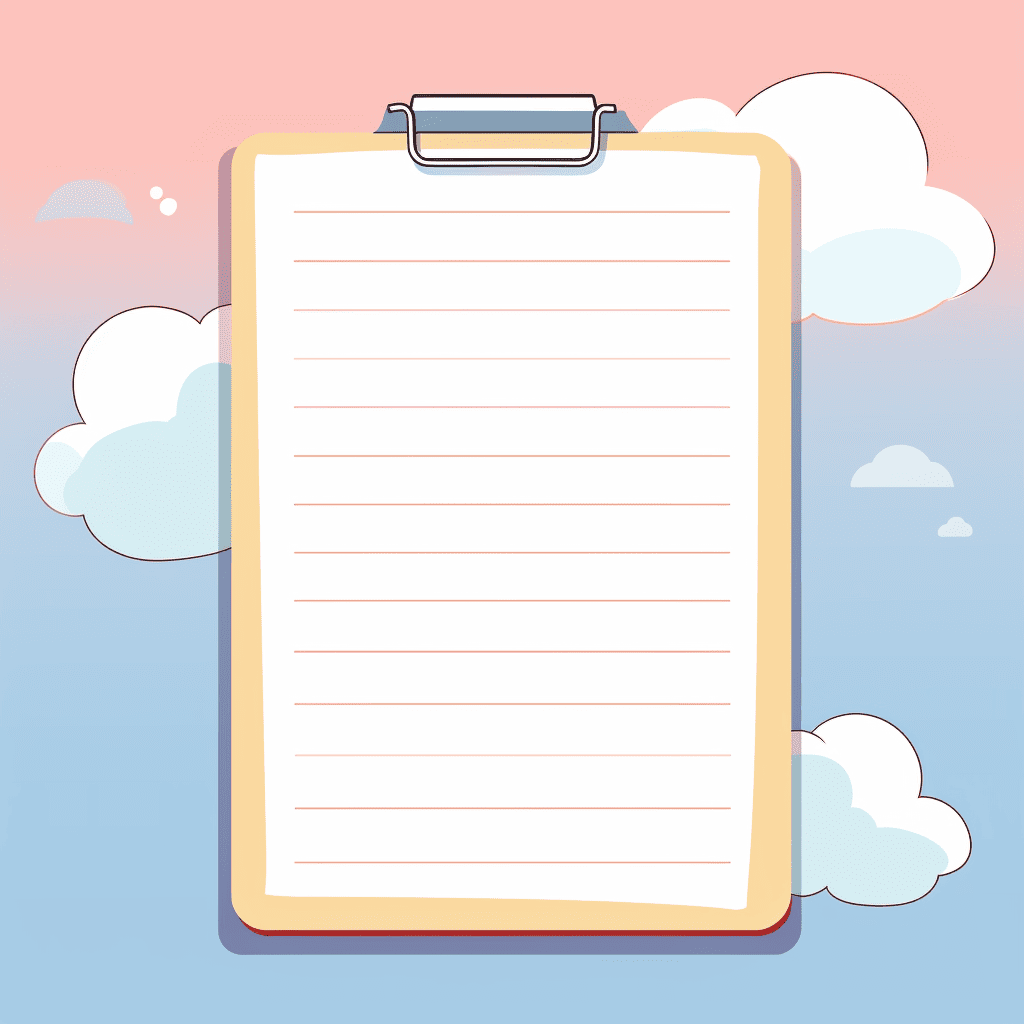
In the world of experiments, the True Experimental Design is like the superstar quarterback everyone talks about. Born out of the early 20th-century work of statisticians like Ronald A. Fisher, this design is all about control, precision, and reliability.
Researchers carefully pick an independent variable to manipulate (remember, that's the thing they're changing on purpose) and measure the dependent variable (the effect they're studying). Then comes the magic trick—randomization. By randomly putting participants into either the control or experimental group, scientists make sure their experiment is as fair as possible.
No sneaky biases here!
True Experimental Design Pros
The pros of True Experimental Design are like the perks of a VIP ticket at a concert: you get the best and most trustworthy results. Because everything is controlled and randomized, you can feel pretty confident that the results aren't just a fluke.
True Experimental Design Cons
However, there's a catch. Sometimes, it's really tough to set up these experiments in a real-world situation. Imagine trying to control every single detail of your day, from the food you eat to the air you breathe. Not so easy, right?
True Experimental Design Uses
The fields that get the most out of True Experimental Designs are those that need super reliable results, like medical research.
When scientists were developing COVID-19 vaccines, they used this design to run clinical trials. They had control groups that received a placebo (a harmless substance with no effect) and experimental groups that got the actual vaccine. Then they measured how many people in each group got sick. By comparing the two, they could say, "Yep, this vaccine works!"
So next time you read about a groundbreaking discovery in medicine or technology, chances are a True Experimental Design was the VIP behind the scenes, making sure everything was on point. It's been the go-to for rigorous scientific inquiry for nearly a century, and it's not stepping off the stage anytime soon.
2) Quasi-Experimental Design
So, let's talk about the Quasi-Experimental Design. Think of this one as the cool cousin of True Experimental Design. It wants to be just like its famous relative, but it's a bit more laid-back and flexible. You'll find quasi-experimental designs when it's tricky to set up a full-blown True Experimental Design with all the bells and whistles.
Quasi-experiments still play with an independent variable, just like their stricter cousins. The big difference? They don't use randomization. It's like wanting to divide a bag of jelly beans equally between your friends, but you can't quite do it perfectly.
In real life, it's often not possible or ethical to randomly assign people to different groups, especially when dealing with sensitive topics like education or social issues. And that's where quasi-experiments come in.
Quasi-Experimental Design Pros
Even though they lack full randomization, quasi-experimental designs are like the Swiss Army knives of research: versatile and practical. They're especially popular in fields like education, sociology, and public policy.
For instance, when researchers wanted to figure out if the Head Start program , aimed at giving young kids a "head start" in school, was effective, they used a quasi-experimental design. They couldn't randomly assign kids to go or not go to preschool, but they could compare kids who did with kids who didn't.
Quasi-Experimental Design Cons
Of course, quasi-experiments come with their own bag of pros and cons. On the plus side, they're easier to set up and often cheaper than true experiments. But the flip side is that they're not as rock-solid in their conclusions. Because the groups aren't randomly assigned, there's always that little voice saying, "Hey, are we missing something here?"
Quasi-Experimental Design Uses
Quasi-Experimental Design gained traction in the mid-20th century. Researchers were grappling with real-world problems that didn't fit neatly into a laboratory setting. Plus, as society became more aware of ethical considerations, the need for flexible designs increased. So, the quasi-experimental approach was like a breath of fresh air for scientists wanting to study complex issues without a laundry list of restrictions.
In short, if True Experimental Design is the superstar quarterback, Quasi-Experimental Design is the versatile player who can adapt and still make significant contributions to the game.
3) Pre-Experimental Design
Now, let's talk about the Pre-Experimental Design. Imagine it as the beginner's skateboard you get before you try out for all the cool tricks. It has wheels, it rolls, but it's not built for the professional skatepark.
Similarly, pre-experimental designs give researchers a starting point. They let you dip your toes in the water of scientific research without diving in head-first.
So, what's the deal with pre-experimental designs?
Pre-Experimental Designs are the basic, no-frills versions of experiments. Researchers still mess around with an independent variable and measure a dependent variable, but they skip over the whole randomization thing and often don't even have a control group.
It's like baking a cake but forgetting the frosting and sprinkles; you'll get some results, but they might not be as complete or reliable as you'd like.
Pre-Experimental Design Pros
Why use such a simple setup? Because sometimes, you just need to get the ball rolling. Pre-experimental designs are great for quick-and-dirty research when you're short on time or resources. They give you a rough idea of what's happening, which you can use to plan more detailed studies later.
A good example of this is early studies on the effects of screen time on kids. Researchers couldn't control every aspect of a child's life, but they could easily ask parents to track how much time their kids spent in front of screens and then look for trends in behavior or school performance.
Pre-Experimental Design Cons
But here's the catch: pre-experimental designs are like that first draft of an essay. It helps you get your ideas down, but you wouldn't want to turn it in for a grade. Because these designs lack the rigorous structure of true or quasi-experimental setups, they can't give you rock-solid conclusions. They're more like clues or signposts pointing you in a certain direction.
Pre-Experimental Design Uses
This type of design became popular in the early stages of various scientific fields. Researchers used them to scratch the surface of a topic, generate some initial data, and then decide if it's worth exploring further. In other words, pre-experimental designs were the stepping stones that led to more complex, thorough investigations.
So, while Pre-Experimental Design may not be the star player on the team, it's like the practice squad that helps everyone get better. It's the starting point that can lead to bigger and better things.
4) Factorial Design
Now, buckle up, because we're moving into the world of Factorial Design, the multi-tasker of the experimental universe.
Imagine juggling not just one, but multiple balls in the air—that's what researchers do in a factorial design.
In Factorial Design, researchers are not satisfied with just studying one independent variable. Nope, they want to study two or more at the same time to see how they interact.
It's like cooking with several spices to see how they blend together to create unique flavors.
Factorial Design became the talk of the town with the rise of computers. Why? Because this design produces a lot of data, and computers are the number crunchers that help make sense of it all. So, thanks to our silicon friends, researchers can study complicated questions like, "How do diet AND exercise together affect weight loss?" instead of looking at just one of those factors.
Factorial Design Pros
This design's main selling point is its ability to explore interactions between variables. For instance, maybe a new study drug works really well for young people but not so great for older adults. A factorial design could reveal that age is a crucial factor, something you might miss if you only studied the drug's effectiveness in general. It's like being a detective who looks for clues not just in one room but throughout the entire house.
Factorial Design Cons
However, factorial designs have their own bag of challenges. First off, they can be pretty complicated to set up and run. Imagine coordinating a four-way intersection with lots of cars coming from all directions—you've got to make sure everything runs smoothly, or you'll end up with a traffic jam. Similarly, researchers need to carefully plan how they'll measure and analyze all the different variables.
Factorial Design Uses
Factorial designs are widely used in psychology to untangle the web of factors that influence human behavior. They're also popular in fields like marketing, where companies want to understand how different aspects like price, packaging, and advertising influence a product's success.
And speaking of success, the factorial design has been a hit since statisticians like Ronald A. Fisher (yep, him again!) expanded on it in the early-to-mid 20th century. It offered a more nuanced way of understanding the world, proving that sometimes, to get the full picture, you've got to juggle more than one ball at a time.
So, if True Experimental Design is the quarterback and Quasi-Experimental Design is the versatile player, Factorial Design is the strategist who sees the entire game board and makes moves accordingly.
5) Longitudinal Design
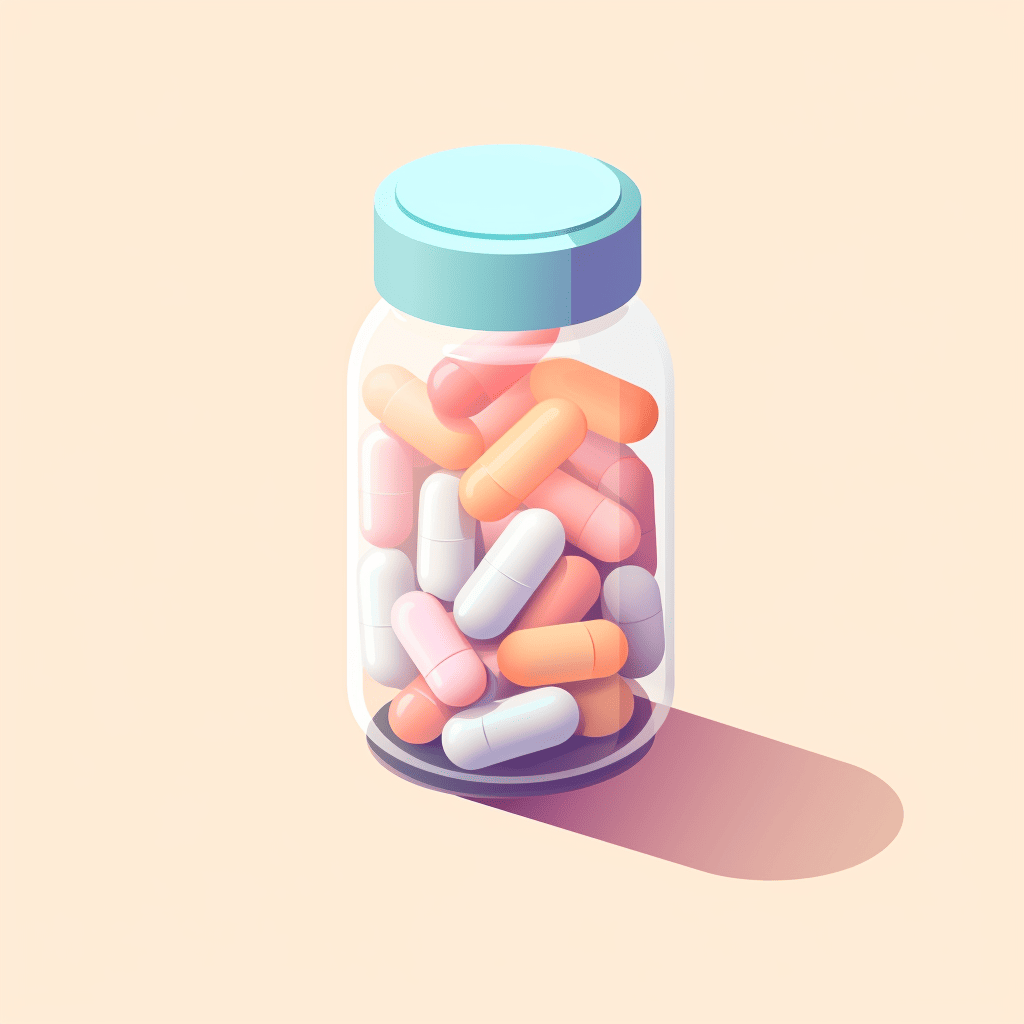
Alright, let's take a step into the world of Longitudinal Design. Picture it as the grand storyteller, the kind who doesn't just tell you about a single event but spins an epic tale that stretches over years or even decades. This design isn't about quick snapshots; it's about capturing the whole movie of someone's life or a long-running process.
You know how you might take a photo every year on your birthday to see how you've changed? Longitudinal Design is kind of like that, but for scientific research.
With Longitudinal Design, instead of measuring something just once, researchers come back again and again, sometimes over many years, to see how things are going. This helps them understand not just what's happening, but why it's happening and how it changes over time.
This design really started to shine in the latter half of the 20th century, when researchers began to realize that some questions can't be answered in a hurry. Think about studies that look at how kids grow up, or research on how a certain medicine affects you over a long period. These aren't things you can rush.
The famous Framingham Heart Study , started in 1948, is a prime example. It's been studying heart health in a small town in Massachusetts for decades, and the findings have shaped what we know about heart disease.
Longitudinal Design Pros
So, what's to love about Longitudinal Design? First off, it's the go-to for studying change over time, whether that's how people age or how a forest recovers from a fire.
Longitudinal Design Cons
But it's not all sunshine and rainbows. Longitudinal studies take a lot of patience and resources. Plus, keeping track of participants over many years can be like herding cats—difficult and full of surprises.
Longitudinal Design Uses
Despite these challenges, longitudinal studies have been key in fields like psychology, sociology, and medicine. They provide the kind of deep, long-term insights that other designs just can't match.
So, if the True Experimental Design is the superstar quarterback, and the Quasi-Experimental Design is the flexible athlete, then the Factorial Design is the strategist, and the Longitudinal Design is the wise elder who has seen it all and has stories to tell.
6) Cross-Sectional Design
Now, let's flip the script and talk about Cross-Sectional Design, the polar opposite of the Longitudinal Design. If Longitudinal is the grand storyteller, think of Cross-Sectional as the snapshot photographer. It captures a single moment in time, like a selfie that you take to remember a fun day. Researchers using this design collect all their data at one point, providing a kind of "snapshot" of whatever they're studying.
In a Cross-Sectional Design, researchers look at multiple groups all at the same time to see how they're different or similar.
This design rose to popularity in the mid-20th century, mainly because it's so quick and efficient. Imagine wanting to know how people of different ages feel about a new video game. Instead of waiting for years to see how opinions change, you could just ask people of all ages what they think right now. That's Cross-Sectional Design for you—fast and straightforward.
You'll find this type of research everywhere from marketing studies to healthcare. For instance, you might have heard about surveys asking people what they think about a new product or political issue. Those are usually cross-sectional studies, aimed at getting a quick read on public opinion.
Cross-Sectional Design Pros
So, what's the big deal with Cross-Sectional Design? Well, it's the go-to when you need answers fast and don't have the time or resources for a more complicated setup.
Cross-Sectional Design Cons
Remember, speed comes with trade-offs. While you get your results quickly, those results are stuck in time. They can't tell you how things change or why they're changing, just what's happening right now.
Cross-Sectional Design Uses
Also, because they're so quick and simple, cross-sectional studies often serve as the first step in research. They give scientists an idea of what's going on so they can decide if it's worth digging deeper. In that way, they're a bit like a movie trailer, giving you a taste of the action to see if you're interested in seeing the whole film.
So, in our lineup of experimental designs, if True Experimental Design is the superstar quarterback and Longitudinal Design is the wise elder, then Cross-Sectional Design is like the speedy running back—fast, agile, but not designed for long, drawn-out plays.
7) Correlational Design
Next on our roster is the Correlational Design, the keen observer of the experimental world. Imagine this design as the person at a party who loves people-watching. They don't interfere or get involved; they just observe and take mental notes about what's going on.
In a correlational study, researchers don't change or control anything; they simply observe and measure how two variables relate to each other.
The correlational design has roots in the early days of psychology and sociology. Pioneers like Sir Francis Galton used it to study how qualities like intelligence or height could be related within families.
This design is all about asking, "Hey, when this thing happens, does that other thing usually happen too?" For example, researchers might study whether students who have more study time get better grades or whether people who exercise more have lower stress levels.
One of the most famous correlational studies you might have heard of is the link between smoking and lung cancer. Back in the mid-20th century, researchers started noticing that people who smoked a lot also seemed to get lung cancer more often. They couldn't say smoking caused cancer—that would require a true experiment—but the strong correlation was a red flag that led to more research and eventually, health warnings.
Correlational Design Pros
This design is great at proving that two (or more) things can be related. Correlational designs can help prove that more detailed research is needed on a topic. They can help us see patterns or possible causes for things that we otherwise might not have realized.
Correlational Design Cons
But here's where you need to be careful: correlational designs can be tricky. Just because two things are related doesn't mean one causes the other. That's like saying, "Every time I wear my lucky socks, my team wins." Well, it's a fun thought, but those socks aren't really controlling the game.
Correlational Design Uses
Despite this limitation, correlational designs are popular in psychology, economics, and epidemiology, to name a few fields. They're often the first step in exploring a possible relationship between variables. Once a strong correlation is found, researchers may decide to conduct more rigorous experimental studies to examine cause and effect.
So, if the True Experimental Design is the superstar quarterback and the Longitudinal Design is the wise elder, the Factorial Design is the strategist, and the Cross-Sectional Design is the speedster, then the Correlational Design is the clever scout, identifying interesting patterns but leaving the heavy lifting of proving cause and effect to the other types of designs.
8) Meta-Analysis
Last but not least, let's talk about Meta-Analysis, the librarian of experimental designs.
If other designs are all about creating new research, Meta-Analysis is about gathering up everyone else's research, sorting it, and figuring out what it all means when you put it together.
Imagine a jigsaw puzzle where each piece is a different study. Meta-Analysis is the process of fitting all those pieces together to see the big picture.
The concept of Meta-Analysis started to take shape in the late 20th century, when computers became powerful enough to handle massive amounts of data. It was like someone handed researchers a super-powered magnifying glass, letting them examine multiple studies at the same time to find common trends or results.
You might have heard of the Cochrane Reviews in healthcare . These are big collections of meta-analyses that help doctors and policymakers figure out what treatments work best based on all the research that's been done.
For example, if ten different studies show that a certain medicine helps lower blood pressure, a meta-analysis would pull all that information together to give a more accurate answer.
Meta-Analysis Pros
The beauty of Meta-Analysis is that it can provide really strong evidence. Instead of relying on one study, you're looking at the whole landscape of research on a topic.
Meta-Analysis Cons
However, it does have some downsides. For one, Meta-Analysis is only as good as the studies it includes. If those studies are flawed, the meta-analysis will be too. It's like baking a cake: if you use bad ingredients, it doesn't matter how good your recipe is—the cake won't turn out well.
Meta-Analysis Uses
Despite these challenges, meta-analyses are highly respected and widely used in many fields like medicine, psychology, and education. They help us make sense of a world that's bursting with information by showing us the big picture drawn from many smaller snapshots.
So, in our all-star lineup, if True Experimental Design is the quarterback and Longitudinal Design is the wise elder, the Factorial Design is the strategist, the Cross-Sectional Design is the speedster, and the Correlational Design is the scout, then the Meta-Analysis is like the coach, using insights from everyone else's plays to come up with the best game plan.
9) Non-Experimental Design
Now, let's talk about a player who's a bit of an outsider on this team of experimental designs—the Non-Experimental Design. Think of this design as the commentator or the journalist who covers the game but doesn't actually play.
In a Non-Experimental Design, researchers are like reporters gathering facts, but they don't interfere or change anything. They're simply there to describe and analyze.
Non-Experimental Design Pros
So, what's the deal with Non-Experimental Design? Its strength is in description and exploration. It's really good for studying things as they are in the real world, without changing any conditions.
Non-Experimental Design Cons
Because a non-experimental design doesn't manipulate variables, it can't prove cause and effect. It's like a weather reporter: they can tell you it's raining, but they can't tell you why it's raining.
The downside? Since researchers aren't controlling variables, it's hard to rule out other explanations for what they observe. It's like hearing one side of a story—you get an idea of what happened, but it might not be the complete picture.
Non-Experimental Design Uses
Non-Experimental Design has always been a part of research, especially in fields like anthropology, sociology, and some areas of psychology.
For instance, if you've ever heard of studies that describe how people behave in different cultures or what teens like to do in their free time, that's often Non-Experimental Design at work. These studies aim to capture the essence of a situation, like painting a portrait instead of taking a snapshot.
One well-known example you might have heard about is the Kinsey Reports from the 1940s and 1950s, which described sexual behavior in men and women. Researchers interviewed thousands of people but didn't manipulate any variables like you would in a true experiment. They simply collected data to create a comprehensive picture of the subject matter.
So, in our metaphorical team of research designs, if True Experimental Design is the quarterback and Longitudinal Design is the wise elder, Factorial Design is the strategist, Cross-Sectional Design is the speedster, Correlational Design is the scout, and Meta-Analysis is the coach, then Non-Experimental Design is the sports journalist—always present, capturing the game, but not part of the action itself.
10) Repeated Measures Design
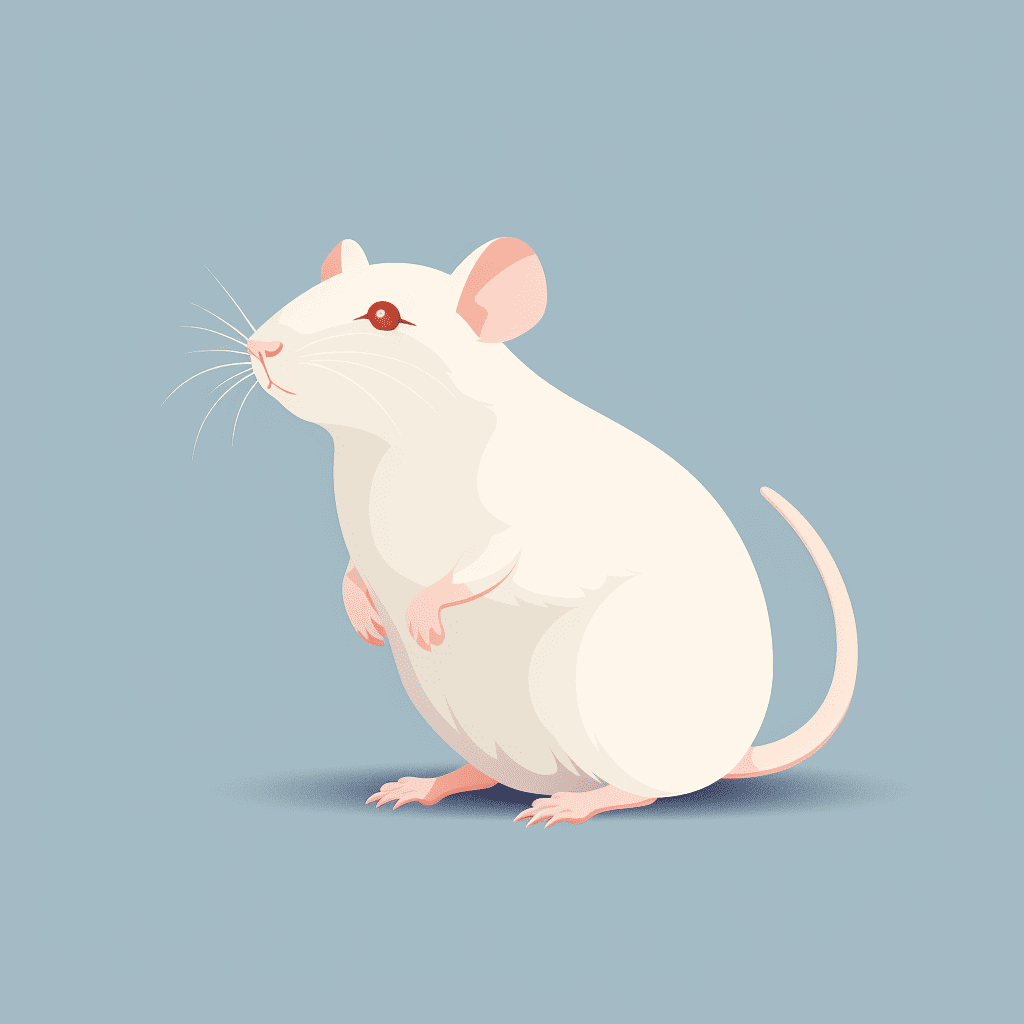
Time to meet the Repeated Measures Design, the time traveler of our research team. If this design were a player in a sports game, it would be the one who keeps revisiting past plays to figure out how to improve the next one.
Repeated Measures Design is all about studying the same people or subjects multiple times to see how they change or react under different conditions.
The idea behind Repeated Measures Design isn't new; it's been around since the early days of psychology and medicine. You could say it's a cousin to the Longitudinal Design, but instead of looking at how things naturally change over time, it focuses on how the same group reacts to different things.
Imagine a study looking at how a new energy drink affects people's running speed. Instead of comparing one group that drank the energy drink to another group that didn't, a Repeated Measures Design would have the same group of people run multiple times—once with the energy drink, and once without. This way, you're really zeroing in on the effect of that energy drink, making the results more reliable.
Repeated Measures Design Pros
The strong point of Repeated Measures Design is that it's super focused. Because it uses the same subjects, you don't have to worry about differences between groups messing up your results.
Repeated Measures Design Cons
But the downside? Well, people can get tired or bored if they're tested too many times, which might affect how they respond.
Repeated Measures Design Uses
A famous example of this design is the "Little Albert" experiment, conducted by John B. Watson and Rosalie Rayner in 1920. In this study, a young boy was exposed to a white rat and other stimuli several times to see how his emotional responses changed. Though the ethical standards of this experiment are often criticized today, it was groundbreaking in understanding conditioned emotional responses.
In our metaphorical lineup of research designs, if True Experimental Design is the quarterback and Longitudinal Design is the wise elder, Factorial Design is the strategist, Cross-Sectional Design is the speedster, Correlational Design is the scout, Meta-Analysis is the coach, and Non-Experimental Design is the journalist, then Repeated Measures Design is the time traveler—always looping back to fine-tune the game plan.
11) Crossover Design
Next up is Crossover Design, the switch-hitter of the research world. If you're familiar with baseball, you'll know a switch-hitter is someone who can bat both right-handed and left-handed.
In a similar way, Crossover Design allows subjects to experience multiple conditions, flipping them around so that everyone gets a turn in each role.
This design is like the utility player on our team—versatile, flexible, and really good at adapting.
The Crossover Design has its roots in medical research and has been popular since the mid-20th century. It's often used in clinical trials to test the effectiveness of different treatments.
Crossover Design Pros
The neat thing about this design is that it allows each participant to serve as their own control group. Imagine you're testing two new kinds of headache medicine. Instead of giving one type to one group and another type to a different group, you'd give both kinds to the same people but at different times.
Crossover Design Cons
What's the big deal with Crossover Design? Its major strength is in reducing the "noise" that comes from individual differences. Since each person experiences all conditions, it's easier to see real effects. However, there's a catch. This design assumes that there's no lasting effect from the first condition when you switch to the second one. That might not always be true. If the first treatment has a long-lasting effect, it could mess up the results when you switch to the second treatment.
Crossover Design Uses
A well-known example of Crossover Design is in studies that look at the effects of different types of diets—like low-carb vs. low-fat diets. Researchers might have participants follow a low-carb diet for a few weeks, then switch them to a low-fat diet. By doing this, they can more accurately measure how each diet affects the same group of people.
In our team of experimental designs, if True Experimental Design is the quarterback and Longitudinal Design is the wise elder, Factorial Design is the strategist, Cross-Sectional Design is the speedster, Correlational Design is the scout, Meta-Analysis is the coach, Non-Experimental Design is the journalist, and Repeated Measures Design is the time traveler, then Crossover Design is the versatile utility player—always ready to adapt and play multiple roles to get the most accurate results.
12) Cluster Randomized Design
Meet the Cluster Randomized Design, the team captain of group-focused research. In our imaginary lineup of experimental designs, if other designs focus on individual players, then Cluster Randomized Design is looking at how the entire team functions.
This approach is especially common in educational and community-based research, and it's been gaining traction since the late 20th century.
Here's how Cluster Randomized Design works: Instead of assigning individual people to different conditions, researchers assign entire groups, or "clusters." These could be schools, neighborhoods, or even entire towns. This helps you see how the new method works in a real-world setting.
Imagine you want to see if a new anti-bullying program really works. Instead of selecting individual students, you'd introduce the program to a whole school or maybe even several schools, and then compare the results to schools without the program.
Cluster Randomized Design Pros
Why use Cluster Randomized Design? Well, sometimes it's just not practical to assign conditions at the individual level. For example, you can't really have half a school following a new reading program while the other half sticks with the old one; that would be way too confusing! Cluster Randomization helps get around this problem by treating each "cluster" as its own mini-experiment.
Cluster Randomized Design Cons
There's a downside, too. Because entire groups are assigned to each condition, there's a risk that the groups might be different in some important way that the researchers didn't account for. That's like having one sports team that's full of veterans playing against a team of rookies; the match wouldn't be fair.
Cluster Randomized Design Uses
A famous example is the research conducted to test the effectiveness of different public health interventions, like vaccination programs. Researchers might roll out a vaccination program in one community but not in another, then compare the rates of disease in both.
In our metaphorical research team, if True Experimental Design is the quarterback, Longitudinal Design is the wise elder, Factorial Design is the strategist, Cross-Sectional Design is the speedster, Correlational Design is the scout, Meta-Analysis is the coach, Non-Experimental Design is the journalist, Repeated Measures Design is the time traveler, and Crossover Design is the utility player, then Cluster Randomized Design is the team captain—always looking out for the group as a whole.
13) Mixed-Methods Design
Say hello to Mixed-Methods Design, the all-rounder or the "Renaissance player" of our research team.
Mixed-Methods Design uses a blend of both qualitative and quantitative methods to get a more complete picture, just like a Renaissance person who's good at lots of different things. It's like being good at both offense and defense in a sport; you've got all your bases covered!
Mixed-Methods Design is a fairly new kid on the block, becoming more popular in the late 20th and early 21st centuries as researchers began to see the value in using multiple approaches to tackle complex questions. It's the Swiss Army knife in our research toolkit, combining the best parts of other designs to be more versatile.
Here's how it could work: Imagine you're studying the effects of a new educational app on students' math skills. You might use quantitative methods like tests and grades to measure how much the students improve—that's the 'numbers part.'
But you also want to know how the students feel about math now, or why they think they got better or worse. For that, you could conduct interviews or have students fill out journals—that's the 'story part.'
Mixed-Methods Design Pros
So, what's the scoop on Mixed-Methods Design? The strength is its versatility and depth; you're not just getting numbers or stories, you're getting both, which gives a fuller picture.
Mixed-Methods Design Cons
But, it's also more challenging. Imagine trying to play two sports at the same time! You have to be skilled in different research methods and know how to combine them effectively.
Mixed-Methods Design Uses
A high-profile example of Mixed-Methods Design is research on climate change. Scientists use numbers and data to show temperature changes (quantitative), but they also interview people to understand how these changes are affecting communities (qualitative).
In our team of experimental designs, if True Experimental Design is the quarterback, Longitudinal Design is the wise elder, Factorial Design is the strategist, Cross-Sectional Design is the speedster, Correlational Design is the scout, Meta-Analysis is the coach, Non-Experimental Design is the journalist, Repeated Measures Design is the time traveler, Crossover Design is the utility player, and Cluster Randomized Design is the team captain, then Mixed-Methods Design is the Renaissance player—skilled in multiple areas and able to bring them all together for a winning strategy.
14) Multivariate Design
Now, let's turn our attention to Multivariate Design, the multitasker of the research world.
If our lineup of research designs were like players on a basketball court, Multivariate Design would be the player dribbling, passing, and shooting all at once. This design doesn't just look at one or two things; it looks at several variables simultaneously to see how they interact and affect each other.
Multivariate Design is like baking a cake with many ingredients. Instead of just looking at how flour affects the cake, you also consider sugar, eggs, and milk all at once. This way, you understand how everything works together to make the cake taste good or bad.
Multivariate Design has been a go-to method in psychology, economics, and social sciences since the latter half of the 20th century. With the advent of computers and advanced statistical software, analyzing multiple variables at once became a lot easier, and Multivariate Design soared in popularity.
Multivariate Design Pros
So, what's the benefit of using Multivariate Design? Its power lies in its complexity. By studying multiple variables at the same time, you can get a really rich, detailed understanding of what's going on.
Multivariate Design Cons
But that complexity can also be a drawback. With so many variables, it can be tough to tell which ones are really making a difference and which ones are just along for the ride.
Multivariate Design Uses
Imagine you're a coach trying to figure out the best strategy to win games. You wouldn't just look at how many points your star player scores; you'd also consider assists, rebounds, turnovers, and maybe even how loud the crowd is. A Multivariate Design would help you understand how all these factors work together to determine whether you win or lose.
A well-known example of Multivariate Design is in market research. Companies often use this approach to figure out how different factors—like price, packaging, and advertising—affect sales. By studying multiple variables at once, they can find the best combination to boost profits.
In our metaphorical research team, if True Experimental Design is the quarterback, Longitudinal Design is the wise elder, Factorial Design is the strategist, Cross-Sectional Design is the speedster, Correlational Design is the scout, Meta-Analysis is the coach, Non-Experimental Design is the journalist, Repeated Measures Design is the time traveler, Crossover Design is the utility player, Cluster Randomized Design is the team captain, and Mixed-Methods Design is the Renaissance player, then Multivariate Design is the multitasker—juggling many variables at once to get a fuller picture of what's happening.
15) Pretest-Posttest Design
Let's introduce Pretest-Posttest Design, the "Before and After" superstar of our research team. You've probably seen those before-and-after pictures in ads for weight loss programs or home renovations, right?
Well, this design is like that, but for science! Pretest-Posttest Design checks out what things are like before the experiment starts and then compares that to what things are like after the experiment ends.
This design is one of the classics, a staple in research for decades across various fields like psychology, education, and healthcare. It's so simple and straightforward that it has stayed popular for a long time.
In Pretest-Posttest Design, you measure your subject's behavior or condition before you introduce any changes—that's your "before" or "pretest." Then you do your experiment, and after it's done, you measure the same thing again—that's your "after" or "posttest."
Pretest-Posttest Design Pros
What makes Pretest-Posttest Design special? It's pretty easy to understand and doesn't require fancy statistics.
Pretest-Posttest Design Cons
But there are some pitfalls. For example, what if the kids in our math example get better at multiplication just because they're older or because they've taken the test before? That would make it hard to tell if the program is really effective or not.
Pretest-Posttest Design Uses
Let's say you're a teacher and you want to know if a new math program helps kids get better at multiplication. First, you'd give all the kids a multiplication test—that's your pretest. Then you'd teach them using the new math program. At the end, you'd give them the same test again—that's your posttest. If the kids do better on the second test, you might conclude that the program works.
One famous use of Pretest-Posttest Design is in evaluating the effectiveness of driver's education courses. Researchers will measure people's driving skills before and after the course to see if they've improved.
16) Solomon Four-Group Design
Next up is the Solomon Four-Group Design, the "chess master" of our research team. This design is all about strategy and careful planning. Named after Richard L. Solomon who introduced it in the 1940s, this method tries to correct some of the weaknesses in simpler designs, like the Pretest-Posttest Design.
Here's how it rolls: The Solomon Four-Group Design uses four different groups to test a hypothesis. Two groups get a pretest, then one of them receives the treatment or intervention, and both get a posttest. The other two groups skip the pretest, and only one of them receives the treatment before they both get a posttest.
Sound complicated? It's like playing 4D chess; you're thinking several moves ahead!
Solomon Four-Group Design Pros
What's the pro and con of the Solomon Four-Group Design? On the plus side, it provides really robust results because it accounts for so many variables.
Solomon Four-Group Design Cons
The downside? It's a lot of work and requires a lot of participants, making it more time-consuming and costly.
Solomon Four-Group Design Uses
Let's say you want to figure out if a new way of teaching history helps students remember facts better. Two classes take a history quiz (pretest), then one class uses the new teaching method while the other sticks with the old way. Both classes take another quiz afterward (posttest).
Meanwhile, two more classes skip the initial quiz, and then one uses the new method before both take the final quiz. Comparing all four groups will give you a much clearer picture of whether the new teaching method works and whether the pretest itself affects the outcome.
The Solomon Four-Group Design is less commonly used than simpler designs but is highly respected for its ability to control for more variables. It's a favorite in educational and psychological research where you really want to dig deep and figure out what's actually causing changes.
17) Adaptive Designs
Now, let's talk about Adaptive Designs, the chameleons of the experimental world.
Imagine you're a detective, and halfway through solving a case, you find a clue that changes everything. You wouldn't just stick to your old plan; you'd adapt and change your approach, right? That's exactly what Adaptive Designs allow researchers to do.
In an Adaptive Design, researchers can make changes to the study as it's happening, based on early results. In a traditional study, once you set your plan, you stick to it from start to finish.
Adaptive Design Pros
This method is particularly useful in fast-paced or high-stakes situations, like developing a new vaccine in the middle of a pandemic. The ability to adapt can save both time and resources, and more importantly, it can save lives by getting effective treatments out faster.
Adaptive Design Cons
But Adaptive Designs aren't without their drawbacks. They can be very complex to plan and carry out, and there's always a risk that the changes made during the study could introduce bias or errors.
Adaptive Design Uses
Adaptive Designs are most often seen in clinical trials, particularly in the medical and pharmaceutical fields.
For instance, if a new drug is showing really promising results, the study might be adjusted to give more participants the new treatment instead of a placebo. Or if one dose level is showing bad side effects, it might be dropped from the study.
The best part is, these changes are pre-planned. Researchers lay out in advance what changes might be made and under what conditions, which helps keep everything scientific and above board.
In terms of applications, besides their heavy usage in medical and pharmaceutical research, Adaptive Designs are also becoming increasingly popular in software testing and market research. In these fields, being able to quickly adjust to early results can give companies a significant advantage.
Adaptive Designs are like the agile startups of the research world—quick to pivot, keen to learn from ongoing results, and focused on rapid, efficient progress. However, they require a great deal of expertise and careful planning to ensure that the adaptability doesn't compromise the integrity of the research.
18) Bayesian Designs
Next, let's dive into Bayesian Designs, the data detectives of the research universe. Named after Thomas Bayes, an 18th-century statistician and minister, this design doesn't just look at what's happening now; it also takes into account what's happened before.
Imagine if you were a detective who not only looked at the evidence in front of you but also used your past cases to make better guesses about your current one. That's the essence of Bayesian Designs.
Bayesian Designs are like detective work in science. As you gather more clues (or data), you update your best guess on what's really happening. This way, your experiment gets smarter as it goes along.
In the world of research, Bayesian Designs are most notably used in areas where you have some prior knowledge that can inform your current study. For example, if earlier research shows that a certain type of medicine usually works well for a specific illness, a Bayesian Design would include that information when studying a new group of patients with the same illness.
Bayesian Design Pros
One of the major advantages of Bayesian Designs is their efficiency. Because they use existing data to inform the current experiment, often fewer resources are needed to reach a reliable conclusion.
Bayesian Design Cons
However, they can be quite complicated to set up and require a deep understanding of both statistics and the subject matter at hand.
Bayesian Design Uses
Bayesian Designs are highly valued in medical research, finance, environmental science, and even in Internet search algorithms. Their ability to continually update and refine hypotheses based on new evidence makes them particularly useful in fields where data is constantly evolving and where quick, informed decisions are crucial.
Here's a real-world example: In the development of personalized medicine, where treatments are tailored to individual patients, Bayesian Designs are invaluable. If a treatment has been effective for patients with similar genetics or symptoms in the past, a Bayesian approach can use that data to predict how well it might work for a new patient.
This type of design is also increasingly popular in machine learning and artificial intelligence. In these fields, Bayesian Designs help algorithms "learn" from past data to make better predictions or decisions in new situations. It's like teaching a computer to be a detective that gets better and better at solving puzzles the more puzzles it sees.
19) Covariate Adaptive Randomization
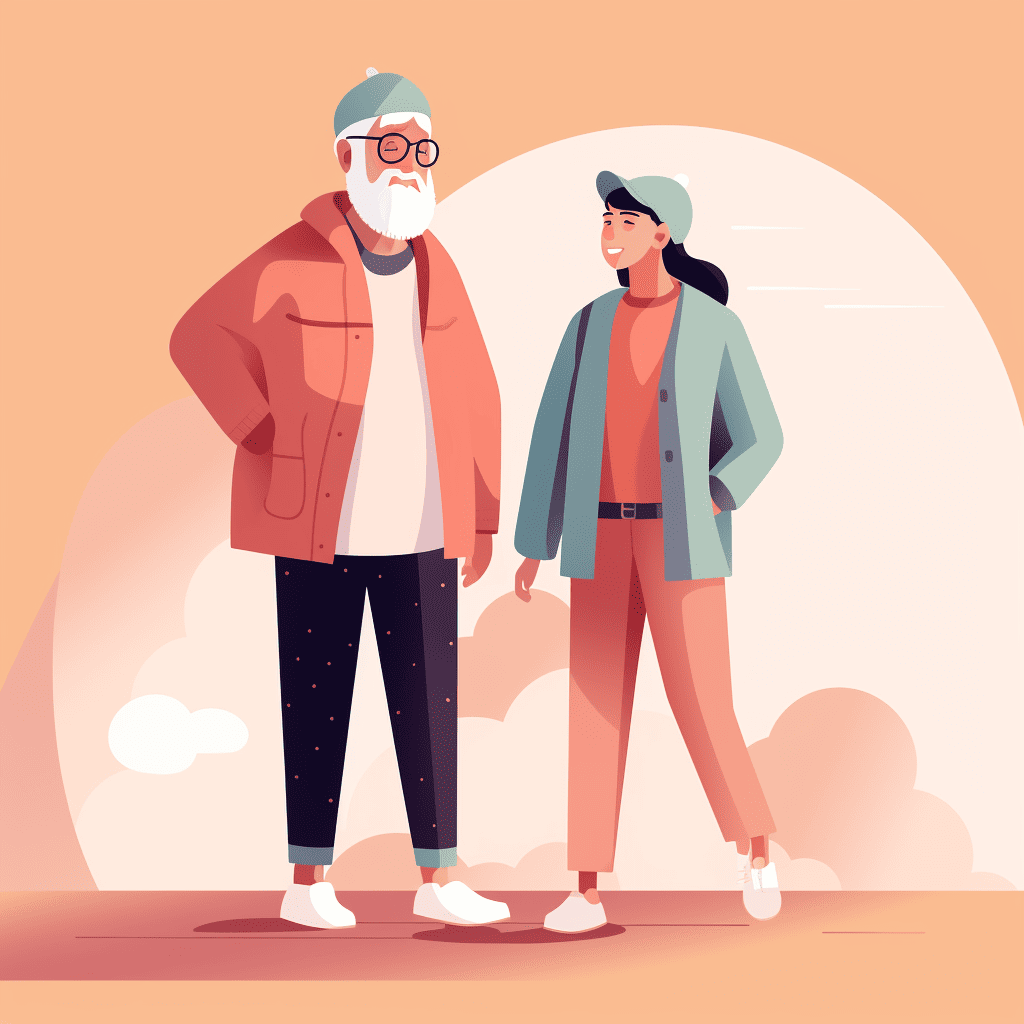
Now let's turn our attention to Covariate Adaptive Randomization, which you can think of as the "matchmaker" of experimental designs.
Picture a soccer coach trying to create the most balanced teams for a friendly match. They wouldn't just randomly assign players; they'd take into account each player's skills, experience, and other traits.
Covariate Adaptive Randomization is all about creating the most evenly matched groups possible for an experiment.
In traditional randomization, participants are allocated to different groups purely by chance. This is a pretty fair way to do things, but it can sometimes lead to unbalanced groups.
Imagine if all the professional-level players ended up on one soccer team and all the beginners on another; that wouldn't be a very informative match! Covariate Adaptive Randomization fixes this by using important traits or characteristics (called "covariates") to guide the randomization process.
Covariate Adaptive Randomization Pros
The benefits of this design are pretty clear: it aims for balance and fairness, making the final results more trustworthy.
Covariate Adaptive Randomization Cons
But it's not perfect. It can be complex to implement and requires a deep understanding of which characteristics are most important to balance.
Covariate Adaptive Randomization Uses
This design is particularly useful in medical trials. Let's say researchers are testing a new medication for high blood pressure. Participants might have different ages, weights, or pre-existing conditions that could affect the results.
Covariate Adaptive Randomization would make sure that each treatment group has a similar mix of these characteristics, making the results more reliable and easier to interpret.
In practical terms, this design is often seen in clinical trials for new drugs or therapies, but its principles are also applicable in fields like psychology, education, and social sciences.
For instance, in educational research, it might be used to ensure that classrooms being compared have similar distributions of students in terms of academic ability, socioeconomic status, and other factors.
Covariate Adaptive Randomization is like the wise elder of the group, ensuring that everyone has an equal opportunity to show their true capabilities, thereby making the collective results as reliable as possible.
20) Stepped Wedge Design
Let's now focus on the Stepped Wedge Design, a thoughtful and cautious member of the experimental design family.
Imagine you're trying out a new gardening technique, but you're not sure how well it will work. You decide to apply it to one section of your garden first, watch how it performs, and then gradually extend the technique to other sections. This way, you get to see its effects over time and across different conditions. That's basically how Stepped Wedge Design works.
In a Stepped Wedge Design, all participants or clusters start off in the control group, and then, at different times, they 'step' over to the intervention or treatment group. This creates a wedge-like pattern over time where more and more participants receive the treatment as the study progresses. It's like rolling out a new policy in phases, monitoring its impact at each stage before extending it to more people.
Stepped Wedge Design Pros
The Stepped Wedge Design offers several advantages. Firstly, it allows for the study of interventions that are expected to do more good than harm, which makes it ethically appealing.
Secondly, it's useful when resources are limited and it's not feasible to roll out a new treatment to everyone at once. Lastly, because everyone eventually receives the treatment, it can be easier to get buy-in from participants or organizations involved in the study.
Stepped Wedge Design Cons
However, this design can be complex to analyze because it has to account for both the time factor and the changing conditions in each 'step' of the wedge. And like any study where participants know they're receiving an intervention, there's the potential for the results to be influenced by the placebo effect or other biases.
Stepped Wedge Design Uses
This design is particularly useful in health and social care research. For instance, if a hospital wants to implement a new hygiene protocol, it might start in one department, assess its impact, and then roll it out to other departments over time. This allows the hospital to adjust and refine the new protocol based on real-world data before it's fully implemented.
In terms of applications, Stepped Wedge Designs are commonly used in public health initiatives, organizational changes in healthcare settings, and social policy trials. They are particularly useful in situations where an intervention is being rolled out gradually and it's important to understand its impacts at each stage.
21) Sequential Design
Next up is Sequential Design, the dynamic and flexible member of our experimental design family.
Imagine you're playing a video game where you can choose different paths. If you take one path and find a treasure chest, you might decide to continue in that direction. If you hit a dead end, you might backtrack and try a different route. Sequential Design operates in a similar fashion, allowing researchers to make decisions at different stages based on what they've learned so far.
In a Sequential Design, the experiment is broken down into smaller parts, or "sequences." After each sequence, researchers pause to look at the data they've collected. Based on those findings, they then decide whether to stop the experiment because they've got enough information, or to continue and perhaps even modify the next sequence.
Sequential Design Pros
This allows for a more efficient use of resources, as you're only continuing with the experiment if the data suggests it's worth doing so.
One of the great things about Sequential Design is its efficiency. Because you're making data-driven decisions along the way, you can often reach conclusions more quickly and with fewer resources.
Sequential Design Cons
However, it requires careful planning and expertise to ensure that these "stop or go" decisions are made correctly and without bias.
Sequential Design Uses
In terms of its applications, besides healthcare and medicine, Sequential Design is also popular in quality control in manufacturing, environmental monitoring, and financial modeling. In these areas, being able to make quick decisions based on incoming data can be a big advantage.
This design is often used in clinical trials involving new medications or treatments. For example, if early results show that a new drug has significant side effects, the trial can be stopped before more people are exposed to it.
On the flip side, if the drug is showing promising results, the trial might be expanded to include more participants or to extend the testing period.
Think of Sequential Design as the nimble athlete of experimental designs, capable of quick pivots and adjustments to reach the finish line in the most effective way possible. But just like an athlete needs a good coach, this design requires expert oversight to make sure it stays on the right track.
22) Field Experiments
Last but certainly not least, let's explore Field Experiments—the adventurers of the experimental design world.
Picture a scientist leaving the controlled environment of a lab to test a theory in the real world, like a biologist studying animals in their natural habitat or a social scientist observing people in a real community. These are Field Experiments, and they're all about getting out there and gathering data in real-world settings.
Field Experiments embrace the messiness of the real world, unlike laboratory experiments, where everything is controlled down to the smallest detail. This makes them both exciting and challenging.
Field Experiment Pros
On one hand, the results often give us a better understanding of how things work outside the lab.
While Field Experiments offer real-world relevance, they come with challenges like controlling for outside factors and the ethical considerations of intervening in people's lives without their knowledge.
Field Experiment Cons
On the other hand, the lack of control can make it harder to tell exactly what's causing what. Yet, despite these challenges, they remain a valuable tool for researchers who want to understand how theories play out in the real world.
Field Experiment Uses
Let's say a school wants to improve student performance. In a Field Experiment, they might change the school's daily schedule for one semester and keep track of how students perform compared to another school where the schedule remained the same.
Because the study is happening in a real school with real students, the results could be very useful for understanding how the change might work in other schools. But since it's the real world, lots of other factors—like changes in teachers or even the weather—could affect the results.
Field Experiments are widely used in economics, psychology, education, and public policy. For example, you might have heard of the famous "Broken Windows" experiment in the 1980s that looked at how small signs of disorder, like broken windows or graffiti, could encourage more serious crime in neighborhoods. This experiment had a big impact on how cities think about crime prevention.
From the foundational concepts of control groups and independent variables to the sophisticated layouts like Covariate Adaptive Randomization and Sequential Design, it's clear that the realm of experimental design is as varied as it is fascinating.
We've seen that each design has its own special talents, ideal for specific situations. Some designs, like the Classic Controlled Experiment, are like reliable old friends you can always count on.
Others, like Sequential Design, are flexible and adaptable, making quick changes based on what they learn. And let's not forget the adventurous Field Experiments, which take us out of the lab and into the real world to discover things we might not see otherwise.
Choosing the right experimental design is like picking the right tool for the job. The method you choose can make a big difference in how reliable your results are and how much people will trust what you've discovered. And as we've learned, there's a design to suit just about every question, every problem, and every curiosity.
So the next time you read about a new discovery in medicine, psychology, or any other field, you'll have a better understanding of the thought and planning that went into figuring things out. Experimental design is more than just a set of rules; it's a structured way to explore the unknown and answer questions that can change the world.
Related posts:
- Experimental Psychologist Career (Salary + Duties + Interviews)
- 40+ Famous Psychologists (Images + Biographies)
- 11+ Psychology Experiment Ideas (Goals + Methods)
- The Little Albert Experiment
- 41+ White Collar Job Examples (Salary + Path)
Reference this article:
About The Author
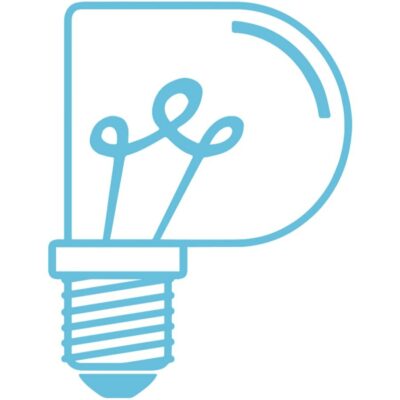
Free Personality Test
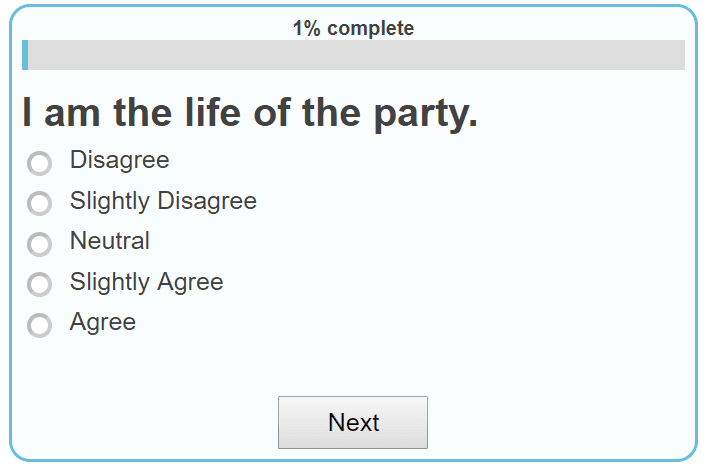
Free Memory Test
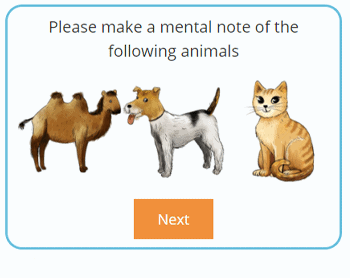
Free IQ Test
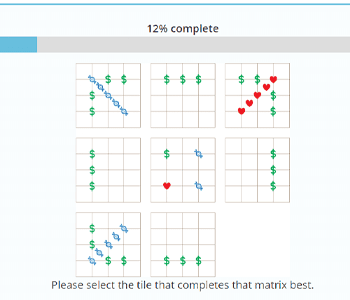
PracticalPie.com is a participant in the Amazon Associates Program. As an Amazon Associate we earn from qualifying purchases.
Follow Us On:
Youtube Facebook Instagram X/Twitter
Psychology Resources
Developmental
Personality
Relationships
Psychologists
Serial Killers
Psychology Tests
Personality Quiz
Memory Test
Depression test
Type A/B Personality Test
© PracticalPsychology. All rights reserved
Privacy Policy | Terms of Use
User Preferences
Content preview.
Arcu felis bibendum ut tristique et egestas quis:
- Ut enim ad minim veniam, quis nostrud exercitation ullamco laboris
- Duis aute irure dolor in reprehenderit in voluptate
- Excepteur sint occaecat cupidatat non proident
Keyboard Shortcuts
1.3 - steps for planning, conducting and analyzing an experiment.
The practical steps needed for planning and conducting an experiment include: recognizing the goal of the experiment, choice of factors, choice of response, choice of the design, analysis and then drawing conclusions. This pretty much covers the steps involved in the scientific method.
- Recognition and statement of the problem
- Choice of factors, levels, and ranges
- Selection of the response variable(s)
- Choice of design
- Conducting the experiment
- Statistical analysis
- Drawing conclusions, and making recommendations
What this course will deal with primarily is the choice of the design. This focus includes all the related issues about how we handle these factors in conducting our experiments.
Factors Section
We usually talk about "treatment" factors, which are the factors of primary interest to you. In addition to treatment factors, there are nuisance factors which are not your primary focus, but you have to deal with them. Sometimes these are called blocking factors, mainly because we will try to block on these factors to prevent them from influencing the results.
There are other ways that we can categorize factors:
Experimental vs. Classification Factors
Quantitative vs. qualitative factors, try it section .
Think about your own field of study and jot down several of the factors that are pertinent in your own research area? Into what categories do these fall?
Get statistical thinking involved early when you are preparing to design an experiment! Getting well into an experiment before you have considered these implications can be disastrous. Think and experiment sequentially. Experimentation is a process where what you know informs the design of the next experiment, and what you learn from it becomes the knowledge base to design the next.
- Privacy Policy

Home » Experimental Design – Types, Methods, Guide
Experimental Design – Types, Methods, Guide
Table of Contents

Experimental Design
Experimental design is a process of planning and conducting scientific experiments to investigate a hypothesis or research question. It involves carefully designing an experiment that can test the hypothesis, and controlling for other variables that may influence the results.
Experimental design typically includes identifying the variables that will be manipulated or measured, defining the sample or population to be studied, selecting an appropriate method of sampling, choosing a method for data collection and analysis, and determining the appropriate statistical tests to use.
Types of Experimental Design
Here are the different types of experimental design:

Completely Randomized Design
In this design, participants are randomly assigned to one of two or more groups, and each group is exposed to a different treatment or condition.
Randomized Block Design
This design involves dividing participants into blocks based on a specific characteristic, such as age or gender, and then randomly assigning participants within each block to one of two or more treatment groups.
Factorial Design
In a factorial design, participants are randomly assigned to one of several groups, each of which receives a different combination of two or more independent variables.
Repeated Measures Design
In this design, each participant is exposed to all of the different treatments or conditions, either in a random order or in a predetermined order.
Crossover Design
This design involves randomly assigning participants to one of two or more treatment groups, with each group receiving one treatment during the first phase of the study and then switching to a different treatment during the second phase.
Split-plot Design
In this design, the researcher manipulates one or more variables at different levels and uses a randomized block design to control for other variables.
Nested Design
This design involves grouping participants within larger units, such as schools or households, and then randomly assigning these units to different treatment groups.
Laboratory Experiment
Laboratory experiments are conducted under controlled conditions, which allows for greater precision and accuracy. However, because laboratory conditions are not always representative of real-world conditions, the results of these experiments may not be generalizable to the population at large.
Field Experiment
Field experiments are conducted in naturalistic settings and allow for more realistic observations. However, because field experiments are not as controlled as laboratory experiments, they may be subject to more sources of error.
Experimental Design Methods
Experimental design methods refer to the techniques and procedures used to design and conduct experiments in scientific research. Here are some common experimental design methods:
Randomization
This involves randomly assigning participants to different groups or treatments to ensure that any observed differences between groups are due to the treatment and not to other factors.
Control Group
The use of a control group is an important experimental design method that involves having a group of participants that do not receive the treatment or intervention being studied. The control group is used as a baseline to compare the effects of the treatment group.
Blinding involves keeping participants, researchers, or both unaware of which treatment group participants are in, in order to reduce the risk of bias in the results.
Counterbalancing
This involves systematically varying the order in which participants receive treatments or interventions in order to control for order effects.
Replication
Replication involves conducting the same experiment with different samples or under different conditions to increase the reliability and validity of the results.
This experimental design method involves manipulating multiple independent variables simultaneously to investigate their combined effects on the dependent variable.
This involves dividing participants into subgroups or blocks based on specific characteristics, such as age or gender, in order to reduce the risk of confounding variables.
Data Collection Method
Experimental design data collection methods are techniques and procedures used to collect data in experimental research. Here are some common experimental design data collection methods:
Direct Observation
This method involves observing and recording the behavior or phenomenon of interest in real time. It may involve the use of structured or unstructured observation, and may be conducted in a laboratory or naturalistic setting.
Self-report Measures
Self-report measures involve asking participants to report their thoughts, feelings, or behaviors using questionnaires, surveys, or interviews. These measures may be administered in person or online.
Behavioral Measures
Behavioral measures involve measuring participants’ behavior directly, such as through reaction time tasks or performance tests. These measures may be administered using specialized equipment or software.
Physiological Measures
Physiological measures involve measuring participants’ physiological responses, such as heart rate, blood pressure, or brain activity, using specialized equipment. These measures may be invasive or non-invasive, and may be administered in a laboratory or clinical setting.
Archival Data
Archival data involves using existing records or data, such as medical records, administrative records, or historical documents, as a source of information. These data may be collected from public or private sources.
Computerized Measures
Computerized measures involve using software or computer programs to collect data on participants’ behavior or responses. These measures may include reaction time tasks, cognitive tests, or other types of computer-based assessments.
Video Recording
Video recording involves recording participants’ behavior or interactions using cameras or other recording equipment. This method can be used to capture detailed information about participants’ behavior or to analyze social interactions.
Data Analysis Method
Experimental design data analysis methods refer to the statistical techniques and procedures used to analyze data collected in experimental research. Here are some common experimental design data analysis methods:
Descriptive Statistics
Descriptive statistics are used to summarize and describe the data collected in the study. This includes measures such as mean, median, mode, range, and standard deviation.
Inferential Statistics
Inferential statistics are used to make inferences or generalizations about a larger population based on the data collected in the study. This includes hypothesis testing and estimation.
Analysis of Variance (ANOVA)
ANOVA is a statistical technique used to compare means across two or more groups in order to determine whether there are significant differences between the groups. There are several types of ANOVA, including one-way ANOVA, two-way ANOVA, and repeated measures ANOVA.
Regression Analysis
Regression analysis is used to model the relationship between two or more variables in order to determine the strength and direction of the relationship. There are several types of regression analysis, including linear regression, logistic regression, and multiple regression.
Factor Analysis
Factor analysis is used to identify underlying factors or dimensions in a set of variables. This can be used to reduce the complexity of the data and identify patterns in the data.
Structural Equation Modeling (SEM)
SEM is a statistical technique used to model complex relationships between variables. It can be used to test complex theories and models of causality.
Cluster Analysis
Cluster analysis is used to group similar cases or observations together based on similarities or differences in their characteristics.
Time Series Analysis
Time series analysis is used to analyze data collected over time in order to identify trends, patterns, or changes in the data.
Multilevel Modeling
Multilevel modeling is used to analyze data that is nested within multiple levels, such as students nested within schools or employees nested within companies.
Applications of Experimental Design
Experimental design is a versatile research methodology that can be applied in many fields. Here are some applications of experimental design:
- Medical Research: Experimental design is commonly used to test new treatments or medications for various medical conditions. This includes clinical trials to evaluate the safety and effectiveness of new drugs or medical devices.
- Agriculture : Experimental design is used to test new crop varieties, fertilizers, and other agricultural practices. This includes randomized field trials to evaluate the effects of different treatments on crop yield, quality, and pest resistance.
- Environmental science: Experimental design is used to study the effects of environmental factors, such as pollution or climate change, on ecosystems and wildlife. This includes controlled experiments to study the effects of pollutants on plant growth or animal behavior.
- Psychology : Experimental design is used to study human behavior and cognitive processes. This includes experiments to test the effects of different interventions, such as therapy or medication, on mental health outcomes.
- Engineering : Experimental design is used to test new materials, designs, and manufacturing processes in engineering applications. This includes laboratory experiments to test the strength and durability of new materials, or field experiments to test the performance of new technologies.
- Education : Experimental design is used to evaluate the effectiveness of teaching methods, educational interventions, and programs. This includes randomized controlled trials to compare different teaching methods or evaluate the impact of educational programs on student outcomes.
- Marketing : Experimental design is used to test the effectiveness of marketing campaigns, pricing strategies, and product designs. This includes experiments to test the impact of different marketing messages or pricing schemes on consumer behavior.
Examples of Experimental Design
Here are some examples of experimental design in different fields:
- Example in Medical research : A study that investigates the effectiveness of a new drug treatment for a particular condition. Patients are randomly assigned to either a treatment group or a control group, with the treatment group receiving the new drug and the control group receiving a placebo. The outcomes, such as improvement in symptoms or side effects, are measured and compared between the two groups.
- Example in Education research: A study that examines the impact of a new teaching method on student learning outcomes. Students are randomly assigned to either a group that receives the new teaching method or a group that receives the traditional teaching method. Student achievement is measured before and after the intervention, and the results are compared between the two groups.
- Example in Environmental science: A study that tests the effectiveness of a new method for reducing pollution in a river. Two sections of the river are selected, with one section treated with the new method and the other section left untreated. The water quality is measured before and after the intervention, and the results are compared between the two sections.
- Example in Marketing research: A study that investigates the impact of a new advertising campaign on consumer behavior. Participants are randomly assigned to either a group that is exposed to the new campaign or a group that is not. Their behavior, such as purchasing or product awareness, is measured and compared between the two groups.
- Example in Social psychology: A study that examines the effect of a new social intervention on reducing prejudice towards a marginalized group. Participants are randomly assigned to either a group that receives the intervention or a control group that does not. Their attitudes and behavior towards the marginalized group are measured before and after the intervention, and the results are compared between the two groups.
When to use Experimental Research Design
Experimental research design should be used when a researcher wants to establish a cause-and-effect relationship between variables. It is particularly useful when studying the impact of an intervention or treatment on a particular outcome.
Here are some situations where experimental research design may be appropriate:
- When studying the effects of a new drug or medical treatment: Experimental research design is commonly used in medical research to test the effectiveness and safety of new drugs or medical treatments. By randomly assigning patients to treatment and control groups, researchers can determine whether the treatment is effective in improving health outcomes.
- When evaluating the effectiveness of an educational intervention: An experimental research design can be used to evaluate the impact of a new teaching method or educational program on student learning outcomes. By randomly assigning students to treatment and control groups, researchers can determine whether the intervention is effective in improving academic performance.
- When testing the effectiveness of a marketing campaign: An experimental research design can be used to test the effectiveness of different marketing messages or strategies. By randomly assigning participants to treatment and control groups, researchers can determine whether the marketing campaign is effective in changing consumer behavior.
- When studying the effects of an environmental intervention: Experimental research design can be used to study the impact of environmental interventions, such as pollution reduction programs or conservation efforts. By randomly assigning locations or areas to treatment and control groups, researchers can determine whether the intervention is effective in improving environmental outcomes.
- When testing the effects of a new technology: An experimental research design can be used to test the effectiveness and safety of new technologies or engineering designs. By randomly assigning participants or locations to treatment and control groups, researchers can determine whether the new technology is effective in achieving its intended purpose.
How to Conduct Experimental Research
Here are the steps to conduct Experimental Research:
- Identify a Research Question : Start by identifying a research question that you want to answer through the experiment. The question should be clear, specific, and testable.
- Develop a Hypothesis: Based on your research question, develop a hypothesis that predicts the relationship between the independent and dependent variables. The hypothesis should be clear and testable.
- Design the Experiment : Determine the type of experimental design you will use, such as a between-subjects design or a within-subjects design. Also, decide on the experimental conditions, such as the number of independent variables, the levels of the independent variable, and the dependent variable to be measured.
- Select Participants: Select the participants who will take part in the experiment. They should be representative of the population you are interested in studying.
- Randomly Assign Participants to Groups: If you are using a between-subjects design, randomly assign participants to groups to control for individual differences.
- Conduct the Experiment : Conduct the experiment by manipulating the independent variable(s) and measuring the dependent variable(s) across the different conditions.
- Analyze the Data: Analyze the data using appropriate statistical methods to determine if there is a significant effect of the independent variable(s) on the dependent variable(s).
- Draw Conclusions: Based on the data analysis, draw conclusions about the relationship between the independent and dependent variables. If the results support the hypothesis, then it is accepted. If the results do not support the hypothesis, then it is rejected.
- Communicate the Results: Finally, communicate the results of the experiment through a research report or presentation. Include the purpose of the study, the methods used, the results obtained, and the conclusions drawn.
Purpose of Experimental Design
The purpose of experimental design is to control and manipulate one or more independent variables to determine their effect on a dependent variable. Experimental design allows researchers to systematically investigate causal relationships between variables, and to establish cause-and-effect relationships between the independent and dependent variables. Through experimental design, researchers can test hypotheses and make inferences about the population from which the sample was drawn.
Experimental design provides a structured approach to designing and conducting experiments, ensuring that the results are reliable and valid. By carefully controlling for extraneous variables that may affect the outcome of the study, experimental design allows researchers to isolate the effect of the independent variable(s) on the dependent variable(s), and to minimize the influence of other factors that may confound the results.
Experimental design also allows researchers to generalize their findings to the larger population from which the sample was drawn. By randomly selecting participants and using statistical techniques to analyze the data, researchers can make inferences about the larger population with a high degree of confidence.
Overall, the purpose of experimental design is to provide a rigorous, systematic, and scientific method for testing hypotheses and establishing cause-and-effect relationships between variables. Experimental design is a powerful tool for advancing scientific knowledge and informing evidence-based practice in various fields, including psychology, biology, medicine, engineering, and social sciences.
Advantages of Experimental Design
Experimental design offers several advantages in research. Here are some of the main advantages:
- Control over extraneous variables: Experimental design allows researchers to control for extraneous variables that may affect the outcome of the study. By manipulating the independent variable and holding all other variables constant, researchers can isolate the effect of the independent variable on the dependent variable.
- Establishing causality: Experimental design allows researchers to establish causality by manipulating the independent variable and observing its effect on the dependent variable. This allows researchers to determine whether changes in the independent variable cause changes in the dependent variable.
- Replication : Experimental design allows researchers to replicate their experiments to ensure that the findings are consistent and reliable. Replication is important for establishing the validity and generalizability of the findings.
- Random assignment: Experimental design often involves randomly assigning participants to conditions. This helps to ensure that individual differences between participants are evenly distributed across conditions, which increases the internal validity of the study.
- Precision : Experimental design allows researchers to measure variables with precision, which can increase the accuracy and reliability of the data.
- Generalizability : If the study is well-designed, experimental design can increase the generalizability of the findings. By controlling for extraneous variables and using random assignment, researchers can increase the likelihood that the findings will apply to other populations and contexts.
Limitations of Experimental Design
Experimental design has some limitations that researchers should be aware of. Here are some of the main limitations:
- Artificiality : Experimental design often involves creating artificial situations that may not reflect real-world situations. This can limit the external validity of the findings, or the extent to which the findings can be generalized to real-world settings.
- Ethical concerns: Some experimental designs may raise ethical concerns, particularly if they involve manipulating variables that could cause harm to participants or if they involve deception.
- Participant bias : Participants in experimental studies may modify their behavior in response to the experiment, which can lead to participant bias.
- Limited generalizability: The conditions of the experiment may not reflect the complexities of real-world situations. As a result, the findings may not be applicable to all populations and contexts.
- Cost and time : Experimental design can be expensive and time-consuming, particularly if the experiment requires specialized equipment or if the sample size is large.
- Researcher bias : Researchers may unintentionally bias the results of the experiment if they have expectations or preferences for certain outcomes.
- Lack of feasibility : Experimental design may not be feasible in some cases, particularly if the research question involves variables that cannot be manipulated or controlled.
About the author
Muhammad Hassan
Researcher, Academic Writer, Web developer
You may also like

Textual Analysis – Types, Examples and Guide

Explanatory Research – Types, Methods, Guide

Observational Research – Methods and Guide

Quantitative Research – Methods, Types and...

Qualitative Research Methods

Exploratory Research – Types, Methods and...
All Subjects
study guides for every class
That actually explain what's on your next test, experimental setup, from class:, ap psychology.
An experimental setup refers to the specific arrangement and conditions in which an experiment is conducted to investigate a hypothesis or research question. It involves manipulating independent variables, measuring dependent variables, and controlling extraneous factors.
Related terms
Control Group : A control group is a group in an experiment that does not receive the treatment or manipulation being tested. It serves as a baseline for comparison with the experimental group.
Independent Variable : The independent variable is the factor that researchers deliberately manipulate or change in an experiment to observe its effect on the dependent variable.
Dependent Variable : The dependent variable is the outcome or response that researchers measure or observe in an experiment. Its value depends on changes made to the independent variable.
" Experimental Setup " also found in:
Subjects ( 5 ).
- Mathematical Crystallography
- Plasma Medicine
- Plasma-assisted Manufacturing
- Statistical Mechanics
- Terahertz Engineering
Practice Questions ( 6 )
- What experimental setup could be used to explore the impact of stress on academic performance?
- If you want to examine whether neurogenesis affects memory retention, which experimental setup would be most effective?
- Which experimental setup would effectively illustrate the concept of shaping in operant conditioning?
- Which experimental setup would be most suitable for evaluating the effect of sensorimotor activities on cognitive development in toddlers?
- What experimental setup could investigate the relationship between self-efficacy and goal-setting among college students?
- Which experimental setup could effectively examine the role of peer pressure in altering teenage smoking attitudes?
© 2024 Fiveable Inc. All rights reserved.
Ap® and sat® are trademarks registered by the college board, which is not affiliated with, and does not endorse this website..
Experimentation in Scientific Research: Variables and controls in practice
by Anthony Carpi, Ph.D., Anne E. Egger, Ph.D.
Listen to this reading
Did you know that experimental design was developed more than a thousand years ago by a Middle Eastern scientist who studied light? All of us use a form of experimental research in our day to day lives when we try to find the spot with the best cell phone reception, try out new cooking recipes, and more. Scientific experiments are built on similar principles.
Experimentation is a research method in which one or more variables are consciously manipulated and the outcome or effect of that manipulation on other variables is observed.
Experimental designs often make use of controls that provide a measure of variability within a system and a check for sources of error.
Experimental methods are commonly applied to determine causal relationships or to quantify the magnitude of response of a variable.
Anyone who has used a cellular phone knows that certain situations require a bit of research: If you suddenly find yourself in an area with poor phone reception, you might move a bit to the left or right, walk a few steps forward or back, or even hold the phone over your head to get a better signal. While the actions of a cell phone user might seem obvious, the person seeking cell phone reception is actually performing a scientific experiment: consciously manipulating one component (the location of the cell phone) and observing the effect of that action on another component (the phone's reception). Scientific experiments are obviously a bit more complicated, and generally involve more rigorous use of controls , but they draw on the same type of reasoning that we use in many everyday situations. In fact, the earliest documented scientific experiments were devised to answer a very common everyday question: how vision works.
- A brief history of experimental methods
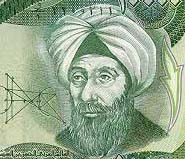
Figure 1: Alhazen (965-ca.1039) as pictured on an Iraqi 10,000-dinar note
One of the first ideas regarding how human vision works came from the Greek philosopher Empedocles around 450 BCE . Empedocles reasoned that the Greek goddess Aphrodite had lit a fire in the human eye, and vision was possible because light rays from this fire emanated from the eye, illuminating objects around us. While a number of people challenged this proposal, the idea that light radiated from the human eye proved surprisingly persistent until around 1,000 CE , when a Middle Eastern scientist advanced our knowledge of the nature of light and, in so doing, developed a new and more rigorous approach to scientific research . Abū 'Alī al-Hasan ibn al-Hasan ibn al-Haytham, also known as Alhazen , was born in 965 CE in the Arabian city of Basra in what is present-day Iraq. He began his scientific studies in physics, mathematics, and other sciences after reading the works of several Greek philosophers. One of Alhazen's most significant contributions was a seven-volume work on optics titled Kitab al-Manazir (later translated to Latin as Opticae Thesaurus Alhazeni – Alhazen's Book of Optics ). Beyond the contributions this book made to the field of optics, it was a remarkable work in that it based conclusions on experimental evidence rather than abstract reasoning – the first major publication to do so. Alhazen's contributions have proved so significant that his likeness was immortalized on the 2003 10,000-dinar note issued by Iraq (Figure 1).
Alhazen invested significant time studying light , color, shadows, rainbows, and other optical phenomena. Among this work was a study in which he stood in a darkened room with a small hole in one wall. Outside of the room, he hung two lanterns at different heights. Alhazen observed that the light from each lantern illuminated a different spot in the room, and each lighted spot formed a direct line with the hole and one of the lanterns outside the room. He also found that covering a lantern caused the spot it illuminated to darken, and exposing the lantern caused the spot to reappear. Thus, Alhazen provided some of the first experimental evidence that light does not emanate from the human eye but rather is emitted by certain objects (like lanterns) and travels from these objects in straight lines. Alhazen's experiment may seem simplistic today, but his methodology was groundbreaking: He developed a hypothesis based on observations of physical relationships (that light comes from objects), and then designed an experiment to test that hypothesis. Despite the simplicity of the method , Alhazen's experiment was a critical step in refuting the long-standing theory that light emanated from the human eye, and it was a major event in the development of modern scientific research methodology.
Comprehension Checkpoint
- Experimentation as a scientific research method
Experimentation is one scientific research method , perhaps the most recognizable, in a spectrum of methods that also includes description, comparison, and modeling (see our Description , Comparison , and Modeling modules). While all of these methods share in common a scientific approach, experimentation is unique in that it involves the conscious manipulation of certain aspects of a real system and the observation of the effects of that manipulation. You could solve a cell phone reception problem by walking around a neighborhood until you see a cell phone tower, observing other cell phone users to see where those people who get the best reception are standing, or looking on the web for a map of cell phone signal coverage. All of these methods could also provide answers, but by moving around and testing reception yourself, you are experimenting.
- Variables: Independent and dependent
In the experimental method , a condition or a parameter , generally referred to as a variable , is consciously manipulated (often referred to as a treatment) and the outcome or effect of that manipulation is observed on other variables. Variables are given different names depending on whether they are the ones manipulated or the ones observed:
- Independent variable refers to a condition within an experiment that is manipulated by the scientist.
- Dependent variable refers to an event or outcome of an experiment that might be affected by the manipulation of the independent variable .
Scientific experimentation helps to determine the nature of the relationship between independent and dependent variables . While it is often difficult, or sometimes impossible, to manipulate a single variable in an experiment , scientists often work to minimize the number of variables being manipulated. For example, as we move from one location to another to get better cell reception, we likely change the orientation of our body, perhaps from south-facing to east-facing, or we hold the cell phone at a different angle. Which variable affected reception: location, orientation, or angle of the phone? It is critical that scientists understand which aspects of their experiment they are manipulating so that they can accurately determine the impacts of that manipulation . In order to constrain the possible outcomes of an experimental procedure, most scientific experiments use a system of controls .
- Controls: Negative, positive, and placebos
In a controlled study, a scientist essentially runs two (or more) parallel and simultaneous experiments: a treatment group, in which the effect of an experimental manipulation is observed on a dependent variable , and a control group, which uses all of the same conditions as the first with the exception of the actual treatment. Controls can fall into one of two groups: negative controls and positive controls .
In a negative control , the control group is exposed to all of the experimental conditions except for the actual treatment . The need to match all experimental conditions exactly is so great that, for example, in a trial for a new drug, the negative control group will be given a pill or liquid that looks exactly like the drug, except that it will not contain the drug itself, a control often referred to as a placebo . Negative controls allow scientists to measure the natural variability of the dependent variable(s), provide a means of measuring error in the experiment , and also provide a baseline to measure against the experimental treatment.
Some experimental designs also make use of positive controls . A positive control is run as a parallel experiment and generally involves the use of an alternative treatment that the researcher knows will have an effect on the dependent variable . For example, when testing the effectiveness of a new drug for pain relief, a scientist might administer treatment placebo to one group of patients as a negative control , and a known treatment like aspirin to a separate group of individuals as a positive control since the pain-relieving aspects of aspirin are well documented. In both cases, the controls allow scientists to quantify background variability and reject alternative hypotheses that might otherwise explain the effect of the treatment on the dependent variable .
- Experimentation in practice: The case of Louis Pasteur
Well-controlled experiments generally provide strong evidence of causality, demonstrating whether the manipulation of one variable causes a response in another variable. For example, as early as the 6th century BCE , Anaximander , a Greek philosopher, speculated that life could be formed from a mixture of sea water, mud, and sunlight. The idea probably stemmed from the observation of worms, mosquitoes, and other insects "magically" appearing in mudflats and other shallow areas. While the suggestion was challenged on a number of occasions, the idea that living microorganisms could be spontaneously generated from air persisted until the middle of the 18 th century.
In the 1750s, John Needham, a Scottish clergyman and naturalist, claimed to have proved that spontaneous generation does occur when he showed that microorganisms flourished in certain foods such as soup broth, even after they had been briefly boiled and covered. Several years later, the Italian abbot and biologist Lazzaro Spallanzani , boiled soup broth for over an hour and then placed bowls of this soup in different conditions, sealing some and leaving others exposed to air. Spallanzani found that microorganisms grew in the soup exposed to air but were absent from the sealed soup. He therefore challenged Needham's conclusions and hypothesized that microorganisms suspended in air settled onto the exposed soup but not the sealed soup, and rejected the idea of spontaneous generation .
Needham countered, arguing that the growth of bacteria in the soup was not due to microbes settling onto the soup from the air, but rather because spontaneous generation required contact with an intangible "life force" in the air itself. He proposed that Spallanzani's extensive boiling destroyed the "life force" present in the soup, preventing spontaneous generation in the sealed bowls but allowing air to replenish the life force in the open bowls. For several decades, scientists continued to debate the spontaneous generation theory of life, with support for the theory coming from several notable scientists including Félix Pouchet and Henry Bastion. Pouchet, Director of the Rouen Museum of Natural History in France, and Bastion, a well-known British bacteriologist, argued that living organisms could spontaneously arise from chemical processes such as fermentation and putrefaction. The debate became so heated that in 1860, the French Academy of Sciences established the Alhumbert prize of 2,500 francs to the first person who could conclusively resolve the conflict. In 1864, Louis Pasteur achieved that result with a series of well-controlled experiments and in doing so claimed the Alhumbert prize.
Pasteur prepared for his experiments by studying the work of others that came before him. In fact, in April 1861 Pasteur wrote to Pouchet to obtain a research description that Pouchet had published. In this letter, Pasteur writes:
Paris, April 3, 1861 Dear Colleague, The difference of our opinions on the famous question of spontaneous generation does not prevent me from esteeming highly your labor and praiseworthy efforts... The sincerity of these sentiments...permits me to have recourse to your obligingness in full confidence. I read with great care everything that you write on the subject that occupies both of us. Now, I cannot obtain a brochure that I understand you have just published.... I would be happy to have a copy of it because I am at present editing the totality of my observations, where naturally I criticize your assertions. L. Pasteur (Porter, 1961)
Pasteur received the brochure from Pouchet several days later and went on to conduct his own experiments . In these, he repeated Spallanzani's method of boiling soup broth, but he divided the broth into portions and exposed these portions to different controlled conditions. Some broth was placed in flasks that had straight necks that were open to the air, some broth was placed in sealed flasks that were not open to the air, and some broth was placed into a specially designed set of swan-necked flasks, in which the broth would be open to the air but the air would have to travel a curved path before reaching the broth, thus preventing anything that might be present in the air from simply settling onto the soup (Figure 2). Pasteur then observed the response of the dependent variable (the growth of microorganisms) in response to the independent variable (the design of the flask). Pasteur's experiments contained both positive controls (samples in the straight-necked flasks that he knew would become contaminated with microorganisms) and negative controls (samples in the sealed flasks that he knew would remain sterile). If spontaneous generation did indeed occur upon exposure to air, Pasteur hypothesized, microorganisms would be found in both the swan-neck flasks and the straight-necked flasks, but not in the sealed flasks. Instead, Pasteur found that microorganisms appeared in the straight-necked flasks, but not in the sealed flasks or the swan-necked flasks.
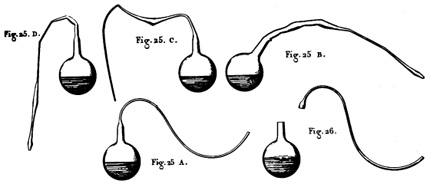
Figure 2: Pasteur's drawings of the flasks he used (Pasteur, 1861). Fig. 25 D, C, and B (top) show various sealed flasks (negative controls); Fig. 26 (bottom right) illustrates a straight-necked flask directly open to the atmosphere (positive control); and Fig. 25 A (bottom left) illustrates the specially designed swan-necked flask (treatment group).
By using controls and replicating his experiment (he used more than one of each type of flask), Pasteur was able to answer many of the questions that still surrounded the issue of spontaneous generation. Pasteur said of his experimental design, "I affirm with the most perfect sincerity that I have never had a single experiment, arranged as I have just explained, which gave me a doubtful result" (Porter, 1961). Pasteur's work helped refute the theory of spontaneous generation – his experiments showed that air alone was not the cause of bacterial growth in the flask, and his research supported the hypothesis that live microorganisms suspended in air could settle onto the broth in open-necked flasks via gravity .
- Experimentation across disciplines
Experiments are used across all scientific disciplines to investigate a multitude of questions. In some cases, scientific experiments are used for exploratory purposes in which the scientist does not know what the dependent variable is. In this type of experiment, the scientist will manipulate an independent variable and observe what the effect of the manipulation is in order to identify a dependent variable (or variables). Exploratory experiments are sometimes used in nutritional biology when scientists probe the function and purpose of dietary nutrients . In one approach, a scientist will expose one group of animals to a normal diet, and a second group to a similar diet except that it is lacking a specific vitamin or nutrient. The researcher will then observe the two groups to see what specific physiological changes or medical problems arise in the group lacking the nutrient being studied.
Scientific experiments are also commonly used to quantify the magnitude of a relationship between two or more variables . For example, in the fields of pharmacology and toxicology, scientific experiments are used to determine the dose-response relationship of a new drug or chemical. In these approaches, researchers perform a series of experiments in which a population of organisms , such as laboratory mice, is separated into groups and each group is exposed to a different amount of the drug or chemical of interest. The analysis of the data that result from these experiments (see our Data Analysis and Interpretation module) involves comparing the degree of the organism's response to the dose of the substance administered.
In this context, experiments can provide additional evidence to complement other research methods . For example, in the 1950s a great debate ensued over whether or not the chemicals in cigarette smoke cause cancer. Several researchers had conducted comparative studies (see our Comparison in Scientific Research module) that indicated that patients who smoked had a higher probability of developing lung cancer when compared to nonsmokers. Comparative studies differ slightly from experimental methods in that you do not consciously manipulate a variable ; rather you observe differences between two or more groups depending on whether or not they fall into a treatment or control group. Cigarette companies and lobbyists criticized these studies, suggesting that the relationship between smoking and lung cancer was coincidental. Several researchers noted the need for a clear dose-response study; however, the difficulties in getting cigarette smoke into the lungs of laboratory animals prevented this research. In the mid-1950s, Ernest Wynder and colleagues had an ingenious idea: They condensed the chemicals from cigarette smoke into a liquid and applied this in various doses to the skin of groups of mice. The researchers published data from a dose-response experiment of the effect of tobacco smoke condensate on mice (Wynder et al., 1957).
As seen in Figure 3, the researchers found a positive relationship between the amount of condensate applied to the skin of mice and the number of cancers that developed. The graph shows the results of a study in which different groups of mice were exposed to increasing amounts of cigarette tar. The black dots indicate the percentage of each sample group of mice that developed cancer for a given amount cigarette smoke "condensate" applied to their skin. The vertical lines are error bars, showing the amount of uncertainty . The graph shows generally increasing cancer rates with greater exposure. This study was one of the first pieces of experimental evidence in the cigarette smoking debate , and it helped strengthen the case for cigarette smoke as the causative agent in lung cancer in smokers.
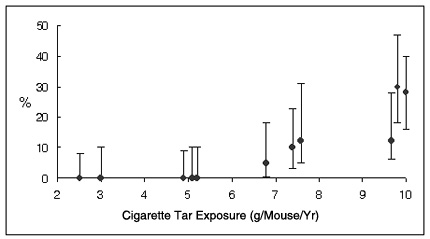
Figure 3: Percentage of mice with cancer versus the amount cigarette smoke "condensate" applied to their skin (source: Wynder et al., 1957).
Sometimes experimental approaches and other research methods are not clearly distinct, or scientists may even use multiple research approaches in combination. For example, at 1:52 a.m. EDT on July 4, 2005, scientists with the National Aeronautics and Space Administration (NASA) conducted a study in which a 370 kg spacecraft named Deep Impact was purposely slammed into passing comet Tempel 1. A nearby spacecraft observed the impact and radioed data back to Earth. The research was partially descriptive in that it documented the chemical composition of the comet, but it was also partly experimental in that the effect of slamming the Deep Impact probe into the comet on the volatilization of previously undetected compounds , such as water, was assessed (A'Hearn et al., 2005). It is particularly common that experimentation and description overlap: Another example is Jane Goodall 's research on the behavior of chimpanzees, which can be read in our Description in Scientific Research module.
- Limitations of experimental methods
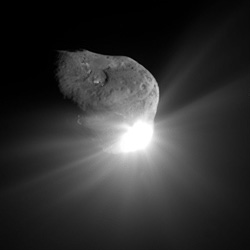
Figure 4: An image of comet Tempel 1 67 seconds after collision with the Deep Impact impactor. Image credit: NASA/JPL-Caltech/UMD http://deepimpact.umd.edu/gallery/HRI_937_1.html
While scientific experiments provide invaluable data regarding causal relationships, they do have limitations. One criticism of experiments is that they do not necessarily represent real-world situations. In order to clearly identify the relationship between an independent variable and a dependent variable , experiments are designed so that many other contributing variables are fixed or eliminated. For example, in an experiment designed to quantify the effect of vitamin A dose on the metabolism of beta-carotene in humans, Shawna Lemke and colleagues had to precisely control the diet of their human volunteers (Lemke, Dueker et al. 2003). They asked their participants to limit their intake of foods rich in vitamin A and further asked that they maintain a precise log of all foods eaten for 1 week prior to their study. At the time of their study, they controlled their participants' diet by feeding them all the same meals, described in the methods section of their research article in this way:
Meals were controlled for time and content on the dose administration day. Lunch was served at 5.5 h postdosing and consisted of a frozen dinner (Enchiladas, Amy's Kitchen, Petaluma, CA), a blueberry bagel with jelly, 1 apple and 1 banana, and a large chocolate chunk cookie (Pepperidge Farm). Dinner was served 10.5 h post dose and consisted of a frozen dinner (Chinese Stir Fry, Amy's Kitchen) plus the bagel and fruit taken for lunch.
While this is an important aspect of making an experiment manageable and informative, it is often not representative of the real world, in which many variables may change at once, including the foods you eat. Still, experimental research is an excellent way of determining relationships between variables that can be later validated in real world settings through descriptive or comparative studies.
Design is critical to the success or failure of an experiment . Slight variations in the experimental set-up could strongly affect the outcome being measured. For example, during the 1950s, a number of experiments were conducted to evaluate the toxicity in mammals of the metal molybdenum, using rats as experimental subjects . Unexpectedly, these experiments seemed to indicate that the type of cage the rats were housed in affected the toxicity of molybdenum. In response, G. Brinkman and Russell Miller set up an experiment to investigate this observation (Brinkman & Miller, 1961). Brinkman and Miller fed two groups of rats a normal diet that was supplemented with 200 parts per million (ppm) of molybdenum. One group of rats was housed in galvanized steel (steel coated with zinc to reduce corrosion) cages and the second group was housed in stainless steel cages. Rats housed in the galvanized steel cages suffered more from molybdenum toxicity than the other group: They had higher concentrations of molybdenum in their livers and lower blood hemoglobin levels. It was then shown that when the rats chewed on their cages, those housed in the galvanized metal cages absorbed zinc plated onto the metal bars, and zinc is now known to affect the toxicity of molybdenum. In order to control for zinc exposure, then, stainless steel cages needed to be used for all rats.
Scientists also have an obligation to adhere to ethical limits in designing and conducting experiments . During World War II, doctors working in Nazi Germany conducted many heinous experiments using human subjects . Among them was an experiment meant to identify effective treatments for hypothermia in humans, in which concentration camp prisoners were forced to sit in ice water or left naked outdoors in freezing temperatures and then re-warmed by various means. Many of the exposed victims froze to death or suffered permanent injuries. As a result of the Nazi experiments and other unethical research , strict scientific ethical standards have been adopted by the United States and other governments, and by the scientific community at large. Among other things, ethical standards (see our Scientific Ethics module) require that the benefits of research outweigh the risks to human subjects, and those who participate do so voluntarily and only after they have been made fully aware of all the risks posed by the research. These guidelines have far-reaching effects: While the clearest indication of causation in the cigarette smoke and lung cancer debate would have been to design an experiment in which one group of people was asked to take up smoking and another group was asked to refrain from smoking, it would be highly unethical for a scientist to purposefully expose a group of healthy people to a suspected cancer causing agent. As an alternative, comparative studies (see our Comparison in Scientific Research module) were initiated in humans, and experimental studies focused on animal subjects. The combination of these and other studies provided even stronger evidence of the link between smoking and lung cancer than either one method alone would have.
- Experimentation in modern practice
Like all scientific research , the results of experiments are shared with the scientific community, are built upon, and inspire additional experiments and research. For example, once Alhazen established that light given off by objects enters the human eye, the natural question that was asked was "What is the nature of light that enters the human eye?" Two common theories about the nature of light were debated for many years. Sir Isaac Newton was among the principal proponents of a theory suggesting that light was made of small particles . The English naturalist Robert Hooke (who held the interesting title of Curator of Experiments at the Royal Society of London) supported a different theory stating that light was a type of wave, like sound waves . In 1801, Thomas Young conducted a now classic scientific experiment that helped resolve this controversy . Young, like Alhazen, worked in a darkened room and allowed light to enter only through a small hole in a window shade (Figure 5). Young refocused the beam of light with mirrors and split the beam with a paper-thin card. The split light beams were then projected onto a screen, and formed an alternating light and dark banding pattern – that was a sign that light was indeed a wave (see our Light I: Particle or Wave? module).
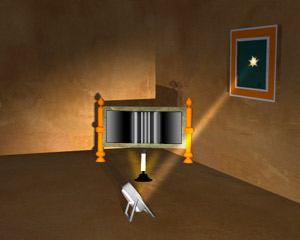
Figure 5: Young's split-light beam experiment helped clarify the wave nature of light.
Approximately 100 years later, in 1905, new experiments led Albert Einstein to conclude that light exhibits properties of both waves and particles . Einstein's dual wave-particle theory is now generally accepted by scientists.
Experiments continue to help refine our understanding of light even today. In addition to his wave-particle theory , Einstein also proposed that the speed of light was unchanging and absolute. Yet in 1998 a group of scientists led by Lene Hau showed that light could be slowed from its normal speed of 3 x 10 8 meters per second to a mere 17 meters per second with a special experimental apparatus (Hau et al., 1999). The series of experiments that began with Alhazen 's work 1000 years ago has led to a progressively deeper understanding of the nature of light. Although the tools with which scientists conduct experiments may have become more complex, the principles behind controlled experiments are remarkably similar to those used by Pasteur and Alhazen hundreds of years ago.
Table of Contents
Activate glossary term highlighting to easily identify key terms within the module. Once highlighted, you can click on these terms to view their definitions.
Activate NGSS annotations to easily identify NGSS standards within the module. Once highlighted, you can click on them to view these standards.
Creating Experimental Setups
- First Online: 12 April 2023
Cite this chapter
- Raban Iten 2
653 Accesses
1 Altmetric
Creating experimental setups is a fundamental step in a physicist’s discovery process. This task is particularly challenging for quantum systems, since the behavior of such systems is often unintuitive. In this chapter, we discuss how a special kind of reinforcement learning, called projective simulation, can help to automate the creation of experimental setups.
This is a preview of subscription content, log in via an institution to check access.
Access this chapter
Subscribe and save.
- Get 10 units per month
- Download Article/Chapter or eBook
- 1 Unit = 1 Article or 1 Chapter
- Cancel anytime
- Available as PDF
- Read on any device
- Instant download
- Own it forever
- Available as EPUB and PDF
- Compact, lightweight edition
- Dispatched in 3 to 5 business days
- Free shipping worldwide - see info
- Durable hardcover edition
Tax calculation will be finalised at checkout
Purchases are for personal use only
Institutional subscriptions
A Hilbert space is a real or complex inner product space that is a complete metric space with respect to the distance function induced by the inner product. The distance function, induced by an inner product \(\left\langle \cdot |\cdot \right\rangle \) , between two vectors x and y is defined by \(\left\langle x-y|x-y\right\rangle \) .
The notation \(\left\langle \psi _{AB}|\psi _{AB}\right\rangle \) abbreviates the expression \(\left\langle | \psi _{AB} \rangle || \psi _{AB} \rangle \right\rangle \) and is actually one of the underlying reasons why it is common to bracket a quantum state by \(| \cdot \rangle \) , which is called the “ket”-notation. Together with the “bra”-notation \(\langle \psi _{AB} |\) , denoting the complex conjugate row vector of the column vector \(\psi _{AB}\) , the “braket”-notation \(\left\langle \psi _{AB}|\psi _{AB}\right\rangle \) can be read as the matrix multiplication of \(\langle \psi _{AB} |\) with \(| \psi _{AB} \rangle \) .
Author information
Authors and affiliations.
ETH Zürich, Zürich, Switzerland
You can also search for this author in PubMed Google Scholar
Corresponding author
Correspondence to Raban Iten .
Rights and permissions
Reprints and permissions
Copyright information
© 2023 The Author(s), under exclusive license to Springer Nature Switzerland AG
About this chapter
Iten, R. (2023). Creating Experimental Setups. In: Artificial Intelligence for Scientific Discoveries. Springer, Cham. https://doi.org/10.1007/978-3-031-27019-2_5
Download citation
DOI : https://doi.org/10.1007/978-3-031-27019-2_5
Published : 12 April 2023
Publisher Name : Springer, Cham
Print ISBN : 978-3-031-27018-5
Online ISBN : 978-3-031-27019-2
eBook Packages : Physics and Astronomy Physics and Astronomy (R0)
Share this chapter
Anyone you share the following link with will be able to read this content:
Sorry, a shareable link is not currently available for this article.
Provided by the Springer Nature SharedIt content-sharing initiative
- Publish with us
Policies and ethics
- Find a journal
- Track your research
- PRO Courses Guides New Tech Help Pro Expert Videos About wikiHow Pro Upgrade Sign In
- EDIT Edit this Article
- EXPLORE Tech Help Pro About Us Random Article Quizzes Request a New Article Community Dashboard This Or That Game Happiness Hub Popular Categories Arts and Entertainment Artwork Books Movies Computers and Electronics Computers Phone Skills Technology Hacks Health Men's Health Mental Health Women's Health Relationships Dating Love Relationship Issues Hobbies and Crafts Crafts Drawing Games Education & Communication Communication Skills Personal Development Studying Personal Care and Style Fashion Hair Care Personal Hygiene Youth Personal Care School Stuff Dating All Categories Arts and Entertainment Finance and Business Home and Garden Relationship Quizzes Cars & Other Vehicles Food and Entertaining Personal Care and Style Sports and Fitness Computers and Electronics Health Pets and Animals Travel Education & Communication Hobbies and Crafts Philosophy and Religion Work World Family Life Holidays and Traditions Relationships Youth
- Browse Articles
- Learn Something New
- Quizzes Hot
- Happiness Hub
- This Or That Game
- Train Your Brain
- Explore More
- Support wikiHow
- About wikiHow
- Log in / Sign up
- Education and Communications
- Science Experiments
How to Conduct a Science Experiment
Last Updated: June 5, 2024 Fact Checked
This article was co-authored by Meredith Juncker, PhD . Meredith Juncker is a PhD candidate in Biochemistry and Molecular Biology at Louisiana State University Health Sciences Center. Her studies are focused on proteins and neurodegenerative diseases. There are 10 references cited in this article, which can be found at the bottom of the page. This article has been fact-checked, ensuring the accuracy of any cited facts and confirming the authority of its sources. This article has been viewed 196,981 times.
Experimentation is the method by which scientists test natural phenomena in the hopes of gaining new knowledge.. Good experiments follow a logical design to isolate and test specific, precisely-defined variables. By learning the fundamental principles behind experimental design, you'll be able to apply these principles to your own experiments. Regardless of their scope, all good experiments operate according to the logical, deductive principles of the scientific method, from fifth-grade potato clock science fair projects to cutting-edge Higgs Boson research. [1] X Research source
Designing a Scientifically Sound Experiment

- For instance, if you want to do an experiment on agricultural fertilizer, don't seek to answer the question, "Which kind of fertilizer is best for growing plants?" There are many different types of fertilizer and many different kinds of plants in the world - one experiment won't be able to draw universal conclusions about either. A much better question to design an experiment around would be "What concentration of nitrogen in fertilizer produces the largest corn crops?"
- Modern scientific knowledge is very, very vast. If you intend to do serious scientific research, research your topic extensively before you even begin to plan your experiment. Have past experiments answered the question you want your experiment to study? If so, is there a way to adjust your topic so that it addresses questions left unanswered by existing research?

- For instance, in our fertilizer experiment example, our scientist would grow multiple corn crops in soil supplemented with fertilizers whose nitrogen concentration differs. He would give each corn crop the exact same amount of fertilizer. He would make sure the chemical composition of his fertilizers used did not differ in some way besides its nitrogen concentration - for instance, he would not use a fertilizer with a higher concentration of magnesium for one of his corn crops. He would also grow the exact same number and species of corn crops at the same time and in the same type of soil in each replication of his experiment.

- Typically, a hypothesis is expressed as a quantitative declarative sentence. A hypothesis also takes into account the ways that the experimental parameters will be measured. A good hypothesis for our fertilizer example is: "Corn crops supplemented with 1 pound of nitrogen per bushel will result in a greater yield mass than equivalent corn crops grown with differing nitrogen supplements."

- Timing is incredibly important, so stick to your plan as close as possible. That way, if you see changes in your results, you can rule out different time constraints as the cause of the change.
- Making a data table beforehand is a great idea - you'll be able to simply insert your data values into the table as you record them.
- Know the difference between your dependent and independent variables. An independent variable is a variable that you change and a dependent variable is the one affected by the independent variable. In our example, "nitrogen content" is the independent variable, and "yield (in kg)" is the dependent variable. A basic table will have columns for both variables as they change over time.

- Good experimental design incorporates what's known as a control. One of your experimental replications should not include the variable you're testing for at all. In our fertilizer example, we'll include one corn crop which receives a fertilizer with no nitrogen in it. This will be our control - it will be the baseline against which we'll measure the growth of our other corn crops.
- Observe any and all safety measures associated with hazardous materials or processes in your experiment. [6] X Research source

- It's always a good idea to represent your data visually if you can. Plot data points on a graph and express trends with a line or curve of best fit. This will help you (and anyone else who sees the graph) visualize patterns in the data. For most basic experiments, the independent variable is represented on the horizontal x axis and the dependent variable is on the vertical y axis.

- To share your results, write a comprehensive scientific paper. Knowing how to write a scientific paper is a useful skill - the results of most new research must be written and published according to a specific format, often dictated by the style guide for a relevant, peer-reviewed academic journal.
Running an Example Experiment

- In this case, the type of aerosol fuel we use is the independent variable (the variable we change), while the range of the projectile is the dependent variable.
- Things to consider for this experiment - is there a way to ensure each potato projectile has the same weight? Is there a way to administer the same amount of aerosol fuel for each firing? Both of these can potentially affect the range of the gun. Weigh each projectile beforehand and fuel each shot with the same amount of aerosol spray.

- The furthest-left column will be labeled "Trial #." The cells in this column will simply contain the numbers 1-10, signifying each firing attempt.
- The following four columns will be labeled with the names of the aerosol sprays we're using in our experiment. The ten cells beneath each column header will contain the range (in meters) of each firing attempt.
- Below the four columns for each fuel, leave a space to write the average value of the ranges.

- Like many experiments, our experiment has certain safety concerns we need to observe. The aerosol fuels we're using are flammable - we should be sure to close the potato gun's firing cap securely and to wear heavy gloves while igniting the fuel. To avoid accidental injuries from the projectiles, we should also make sure that we (and any observers) are standing to the side of the gun as it fires - not in front of it or behind it.

- We can even share our results with the world in the form of a scientific paper - given the subject matter of our experiment, it may be more appropriate to present this information in the form of a tri-fold science fair display.
Community Q&A

- Science is about asking big questions. Don't be afraid to choose a topic you haven't looked at before. Thanks Helpful 0 Not Helpful 0
- Have fun and stay safe. Thanks Helpful 0 Not Helpful 0
- In upper-level sciences, most data isn't used unless it is reproducible at least 3 times. Thanks Helpful 0 Not Helpful 0

- Wear eye protection Thanks Helpful 29 Not Helpful 1
- Wash your hands before and after an experiment. Thanks Helpful 29 Not Helpful 3
- Do not have any food or drinks near your workstation. Thanks Helpful 25 Not Helpful 5
- If anything gets in your eyes rinse them out thoroughly with water for 15 minutes, then seek immediate medical attention. Thanks Helpful 7 Not Helpful 0
- When using sharp knives, dangerous chemicals, or hot flames, make sure you have an adult supervising you at all times. Thanks Helpful 15 Not Helpful 3
- Tie loose hair back Thanks Helpful 23 Not Helpful 7
- Wear rubber gloves when handling chemicals Thanks Helpful 23 Not Helpful 8
You Might Also Like

- ↑ https://www.khanacademy.org/science/high-school-biology/hs-biology-foundations/hs-biology-and-the-scientific-method/a/experiments-and-observations
- ↑ https://www.sciencebuddies.org/science-fair-projects/project-ideas/list
- ↑ https://www.sciencebuddies.org/science-fair-projects/science-fair/variables
- ↑ https://www.livescience.com/21490-what-is-a-scientific-hypothesis-definition-of-hypothesis.html
- ↑ https://sciencing.com/collect-data-science-project-5988780.html
- ↑ https://ehsdailyadvisor.blr.com/2012/04/11-rules-for-safe-handling-of-hazardous-materials/
- ↑ https://www.sciencebuddies.org/science-fair-projects/science-fair/conducting-an-experiment
- ↑ https://www.sciencebuddies.org/science-fair-projects/science-fair/writing-a-hypothesis
- ↑ https://www.sciencebuddies.org/science-fair-projects/science-fair/steps-of-the-scientific-method
- ↑ https://www.sciencebuddies.org/science-fair-projects/science-fair/data-analysis-graphs
About This Article

If you want to conduct a science experiment, first come up with a question you want to answer, then devise a way to test that question. Make sure you have a control, or an untested component to your experiment. For example, if you want to find out which fertilizer is best for growing crops, you would have one plant for each type of fertilizer, plus one plant that doesn’t get any fertilizer. Write down each step of your experiment carefully, along with the final result. For tips on organizing your data collection, read on! Did this summary help you? Yes No
- Send fan mail to authors
Reader Success Stories

Latricia Dyer
Sep 28, 2016
Did this article help you?

Alexandra Matusescu
Feb 17, 2017

Featured Articles

Trending Articles

Watch Articles

- Terms of Use
- Privacy Policy
- Do Not Sell or Share My Info
- Not Selling Info
Get all the best how-tos!
Sign up for wikiHow's weekly email newsletter

- Science Notes Posts
- Contact Science Notes
- Todd Helmenstine Biography
- Anne Helmenstine Biography
- Free Printable Periodic Tables (PDF and PNG)
- Periodic Table Wallpapers
- Interactive Periodic Table
- Periodic Table Posters
- Science Experiments for Kids
- How to Grow Crystals
- Chemistry Projects
- Fire and Flames Projects
- Holiday Science
- Chemistry Problems With Answers
- Physics Problems
- Unit Conversion Example Problems
- Chemistry Worksheets
- Biology Worksheets
- Periodic Table Worksheets
- Physical Science Worksheets
- Science Lab Worksheets
- My Amazon Books
Experiment Definition in Science – What Is a Science Experiment?

In science, an experiment is simply a test of a hypothesis in the scientific method . It is a controlled examination of cause and effect. Here is a look at what a science experiment is (and is not), the key factors in an experiment, examples, and types of experiments.
Experiment Definition in Science
By definition, an experiment is a procedure that tests a hypothesis. A hypothesis, in turn, is a prediction of cause and effect or the predicted outcome of changing one factor of a situation. Both the hypothesis and experiment are components of the scientific method. The steps of the scientific method are:
- Make observations.
- Ask a question or identify a problem.
- State a hypothesis.
- Perform an experiment that tests the hypothesis.
- Based on the results of the experiment, either accept or reject the hypothesis.
- Draw conclusions and report the outcome of the experiment.
Key Parts of an Experiment
The two key parts of an experiment are the independent and dependent variables. The independent variable is the one factor that you control or change in an experiment. The dependent variable is the factor that you measure that responds to the independent variable. An experiment often includes other types of variables , but at its heart, it’s all about the relationship between the independent and dependent variable.
Examples of Experiments
Fertilizer and plant size.
For example, you think a certain fertilizer helps plants grow better. You’ve watched your plants grow and they seem to do better when they have the fertilizer compared to when they don’t. But, observations are only the beginning of science. So, you state a hypothesis: Adding fertilizer increases plant size. Note, you could have stated the hypothesis in different ways. Maybe you think the fertilizer increases plant mass or fruit production, for example. However you state the hypothesis, it includes both the independent and dependent variables. In this case, the independent variable is the presence or absence of fertilizer. The dependent variable is the response to the independent variable, which is the size of the plants.
Now that you have a hypothesis, the next step is designing an experiment that tests it. Experimental design is very important because the way you conduct an experiment influences its outcome. For example, if you use too small of an amount of fertilizer you may see no effect from the treatment. Or, if you dump an entire container of fertilizer on a plant you could kill it! So, recording the steps of the experiment help you judge the outcome of the experiment and aid others who come after you and examine your work. Other factors that might influence your results might include the species of plant and duration of the treatment. Record any conditions that might affect the outcome. Ideally, you want the only difference between your two groups of plants to be whether or not they receive fertilizer. Then, measure the height of the plants and see if there is a difference between the two groups.
Salt and Cookies
You don’t need a lab for an experiment. For example, consider a baking experiment. Let’s say you like the flavor of salt in your cookies, but you’re pretty sure the batch you made using extra salt fell a bit flat. If you double the amount of salt in a recipe, will it affect their size? Here, the independent variable is the amount of salt in the recipe and the dependent variable is cookie size.
Test this hypothesis with an experiment. Bake cookies using the normal recipe (your control group ) and bake some using twice the salt (the experimental group). Make sure it’s the exact same recipe. Bake the cookies at the same temperature and for the same time. Only change the amount of salt in the recipe. Then measure the height or diameter of the cookies and decide whether to accept or reject the hypothesis.
Examples of Things That Are Not Experiments
Based on the examples of experiments, you should see what is not an experiment:
- Making observations does not constitute an experiment. Initial observations often lead to an experiment, but are not a substitute for one.
- Making a model is not an experiment.
- Neither is making a poster.
- Just trying something to see what happens is not an experiment. You need a hypothesis or prediction about the outcome.
- Changing a lot of things at once isn’t an experiment. You only have one independent and one dependent variable. However, in an experiment, you might suspect the independent variable has an effect on a separate. So, you design a new experiment to test this.
Types of Experiments
There are three main types of experiments: controlled experiments, natural experiments, and field experiments,
- Controlled experiment : A controlled experiment compares two groups of samples that differ only in independent variable. For example, a drug trial compares the effect of a group taking a placebo (control group) against those getting the drug (the treatment group). Experiments in a lab or home generally are controlled experiments
- Natural experiment : Another name for a natural experiment is a quasi-experiment. In this type of experiment, the researcher does not directly control the independent variable, plus there may be other variables at play. Here, the goal is establishing a correlation between the independent and dependent variable. For example, in the formation of new elements a scientist hypothesizes that a certain collision between particles creates a new atom. But, other outcomes may be possible. Or, perhaps only decay products are observed that indicate the element, and not the new atom itself. Many fields of science rely on natural experiments, since controlled experiments aren’t always possible.
- Field experiment : While a controlled experiments takes place in a lab or other controlled setting, a field experiment occurs in a natural setting. Some phenomena cannot be readily studied in a lab or else the setting exerts an influence that affects the results. So, a field experiment may have higher validity. However, since the setting is not controlled, it is also subject to external factors and potential contamination. For example, if you study whether a certain plumage color affects bird mate selection, a field experiment in a natural environment eliminates the stressors of an artificial environment. Yet, other factors that could be controlled in a lab may influence results. For example, nutrition and health are controlled in a lab, but not in the field.
- Bailey, R.A. (2008). Design of Comparative Experiments . Cambridge: Cambridge University Press. ISBN 9780521683579.
- di Francia, G. Toraldo (1981). The Investigation of the Physical World . Cambridge University Press. ISBN 0-521-29925-X.
- Hinkelmann, Klaus; Kempthorne, Oscar (2008). Design and Analysis of Experiments. Volume I: Introduction to Experimental Design (2nd ed.). Wiley. ISBN 978-0-471-72756-9.
- Holland, Paul W. (December 1986). “Statistics and Causal Inference”. Journal of the American Statistical Association . 81 (396): 945–960. doi: 10.2307/2289064
- Stohr-Hunt, Patricia (1996). “An Analysis of Frequency of Hands-on Experience and Science Achievement”. Journal of Research in Science Teaching . 33 (1): 101–109. doi: 10.1002/(SICI)1098-2736(199601)33:1<101::AID-TEA6>3.0.CO;2-Z
Related Posts

- Activities, Experiments, Online Games, Visual Aids
- Activities, Experiments, and Investigations
- Experimental Design and the Scientific Method
Experimental Design - Independent, Dependent, and Controlled Variables
To view these resources with no ads, please login or subscribe to help support our content development. school subscriptions can access more than 175 downloadable unit bundles in our store for free (a value of $1,500). district subscriptions provide huge group discounts for their schools. email for a quote: [email protected] ..
Scientific experiments are meant to show cause and effect of a phenomena (relationships in nature). The “ variables ” are any factor, trait, or condition that can be changed in the experiment and that can have an effect on the outcome of the experiment.
An experiment can have three kinds of variables: i ndependent, dependent, and controlled .
- The independent variable is one single factor that is changed by the scientist followed by observation to watch for changes. It is important that there is just one independent variable, so that results are not confusing.
- The dependent variable is the factor that changes as a result of the change to the independent variable.
- The controlled variables (or constant variables) are factors that the scientist wants to remain constant if the experiment is to show accurate results. To be able to measure results, each of the variables must be able to be measured.
For example, let’s design an experiment with two plants sitting in the sun side by side. The controlled variables (or constants) are that at the beginning of the experiment, the plants are the same size, get the same amount of sunlight, experience the same ambient temperature and are in the same amount and consistency of soil (the weight of the soil and container should be measured before the plants are added). The independent variable is that one plant is getting watered (1 cup of water) every day and one plant is getting watered (1 cup of water) once a week. The dependent variables are the changes in the two plants that the scientist observes over time.
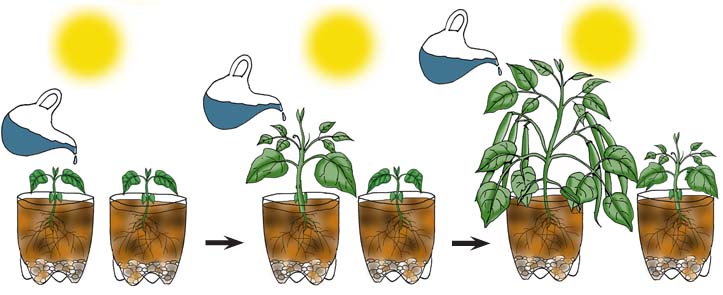
Can you describe the dependent variable that may result from this experiment? After four weeks, the dependent variable may be that one plant is taller, heavier and more developed than the other. These results can be recorded and graphed by measuring and comparing both plants’ height, weight (removing the weight of the soil and container recorded beforehand) and a comparison of observable foliage.
Using What You Learned: Design another experiment using the two plants, but change the independent variable. Can you describe the dependent variable that may result from this new experiment?
Think of another simple experiment and name the independent, dependent, and controlled variables. Use the graphic organizer included in the PDF below to organize your experiment's variables.
Please Login or Subscribe to access downloadable content.
Citing Research References
When you research information you must cite the reference. Citing for websites is different from citing from books, magazines and periodicals. The style of citing shown here is from the MLA Style Citations (Modern Language Association).
When citing a WEBSITE the general format is as follows. Author Last Name, First Name(s). "Title: Subtitle of Part of Web Page, if appropriate." Title: Subtitle: Section of Page if appropriate. Sponsoring/Publishing Agency, If Given. Additional significant descriptive information. Date of Electronic Publication or other Date, such as Last Updated. Day Month Year of access < URL >.
Here is an example of citing this page:
Amsel, Sheri. "Experimental Design - Independent, Dependent, and Controlled Variables" Exploring Nature Educational Resource ©2005-2024. March 25, 2024 < http://www.exploringnature.org/db/view/Experimental-Design-Independent-Dependent-and-Controlled-Variables >
Exploringnature.org has more than 2,000 illustrated animals. Read about them, color them, label them, learn to draw them.

10 Experimental research
Experimental research—often considered to be the ‘gold standard’ in research designs—is one of the most rigorous of all research designs. In this design, one or more independent variables are manipulated by the researcher (as treatments), subjects are randomly assigned to different treatment levels (random assignment), and the results of the treatments on outcomes (dependent variables) are observed. The unique strength of experimental research is its internal validity (causality) due to its ability to link cause and effect through treatment manipulation, while controlling for the spurious effect of extraneous variable.
Experimental research is best suited for explanatory research—rather than for descriptive or exploratory research—where the goal of the study is to examine cause-effect relationships. It also works well for research that involves a relatively limited and well-defined set of independent variables that can either be manipulated or controlled. Experimental research can be conducted in laboratory or field settings. Laboratory experiments , conducted in laboratory (artificial) settings, tend to be high in internal validity, but this comes at the cost of low external validity (generalisability), because the artificial (laboratory) setting in which the study is conducted may not reflect the real world. Field experiments are conducted in field settings such as in a real organisation, and are high in both internal and external validity. But such experiments are relatively rare, because of the difficulties associated with manipulating treatments and controlling for extraneous effects in a field setting.
Experimental research can be grouped into two broad categories: true experimental designs and quasi-experimental designs. Both designs require treatment manipulation, but while true experiments also require random assignment, quasi-experiments do not. Sometimes, we also refer to non-experimental research, which is not really a research design, but an all-inclusive term that includes all types of research that do not employ treatment manipulation or random assignment, such as survey research, observational research, and correlational studies.
Basic concepts
Treatment and control groups. In experimental research, some subjects are administered one or more experimental stimulus called a treatment (the treatment group ) while other subjects are not given such a stimulus (the control group ). The treatment may be considered successful if subjects in the treatment group rate more favourably on outcome variables than control group subjects. Multiple levels of experimental stimulus may be administered, in which case, there may be more than one treatment group. For example, in order to test the effects of a new drug intended to treat a certain medical condition like dementia, if a sample of dementia patients is randomly divided into three groups, with the first group receiving a high dosage of the drug, the second group receiving a low dosage, and the third group receiving a placebo such as a sugar pill (control group), then the first two groups are experimental groups and the third group is a control group. After administering the drug for a period of time, if the condition of the experimental group subjects improved significantly more than the control group subjects, we can say that the drug is effective. We can also compare the conditions of the high and low dosage experimental groups to determine if the high dose is more effective than the low dose.
Treatment manipulation. Treatments are the unique feature of experimental research that sets this design apart from all other research methods. Treatment manipulation helps control for the ‘cause’ in cause-effect relationships. Naturally, the validity of experimental research depends on how well the treatment was manipulated. Treatment manipulation must be checked using pretests and pilot tests prior to the experimental study. Any measurements conducted before the treatment is administered are called pretest measures , while those conducted after the treatment are posttest measures .
Random selection and assignment. Random selection is the process of randomly drawing a sample from a population or a sampling frame. This approach is typically employed in survey research, and ensures that each unit in the population has a positive chance of being selected into the sample. Random assignment, however, is a process of randomly assigning subjects to experimental or control groups. This is a standard practice in true experimental research to ensure that treatment groups are similar (equivalent) to each other and to the control group prior to treatment administration. Random selection is related to sampling, and is therefore more closely related to the external validity (generalisability) of findings. However, random assignment is related to design, and is therefore most related to internal validity. It is possible to have both random selection and random assignment in well-designed experimental research, but quasi-experimental research involves neither random selection nor random assignment.
Threats to internal validity. Although experimental designs are considered more rigorous than other research methods in terms of the internal validity of their inferences (by virtue of their ability to control causes through treatment manipulation), they are not immune to internal validity threats. Some of these threats to internal validity are described below, within the context of a study of the impact of a special remedial math tutoring program for improving the math abilities of high school students.
History threat is the possibility that the observed effects (dependent variables) are caused by extraneous or historical events rather than by the experimental treatment. For instance, students’ post-remedial math score improvement may have been caused by their preparation for a math exam at their school, rather than the remedial math program.
Maturation threat refers to the possibility that observed effects are caused by natural maturation of subjects (e.g., a general improvement in their intellectual ability to understand complex concepts) rather than the experimental treatment.
Testing threat is a threat in pre-post designs where subjects’ posttest responses are conditioned by their pretest responses. For instance, if students remember their answers from the pretest evaluation, they may tend to repeat them in the posttest exam.
Not conducting a pretest can help avoid this threat.
Instrumentation threat , which also occurs in pre-post designs, refers to the possibility that the difference between pretest and posttest scores is not due to the remedial math program, but due to changes in the administered test, such as the posttest having a higher or lower degree of difficulty than the pretest.
Mortality threat refers to the possibility that subjects may be dropping out of the study at differential rates between the treatment and control groups due to a systematic reason, such that the dropouts were mostly students who scored low on the pretest. If the low-performing students drop out, the results of the posttest will be artificially inflated by the preponderance of high-performing students.
Regression threat —also called a regression to the mean—refers to the statistical tendency of a group’s overall performance to regress toward the mean during a posttest rather than in the anticipated direction. For instance, if subjects scored high on a pretest, they will have a tendency to score lower on the posttest (closer to the mean) because their high scores (away from the mean) during the pretest were possibly a statistical aberration. This problem tends to be more prevalent in non-random samples and when the two measures are imperfectly correlated.
Two-group experimental designs

Pretest-posttest control group design . In this design, subjects are randomly assigned to treatment and control groups, subjected to an initial (pretest) measurement of the dependent variables of interest, the treatment group is administered a treatment (representing the independent variable of interest), and the dependent variables measured again (posttest). The notation of this design is shown in Figure 10.1.

Statistical analysis of this design involves a simple analysis of variance (ANOVA) between the treatment and control groups. The pretest-posttest design handles several threats to internal validity, such as maturation, testing, and regression, since these threats can be expected to influence both treatment and control groups in a similar (random) manner. The selection threat is controlled via random assignment. However, additional threats to internal validity may exist. For instance, mortality can be a problem if there are differential dropout rates between the two groups, and the pretest measurement may bias the posttest measurement—especially if the pretest introduces unusual topics or content.
Posttest -only control group design . This design is a simpler version of the pretest-posttest design where pretest measurements are omitted. The design notation is shown in Figure 10.2.

The treatment effect is measured simply as the difference in the posttest scores between the two groups:
![Rendered by QuickLaTeX.com \[E = (O_{1} - O_{2})\,.\]](https://usq.pressbooks.pub/app/uploads/quicklatex/quicklatex.com-90f2ce47275e7b35def5c5b52b7d7d45_l3.png)
The appropriate statistical analysis of this design is also a two-group analysis of variance (ANOVA). The simplicity of this design makes it more attractive than the pretest-posttest design in terms of internal validity. This design controls for maturation, testing, regression, selection, and pretest-posttest interaction, though the mortality threat may continue to exist.

Because the pretest measure is not a measurement of the dependent variable, but rather a covariate, the treatment effect is measured as the difference in the posttest scores between the treatment and control groups as:
Due to the presence of covariates, the right statistical analysis of this design is a two-group analysis of covariance (ANCOVA). This design has all the advantages of posttest-only design, but with internal validity due to the controlling of covariates. Covariance designs can also be extended to pretest-posttest control group design.
Factorial designs
Two-group designs are inadequate if your research requires manipulation of two or more independent variables (treatments). In such cases, you would need four or higher-group designs. Such designs, quite popular in experimental research, are commonly called factorial designs. Each independent variable in this design is called a factor , and each subdivision of a factor is called a level . Factorial designs enable the researcher to examine not only the individual effect of each treatment on the dependent variables (called main effects), but also their joint effect (called interaction effects).

In a factorial design, a main effect is said to exist if the dependent variable shows a significant difference between multiple levels of one factor, at all levels of other factors. No change in the dependent variable across factor levels is the null case (baseline), from which main effects are evaluated. In the above example, you may see a main effect of instructional type, instructional time, or both on learning outcomes. An interaction effect exists when the effect of differences in one factor depends upon the level of a second factor. In our example, if the effect of instructional type on learning outcomes is greater for three hours/week of instructional time than for one and a half hours/week, then we can say that there is an interaction effect between instructional type and instructional time on learning outcomes. Note that the presence of interaction effects dominate and make main effects irrelevant, and it is not meaningful to interpret main effects if interaction effects are significant.
Hybrid experimental designs
Hybrid designs are those that are formed by combining features of more established designs. Three such hybrid designs are randomised bocks design, Solomon four-group design, and switched replications design.
Randomised block design. This is a variation of the posttest-only or pretest-posttest control group design where the subject population can be grouped into relatively homogeneous subgroups (called blocks ) within which the experiment is replicated. For instance, if you want to replicate the same posttest-only design among university students and full-time working professionals (two homogeneous blocks), subjects in both blocks are randomly split between the treatment group (receiving the same treatment) and the control group (see Figure 10.5). The purpose of this design is to reduce the ‘noise’ or variance in data that may be attributable to differences between the blocks so that the actual effect of interest can be detected more accurately.
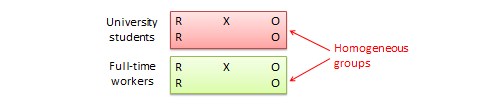
Solomon four-group design . In this design, the sample is divided into two treatment groups and two control groups. One treatment group and one control group receive the pretest, and the other two groups do not. This design represents a combination of posttest-only and pretest-posttest control group design, and is intended to test for the potential biasing effect of pretest measurement on posttest measures that tends to occur in pretest-posttest designs, but not in posttest-only designs. The design notation is shown in Figure 10.6.
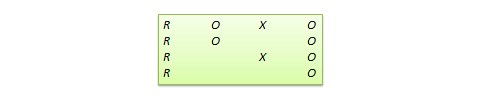
Switched replication design . This is a two-group design implemented in two phases with three waves of measurement. The treatment group in the first phase serves as the control group in the second phase, and the control group in the first phase becomes the treatment group in the second phase, as illustrated in Figure 10.7. In other words, the original design is repeated or replicated temporally with treatment/control roles switched between the two groups. By the end of the study, all participants will have received the treatment either during the first or the second phase. This design is most feasible in organisational contexts where organisational programs (e.g., employee training) are implemented in a phased manner or are repeated at regular intervals.

Quasi-experimental designs
Quasi-experimental designs are almost identical to true experimental designs, but lacking one key ingredient: random assignment. For instance, one entire class section or one organisation is used as the treatment group, while another section of the same class or a different organisation in the same industry is used as the control group. This lack of random assignment potentially results in groups that are non-equivalent, such as one group possessing greater mastery of certain content than the other group, say by virtue of having a better teacher in a previous semester, which introduces the possibility of selection bias . Quasi-experimental designs are therefore inferior to true experimental designs in interval validity due to the presence of a variety of selection related threats such as selection-maturation threat (the treatment and control groups maturing at different rates), selection-history threat (the treatment and control groups being differentially impacted by extraneous or historical events), selection-regression threat (the treatment and control groups regressing toward the mean between pretest and posttest at different rates), selection-instrumentation threat (the treatment and control groups responding differently to the measurement), selection-testing (the treatment and control groups responding differently to the pretest), and selection-mortality (the treatment and control groups demonstrating differential dropout rates). Given these selection threats, it is generally preferable to avoid quasi-experimental designs to the greatest extent possible.

In addition, there are quite a few unique non-equivalent designs without corresponding true experimental design cousins. Some of the more useful of these designs are discussed next.
Regression discontinuity (RD) design . This is a non-equivalent pretest-posttest design where subjects are assigned to the treatment or control group based on a cut-off score on a preprogram measure. For instance, patients who are severely ill may be assigned to a treatment group to test the efficacy of a new drug or treatment protocol and those who are mildly ill are assigned to the control group. In another example, students who are lagging behind on standardised test scores may be selected for a remedial curriculum program intended to improve their performance, while those who score high on such tests are not selected from the remedial program.

Because of the use of a cut-off score, it is possible that the observed results may be a function of the cut-off score rather than the treatment, which introduces a new threat to internal validity. However, using the cut-off score also ensures that limited or costly resources are distributed to people who need them the most, rather than randomly across a population, while simultaneously allowing a quasi-experimental treatment. The control group scores in the RD design do not serve as a benchmark for comparing treatment group scores, given the systematic non-equivalence between the two groups. Rather, if there is no discontinuity between pretest and posttest scores in the control group, but such a discontinuity persists in the treatment group, then this discontinuity is viewed as evidence of the treatment effect.
Proxy pretest design . This design, shown in Figure 10.11, looks very similar to the standard NEGD (pretest-posttest) design, with one critical difference: the pretest score is collected after the treatment is administered. A typical application of this design is when a researcher is brought in to test the efficacy of a program (e.g., an educational program) after the program has already started and pretest data is not available. Under such circumstances, the best option for the researcher is often to use a different prerecorded measure, such as students’ grade point average before the start of the program, as a proxy for pretest data. A variation of the proxy pretest design is to use subjects’ posttest recollection of pretest data, which may be subject to recall bias, but nevertheless may provide a measure of perceived gain or change in the dependent variable.

Separate pretest-posttest samples design . This design is useful if it is not possible to collect pretest and posttest data from the same subjects for some reason. As shown in Figure 10.12, there are four groups in this design, but two groups come from a single non-equivalent group, while the other two groups come from a different non-equivalent group. For instance, say you want to test customer satisfaction with a new online service that is implemented in one city but not in another. In this case, customers in the first city serve as the treatment group and those in the second city constitute the control group. If it is not possible to obtain pretest and posttest measures from the same customers, you can measure customer satisfaction at one point in time, implement the new service program, and measure customer satisfaction (with a different set of customers) after the program is implemented. Customer satisfaction is also measured in the control group at the same times as in the treatment group, but without the new program implementation. The design is not particularly strong, because you cannot examine the changes in any specific customer’s satisfaction score before and after the implementation, but you can only examine average customer satisfaction scores. Despite the lower internal validity, this design may still be a useful way of collecting quasi-experimental data when pretest and posttest data is not available from the same subjects.
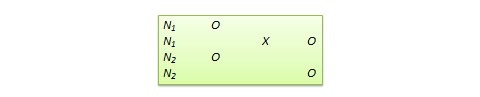
An interesting variation of the NEDV design is a pattern-matching NEDV design , which employs multiple outcome variables and a theory that explains how much each variable will be affected by the treatment. The researcher can then examine if the theoretical prediction is matched in actual observations. This pattern-matching technique—based on the degree of correspondence between theoretical and observed patterns—is a powerful way of alleviating internal validity concerns in the original NEDV design.

Perils of experimental research
Experimental research is one of the most difficult of research designs, and should not be taken lightly. This type of research is often best with a multitude of methodological problems. First, though experimental research requires theories for framing hypotheses for testing, much of current experimental research is atheoretical. Without theories, the hypotheses being tested tend to be ad hoc, possibly illogical, and meaningless. Second, many of the measurement instruments used in experimental research are not tested for reliability and validity, and are incomparable across studies. Consequently, results generated using such instruments are also incomparable. Third, often experimental research uses inappropriate research designs, such as irrelevant dependent variables, no interaction effects, no experimental controls, and non-equivalent stimulus across treatment groups. Findings from such studies tend to lack internal validity and are highly suspect. Fourth, the treatments (tasks) used in experimental research may be diverse, incomparable, and inconsistent across studies, and sometimes inappropriate for the subject population. For instance, undergraduate student subjects are often asked to pretend that they are marketing managers and asked to perform a complex budget allocation task in which they have no experience or expertise. The use of such inappropriate tasks, introduces new threats to internal validity (i.e., subject’s performance may be an artefact of the content or difficulty of the task setting), generates findings that are non-interpretable and meaningless, and makes integration of findings across studies impossible.
The design of proper experimental treatments is a very important task in experimental design, because the treatment is the raison d’etre of the experimental method, and must never be rushed or neglected. To design an adequate and appropriate task, researchers should use prevalidated tasks if available, conduct treatment manipulation checks to check for the adequacy of such tasks (by debriefing subjects after performing the assigned task), conduct pilot tests (repeatedly, if necessary), and if in doubt, use tasks that are simple and familiar for the respondent sample rather than tasks that are complex or unfamiliar.
In summary, this chapter introduced key concepts in the experimental design research method and introduced a variety of true experimental and quasi-experimental designs. Although these designs vary widely in internal validity, designs with less internal validity should not be overlooked and may sometimes be useful under specific circumstances and empirical contingencies.
Social Science Research: Principles, Methods and Practices (Revised edition) Copyright © 2019 by Anol Bhattacherjee is licensed under a Creative Commons Attribution-NonCommercial-ShareAlike 4.0 International License , except where otherwise noted.
Share This Book
Stack Exchange Network
Stack Exchange network consists of 183 Q&A communities including Stack Overflow , the largest, most trusted online community for developers to learn, share their knowledge, and build their careers.
Q&A for work
Connect and share knowledge within a single location that is structured and easy to search.
What is the difference between the Method and Experimental Setup section
I am writing a Computer Science paper, however my supervisor wants me to describe the methodology in a more general form in the Method section. In the Experimental Setup section I dive into the details. My question is, what is general ?
I always assumed that the Method and Experimental Setup were one section were you describe your setup/method in detail.
- computer-science
- Quite obviously, the Method section is supposed to describe approaches / methods / techniques without implementation details . On the other hand, the Experimental Setup section is the place where those implementation details belong. – Aleksandr Blekh Commented May 2, 2016 at 4:38
The answer to this question depends a little on the field of application.
In fields like computational sciences, this is the section where you describe a set of algorithms to be implemented. In fields like material engineering and life sciences, you describe the general procedure to be followed to solve the problem defined in the problem statement. " General " here refers to more of an overview of your implementation rather than its deeper aspects.
Experimental Setup
This is where you explain the implementation aspects in detail. You describe where and how the algorithms are applied in computation. You depict the use of instruments, apparatus, and other tangible items in material engineering and sciences.
In short, I presume your supervisor wants you to give a brief overview of your implementation in the Method section and would like you to explain it in detail in the Experimental Setup section. However, the level of detail can vary widely among the above sections.
You must log in to answer this question.
Not the answer you're looking for browse other questions tagged computer-science formatting ..
- Featured on Meta
- Preventing unauthorized automated access to the network
- User activation: Learnings and opportunities
- Join Stack Overflow’s CEO and me for the first Stack IRL Community Event in...
Hot Network Questions
- How to format units inside math environment?
- What is "illegal, immoral or improper" use in CPOL?
- Does legislation on transgender healthcare affect medical researchers?
- Five Hundred Cigarettes
- Java class subset of C++ std::list with efficient std::list::sort()
- What causes, and how to avoid, finger numbness?
- Is it possible to speed up this function?
- How many natural operations on subsets are there?
- Matter made of neutral charges does not radiate?
- Is “No Time To Die” the first Bond film to feature children?
- Does this work for page turns in a busy violin part?
- If two subgroups intersect in only the identity, do their cosets intersect in at most one element?
- If Voyager is still an active NASA spacecraft, does it have a flight director? Is that a part time job?
- Are logic and mathematics the only fields in which certainty (proof) can be obtained?
- Is the Earth still capable of massive volcanism, like the kind that caused the formation of the Siberian Traps?
- How to do automated content publishing from CLI in XMCloud
- How do we define a non-standard model of natural numbers in ZFC, including predecessor operations?
- Undamaged tire repeatedly deflating
- Vibrato in Liszt’s Oeuvre
- Is there a fast/clever way to return a logical vector if elements of a vector are in at least one interval?
- Geometry Math Problem Part 2
- Problems regressing y on x/y?
- Do early termination fees hold up in court?
- Why would an ocean world prevent the creation of ocean bases but allow ships?
Thank you for visiting nature.com. You are using a browser version with limited support for CSS. To obtain the best experience, we recommend you use a more up to date browser (or turn off compatibility mode in Internet Explorer). In the meantime, to ensure continued support, we are displaying the site without styles and JavaScript.
- View all journals
- Explore content
- About the journal
- Publish with us
- Sign up for alerts
- Open access
- Published: 26 September 2024
Laser solid-phase synthesis of graphene shell-encapsulated high-entropy alloy nanoparticles
- Yuxiang Liu 1 ,
- Jianghuai Yuan 1 ,
- Jiantao Zhou 1 ,
- Kewen Pan 1 ,
- Ran Zhang 1 ,
- Rongxia Zhao 1 ,
- Yihe Huang 1 &
- Zhu Liu 1
Light: Science & Applications volume 13 , Article number: 270 ( 2024 ) Cite this article
Metrics details
- Lasers, LEDs and light sources
Rapid synthesis of high-entropy alloy nanoparticles (HEA NPs) offers new opportunities to develop functional materials in widespread applications. Although some methods have successfully produced HEA NPs, these methods generally require rigorous conditions such as high pressure, high temperature, restricted atmosphere, and limited substrates, which impede practical viability. In this work, we report laser solid-phase synthesis of CrMnFeCoNi nanoparticles by laser irradiation of mixed metal precursors on a laser-induced graphene (LIG) support with a 3D porous structure. The CrMnFeCoNi nanoparticles are embraced by several graphene layers, forming graphene shell-encapsulated HEA nanoparticles. The mechanisms of the laser solid-phase synthesis of HEA NPs on LIG supports are investigated through theoretical simulation and experimental observations, in consideration of mixed metal precursor adsorption, thermal decomposition, reduction through electrons from laser-induced thermionic emission, and liquid beads splitting. The production rate reaches up to 30 g/h under the current laser setup. The laser-synthesized graphene shell-encapsulated CrMnFeCoNi NPs loaded on LIG-coated carbon paper are used directly as 3D binder-free integrated electrodes and exhibited excellent electrocatalytic activity towards oxygen evolution reaction with an overpotential of 293 mV at the current density of 10 mA/cm 2 and exceptional stability over 428 h in alkaline media, outperforming the commercial RuO 2 catalyst and the relevant catalysts reported by other methods. This work also demonstrates the versatility of this technique through the successful synthesis of CrMnFeCoNi oxide, sulfide, and phosphide nanoparticles.
Similar content being viewed by others
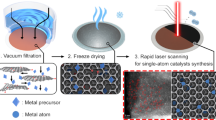
Laser solid-phase synthesis of single-atom catalysts
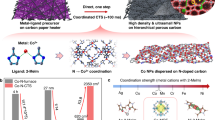
Transient and general synthesis of high-density and ultrasmall nanoparticles on two-dimensional porous carbon via coordinated carbothermal shock
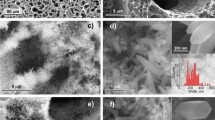
Electrochemical and photoluminescence response of laser-induced graphene/electrodeposited ZnO composites
Introduction.
High-entropy alloys (HEAs) contain five or more principal elements in equimolar or near-equimolar ratios. When the size of HEAs decreases to nanoscale, such as nanoparticles, the high specific surface area, quantum size effect, strong synergistic interaction, and lattice distortion make them an ideal platform for a variety of surface reactions, showing great promise for a wide range of emerging energy-related applications, particularly in the field of catalysis 1 , 2 , 3 , 4 .
There are two categories of methods for the preparation of high-entropy alloy nanoparticles (HEA NPs), generally classified as the “top-down” physical methods by crushing bulk materials and the “bottom-up” chemical methods by reducing metal salt precursors. The HEA NPs prepared by ball milling usually display a large distribution in the composition 5 . Wet chemical synthesis can effectively control the composition, phase structure, and particle size of the target elements 6 , 7 , 8 . However, due to the huge differences in the chemical and physical properties of metal salt precursors (e.g., redox potentials of individual components, thermal decomposition temperature, etc.), it is difficult to achieve simultaneous decomposition or reduction 8 . Therefore, it is prone to producing alloy nanoparticles with severe phase separation 8 , which impedes the HEA material design, mechanism studies and performance optimization.
In recent years, advances in the exploration of rapid and controllable methods for HEA NPs without phase separation have been made 9 , 10 , 11 , 12 , 13 . These methods require high reaction temperatures, and rapid cooling to maintain a high-entropy state at room temperature. As a result, the preparation of high-entropy nanoparticles with a single solid solution can be achieved in a non-equilibrium state by suppressing the formation of secondary phases. In 2018, a carbothermal shock method was reported by Yao et al. to alloy up to eight elements into HEA NPs with the designated composition and size 9 . This method involves a 55-ms heating of mixed metal precursors on carbon supports at a peak temperature of 2000 K in argon and cooling at a cooling rate of ∼ 10 5 K/s. The high temperature ensures uniform mixtures of multiple elements by fission/fusion and catalytically driven particle dispersion mechanisms. This work has made an important step in the rapid synthesis of high-entropy alloy nanoparticles. However, this method can only be applied to conductive, surface-oxidized carbon support materials. Since then, more work has been reported on the rapid synthesis of HEA NPs within milliseconds to seconds, including fast-moving bed heating 10 , electrical sparkling 11 , Joule heating 12 and microwave heating 13 . For example, the microwave heating method with a heating temperature of ∼ 2000 K within 5 s, and a cooling rate of ∼ 10 4 K/s, resulted in the decomposition of the precursors into liquid metal, to form PtPdFeCoNi HEA-NPs, with an average particle size of ∼ 12 nm and uniform elemental mixing 13 . These methods require fast heating and cooling rates, complex heating equipment, and good matrix conductivity or microwave absorption capacity. Therefore, a more cost-effective, versatile, and adaptable technology for large-scale manufacturing of high-entropy alloy nanomaterials is needed to be developed for practical applications.
Laser heating is mainly based on the absorption of laser beams by materials, which can produce a very high temporal temperature and rapid cooling on the surface of the materials in a controllable manner. This offers an alternative way for the rapid synthesis of HEA nanomaterials. Pulsed laser ablation from a solid target immersed in liquid has been considered as a green physical route for scalable nanoparticle fabrication 14 , 15 , 16 . In 2019, Waag et al. reported the fabrication of isolated, colloidal CoCrFeNiMn NPs by picosecond-pulsed laser ablation of a solid CoCrFeNiMn HEA target immersed in a flow cell 16 . This is a typical “top-down” method, which requires the target with the same composition as the HEA NPs to be produced. In 2022, Yao and Zou et al. extended the laser ablation in liquid method, by using a 5 ns pulsed laser to ablate the mixed metal precursor immersed in liquid, leading to synthesizing a series of high-entropy alloy and ceramic nanoparticles loading on various substrates 17 . In addition, Jiang et al. reported the direct fabrication of HEA NPs on carbonaceous support under atmospheric conditions via nanosecond pulsed laser reduction of powdery metal salt precursors in a container, based on laser-induced thermionic emission mechanism 18 , further demonstrating the potential of laser technology in the field of HEA NPs fabrication. In 2023, Li et al. reported another method using a continuous wave 532 nm wavelength laser, under a nitrogen/ambient atmosphere, to synthesize high-entropy alloy, oxide, and nitride nanoparticles on porous carbon nanofiber and graphene oxide-coated glass substrates, and also investigated the laser annealing induced phase transformation behaviors 19 . To date, the laser preparation of HEA NPs is in an early stage. When a laser beam irradiates on a material surface, the temperature distribution along the depth decreases. In addition, due to the large differences in the physiochemical properties of metal salt precursors in terms of decomposition temperature, melting temperature of corresponding metals, etc., the underlying mechanisms involved in laser synthesis are rather complicated. On the other hand, we still need to further explore laser technology to achieve a highly productive and cost-effective laser synthesis method that can be applied for scalable manufacturing of HEA NPs loaded on various material supports.
In this work, we report a laser solid-phase synthesis of HEA NPs on 3D porous laser-induced graphene (LIG), denoted by HEA/LIG. The focus of the work was placed on the mechanism of the laser synthesis in the consideration of laser beam interaction with metal salt precursors and carbon-based materials. We intended to gain an understanding of the HEA NP formation through thermodynamic modeling and experimental measurements in terms of temperature variation and electron emission under laser irradiation, to provide a theoretical basis and a reliable technical route for the rapid laser synthesis of HEA NPs. We chose CrMnFeCoNi, as CrMnFeCoNi is relatively a well-researched alloy among the HEAs, in which both miscible (Cr/Fe) and immiscible metal pairs (Mn/Co) are present. We used the laser-induced 3D porous graphene from polybenzimidazole (PBI) to serve as the host to adsorb mixed metal salt precursors and then subjected it to laser irradiation using a Nd:YAG laser with a wavelength of 1064 nm and a pulse width of 30 ns. The effects of laser fluences and concentrations of the mixed metal precursors on the size of HEA NPs were investigated. We studied the synthesis mechanism through laser-induced photothermal decomposition of the metal precursors in combination with laser-induced thermionic emission reduction. Compared with the laser ablation in liquid, laser solid-phase synthesis offers a new route of HEA NP synthesis which can be characterized by fine controllability, no post-treatment, simple and easy operation, scalability and low cost, and high production rate of 29.6 g/h with the current laser setup. The laser-synthesized CrMnFeCoNi HEA NPs/LIG can be assembled directly to integrated electrodes (IEs), which is denoted by IE-HEA/LIG. The IE-HEA/LIG exhibited higher catalytic activity and exceptional stability toward oxygen evolution reaction (OER) over the commercial RuO 2 /C catalyst, manifesting the potential of the method to produce HEA NPs as a heterogeneous catalyst. This technique has been further extended to the synthesis of CrMnFeCoNi oxide, sulfide, and phosphide, demonstrating its versatility and an industrially viable solution to the challenge of rapid synthesis of HEA and other nanoparticles.
The laser synthesis procedure is illustrated in Fig. 1a . Firstly, metal chlorides (CrCl 3 ·6H 2 O, MnCl 2 , FeCl 3 , CoCl 2 ·6H 2 O and NiCl 2 ·6H 2 O) with equal-molar ratios were dissolved in deionized water. Then, the LIG-coated carbon paper samples, as evidenced by the Raman spectrum (Fig. S1 ), were immersed in the mixed solutions with variable concentrations (1, 5, 10, 15, or 20 mM). The preparation of the LIG was described in our previous work 20 and the Experimental Section. After drying in a vacuum oven at 80 °C for 30 min, the samples were subjected to laser irradiation, using an Nd: YAG laser with a wavelength of 1064 nm and a pulse width of 30 ns, under an Ar atmosphere via rapid scanning at the scanning rate of 2000 mm/s. After the laser treatment, the final samples (HEA/LIG and IE-HEA/LIG) were obtained for further investigations (as detailed in the Experimental Section).
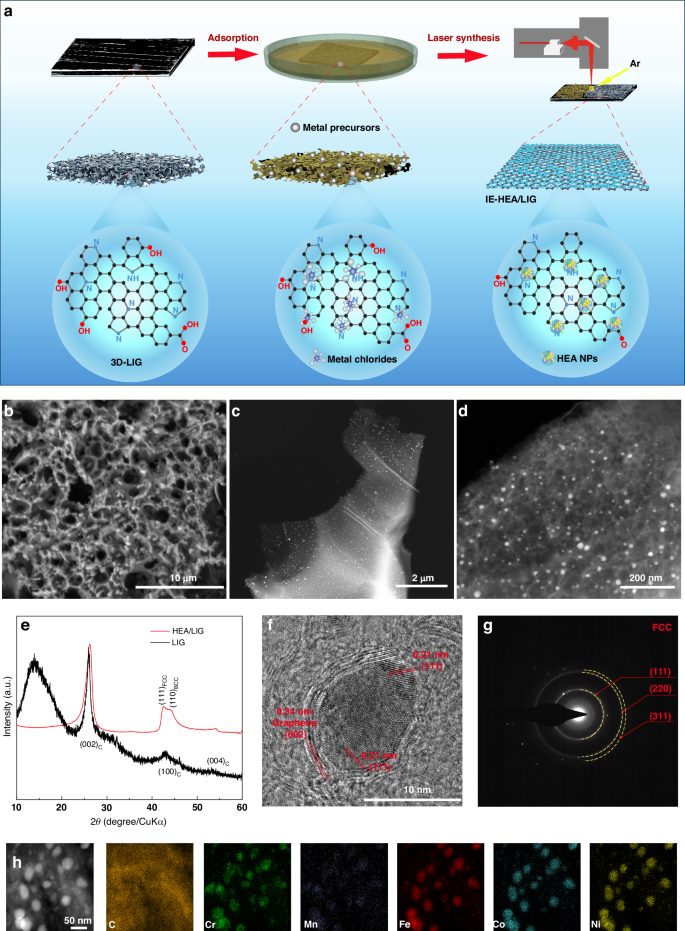
a Schematic diagram of the laser synthesis procedure for the CrMnFeCoNi HEA nanoparticles and IE-HEA/LIG. b FE-SEM images of the metal precursors on LIG. c , d TEM images of the HEA NPs. e XRD spectra of the LIG and HEA/LIG. f , g HR-TEM image of HEA nanoparticles on LIG and the SAED pattern (scale bar = 10 nm in ( f )). h STEM elemental mappings for the HEA/LIG (scale bar = 50 nm)
Characterization of laser-synthesized CrMnFeCoNi NPs
The mixed metal precursor before laser irradiation shows a crystalline structure that does not belong to any of the five metal chlorides (Fig. S2a ). The metal precursors are distributed on the surface and inside the pores of the 3D-LIG (Fig. 1b ). Transmission electron microscope (TEM) investigations of the metal precursors loaded on LIG reveal that the metal precursors are present in the form of irregular particles, around 50 nm and densely loaded on the LIG (Fig. S2b and S2c ). The selected-area electron diffraction (SAED) pattern (Fig. S2d ) shows that the metal precursors are characterized by several diffraction rings of (001), (201), (140), and (402). Energy-dispersive X-ray spectroscopy (EDS) analysis shows all five elements with relatively homogeneous distribution in the particle in Fig. S2e . After the laser treatment, the metal precursors are converted to the HEA nanoparticles, as seen in Fig. 1c , d . The nanoparticles are highly dispersed on the LIG and the particles are uniform in size. The phase composition of the synthesized HEA nanoparticles was examined by X-ray diffraction (XRD) (Fig. 1e ). Only the characteristic peak of C was detected for the LIG samples, while the diffraction peak at 43° appears on the HEA/LIG samples, corresponding to the (111) orientation of the FCC structure. In addition, the high-resolution TEM (HR-TEM) image (Fig. 1f ) and the SAED pattern (Fig. 1g ) show that the nanoparticles are polycrystalline, indexed to (111), (220), and (311), corresponding to the d-spacings of 0.21, 1.94 and 1.75 nm, respectively. The SAED patterns are in agreement with the XRD results, revealing that the crystal structure of CrMnFeCoNi HEA nanoparticles is FCC solid solution. By analyzing the TEM images and EDS elemental mappings (Fig. 1h ), all five elements relatively homogeneously are distributed within the CrMnFeCoNi HEA nanoparticles, in which the contents of the Fe, Co and Ni elements are higher than those of the Cr and Mn (Fig. S3 ), benefiting from their closer reduction potential and fewer valence electrons that form stable compounds while Mn is expected to be partially vaporized during the laser synthesis due to its higher vapor pressure. Moreover, the boiling temperature of 2334 K (Table S3 ) of Mn is the lowest and close to the highest temperature induced by laser, thus the Mn element has a relatively high possibility to evaporate.
The HRTEM images in Fig. 1f and Fig. S4b–d , also show that the HEA nanoparticles are partially embraced by several graphene layers. This is similar to carbon-shell-encapsulated nanoparticles that were reported and achieved by Sharma et al. for improved catalytic durability on electrochemical reactions 21 , 22 .
Figure 2a and Fig. S5 show the variation of the size of CrMnFeCoNi HEA nanoparticles with the concentrations of the metal precursors and laser fluences, with statistical particle size distributions. The particle size becomes bigger with increasing the precursor concentrations, which is more pronounced at lower laser fluences. When the precursor concentration is lower than 0.01 M, the particle size increases with laser fluences. Further increasing the concentrations, the particle size shows insignificant changes with increasing the laser fluences. Overall, the nanoparticles have a uniform size (about 15 nm on average) when the precursor concentration is between 0.005 M and 0.015 M while the average size is significantly increased, reaching up to 23 nm at 0.02 M precursor concentration. Figure 2b–d shows the EDS elemental mappings of the three typical CrMnFeCoNi HEA nanoparticles in different sizes, giving evidence of no apparent phase separation.
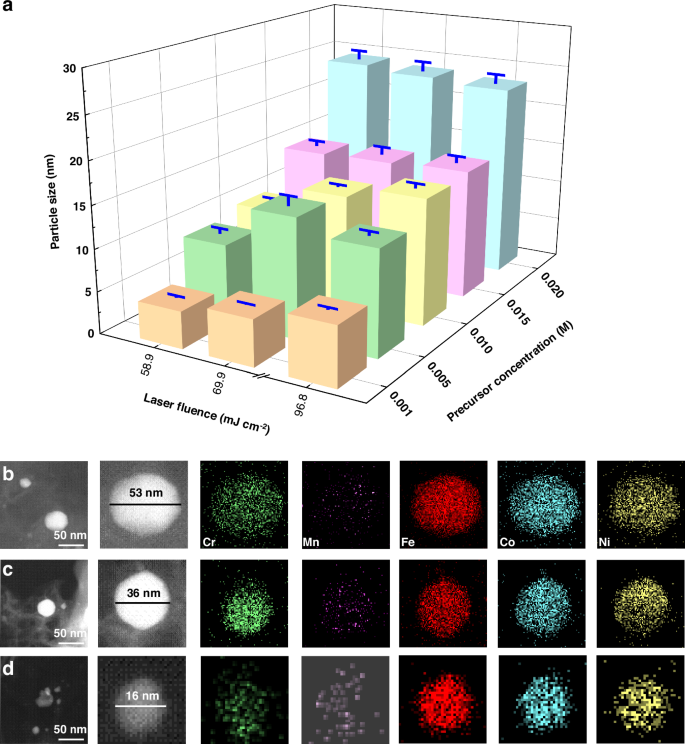
a Particle size varying with laser fluences and precursor concentrations. b – d EDS elemental mappings of the HEA/LIG for different particle sizes
Laser synthesis mechanisms
In order to investigate the laser synthesis mechanism, it is necessary to consider the adsorption behavior of the metal precursors on LIG, in terms of carbon moieties and precursor concentrations. As has been reported before 20 , the LIG has special chemical (surface charge and functional groups, et, al) and physical (surface area, pores, defects, et, al) properties, in addition to the 3D porous structure. In this work, the surface elemental composition and chemical states were further characterized by XPS in Fig. S6 . The results show the N, O, and C elements and carbon moieties including C = C, C-C, C − N, -C = O, O = C-OH, and O-C = O existing on the LIG. Through comparison of the integrated intensity of C, N, and O, the atomic percentages of the three elements are 92.8, 4.97, and 2.23%, respectively. Therefore, the LIG as a host offers abundant adsorption sites to anchor the metal precursors. The adsorption occurs via the mechanisms of complexation 23 , electrostatic interactions 24 , π-cations, and ion exchange 25 . Based on the SEM observation, the adsorption of the metal chlorides on the LIG is in the form of nanoparticles which distribute sparsely and evenly on the LIG, and the particle size is dependent on the concentrations of the metal precursors. At a low concentration such as 0.002 M, the metal chloride monomers (several metal cations and Cl − anions) are attracted or dragged by the active adsorption sites, and the adsorbed metal chlorides are in the sub-nanometer scale due to the low chemical potential and the fast equilibrium between adsorption and desorption of the metal ions. With increasing concentrations, the metal precursors tend to accumulate into larger particles on the LIG (Fig. S7 ).
To understand the interaction between the laser beam and metal precursors as well as the LIG, light transmission spectra of the metal precursors and the metal precursor-adsorbed LIG on carbon paper were measured using UV-Vis Spectroscopy (Fig. S8 ). It reveals that the light transmittance at the wavelength of 1064 nm for the mixed metal salts is 96.77% while it is 0.005% for the metal salt-adsorbed LIG on the carbon paper, suggesting that the metal salts could be considered to be almost transparent to the laser beam, but well absorbed by the LIG on the carbon paper. Therefore, it is likely that upon the laser irradiation, the laser beam goes through the metal precursors to interact with the LIG and generates heat via a photothermal effect, and then the heat conducts to the metal precursors to cause its temperature rise.
The temperature was experimentally recorded and simulated (Fig. 3b–e and Figs. S9 , S10 ). Based on the experimental measurements, at a laser fluence of 58.9 mJ/cm 2 , a scanning rate of 2000 mm/s and a repetition rate of 10 kHz, the localized surface temperature reaches 1960 K. To reveal the thermal evolution, ABAQUS software was used to simulate the temperature dynamics at the same laser operating condition. The simulated temporal thermal profile on the surface in Fig. 3f shows a heating time of 100 μs to reach up to 2381 K, and a cooling rate of 10 7 K/s. In addition, it is known that when a laser beam irradiates on a solid substrate, the laser energy can be converted into heat and the heat will conduct to raise the substrate temperature locally and along the depth of the substrate, the temperature decreases gradually. From the simulation result (Fig. S10b ), it can be seen that the temperature decreases sharply along the depth of the carbon support. Therefore the surface and the interior of the LIG with adsorbed metal precursors experience different thermal evolution.
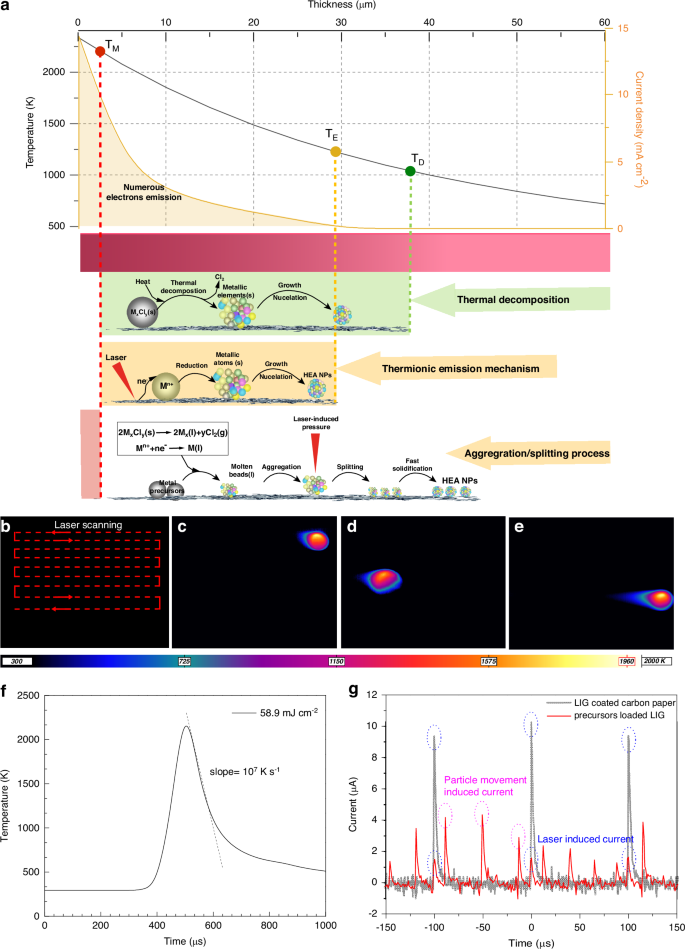
a Schematic diagram of the laser synthesis mechanisms. b Laser scanning method. c–e Temperature recorded (K) in three representative locations during laser irradiation. f Simulated temperature evolution of one point under laser irradiation. g Measured emission current of the LIG and precursors loaded LIG under laser irradiation. Noting: the laser synthesis parameters are of 2000 mm/s scanning rate, 10 kHz pulse repetition rate, 430 μm spot diameter, and 58.9 mJ/cm 2 laser fluences for experiments and simulation in ( b – g )
As shown in Table S2 , the thermal decomposition of the five metal salts occurs at different temperatures. Figure 3a shows the temperature profile along the depth. Once the thermal decomposition temperature (T D ) of the metal precursor is reached but below the melting temperature (T M ) of the corresponding metals, the thermal decomposition of the mixed metal salt particles adsorbed on the LIG occurs accompanied by gas release, and then each CrMnFeCoNi nanoparticle with homogeneous composition is generated by nucleation and growth of the species from the decomposition of each mixed metal salt particle. This particle is likely to be at the site of the original metal salt particle (the light green zone in Fig. 3a ). When the temperature is above T M while below the boiling temperature (T B ) of metals, the metallic elements are in the liquid phase to form molten beads. Since metals are nonwetting with carbon, these liquid metal beads tend to aggregate and grow bigger to minimize their surface energy at high temperatures 26 . However, our observation differs from this behavior, i.e., the size of the CrMnFeCoNi HEA NPs on the surface is on a similar length scale as the nanoparticles found elsewhere. This might be attributed to the laser-induced high pressure 27 , which impacts to the molten beads to cause splitting up the molten beads into small-sized droplets, followed by rapid solidification to form smaller particles (the light red zone in Fig. 3a ).
In addition to the thermal decomposition process described above, there is another process contributing to the formation of CrMnFeCoNi HEA NPs. It is known that graphene is prone to be excited by laser to cause thermionic emission 28 . When a laser beam is absorbed by graphene, electrons from the valence band are excited to the conduction band, and population inversion can be achieved and maintained. Then, hot electrons obtained with sufficient energy can be ejected from the graphene and become free electrons through Auger-like pathways 28 . The emission current can be estimated by Richardson Law 29 , expressed by
Where i is the emission current, S is the emission area, A LIG is Richardson’s constant of the LIG (120 A · cm −2 · K −2 ), ɸ is the work function of the LIG (4.39 eV), k 0 is Boltzmann’s constant (8.617 × 10 −5 eV/K), and T is the local temperature.
To verify the emission of electrons upon the laser irradiation on the LIG, a house-made device was used to detect the ejected electrons from the LIG under the laser irradiation by monitoring the current flow in a circuit (Fig. S11a, b ). The results show that at a bias voltage of 3 kV, the emission current reaches 0.15 μA under ambient conditions (Fig. 3g ). The free electrons play an important role in the formation of CrMnFeCoNi HEA NPs by reducing the metal ions into metal atoms. The calculated current density at different temperatures is plotted in Fig. 3a (the yellow curve). When the temperature is below T E , the current density is considered to be negligible. Towards the surface, the current density increases. At the temperature between T E and T M , the electrons emitted from the LIG support can be captured by the metal ions adsorbed on LIG, leading to the reduction of the metal ions, followed by nucleation and growth to homogeneous nanoparticles, which are also likely to sit on the same site of the original metal salt particles (the light yellow zone in Fig. 3a ). When the temperature is above the melting points of the metallic elements, but lower than the boiling points, T B , the metallic elements are in a liquid phase, forming molten beads which experience the same aggregation and splitting process as discussed above.
As described earlier, the size of the CrMnFeCoNi HEA nanoparticles is strongly dependent on the metal precursor concentrations. This is the result of the increased size of the mixed metal precursor particles with increased concentrations of the metal precursor. On the other hand, the size of the CrMnFeCoNi HEA nanoparticles increases with the laser fluence, which is more pronounced at lower laser fluences. As shown in Fig. 2a , when the concentration of the metal precursors reaches 0.015 mM, the size of the nanoparticles is only slightly increased. This could be explained based on the mechanism described earlier, as follows. With the increasing laser fluence, the temperature above T M has a bigger spatial and temporal region (the light red zone becomes bigger in Fig. 3 ), thus the aggregation of molten beads should become more easily to form even bigger beads. However, the experimental observation confirmed the uniformity of the nanoparticle size, attributing to the more effective splitting driven by the higher laser-induced pressure with laser fluences.
Application in electrocatalysis
Over the last few years, there has been a significant increase in the applications of HEAs in electrocatalysis 30 , 31 , 32 . The emergence of HEAs has shown their unique capability of overcoming the limitations of transition metal alloys in acidic or alkaline environments due to their remarkable resistance to corrosion and toxicity during the electrochemical reaction process 32 . Currently, most electrocatalysts are powder-based. Electrode preparation follows a coating route involving the use of low-conductive polymeric binders, which may elevate resistance levels, block active sites, impede mass transport, and consequently lead to a degradation in catalytic performance 33 , 34 . Additionally, continuous gas evolution during electrochemical reactions may also result in detachment of the electrocatalysts. Therefore, it is highly desirable to develop a method to fabricate binder-free integrated electrocatalytic electrodes that is time-efficient, versatile, green, low-cost, and suitable for large-scale production.
In this work, the laser-synthesized CrMnFeCoNi HEA nanoparticles on LIG-coated carbon paper were used directly as electrocatalytic electrodes for OER to evaluate its electrocatalytic activity and stability in 1 M KOH alkaline media. The linear sweep voltammogram (LSV) curves of the purchased carbon paper, commercial RuO 2 , and IE-HEA/LIG sample after ohmic-drop correction are shown in Fig. 4a . The carbon paper shows negligible current change under the applied voltage from 1.2 to 1.7 V vs RHE. The IE-HEA/LIG exhibits enhanced OER activity compared to the commercial RuO 2 . The overpotentials of IE-HEA/LIG to drive anodic current densities of 10, 50, and 100 mA/cm 2 are 293, 324, and 342 mV, respectively, which are 312, 364, and 401 mV for the commercial RuO 2 . Figure 4b shows the OER kinetics derived from LSV of commercial RuO 2 and the IE-HEA/LIGs. The Tafel slopes are 83.1 and 57.9 mV/dec for commercial RuO 2 and IE-HEA/LIG, respectively, signifying the superior OER rate of the IE-HEA/LIG. The enhanced activity and kinetic of IE-HEA/LIG derive from the solid-solution structure and multiple active sites of the HEA nanoparticles, bringing about more OER paths and lower energy barriers for such reactions.
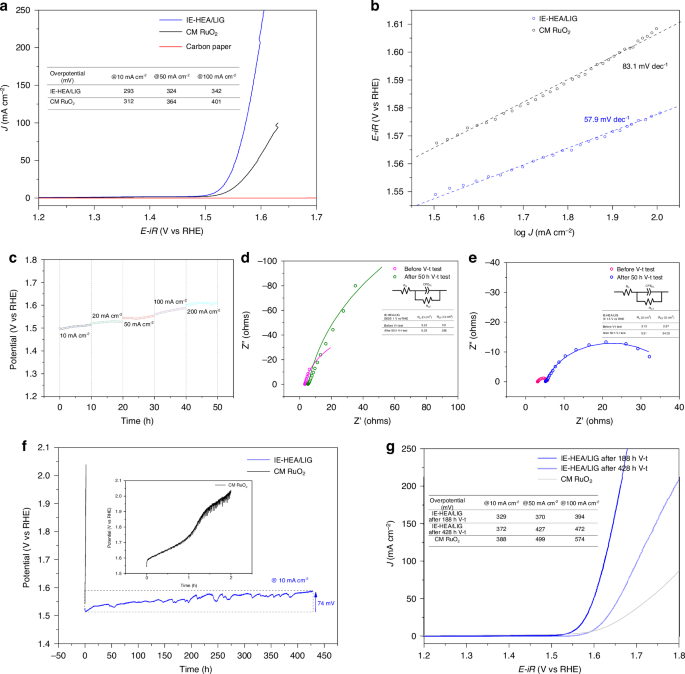
a LSV plots, b Tafel slopes, c V-t curves at 10, 20, 50, 100, 200 mA/cm −2 , d , e Nyquist plots at 1 V and 1.5 V vs. RHE, f Long-term stability at 10 mA/cm −2 , g LSV plots after long-term V-t tests
Figure 4c shows the chronopotentiometric (V-t test) results. The test was conducted on the IE-HEA/LIG samples in a continuous mode for 10 h at 10, 20, 50, 100, and 200 mA/cm 2 , respectively. The potentials remain stable at each current density stage and the IE-HEA/LIG shows excellent stability at high current densities. Electrochemical Impedance Spectroscopy (EIS) measurements were performed on the IE-HEA/LIGs before and after the 50 h stability test at an applied potential of 1 and 1.5 V vs. RHE, respectively, to measure the charge transfer resistance ( R CT ) and evaluate the catalyst/electrolyte interface characteristics. The Nyquist plots and fitting results of the samples in Fig. 4d , e indicate the R CT values of the samples vary with the applied potential and the experimental conditions. Applied with 1 V vs. RHE, the samples have no external force to drive the OER and the electrochemical reaction rate is rather slow, resulting in big R CT (Fig. 4d ) for the sample before and after the V-t test. By comparison, OER is triggered and oxygen bubbles are continuously released on the sample when applied with the potential of 1.5 V vs. RHE, thus the R CT values are small and the electrochemical reaction is running fast. Accompanied by oxygen bubbles, the HEA is oxidized (Fig. S12 , Supporting Information). The oxides change the dielectric and electric double-layer properties of the solid/liquid interface, resulting in a small RC constant and a curved Nyquist plot in Fig. 4e . For the two different applied potentials, the R CT after V-t test is consistently bigger than the values before V-t test. This result can probably be attributed to the oxidation of the HEA catalyst during V-t test. The oxides and hydroxides play a role in increasing the impedance of the OER process. However, they do not suffocate the HEA catalyst for producing oxygen.
To investigate the stability of the IE-HEA/LIGs, long-life V-t tests were conducted and the results are shown in Fig. 4f . The V-t result of commercial RuO 2 was also recorded as a comparison. In Fig. 4f , at 10 mA/cm 2 , the potential of commercial RuO 2 increases sharply within 2 h and reaches over 2 V. Whereas, for IE-HEA/LIGs, the potential has a small rise of about 74 mV for 428 h, showing its excellent stability. The increase of the potential likely originates from the developed oxides/hydroxides and the corresponding electrochemical impedance. Also, the LSV curves of IE-HEA/LIGs after 188 and 428 h V-t tests (Fig. 4g ) show that the overpotentials decay reasonably. However, the overpotential at 10 mA/cm 2 of IE-HEA/LIGs after 428 h V-t test is 372 mV which is still smaller than that (388 mV) of commercial RuO 2 after only 2 h test. Consistently, the overpotential of 372 mV is approximately the sum of the initial overpotential of 293 mV before V-t test and the elevated 74 mV during V-t test. Experimentally, we observed that accompanied by rapid oxygen release, the commercial RuO 2 sample disintegrated within 2 h and black pieces of carbon paper floated on the top of the electrolyte. This phenomenon can also be observed on the IE-HEA/LIGs after 200 h, just with less debris. The disintegration of carbon paper support can be attributed to the rapid oxygen gas evolution rate during electrolysis.
Overall, the OER performance of the IE-HEA/LIG in this work is excellent, outweighing most of the non-precious HEA catalysts reported up to date (Table S4 ). It is worth noting that the stability of the laser-synthesized IE-HEA/LIG outperforms most of the precious and non-precious HEA catalysts.
As described above, during laser irradiation, the conversion of the HEA NPs from the mixed metal precursors adsorbed on LIG involves several processes, including thermal decomposition, metal ion reduction, metallic element melting, molten bead fusion, and fission, depending on the temperature experience. The laser synthesis in this work is based on solid-phase, which is different from liquid-phase synthesis. As the mixed metal precursor is adsorbed on the 3D porous LIG structure and dried before laser irradiation, the size of the nanoparticles can be well controlled by the laser fluence, and the concentration of the precursor due to the limited mobility of the reduced metal species and subsequently the nanoparticles.
The laser-synthesized CrMnFeCoNi nanoparticles are embraced by several graphene layers, forming graphene shell-encapsulated nanoparticles. This might be caused by the bending of graphene layers subjected to laser heating, which was also reported in the work on graphene nanosphere formation by microwave heating 35 . Embracing the metal nanoparticles by graphene layers is beneficial to the dispersion of the nanoparticles by preventing nanoparticles from aggregation. On the other hand, the analysis of the LIG indicates that the LIG is characterized by a high density of edge planes (Fig. S4c , Supporting Information), which are highly defective, offering preferred sites to immobilize the HEA nanoparticles (Fig. S4d , Supporting Information). This phenomenon was also reported in the synthesis of metallic nanoparticles on carbon supports through the Joule heating method 36 . Both characteristics of the laser-synthesized CrMnFeCoNi HEA nanoparticles on LIG support described above offer a reinforced effect on the nanoparticles with strong adhesion to the LIG, which might contribute to the high stability of the CrMnFeCoNi HEA nanoparticles catalysts in catalytic applications.
The electrocatalytic performance described in this work is superior to most of the non-precious HEA catalysts reported up to date. Particularly, the stability of the laser-synthesized IE-HEA/LIG outperforms most of the precious and non-precious HEA catalysts. This might be explained in consideration of the following aspects. Firstly, the laser-synthesized CrMnFeCoNi HEA nanoparticles are the form of the graphene shell-encapsulated. This hybrid structure offers benefits for enhancing the electronic transport from the LIG to the active sites, and the incomplete wrap (Fig. 1f and Fig. S4a, b ) benefits the exposure of the HEA NPs active sites, lowering the barrier of electrons transfer in electrolysis. During the OER performance, the physical constraint effect also prevents nanoparticles from aggregating and peeling off from the LIG support under the harsh working environment, thus enhancing the durability of the catalyst. On the other hand, the carbon shell directly acts as an active site due to the electronic structure modulated by the core metal NP, which is beyond the scope of this paper. Secondly, the laser-synthesized CrMnFeCoNi HEA nanoparticles on LIG support are binder-free, which avoids the deterioration of the mass transfer and electronic conduction caused by the use of organic binder. Thirdly, the 3D interconnected porous structure of the LIG remains unchanged during the laser synthesis for enhanced electronic and mass transferring ability of the IEs.
Extension to HEA oxide, phosphide, and sulfide nanoparticles
Taking advantage of the laser synthesis that produces a non-equilibrium state via instantaneous heating and cooling, we successfully synthesized the CrMnFeCoNi HEA oxide, phosphide, and sulfide nanoparticles. The TEM images in Fig. S13 show the oxide, phosphide, and sulfide nanoparticles have comparable size to the HEA nanoparticles. EDS elemental mapping images show the homogeneous mixing of oxygen, phosphorus, and sulfur elements in the quinary HEA elements. The incorporation of these nonmetallic atoms in HEA structure may result in severe lattice distortion, which might contribute to more exposure of metallic active sites, enhancing the electrocatalytic performance 37 , 38 , 39 .
In summary, we have demonstrated a laser solid-phase synthesis of high-entropy material nanoparticles by laser irradiation on a LIG substrate with a 3D porous structure. The laser-synthesized CrMnFeCoNi HEA nanoparticles on LIG support are in the hybrid form of graphene shell encapsulation. Our strategy provides simplicity, generality, and tunability to synthesize structural-uniform nanomaterials which consist of immiscible elements. The HEA/LIG is synthesized by laser-induced photothermal effect and thermionic emission, offering an active exploration in understanding the thermal decomposition process and electron emission-induced reduction of metal precursors for thermal-based techniques. Also, the CrMnFeCoNi nanoparticles loaded on LIG support can be directly used as 3D binder-free IEs, embodying the scalability of this synthesis technique. The IE-HEA/LIGs exhibit excellent electrocatalytic activity towards OER, especially the electrochemical stability. Our method is economically feasible and technically viable to synthesize composition-tunable nanomaterials, which have broad potential in energy and environmental applications.
Materials and methods
Sample preparation.
Chromium (III) chloride hexahydrate (CrCl 3 ·6H 2 O, 98%), manganese chloride (MnCl 2 , 99%), ferric (III) chloride (FeCl 3 , 99%), cobalt (II) chloride hexahydrate (CoCl 2 ·6H 2 O, 99%), nickel (II) chloride hexahydrate (NiCl 2 ·6H 2 O, 99.3%), ruthenium (IV) oxide (RuO 2 , >85%), polybenzimidazoles (PBI) and N, N-dimethylacetamide (DMAC) were purchased from Casmart and used as received. All chemicals were used as received without further purification.
Firstly, a homogeneous casting solution was prepared by mixing PBI (10 wt%) and DMAC (90 wt%) with continuous stirring and heating at 130 °C. Then, a thin film (40 μm in thickness) was cast on carbon paper by using a doctor blade. After that, the film was placed in deionized water vapor for 1 min before being dried under ambient conditions. After drying, the phase separation finished and the hierarchically porous PBI layers were prepared on carbon paper.
Secondly, a nanosecond laser (having a wavelength of 1064 nm, an average power of 500 W, a pulse length of 30 ns, and a laser beam diameter of 430 μm at the focusing position, JPT Opto-electronics Co., LTD., Shenzhen) was used to treat the phase-separated PBI layer. The carbonization treatment was carried out under ambient conditions with a fluence of 88 mJ/cm 2 , a 10 kHz pulse repetition rate, and a 100 mm/s scanning rate. The production is denoted by LIG using carbon paper as the substrate.
Thirdly, five metal chlorides with equal elemental molar ratios were dissolved in absolute ethanol to prepare salt solutions with concentrations of 1, 5, 10, 15, and 20 mM. Then, the LIG-coated carbon paper samples were immersed in solutions for 8 h and dried in a vacuum at 80 °C for 1 h. Then, the samples were placed in a sealed box filled with Ar gas and irradiated by the same laser as mentioned above. The laser synthesis was conducted with a variation of laser powers (15, 40, and 65 W) and fixed pulse repetition rate of 10 kHz, laser beam diameter of 430 μm, and scanning rate of 2000 mm/s. Finally, the IE with HEA nanoparticles loaded on LIG-coated carbon paper (HEA/LIG) were obtained.
Materials characterization
Scanning electron microscopy (SEM) images were obtained by FEI Quanta 250 FEG equipped with an EDS. XRD spectrum was taken by 2D small angle X-ray scatterometer (SAXA, Xeuss 3.0 UHR) using a Cu Kα source. X-ray photoelectron spectroscopy (XPS) was taken by Kratos AXIS Supra spectrometer. Transmission electron microscopy (TEM, JEOL F200) was used to gain insight into the microstructure and elemental distribution, including dark-field (DF), high-resolution TEM (HR-TEM), SAED. All TEM samples (powders scraped off from IE-HEA/LIGs) were dispersed and sonicated in ethanol as a dilution solution and drop-casted onto the copper grid with a lacy carbon film.
Temperature measurement and simulation
The time-dependent temperature profile of the precursor-loaded LIG under laser irradiation was recorded using an infrared camera (Multifunctional X-ray Photoelectron Spectroscope, AXIS ULTRA DLD) with a measurement range up to 2000 °C.
A two-dimensional thermal-mechanical coupled transient model was established using commercial software (ABAQUS 2018, Dassault Systemes Simulia Corp, USA). Considering the complex process and the computational efficiency, certain assumptions are made in this numerical model: (1) The laser heating source is Gaussian distribution in space; (2) The carbon paper is considered to be homogeneous and isotropic, and the laser absorptivity is regarded as a constant; (3) Effective convective heat transfer inside the molten pool was modeled without directly modeling the material flow. As shown in Fig. S9a , a rectangle is built, sized by 5.00 × 5.00 × 0.25 mm 3 along X, Y, and Z directions. The laser beam scans along the positive X-axis with laser fluences of 58.9, 69.9 and 96.8 mJ/cm 2 , a scanning rate of 2000 mm/s, and a laser beam diameter of 0.43 mm. The coordinate values of the start and end points are (−3.5, 2.5, 0.25) and (−1.5, 2.5, 0.25), respectively. A hexahedral structure grid is employed and the localized area-related laser path is mesh-refined to improve the calculation accuracy. The physical properties of the carbon-based material are listed in Table S1 .
In this model, the conduction inside the material, surface emission, and convection between the protective gas and processed material are considered following Fourier’s Law:
where ρ m , C m , and λ are the density, specific heat capacity, and thermal conductivity. Q laser is the internal heating source, which can be numerically expressed as:
where A is the laser beam absorptivity, 0.56. P Laser , r 0 , and V Laser are the laser power, spot radius, and scanning rate, respectively.
The convection Q c and surface emission Q r can be described as:
where h and ε are the convective coefficient and thermal emissivity, respectively. k is the Steffen-Boltzmann constant with a value of 5.67 × 10 −8 W/(m 2 · k 4 ).
The latent heat α m considering the phase change from solid to liquid can be expressed as:
where ρ s and ρ l are the solid and liquid density, respectively. θ is the phase indicator, which can be given as:
where T s and T l are the solid and liquid temperatures, respectively.
Thermionic emission measurement
The measurement set-up of thermionic emission is shown in Fig. S7a , including components of a high voltage source (Teslaman, TCM6002), precision sampling resistor (30 kΩ, 1/4 W), high-speed oscilloscope (Keysight DSO-X 3034 A) and a homemade metal-insulator structure for sample holding. The LIG samples, aluminum cathode, and anode were mounted under ambient conditions. The anode has a 10 mm diameter hole in the center and is placed 3 mm away from the cathode which is separated by a glass insulating spacer. The high voltage source can bias the anode with voltages from 0 V to 3 kV. For current measurement, the voltage on resistor R1 was measured by a differential probe and was recorded by the high-speed oscilloscope. Thanks to the ultra-low parasitic inductance of the precision resistor and wide-bandwidth differential probe, the RC constant of the current measurement system is much less than the pulse width. Therefore, the current can be directly calculated by Ohm’s law, as illustrated in Fig. S7b . Without laser illumination, the static current of both carbon paper and precursors are close to zero, which confirms the thermionic emission around 300 K can be neglected. When the nanosecond pulse laser was illuminating towards carbon paper with a 10 kHz repetition rate, a strong current signal (10 μA) could be observed. We believe this current signal was caused by the thermionic emitting of electrons from carbon paper because the measured current pulse width (3–5 μs) is orders of magnitude wider than the laser pulse (30 ns). After the substrate was changed from the carbon paper to the precursor loaded-LIG, the measured pulse current was ~5 times lower than the carbon paper and many random current peaks (cannot be aligned with laser signal) were observed in Fig. S7b . This finding indicates that the electrons induced by the laser irradiation were gained by the metal precursors which were reduced at the same time, resulting in the in-situ formation of metal atoms.
Electrocatalytic performance
The evaluation of OER performance was carried out by a conventional three-electrode system using the VersaSTAT 4 potentiostat (AMETEK, USA). An Ag/AgCl (saturated KCl solution) electrode was used as the reference electrode, a platinum wire as the counter electrode, and the IE-HEA/LIG (0.25 cm 2 , with catalyst loading of 6.969 mg/cm 2 ) as the working electrode. The measured potentials were converted to the reversible hydrogen electrode (RHE) potentials by the following equation:
Where \({{\boldsymbol{E}}}_{{\boldsymbol{RHE}}}\) is the measured potential against RHE potentials, \({{\boldsymbol{E}}}_{{\boldsymbol{Ag}}{\boldsymbol{/}}{\boldsymbol{AgCl}}}\) is the measured potential against the reference electrode, and \({{\boldsymbol{E}}}_{{\boldsymbol{Ag}}{\boldsymbol{/}}{\boldsymbol{AgCl}}}^{{\boldsymbol{0}}}\) equals 0.197 V at 298 K.
The Ohmic drops within the system were compensated by applying IR-correction. The electrolyte resistance was determined by EIS at the open-circuit potential. The EIS was measured in a range of 100 kHz to 1 Hz, with a perturbation of 10 mV. The system resistance was then determined at the x-intercept of the Nyquist plot. Meantime, the Nyquist plots were recorded during OER at an overpotential of 0.5 V vs. Ag/AgCl electrode in a range of 100 kHz to 1 Hz to evaluate the catalyst/electrolyte interface characteristics.
The OER performances were tested in O 2 saturated 1 M KOH electrolyte. The catalyst was activated using cyclic voltammetry (CV) in the range of 0 to 0.7 V vs. Ag/AgCl electrode at a scanning rate of 50 mV/s until the CV loops overlapped. The LSV curves were measured at a scanning range of 0 to 0.7 V vs Ag/AgCl. The electrodes were directly used as working electrodes.
To evaluate the OER durability of the IE, chronopotentiometric measurements at different constant current densities of 10, 20, 50, 100, and 200 mA/cm 2 were carried out in O 2 saturated 1.0 M KOH electrolyte.
The OER performances of purchased carbon paper and commercial RuO 2 were measured using the same experimental setups. The purchased carbon paper can be directly used as the working electrode (0.25 cm 2 ). For commercial RuO 2 , the powders (1 mg) were dispersed in a solution containing 900 μL of ethanol and 100 μL of 5 wt% Nafion, followed by sonication for 30 min. Next, 17.4 μL RuO 2 catalyst ink was dropped onto carbon paper (0.25 cm 2 ) to generate a catalyst loading of ~6.969 mg/cm 2 . After drying, the RuO 2 catalyst-loaded carbon paper was used as the working electrodes.
Zhao, K. N. et al. High-entropy alloy nanocatalysts for electrocatalysis. Acta Phys. Chim. Sin. 37 , 2009077 (2021).
Google Scholar
Gao, M. C. et al. High-entropy alloys in hexagonal close-packed structure. Metall. Mater. Trans. A 47 , 3322–3332 (2016).
Article Google Scholar
Yusenko, K. V. et al. First hexagonal close packed high-entropy alloy with outstanding stability under extreme conditions and electrocatalytic activity for methanol oxidation. Scr. Mater. 138 , 22–27 (2017).
Choi, C. et al. A highly active star decahedron Cu nanocatalyst for hydrocarbon production at low overpotentials. Adv. Mater. 31 , 1805405 (2019).
Rekha, M. Y., Mallik, N. & Srivastava, C. First report on high entropy alloy nanoparticle decorated graphene. Sci. Rep. 8 , 8737 (2018).
Article ADS Google Scholar
Zhou, S., Jackson, G. S. & Eichhorn, B. AuPt alloy nanoparticles for CO-tolerant hydrogen activation: architectural effects in Au-Pt bimetallic nanocatalysts. Adv. Funct. Mater. 17 , 3099–3104 (2007).
Alayoglu, S. & Eichhorn, B. Rh−Pt bimetallic catalysts: synthesis, characterization, and catalysis of core−shell, alloy, and monometallic nanoparticles. J. Am. Chem. Soc. 130 , 17479–17486 (2008).
Liu, M. M. et al. Entropy-maximized synthesis of multimetallic nanoparticle catalysts via a ultrasonication-assisted wet chemistry method under ambient conditions. Adv. Mater. Interfaces 6 , 1900015 (2019).
Yao, Y. G. et al. Carbothermal shock synthesis of high-entropy-alloy nanoparticles. Science 359 , 1489–1494 (2018).
Gao, S. J. et al. Synthesis of high-entropy alloy nanoparticles on supports by the fast moving bed pyrolysis. Nat. Commun. 11 , 2016 (2020).
Kim, K. S. et al. Continuous synthesis of high-entropy alloy nanoparticles by in-flight alloying of elemental metals. Nat. Commun. 15 , 1450 (2024).
Ahn, J. et al. Rapid joule heating synthesis of oxide-socketed high-entropy alloy nanoparticles as CO 2 conversion catalysts. ACS Nano 17 , 12188–12199 (2023).
Qiao, H. Y. et al. Scalable synthesis of high entropy alloy nanoparticles by microwave heating. ACS Nano 15 , 14928–14937 (2021).
Yang, G. W. Laser ablation in liquids: applications in the synthesis of nanocrystals. Prog. Mater. Sci. 52 , 648–698 (2007).
Amendola, V. et al. Formation of alloy nanoparticles by laser ablation of Au/Fe multilayer films in liquid environment. J. Colloid Interface Sci. 489 , 18–27 (2017).
Waag, F. et al. Kinetically-controlled laser-synthesis of colloidal high-entropy alloy nanoparticles. RSC Adv. 9 , 18547–18558 (2019).
Wang, B. et al. General synthesis of high-entropy alloy and ceramic nanoparticles in nanoseconds. Nat. Synth. 1 , 138–146 (2022).
Jiang, H. Q. et al. Nanoalloy libraries from laser-induced thermionic emission reduction. Sci. Adv. 8 , eabm6541 (2022).
Li, Y. et al. Laser Annealing-induced phase transformation behaviors of high entropy metal alloy, oxide, and nitride nanoparticle combinations. Adv. Funct. Mater. 33 , 2211279 (2023).
Huang, Y. H. et al. Laser direct writing of heteroatom (N and S)-doped graphene from a polybenzimidazole ink donor on polyethylene terephthalate polymer and glass substrates. Small 14 , 1803143 (2018).
Sharma, M. et al. Work function-tailored graphene via transition metal encapsulation as a highly active and durable catalyst for the oxygen reduction reaction. Energy Environ. Sci. 12 , 2200–2211 (2019).
Yoo, J. M. et al. Carbon shell on active nanocatalyst for stable electrocatalysis. Acc. Chem. Res. 55 , 1278–1289 (2022).
Moreno-Castilla, C. Adsorption of organic molecules from aqueous solutions on carbon materials. Carbon 42 , 83–94 (2004).
Li, H. B. et al. Mechanisms of metal sorption by biochars: biochar characteristics and modifications. Chemosphere 178 , 466–478 (2017).
Yang, X. D. et al. Surface functional groups of carbon-based adsorbents and their roles in the removal of heavy metals from aqueous solutions: a critical review. Chem. Eng. J. 366 , 608–621 (2019).
Eustathopoulos, N., Nicholas, M. G. & Drevet, B. Wettability at High Temperatures. (Amsterdam: Pergamon, 1999).
Sha, Y. et al. 3D binder-free integrated electrodes prepared by phase separation and laser induction (PSLI) method for oxygen electrocatalysis and zinc–air battery. Adv. Energy Mater. 12 , 2200906 (2022).
Zhang, T. F. et al. Macroscopic and direct light propulsion of bulk graphene material. Nat. Photonics 9 , 471–476 (2015).
Wei, X. L. et al. Breakdown of Richardson’s law in electron emission from individual self-joule-heated carbon nanotubes. Sci. Rep. 4 , 5102 (2014).
Qiu, H. J. et al. Noble metal-free nanoporous high-entropy alloys as highly efficient electrocatalysts for oxygen evolution reaction. ACS Mater. Lett. 1 , 526–533 (2019).
Zhang, G. L. et al. High entropy alloy as a highly active and stable electrocatalyst for hydrogen evolution reaction. Electrochim. Acta 279 , 19–23 (2018).
He, B. B., Zu, Y. & Mei, Y. Design of advanced electrocatalysts for the high-entropy alloys: principle, progress, and perspective. J. Alloy. Compd. 958 , 170479 (2023).
Chandrasekaran, S. et al. Developments and perspectives on robust nano- and microstructured binder-free electrodes for bifunctional water electrolysis and beyond. Adv. Energy Mater. 12 , 2200409 (2022).
Yan, X. X., Ha, Y. & Wu, R. B. Binder-free air electrodes for rechargeable zinc-air batteries: recent progress and future perspectives. Small Methods 5 , 2000827 (2021).
Yan, Z. X. et al. Graphene nanosphere as advanced electrode material to promote high performance symmetrical supercapacitor. Small 17 , 2007915 (2021).
Huang, Z. N. et al. Direct observation of the formation and stabilization of metallic nanoparticles on carbon supports. Nat. Commun. 11 , 6373 (2020).
Zhang, L. J., Cai, W. W. & Bao, N. Z. Top-level design strategy to construct an advanced high-entropy Co–Cu–Fe–Mo (Oxy)hydroxide electrocatalyst for the oxygen evolution reaction. Adv. Mater. 33 , 2100745 (2021).
Cui, M. J. et al. High-entropy metal sulfide nanoparticles promise high-performance oxygen evolution reaction. Adv. Energy Mater. 11 , 2002887 (2021).
Liu, K. W. et al. High-performance transition metal phosphide alloy catalyst for oxygen evolution reaction. ACS Nano 12 , 158–167 (2018).
Download references
Acknowledgements
We would like to thank the financial support from Ningbo Yongjiang Science and Technology Programme (2023A-161-C). We would also like to express our gratitude to the Analytical Center, Ningbo Institute of Materials Technology and Engineering, Chinese Academy of Sciences, for the assistance in the characterization work.
Author information
Authors and affiliations.
Research Centre for Laser Extreme Manufacturing, Ningbo Institute of Materials Technology and Engineering, Chinese Academy of Sciences, Ningbo, 315201, China
Yuxiang Liu, Jianghuai Yuan, Jiantao Zhou, Kewen Pan, Ran Zhang, Rongxia Zhao, Lin Li, Yihe Huang & Zhu Liu
You can also search for this author in PubMed Google Scholar
Contributions
Yuxiang Liu designed the experiments, performed the experiments, analyzed the data, and wrote the paper. Jianghuai Yuan: performed the experiments and analyzed the data; Jiantao Zhou performed the modeling work; Kewen Pan and Ran Zhang performed the experiments and analyzed the data; Rongxia Zhao analyzed the data; Lin Li supervised the project and edited the manuscript; Yihe Huang designed the experiments, performed the experiments and analyzed the data; Zhu Liu conceived the concept and designed the experiments, wrote the paper and supervised the project.
Corresponding authors
Correspondence to Yihe Huang or Zhu Liu .
Ethics declarations
Conflict of interest.
L.L. serves as an Editor for the Journal. No other author has reported any other conflict of interest.
Supplementary information
41377_2024_1614_moesm1_esm.docx.
Supplementary information for Supplementary Information for Laser solid-phase synthesis of graphene shell-encapsulated high-entropy alloy nanoparticles
Rights and permissions
Open Access This article is licensed under a Creative Commons Attribution 4.0 International License, which permits use, sharing, adaptation, distribution and reproduction in any medium or format, as long as you give appropriate credit to the original author(s) and the source, provide a link to the Creative Commons licence, and indicate if changes were made. The images or other third party material in this article are included in the article’s Creative Commons licence, unless indicated otherwise in a credit line to the material. If material is not included in the article’s Creative Commons licence and your intended use is not permitted by statutory regulation or exceeds the permitted use, you will need to obtain permission directly from the copyright holder. To view a copy of this licence, visit http://creativecommons.org/licenses/by/4.0/ .
Reprints and permissions
About this article
Cite this article.
Liu, Y., Yuan, J., Zhou, J. et al. Laser solid-phase synthesis of graphene shell-encapsulated high-entropy alloy nanoparticles. Light Sci Appl 13 , 270 (2024). https://doi.org/10.1038/s41377-024-01614-y
Download citation
Received : 24 May 2024
Revised : 16 August 2024
Accepted : 28 August 2024
Published : 26 September 2024
DOI : https://doi.org/10.1038/s41377-024-01614-y
Share this article
Anyone you share the following link with will be able to read this content:
Sorry, a shareable link is not currently available for this article.
Provided by the Springer Nature SharedIt content-sharing initiative
Quick links
- Explore articles by subject
- Guide to authors
- Editorial policies


COMMENTS
Table of contents. Step 1: Define your variables. Step 2: Write your hypothesis. Step 3: Design your experimental treatments. Step 4: Assign your subjects to treatment groups. Step 5: Measure your dependent variable. Other interesting articles. Frequently asked questions about experiments.
In science, the experimental setup is the part of research in which the experimenter analyzes the effect of a specific variable. This setup is quite similar to the control setup; ideally, the only difference involves the variable that the experimenter wants to test in the current project. Consider a scenario in which a researcher wants to ...
A good experimental design requires a strong understanding of the system you are studying. There are five key steps in designing an experiment: Consider your variables and how they are related. Write a specific, testable hypothesis. Design experimental treatments to manipulate your independent variable.
1) True Experimental Design. In the world of experiments, the True Experimental Design is like the superstar quarterback everyone talks about. Born out of the early 20th-century work of statisticians like Ronald A. Fisher, this design is all about control, precision, and reliability.
The practical steps needed for planning and conducting an experiment include: recognizing the goal of the experiment, choice of factors, choice of response, choice of the design, analysis and then drawing conclusions. This pretty much covers the steps involved in the scientific method. Recognition and statement of the problem.
Experimental design is a process of planning and conducting scientific experiments to investigate a hypothesis or research question. It involves carefully designing an experiment that can test the hypothesis, and controlling for other variables that may influence the results. Experimental design typically includes identifying the variables that ...
Actually, a deeper knowledge on the topic could offer a different point of view. The aim of the present tutorial is to introduce the experimental design to beginners, by providing the theoretical basic principles as well as a practical guide to use the most common designs, from the experimental plan to the final optimization.
Definition. An experimental setup refers to the specific arrangement and conditions in which an experiment is conducted to investigate a hypothesis or research question. It involves manipulating independent variables, measuring dependent variables, and controlling extraneous factors.
1. 4. Experimental design 1. 4. 1. The role of experimental design Experimental design concerns the validity and efficiency of the experiment. The experimental design in the following diagram (Box et al., 1978), is represented by a movable window through which certain aspects of the true state of nature, more or less distorted by noise, may be ...
Slight variations in the experimental set-up could strongly affect the outcome being measured. For example, during the 1950s, a number of experiments were conducted to evaluate the toxicity in mammals of the metal molybdenum, using rats as experimental subjects. Unexpectedly, these experiments seemed to indicate that the type of cage the rats ...
As discussed in Sect. 1.2, creating experimental setups is a fundamental step in a physicist's discovery process. Creating experimental setups for quantum systems is particularly challenging, since the behavior of such systems is often unintuitive. In the last view years, automated search techniques and reinforcement-learning based schemes ...
If you're seeing this message, it means we're having trouble loading external resources on our website. If you're behind a web filter, please make sure that the domains *.kastatic.org and *.kasandbox.org are unblocked.
Designing an experiment means planning exactly how you'll test your hypothesis to reach valid conclusions. This video will walk you through the decisions you...
4. Conduct the experiment. We will use each aerosol spray to fire ten projectiles, using the same amount of aerosol spray to fire each projectile. After each firing, we will use a long tape measure to measure the range our projectile traveled. Record this data in the data table.
In science, an experiment is a procedure that tests a hypothesis. In science, an experiment is simply a test of a hypothesis in the scientific method. It is a controlled examination of cause and effect. Here is a look at what a science experiment is (and is not), the key factors in an experiment, examples, and types of experiments.
Scientific experiments are meant to show cause and effect of a phenomena (relationships in nature). The "variables" are any factor, trait, or condition that can be changed in the experiment and that can have an effect on the outcome of the experiment. An experiment can have three kinds of variables: independent, dependent, and controlled.. The independent variable is one single factor that ...
Chemix is a free online editor for drawing science lab diagrams and school experiment apparatus. Easy sketching for both students and teachers. ... it is designed for students and pupils to help them draw diagrams of common laboratory equipment and lab setup of science experiments.
Key Info. Write the experimental procedure like a step-by-step recipe for your science experiment. A good procedure is so detailed and complete that it lets someone else duplicate your experiment exactly! Repeating a science experiment is an important step to verify that your results are consistent and not just an accident.. For a typical experiment, you should plan to repeat it at least three ...
10 Experimental research. 10. Experimental research. Experimental research—often considered to be the 'gold standard' in research designs—is one of the most rigorous of all research designs. In this design, one or more independent variables are manipulated by the researcher (as treatments), subjects are randomly assigned to different ...
A Simple Experimental Setup to Clearly Show That Light Does Not Recombine after Passing through Two Prisms. ERIC Educational Resources Information Center. Garcia-Molina, Rafael; del Mazo, Alejandro; Velasco, Santiago. 2018-01-01. We present a simple and cheap experimental setup that clearly shows how the colors of the white light spectrum after passing a prism do not recombine when emerging ...
I am writing a Computer Science paper, however my supervisor wants me to describe the methodology in a more general form in the Method section. In the Experimental Setup section I dive into the details. ... On the other hand, the Experimental Setup section is the place where those implementation details belong. - Aleksandr Blekh. Commented ...
Experimental set-up: I hypothesize the literacy level depends on books read; Design of experiment — Measure books read and literacy of 100 individuals; Sample size (n) ... Designing experiments in Data Science should be the same. This is the basics of experimental design, which is fundamentally about precise planning and design to ensure that ...
The measurement set-up of thermionic emission is shown in Fig. S7a, including components of a high voltage source (Teslaman, TCM6002), precision sampling resistor (30 kΩ, 1/4 W), high-speed ...