Experimental Methods and their Applications to Fluid Flow

Volumes and issues
Volume 65 january - december 2024 jan - dec 2024.
- Issue 12 December 2024
- Issue 11 November 2024
- Issue 10 October 2024
- Issue 9 September 2024
- Issue 8 August 2024
- Issue 7 July 2024
- Issue 6 June 2024
- Issue 5 May 2024
- Issue 4 April 2024
- Issue 3 March 2024
- Issue 2 February 2024
- Issue 1 January 2024
Volume 64 January - December 2023 Jan - Dec 2023
- Issue 12 December 2023
- Issue 11 November 2023
- Issue 10 October 2023
- Issue 9 September 2023
- Issue 8 August 2023
- Issue 7 July 2023
- Issue 6 June 2023
- Issue 5 May 2023
- Issue 4 April 2023
- Issue 3 March 2023
- Issue 2 February 2023
- Issue 1 January 2023
Volume 63 January - December 2022 Jan - Dec 2022
- Issue 12 December 2022
- Issue 11 November 2022
- Issue 10 October 2022
- Issue 9 September 2022
- Issue 8 August 2022
- Issue 7 July 2022
- Issue 6 June 2022
- Issue 5 May 2022
- Issue 4 April 2022
- Issue 3 March 2022
- Issue 2 February 2022
- Issue 1 January 2022
Volume 62 January - December 2021 Jan - Dec 2021
- Issue 12 December 2021
- Issue 11 November 2021
- Issue 10 October 2021
- Issue 9 September 2021
- Issue 8 August 2021
- Issue 7 July 2021
- Issue 6 June 2021
- Issue 5 May 2021
- Issue 4 April 2021
- Issue 3 March 2021
- Issue 2 February 2021
- Issue 1 January 2021
Volume 61 January - December 2020 Jan - Dec 2020
- Issue 12 December 2020
- Issue 11 November 2020
- Issue 10 October 2020
- Issue 9 September 2020
- Issue 8 August 2020
- Issue 7 July 2020
- Issue 6 June 2020
- Issue 5 May 2020
- Issue 4 April 2020
- Issue 3 March 2020
- Issue 2 February 2020
- Issue 1 January 2020
Volume 60 January - December 2019 Jan - Dec 2019
- Issue 12 December 2019
- Issue 11 November 2019
- Issue 10 October 2019
- Issue 9 September 2019
- Issue 8 August 2019
- Issue 7 July 2019
- Issue 6 June 2019
- Issue 5 May 2019
- Issue 4 April 2019
- Issue 3 March 2019
- Issue 2 February 2019
- Issue 1 January 2019
Volume 59 January - December 2018 Jan - Dec 2018
- Issue 12 December 2018
- Issue 11 November 2018
- Issue 10 October 2018
- Issue 9 September 2018
- Issue 8 August 2018
- Issue 7 July 2018
- Issue 6 June 2018
- Issue 5 May 2018
- Issue 4 April 2018
- Issue 3 March 2018
- Issue 2 February 2018
- Issue 1 January 2018
Volume 58 January - December 2017 Jan - Dec 2017
- Issue 12 December 2017
- Issue 11 November 2017
- Issue 10 October 2017
- Issue 9 September 2017
- Issue 8 August 2017
- Issue 7 July 2017
- Issue 6 June 2017
- Issue 5 May 2017
- Issue 4 April 2017
- Issue 3 March 2017
- Issue 2 February 2017
- Issue 1 January 2017
Volume 57 January - December 2016 Jan - Dec 2016
- Issue 12 December 2016
- Issue 11 November 2016
- Issue 10 October 2016
- Issue 9 September 2016
- Issue 8 August 2016
- Issue 7 July 2016
- Issue 6 June 2016
- Issue 5 May 2016
- Issue 4 April 2016
- Issue 3 March 2016
- Issue 2 February 2016
- Issue 1 January 2016
Volume 56 January - December 2015 Jan - Dec 2015
- Issue 12 December 2015
- Issue 11 November 2015
- Issue 10 October 2015
- Issue 9 September 2015
- Issue 8 August 2015
- Issue 7 July 2015
- Issue 6 June 2015
- Issue 5 May 2015
- Issue 4 April 2015
- Issue 3 March 2015
- Issue 2 February 2015
- Issue 1 January 2015
Volume 55 January - December 2014 Jan - Dec 2014
- Issue 12 December 2014
- Issue 11 November 2014
- Issue 10 October 2014
- Issue 9 September 2014
- Issue 8 August 2014
- Issue 7 July 2014
- Issue 6 June 2014
- Issue 5 May 2014
- Issue 4 April 2014
- Issue 3 March 2014
- Issue 2 February 2014
- Issue 1 January 2014
Volume 54 January - December 2013 Jan - Dec 2013
- Issue 12 December 2013
- Issue 11 November 2013
- Issue 10 October 2013
- Issue 9 September 2013
- Issue 8 August 2013
- Issue 7 July 2013
- Issue 6 June 2013
- Issue 5 May 2013
- Issue 4 April 2013
- Issue 3 March 2013
- Issue 2 February 2013
- Issue 1 January 2013
Volume 53 July - December 2012 Jul - Dec 2012
- Issue 6 December 2012
- Issue 5 November 2012
- Issue 4 October 2012
- Issue 3 September 2012
- Issue 2 August 2012
- Issue 1 July 2012
Volume 52 January - June 2012 Jan - Jun 2012
- Issue 6 June 2012
- Issue 5 May 2012
- Issue 4 April 2012
- Issue 3 March 2012
- Issue 2 February 2012
- Issue 1 January 2012
Volume 51 July - December 2011 Jul - Dec 2011
- Issue 6 December 2011
- Issue 5 November 2011
- Issue 4 October 2011
- Issue 3 September 2011
- Issue 2 August 2011
- Issue 1 July 2011
Volume 50 January - June 2011 Jan - Jun 2011
- Issue 6 June 2011
- Issue 5 May 2011
Special issue: Eighth International Symposium on Particle Image Velocimetry (PIV’09)
- Issue 3 March 2011
- Issue 2 February 2011
- Issue 1 January 2011
Volume 49 July - December 2010 Jul - Dec 2010
- Issue 6 December 2010
- Issue 5 November 2010
Special Issue: Laser Diagnostics in Combustion; Editors: Andreas Dreizler and Volker Sick
- Issue 3 September 2010
- Issue 2 August 2010
Special Issue: Dedicated to Prof. Dr. Donald Rockwell, Former Editor-in-Chief
Volume 48 January - June 2010 Jan - Jun 2010
- Issue 6 June 2010
- Issue 5 May 2010
13th International Symposium on Flow Visualization; Guest Editor: Prof. Kenneth D Kihm, Prof. Jean Pierre Prenel
- Issue 3 March 2010
- Issue 2 February 2010
- Issue 1 January 2010
Volume 47 July - December 2009 Jul - Dec 2009
- Issue 6 December 2009
Selected papers from the 14th International Symposium on Application of Laser Techniques to Fluid Mechanics, Lisbon, Portugal, July 7-10, 2008
- Issue 3 September 2009
- Issue 2 August 2009
- Issue 1 July 2009
Volume 46 January - June 2009 Jan - Jun 2009
- Issue 6 June 2009
Special Issue: Animal Locomotion- The Physics of Flying
- Issue 4 April 2009
- Issue 3 March 2009
- Issue 2 February 2009
- Issue 1 January 2009
Volume 45 July - December 2008 Jul - Dec 2008
- Issue 6 December 2008
- Issue 5 November 2008
Selected papers from the 7th International Symposium on Particle Image Velocimetry, Roma, Italy, 11-14 September 2007
- Issue 3 September 2008
- Issue 2 August 2008
- Issue 1 July 2008
Volume 44 January - June 2008 Jan - Jun 2008
- Issue 6 June 2008
- Issue 5 May 2008
- Issue 4 April 2008
Selected papers from the 12th International Symposium on Flow Visualization, Göttingen, Germany, September 10th -14th, 2006
- Issue 2 February 2008
- Issue 1 January 2008
Volume 43 July - December 2007 Jul - Dec 2007
- Issue 6 December 2007
Special issue: Animal locomotion: The hydrodynamics of swimming
- Issue 4 October 2007
Selected papers from the 13th International Symposium on Application of Laser Techniques to Fluid Mechanics, Lisbon, Portugal, June 26th-29th, 2006
- Issue 1 July 2007
Volume 42 January - June 2007 Jan - Jun 2007
- Issue 6 June 2007
- Issue 5 May 2007
- Issue 4 April 2007
- Issue 3 March 2007
- Issue 2 February 2007
- Issue 1 January 2007
Volume 41 July - December 2006 Jul - Dec 2006
- Issue 6 December 2006
- Issue 5 November 2006
- Issue 4 October 2006
- Issue 3 September 2006
- Issue 2 August 2006
- Issue 1 July 2006
Volume 40 January - June 2006 Jan - Jun 2006
- Issue 6 June 2006
- Issue 5 May 2006
- Issue 4 April 2006
- Issue 3 March 2006
- Issue 2 February 2006
- Issue 1 January 2006
Volume 39 July - December 2005 Jul - Dec 2005
- Issue 6 December 2005
- Issue 5 November 2005
- Issue 4 October 2005
- Issue 3 September 2005
- Issue 2 August 2005
- Issue 1 July 2005
Volume 38 January - June 2005 Jan - Jun 2005
- Issue 6 June 2005
- Issue 5 May 2005
- Issue 4 April 2005
- Issue 3 March 2005
- Issue 2 February 2005
- Issue 1 January 2005
Volume 37 July - December 2004 Jul - Dec 2004
- Issue 6 December 2004
- Issue 5 November 2004
- Issue 4 October 2004
- Issue 3 September 2004
- Issue 2 August 2004
- Issue 1 July 2004
Volume 36 January - June 2004 Jan - Jun 2004
- Issue 6 June 2004
- Issue 5 May 2004
- Issue 4 April 2004
- Issue 3 March 2004
- Issue 2 February 2004
- Issue 1 January 2004
Volume 35 July - December 2003 Jul - Dec 2003
- Issue 6 December 2003
- Issue 5 November 2003
- Issue 4 October 2003
- Issue 3 September 2003
- Issue 2 August 2003
- Issue 1 July 2003
Volume 34 January - June 2003 Jan - Jun 2003
- Issue 6 June 2003
- Issue 5 May 2003
- Issue 4 April 2003
- Issue 3 March 2003
- Issue 2 February 2003
- Issue 1 January 2003
Volume 33 July - December 2002 Jul - Dec 2002
- Issue 6 December 2002
- Issue 5 November 2002
- Issue 4 October 2002
- Issue 3 September 2002
- Issue 2 August 2002
- Issue 1 July 2002
Volume 32 January - June 2002 Jan - Jun 2002
- Issue 6 June 2002
- Issue 5 May 2002
- Issue 4 April 2002
- Issue 3 March 2002
- Issue 2 February 2002
- Issue 1 January 2002
Volume 31 July - December 2001 Jul - Dec 2001
- Issue 6 December 2001
- Issue 5 November 2001
- Issue 4 October 2001
- Issue 3 September 2001
- Issue 2 August 2001
- Issue 1 July 2001
Volume 30 January - June 2001 Jan - Jun 2001
- Issue 6 June 2001
- Issue 5 May 2001
- Issue 4 April 2001
- Issue 3 March 2001
- Issue 2 February 2001
- Issue 1 January 2001
Volume 29 July - December 2000 Jul - Dec 2000
- Issue 6 December 2000
- Issue 1 supplement December 2000
- Issue 5 November 2000
- Issue 4 October 2000
- Issue 3 September 2000
- Issue 2 August 2000
- Issue 1 July 2000
Volume 28 January - June 2000 Jan - Jun 2000
- Issue 6 June 2000
- Issue 5 May 2000
- Issue 4 April 2000
- Issue 3 March 2000
- Issue 2 February 2000
- Issue 1 January 2000
Volume 27 June - November 1999 Jun - Nov 1999
- Issue 6 November 1999
- Issue 5 October 1999
- Issue 4 September 1999
- Issue 3 August 1999
- Issue 2 July 1999
- Issue 1 June 1999
Volume 26 January - May 1999 Jan - May 1999
- Issue 6 May 1999
- Issue 5 April 1999
- Issue 4 March 1999
- Issue 3 February 1999
- Issue 1-2 January 1999
Volume 25 June - October 1998 Jun - Oct 1998
- Issue 5-6 October 1998
- Issue 4 September 1998
- Issue 3 August 1998
- Issue 2 July 1998
- Issue 1 June 1998
Volume 24 January - May 1998 Jan - May 1998
- Issue 5-6 May 1998
- Issue 4 April 1998
- Issue 3 March 1998
- Issue 2 February 1998
- Issue 1 January 1998
Volume 23 May - December 1997 May - Dec 1997
- Issue 6 December 1997
- Issue 5 November 1997
- Issue 4 August 1997
- Issue 3 July 1997
- Issue 2 June 1997
- Issue 1 May 1997
Volume 22 November 1996 - April 1997 Nov 1996 - Apr 1997
- Issue 6 April 1997
- Issue 5 March 1997
- Issue 4 February 1997
- Issue 3 January 1997
- Issue 2 December 1996
- Issue 1 November 1996
Volume 21 May - November 1996 May - Nov 1996
- Issue 6 November 1996
- Issue 5 September 1996
- Issue 4 August 1996
- Issue 3 July 1996
- Issue 2 June 1996
- Issue 1 May 1996
Volume 20 November 1995 - April 1996 Nov 1995 - Apr 1996
- Issue 6 April 1996
- Issue 5 March 1996
- Issue 4 February 1996
- Issue 3 January 1996
- Issue 2 December 1995
- Issue 1 November 1995
Volume 19 May - October 1995 May - Oct 1995
- Issue 6 October 1995
- Issue 5 September 1995
- Issue 4 August 1995
- Issue 3 July 1995
- Issue 2 June 1995
- Issue 1 May 1995
Volume 18 December 1994 - April 1995 Dec 1994 - Apr 1995
- Issue 6 April 1995
- Issue 5 March 1995
- Issue 4 February 1995
- Issue 3 January 1995
- Issue 1-2 December 1994
Volume 17 June - October 1994 Jun - Oct 1994
- Issue 6 October 1994
- Issue 5 September 1994
- Issue 4 August 1994
- Issue 3 July 1994
- Issue 1-2 June 1994
Volume 16 November 1993 - May 1994 Nov 1993 - May 1994
- Issue 6 May 1994
- Issue 5 April 1994
- Issue 3-4 February 1994
- Issue 2 December 1993
- Issue 1 November 1993
Volume 15 June - October 1993 Jun - Oct 1993
- Issue 6 October 1993
- Issue 4-5 September 1993
- Issue 3 August 1993
- Issue 2 July 1993
- Issue 1 June 1993
Volume 14 January - December 1993 Jan - Dec 1993
- Issue 1-2 December 1993
- Issue 6 May 1993
- Issue 5 April 1993
- Issue 4 February 1993
- Issue 3 January 1993
Volume 13 May - October 1992 May - Oct 1992
- Issue 6 October 1992
- Issue 5 September 1992
- Issue 4 August 1992
- Issue 2-3 June 1992
- Issue 1 May 1992
Volume 12 December 1991 - April 1992 Dec 1991 - Apr 1992
- Issue 6 April 1992
- Issue 4-5 March 1992
- Issue 3 January 1992
- Issue 1-2 December 1991
Volume 11 April - October 1991 Apr - Oct 1991
- Issue 6 October 1991
- Issue 5 September 1991
- Issue 4 July 1991
- Issue 2-3 May 1991
- Issue 1 April 1991
Volume 10 October 1990 - March 1991 Oct 1990 - Mar 1991
- Issue 6 March 1991
- Issue 5 February 1991
- Issue 4 January 1991
- Issue 2-3 December 1990
- Issue 1 October 1990
Volume 9 January - September 1990 Jan - Sept 1990
- Issue 6 September 1990
- Issue 5 July 1990
- Issue 4 June 1990
- Issue 3 May 1990
- Issue 1-2 January 1990
Volume 8 October 1989 - March 1990 Oct 1989 - Mar 1990
- Issue 6 March 1990
- Issue 5 February 1990
- Issue 3-4 December 1989
- Issue 1-2 October 1989

Volume 7 December 1988 - November 1989 Dec 1988 - Nov 1989
- Issue 2 November 1989
- Issue 1 October 1989
- Issue 8 September 1989
- Issue 7 July 1989
- Issue 6 June 1989
- Issue 5 April 1989
- Issue 4 February 1989
- Issue 3 December 1988
Volume 6 January 1988 - January 2004 Jan 1988 - Jan 2004
- Issue 2 January 2004
- Issue 8 January 1988
- Issue 7 January 1988
- Issue 6 January 1988
- Issue 5 January 1988
- Issue 4 January 1988
- Issue 3 January 1988
- Issue 1 January 1988
Volume 5 January 1986 - November 1987 Jan 1986 - Nov 1987
- Issue 6 November 1987
- Issue 5 September 1987
- Issue 4 July 1987
- Issue 3 May 1987
- Issue 2 March 1987
- Issue 1 January 1986
Volume 4 January - November 1986 Jan - Nov 1986
- Issue 6 November 1986
- Issue 5 September 1986
- Issue 4 July 1986
- Issue 3 May 1986
- Issue 2 March 1986
Volume 3 January - November 1985 Jan - Nov 1985
- Issue 6 November 1985
- Issue 5 September 1985
- Issue 4 July 1985
- Issue 3 May 1985
- Issue 2 March 1985
- Issue 1 January 1985
Volume 2 March - December 1984 Mar - Dec 1984
- Issue 4 December 1984
- Issue 3 September 1984
- Issue 2 June 1984
- Issue 1 March 1984
Volume 1 March - December 1983 Mar - Dec 1983
- Issue 4 December 1983
- Issue 3 September 1983
- Issue 2 June 1983
- Issue 1 March 1983
- Find a journal
- Publish with us
- Track your research
- Create seminar
- Register or login
Experiments in Fluids Seminar Series
Experiments in Fluids, Springer Nature (United Kingdom)
Experiments in Fluids Seminar Series is held biweekly on Tuesdays at 4pm (Central European Time) with a format offering either a 30-minute seminar followed by a 15 minutes discussion, or two Article Teaser presentations (each 15 minutes + 5 minutes discussion) presenting key results of recent articles appearing in Experiments in Fluids.
Upcoming live seminars
December 2024.
Shaping up to explore and exploit unsteady fluid-structure interactions
The Physics of Flowing Emulsions in Wall-Bounded Turbulence
January 2025
Active control of very-large-scale motions using wall deformation
On Flow Control
February 2025.
Of networks and regularization: new developments in Lagrangian Particle Tracking
Article Teasers
Vortex dynamics in an oscillating flow
On BOS and its progenitor, the Background-Distortion Schlieren Technique
Particles floating on turbulent water
Experimental studies of cardiovascular assist device and coronary artery model with mock circulatory loop
Control of a Blunt Trailing Edge Profiled Body using Unsteady Distributed Forcing
Improving depth uncertainty in plenoptic camera-based velocimetry
Entrainment into a turbulent wake from a turbulent background and the turbulent/turbulent interface
Springer Professional
Experiments in fluids.
Experimental Methods and their Applications to Fluid Flow
Current Publications
Experiments in Fluids 12/2024
Experiments in Fluids 11/2024
Experiments in Fluids 10/2024
Experiments in Fluids 9/2024
Experiments in Fluids 8/2024
Experiments in Fluids 7/2024
Experiments in Fluids 6/2024
Experiments in Fluids 5/2024
Experiments in Fluids 4/2024
Experiments in Fluids 3/2024
scroll for more
use your arrow keys for more
scroll or use arrow keys for more
About this journal
Experiments in Fluids examines the advancement, extension, and improvement of new techniques of flow measurement. The journal also publishes contributions that employ existing experimental techniques to gain an understanding of the underlying flow physics in the areas of turbulence, aerodynamics, hydrodynamics, convective heat transfer, combustion, turbomachinery, multi-phase flows, and chemical, biological and geological flows. In addition, readers will find papers that report on investigations combining experimental and analytical/numerical approaches.
In addition to Research Articles, Experiments in Fluids publishes Letters and Review Articles.
Premium Partners
- Business IT + Informatics
- Construction + Real Estate
- Electrical Engineering + Electronics
- Energy + Sustainability
- Insurance + Risk
- Finance + Banking
- Management + Leadership
- Marketing + Sales
- Mechanical Engineering + Materials
- Start single access now
- Access for companies
Thank you for visiting nature.com. You are using a browser version with limited support for CSS. To obtain the best experience, we recommend you use a more up to date browser (or turn off compatibility mode in Internet Explorer). In the meantime, to ensure continued support, we are displaying the site without styles and JavaScript.
- View all journals
- Explore content
- About the journal
- Publish with us
- Sign up for alerts
- Perspective
- Published: 10 August 2023
The transformative potential of machine learning for experiments in fluid mechanics
- Ricardo Vinuesa ORCID: orcid.org/0000-0001-6570-5499 1 , 2 ,
- Steven L. Brunton 3 &
- Beverley J. McKeon 4
Nature Reviews Physics volume 5 , pages 536–545 ( 2023 ) Cite this article
2611 Accesses
29 Citations
10 Altmetric
Metrics details
- Engineering
- Fluid dynamics
The field of machine learning (ML) has rapidly advanced the state of the art in many fields of science and engineering, including experimental fluid dynamics, which is one of the original big-data disciplines. This Perspective article highlights several aspects of experimental fluid mechanics that stand to benefit from progress in ML, including augmenting the fidelity and quality of measurement techniques, improving experimental design and surrogate digital-twin models and enabling real-time estimation and control. In each case, we discuss recent success stories and ongoing challenges, along with caveats and limitations, and outline the potential for new avenues of ML-augmented and ML-enabled experimental fluid mechanics.
This is a preview of subscription content, access via your institution
Access options
Access Nature and 54 other Nature Portfolio journals
Get Nature+, our best-value online-access subscription
24,99 € / 30 days
cancel any time
Subscribe to this journal
Receive 12 digital issues and online access to articles
92,52 € per year
only 7,71 € per issue
Buy this article
- Purchase on SpringerLink
- Instant access to full article PDF
Prices may be subject to local taxes which are calculated during checkout
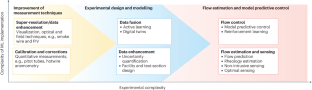
Similar content being viewed by others
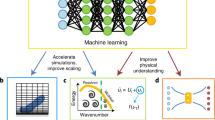
Enhancing computational fluid dynamics with machine learning
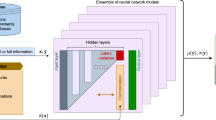
Multi-fidelity information fusion with concatenated neural networks
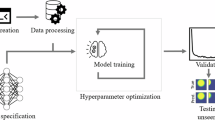
Setting the standard for machine learning in phase field prediction: a benchmark dataset and baseline metrics
Taira, K. et al. Modal analysis of fluid flows: an overview. AIAA J. 55 , 4013–4041 (2017).
Article ADS Google Scholar
Brunton, S. L., Noack, B. R. & Koumoutsakos, P. Machine learning for fluid mechanics. Annu. Rev. Fluid Mech. 52 , 477–508 (2020).
Article ADS MathSciNet MATH Google Scholar
Brenner, M., Eldredge, J. & Freund, J. Perspective on machine learning for advancing fluid mechanics. Phys. Rev. Fluids 4 , 100501 (2019).
Vinuesa, R. & Brunton, S. L. Enhancing computational fluid dynamics with machine learning. Nat. Comput. Sci. 2 , 358–366 (2022).
Article Google Scholar
LeCun, Y., Bengio, Y. & Hinton, G. Deep learning. Nature 521 , 436–444 (2015).
Bailey, S. C. C. et al. Obtaining accurate mean velocity measurements in high Reynolds number turbulent boundary layers using pitot tubes. J. Fluid Mech. 715 , 642–670 (2013).
Article ADS MATH Google Scholar
Tavoularis, S. Measurement in Fluid Mechanics (Cambridge Univ. Press, 2005).
McKeon, B. J., Li, J., Jiang, W., Morrison, J. F. & Smits, A. J. Pitot probe corrections in fully developed turbulent pipe flow. Meas. Sci. Technol. 14 , 1449–1458 (2003).
Vinuesa, R. & Nagib, H. M. Enhancing the accuracy of measurement techniques in high Reynolds number turbulent boundary layers for more representative comparison to their canonical representations. Eur. J. Mech. B/Fluids 55 , 300–312 (2016).
Örlü, R., Fransson, J. H. M. & Alfredsson, P. H. On near wall measurements of wall bounded flows: the necessity of an accurate determination of the wall position. Prog. Aerosp. Sci. 46 , 353–387 (2010).
Vinuesa, R., Schlatter, P. & Nagib, H. M. Role of data uncertainties in identifying the logarithmic region of turbulent boundary layers. Exp. Fluids 55 , 1751 (2014).
Ashok, A., Bailey, S. C. C., Hultmark, M. & Smits, A. J. Hot-wire spatial resolution effects in measurements of grid-generated turbulence. Exp. Fluids 53 , 1713–1722 (2012).
Chin, C., Hutchins, N., Ooi, A. & Marusic, I. Spatial resolution correction for hot-wire anemometry in wall turbulence. Exp. Fluids 50 , 1443–1453 (2011).
Monkewitz, P. A., Duncan, R. D. & Nagib, H. M. Correcting hot-wire measurements of stream-wise turbulence intensity in boundary layers. Phys. Fluids 22 , 091701 (2010).
Koza, J. R. Genetic Programming : On the Programming of Computers by Means of Natural Selection (MIT Press, 1992).
Brunton, S. L., Proctor, J. L. & Kutz, J. N. Discovering governing equations from data by sparse identification of nonlinear dynamical systems. Proc. Natl Acad. Sci. USA 113 , 3932–3937 (2016).
Lu, L., Jin, P., Pang, G., Zhang, Z. & Karniadakis, G. E. Learning nonlinear operators via DeepONet based on the universal approximation theorem of operators. Nat. Mach. Intell. 3 , 218–229 (2021).
Li, Z. et al. Neural operator: graph kernel network for partial differential equations. Preprint at https://arxiv.org/abs/2003.03485 (2020).
Batill, S. M. & Mueller, T. J. Visualization of transition in the flow over an airfoil using the smoke-wire technique. AIAA J. 19 , 340–345 (1981).
Cardona, J. L., Howland, M. F. & Dabiri, J. O. Seeing the wind: visual wind speed prediction with a coupled convolutional and recurrent neural network. In Advances in Neural Information Processing Systems 1130–1140 (Curran Associates, Inc., 2017).
Guastoni, L. et al. Convolutional-network models to predict wall-bounded turbulence from wall quantities. J. Fluid Mech. 928 , A27 (2021).
Article MathSciNet Google Scholar
Güemes, A. et al. From coarse wall measurements to turbulent velocity fields through deep learning. Phys. Fluids 33 , 075121 (2021).
Liu, B., Tang, J., Huang, H. & Lu, X. Y. Deep learning methods for super-resolution reconstruction of turbulent flows. Phys. Fluids 32 , 025105 (2020).
Fukami, K., Maulik, R., Ramachandra, N., Fukagata, K. & Taira, K. Global field reconstruction from sparse sensors with Voronoi tessellation-assisted deep learning. Nat. Mach. Intell. 3 , 945–951 (2021).
Adrian, R. J. Twenty years of particle image velocimetry. Exp. Fluids 39 , 159–169 (2005).
Scarano, F. Tomographic PIV: principles and practice. Meas. Sci. Technol. 24 , 012001 (2013).
Atkinson, C. & Soria, J. An efficient simultaneous reconstruction technique for tomographic particle image velocimetry. Exp. Fluids 47 , 553–568 (2009).
Lumley, J. L. The structure of inhomogeneous turbulence. In Atmospheric Turbulence and Wave Propagation (eds Yaglom, A. M. & Tatarski, V. I.) 166–178 (Nauka, 1967).
Méndez, M. A. et al. POD-based background removal for particle image velocimetry. Exp. Therm. Fluid Sci. 80 , 181–192 (2017).
Scherl, I. et al. Robust principal component analysis for modal decomposition of corrupt fluid flows. Phys. Rev. Fluids 5 , 054401 (2020).
Rabault, J., Kolaas, J. & Jensen, A. Performing particle image velocimetry using artificial neural networks: a proof-of-concept. Meas. Sci. Technol. 28 , 125301 (2017).
Fukami, K., Fukagata, K. & Taira, K. Super-resolution reconstruction of turbulent flows with machine learning. J. Fluid Mech. 870 , 106–120 (2019).
Morimoto, M., Fukami, K. & Fukagata, K. Experimental velocity data estimation for imperfect particle image using machine learning. Phys. Fluids 33 , 087121 (2021).
Lee, Y., Yang, H. & Yin, Z. PIV-DCNN: cascaded deep convolutional neural networks for particle image velocimetry. Exp. Fluids 58 , 171 (2017).
Raissi, M., Perdikaris, P. & Karniadakis, G. E. Physics-informed neural networks: a deep learning framework for solving forward and inverse problems involving nonlinear partial differential equations. J. Comput. Phys. 378 , 686–707 (2019).
Raissi, M., Yazdani, A. & Karniadakis, G. E. Hidden fluid mechanics: learning velocity and pressure fields from flow visualizations. Science 367 , 1026–1030 (2020).
Arzani, A., Cassel, K. W. & D’Souza, R. M. Theory-guided physics-informed neural networks for boundary layer problems with singular perturbation. J. Comput. Phys. 473 , 111768 (2023).
Article MathSciNet MATH Google Scholar
Eivazi, H. & Vinuesa, R. Physics-informed deep-learning applications to experimental fluid mechanics. Preprint at https://arxiv.org/abs/2203.15402 (2022).
Zhou, K., Li, J., Hong, J. & Grauer, S. J. Stochastic particle advection velocimetry (SPAV): theory, simulations, and proof-of-concept experiments. Meas. Sci. Technol. 34 , 065302 (2023).
Christiansen, J. P. Numerical simulation of hydrodynamics by the method of point vortices. J. Comput. Phys. 13 , 363–379 (1973).
Schneiders, J., Dwight, R. & Scarano, F. Time-supersampling of 3D-PIV measurements with vortex-in-cell simulation. Exp. Fluids 55 , 1692 (2014).
Doan, N. A. K., Polifke, W. & Magri, L. Short- and long-term predictions of chaotic flows and extreme events: a physics-constrained reservoir computing approach. Proc. R. Soc. A 477 , 20210135 (2021).
Article ADS MathSciNet Google Scholar
Discetti, S. & Liu, Y. Machine learning for flow field measurements: a perspective. Meas. Sci. Technol. 34 , 021001 (2023).
Rezaeiravesh, S., Vinuesa, R., Liefvendahl, M. & Schlatter, P. Assessment of uncertainties in hot-wire anemometry and oil-film interferometry measurements for wall-bounded turbulent flows. Eur. J. Mech. B/Fluids 72 , 57–73 (2018).
Kapteyn, M. G., Pretorius, J. V. R. & Willcox, K. E. A probabilistic graphical model foundation for enabling predictive digital twins at scale. Nat. Comput. Sci. 1 , 337–347 (2021).
Niederer, S. A., Sacks, M. S., Girolami, M. & Willcox, K. Scaling digital twins from the artisanal to the industrial. Nat. Comput. Sci. 1 , 313–320 (2021).
Brunton, S. L. et al. Data-driven aerospace engineering: reframing the industry with machine learning. AIAA J. 59 , 2820–2847 (2021).
Google Scholar
Herriot, J. G. Blockage Corrections for Three-Dimensional-Flow Closed-Throat Wind Tunnels, with Consideration of the Effect of Compressibility. Technical Report NACA-RM-A7B28 (National Advisory Committee for Aeronautics, 1947).
Pirozzoli, S., Modesti, D., Orlandi, P. & Grasso, F. Turbulence and secondary motions in square duct flow. J. Fluid Mech. 840 , 631–655 (2018).
Tabatabaei, N. et al. RANS modelling of a NACA4412 wake using wind tunnel measurements. Fluids 7 , 153 (2022).
Morita, Y. et al. Applying Bayesian optimization with Gaussian-process regression to computational fluid dynamics problems. J. Comput. Phys. 449 , 110788 (2022).
Nocedal, J. & Wright, S. Numerical Optimization (Springer, 2006).
Rowley, C. W., Mezic, I., Bagheri, S., Schlatter, P. & Henningson, D. Spectral analysis of nonlinear flows. J. Fluid Mech. 645 , 115–127 (2009).
Schmid, P. J. Dynamic mode decomposition of numerical and experimental data. J. Fluid Mech. 656 , 5–28 (2010).
Kutz, J. N., Brunton, S. L., Brunton, B. W. & Proctor, J. L. Dynamic Mode Decomposition: Data-Driven Modeling of Complex Systems (SIAM, 2016).
Rowley, C. W. & Dawson, S. T. Model reduction for flow analysis and control. Annu. Rev. Fluid Mech. 49 , 387–417 (2017).
Le Clainche, S. & Vega, J. M. Higher order dynamic mode decomposition. SIAM J. Appl. Dyn. Syst. 16 , 882–925 (2017).
Rudy, S. H., Brunton, S. L., Proctor, J. L. & Kutz, J. N. Data-driven discovery of partial differential equations. Sci. Adv. 3 , e1602614 (2017).
Bongard, J. & Lipson, H. Automated reverse engineering of nonlinear dynamical systems. Proc. Natl Acad. Sci. USA 104 , 9943–9948 (2007).
Schmidt, M. & Lipson, H. Distilling free-form natural laws from experimental data. Science 324 , 81–85 (2009).
Cranmer, M., Xu, R., Battaglia, P. & Ho, S. Learning symbolic physics with graph networks. Preprint at https://arxiv.org/abs/1909.05862 (2019).
Cranmer, M. et al. Discovering symbolic models from deep learning with inductive biases. Advances in Neural Information Processing Systems ( NeurIPS 2020) (Curran Associates, Inc., 2020).
Vlachas, P. R., Byeon, W., Wan, Z. Y., Sapsis, T. P. & Koumoutsakos, P. Data-driven forecasting of high-dimensional chaotic systems with long short-term memory networks. Proc. R. Soc. A 474 , 20170844 (2018).
Pathak, J., Hunt, B., Girvan, M., Lu, Z. & Ott, E. Model-free prediction of large spatiotemporally chaotic systems from data: a reservoir computing approach. Phys. Rev. Lett. 120 , 024102 (2018).
Cranmer, M. et al. Lagrangian neural networks. Preprint at https://arxiv.org/abs/2003.04630 (2020).
Chen, R. T., Rubanova, Y., Bettencourt, J. & Duvenaud, D. Neural ordinary differential equations. Preprint at https://arxiv.org/abs/1806.07366 (2018).
Reinbold, P. A., Kageorge, L. M., Schatz, M. F. & Grigoriev, R. O. Robust learning from noisy, incomplete, high-dimensional experimental data via physically constrained symbolic regression. Nat. Commun. 12 , 3219 (2021).
Callaham, J. L., Rigas, G., Loiseau, J.-C. & Brunton, S. L. An empirical mean-field model of symmetry-breaking in a turbulent wake. Sci. Adv. 8 , eabm4786 (2022).
Supekar, R. et al. Learning hydrodynamic equations for active matter from particle simulations and experiments. Proc. Natl Acad. Sci. USA 120 , e2206994120 (2023).
Zanna, L. & Bolton, T. Data-driven equation discovery of ocean mesoscale closures. Geophys. Res. Lett. 47 , e2020GL088376 (2020).
Schmelzer, M., Dwight, R. P. & Cinnella, P. Discovery of algebraic Reynolds-stress models using sparse symbolic regression. Flow Turbul. Combust. 104 , 579–603 (2020).
Beetham, S. & Capecelatro, J. Formulating turbulence closures using sparse regression with embedded form invariance. Phys. Rev. Fluids 5 , 084611 (2020).
Beetham, S., Fox, R. O. & Capecelatro, J. Sparse identification of multiphase turbulence closures for coupled fluid–particle flows. J. Fluid Mech. 914 , A11 (2021).
Wang, M. & Zaki, T. A. Synchronization of turbulence in channel flow. J. Fluid Mech. 943 , A4 (2022).
Herrmann, B., Oswald, P., Semaan, R. & Brunton, S. L. Modeling synchronization in forced turbulent oscillator flows. Commun. Phys. 3 , 195 (2020).
Nóvoa, A. & Magri, L. Real-time thermoacoustic data assimilation. J. Fluid Mech. 948 , A35 (2022).
Jahanbakhshi, R. & Zaki, T. A. Nonlinearly most dangerous disturbance for high-speed boundary-layer transition. J. Fluid Mech. 876 , 87–121 (2019).
da Silva, A. F. C. & Colonius, T. Flow state estimation in the presence of discretization errors. J. Fluid Mech. 890 , A10 (2020).
Sobol, I. Global sensitivity indices for nonlinear mathematical models and their Monte Carlo estimates. Math. Comput. Simul. 55 , 271–280 (2001).
Xiu, D. & Karniadakis, G. E. The Wiener–Askey polynomial chaos for stochastic differential equations. SIAM J. Sci. Comput. 24 , 619–644 (2002).
Tanner, L. H. & Blows, L. G. A study of the motion of oil films on surfaces in air flow, with application to the measurement of skin friction. J. Phys. E Sci. Instrum. 9 , 194–202 (1976).
Nagib, H. M., Christophorou, C., Rüedi, J.-D., Monkewitz, P. A. & Österlund, J. M. Can we ever rely on results from wall-bounded turbulent flows without direct measurements of wall shear stress? 24th AIAA Aerodynamic Measurement Technology and Ground Testing Conference (AIAA, 2004).
Rezaeiravesh, S., Vinuesa, R. & Schlatter, P. On numerical uncertainties in scale-resolving simulations of canonical wall turbulence. Comput. Fluids 227 , 105024 (2021).
Fan, D. et al. A robotic intelligent towing tank for learning complex fluid-structure dynamics. Sci. Robot. 4 , eaay5063 (2019).
Manohar, K., Brunton, B. W., Kutz, J. N. & Brunton, S. L. Data-driven sparse sensor placement for reconstruction: demonstrating the benefits of exploiting known patterns. IEEE Contr. Syst. Mag. 38 , 63–86 (2018).
Brunton, S. L. & Noack, B. R. Closed-loop turbulence control: progress and challenges. Appl. Mech. Rev. 67 , 050801-1–050801-48 (2015).
Morton, J., Witherden, F. D., Jameson, A. & Kochenderfer, M. J. Deep dynamical modeling and control of unsteady fluid flows. In 32nd Conference on Neural Information Processing Systems ( NeurIPS 2018 ) (Curran Associates, Inc., 2018).
Bieker, K., Peitz, S., Brunton, S. L., Kutz, J. N. & Dellnitz, M. Deep model predictive flow control with limited sensor data and online learning. Theoret. Computat. Fluid Dyn. 34 , 577–591 (2020).
Suzuki, T. & Hasegawa, Y. Estimation of turbulent channel flow at Re θ = 100 based on the wall measurement using a simple sequential approach. J. Fluid Mech. 830 , 760–796 (2017).
Encinar, M. & Jiménez, J. Logarithmic-layer turbulence: a view from the wall. Phys. Rev. Fluids 4 , 114603 (2019).
Borée, J. Extended proper orthogonal decomposition: a tool to analyse correlated events in turbulent flows. Exp. Fluids 35 , 188–192 (2003).
Agostini, L. & Leschziner, M. Predicting the response of small-scale near-wall turbulence to large-scale outer motions. Phys. Fluids 28 , 015107 (2016).
Mathis, R., Hutchins, N. & Marusic, I. Large-scale amplitude modulation of the small-scale structures in turbulent boundary layers. J. Fluid Mech. 628 , 311–337 (2009).
Güemes, A., Discetti, S. & Ianiro, A. Sensing the turbulent large-scale motions with their wall signature. Phys. Fluids 31 , 125112 (2019).
Kim, J. & Lee, C. Prediction of turbulent heat transfer using convolutional neural networks. J. Fluid Mech. 882 , A18 (2020).
Goodfellow, I. et al. Generative adversarial networks. Commun. ACM 63 , 139–144 (2020).
Abbassi, M. R., Baars, W. J., Hutchins, N. & Marusic, I. Skin-friction drag reduction in a high-Reynolds-number turbulent boundary layer via real-time control of large-scale structures. Int. J. Heat Fluid Flow 67 , 30–41 (2017).
Geetha Balasubramanian, A., Vinuesa, R. & Tammisola, O. Prediction of wall-bounded turbulence in a viscoelastic channel flow using convolutional neural networks. In Proc. European Drag Reduction and Flow Control Meeting ( EDRFCM ) (EDRFCM, 2022).
Mahmoudabadbozchelou, M., Kamani, K. M., Rogers, S. A. & Jamali, S. Digital rheometer twins: learning the hidden rheology of complex fluids through rheology-informed graph neural networks. Proc. Natl Acad. Sci. USA 119 , e2202234119 (2022).
Brunton, S. L. & Kutz, J. N. Data-Driven Science and Engineering: Machine Learning, Dynamical Systems, and Control (Cambridge Univ. Press, 2022).
Vinuesa, R. & Sirmacek, B. Interpretable deep-learning models to help achieve the sustainable development goals. Nat. Mach. Intell. 3 , 926 (2021).
Lundberg, S. M. & Lee, S.-I. A unified approach to interpreting model predictions. In 31st Conference on Neural Information Processing Systems ( NIPS 2017) (Curran Associates, Inc., 2017).
Lee, S., Yang, J., Forooghi, P., Stroh, A. & Bagheri, S. Predicting drag on rough surfaces by transfer learning of empirical correlations. J. Fluid Mech. 933 , A18 (2022).
Mahfoze, O. A., Moody, A., Wynn, A., Whalley, R. D. & Laizet, S. Reducing the skin-friction drag of a turbulent boundary-layer flow with low-amplitude wall-normal blowing within a Bayesian optimization framework. Phys. Rev. Fluids 4 , 094601 (2019).
Kornilov, V. I. & Boiko, A. V. Efficiency of air microblowing through microperforated wall for flat plate drag reduction. AIAA J. 50 , 724–732 (2012).
Li, R., Noack, B. R., Cordier, L., Borée, J. & Harambat, F. Drag reduction of a car model by linear genetic programming control. Exp. Fluids 58 , 103 (2017).
Minelli, G., Dong, T., Noack, B. & Krajnović, S. Upstream actuation for bluff-body wake control driven by a genetically inspired optimization. J. Fluid Mech. 893 , A1 (2020).
Choi, H., Moin, P. & Kim, J. Active turbulence control for drag reduction in wall-bounded flows. J. Fluid Mech. 262 , 75–110 (1994).
Marusic, I. et al. An energy-efficient pathway to turbulent drag reduction. Nat. Commun. 12 , 5805 (2021).
Sutton, R. S. & Barto, A. G. Reinforcement Learning: An Introduction Vol. 1 (MIT Press, 1998).
Recht, B. A tour of reinforcement learning: the view from continuous control. Annu. Rev. Control Robot. Auton. Syst. 2 , 253–279 (2019).
Mnih, V. et al. Human-level control through deep reinforcement learning. Nature 518 , 529 (2015).
Silver, D. et al. A general reinforcement learning algorithm that masters chess, shogi, and Go through self-play. Science 362 , 1140–1144 (2018).
Reddy, S., Dragan, A. D. & Levine, S. Shared autonomy via deep reinforcement learning. Preprint at https://arxiv.org/abs/1802.01744 (2018).
Vinyals, O. et al. Grandmaster level in StarCraft II using multi-agent reinforcement learning. Nature 575 , 350–354 (2019).
Verma, S., Novati, G. & Koumoutsakos, P. Efficient collective swimming by harnessing vortices through deep reinforcement learning. Proc. Natl Acad. Sci. USA 115 , 5849–5854 (2018).
Novati, G., Mahadevan, L. & Koumoutsakos, P. Controlled gliding and perching through deep-reinforcement-learning. Phys. Rev. Fluids 4 , 093902 (2019).
Rabault, J., Kuchta, M., Jensen, A., Réglade, U. & Cerardi, N. Artificial neural networks trained through deep reinforcement learning discover control strategies for active flow control. J. Fluid Mech. 865 , 281–302 (2019).
Wang, Q. et al. DRLinFluids: an open-source Python platform of coupling deep reinforcement learning and OpenFOAM. Phys. Fluids 34 , 081801 (2022).
Fan, D., Yang, L., Wang, Z., Triantafyllou, M. S. & Karniadakis, G. E. Reinforcement learning for bluff body active flow control in experiments and simulations. Proc. Natl Acad. Sci. USA 117 , 26091–26098 (2020).
Novati, G., de Laroussilhe, H. L. & Koumoutsakos, P. Automating turbulence modelling by multi-agent reinforcement learning. Nat. Mach. Intell. 3 , 87–96 (2021).
Gunnarson, P., Mandralis, I., Novati, G., Koumoutsakos, P. & Dabiri, J. O. Learning efficient navigation in vortical flow fields. Nat. Commun. 12 , 7143 (2021).
Vinuesa, R., Lehmkuhl, O., Lozano-Durán, A. & Rabault, J. Flow control in wings and discovery of novel approaches via deep reinforcement learning. Fluids 7 , 62 (2022).
Bae, H. J. & Koumoutsakos, P. Scientific agent reinforcement learning for wall-models of turbulent flows. Nat. Commun. 13 , 1443 (2022).
Guastoni, L., Rabault, J., Schlatter, P., Azizpour, H. & Vinuesa, R. Deep reinforcement learning for turbulent drag reduction in channel flows. Eur. Phys. J. E 46 , 27 (2023).
Sonoda, T., Liu, Z., Itoh, T. & Hasegawa, Y. Reinforcement learning of control strategies for reducing skin friction drag in a fully developed channel flow. J. Fluid Mech. 960 , A30 (2023).
Vignon, C., Rabault, J. & Vinuesa, R. Recent advances in applying deep reinforcement learning for flow control: perspectives and future directions. Phys. Fluids 35 , 031301 (2023).
Eastwood, C. & Williams, C. K. I. A framework for the quantitative evaluation of disentangled representations. In International Conference on Learning Representations (2018).
Download references
Acknowledgements
The authors gratefully acknowledge valuable discussions with B. Noack early in the development of this Perspective article. R.V. acknowledges financial support from ERC grant no. 2021-CoG-101043998, DEEPCONTROL. Views and opinions expressed are however those of the author(s) only and do not necessarily reflect those of the European Union or the European Research Council. Neither the European Union nor the granting authority can be held responsible for them. S.L.B. acknowledges support from the National Science Foundation AI Institute in Dynamic Systems (grant no. 2112085). B.J.M. is grateful for the support of the U.S. ONR through a Vannevar Bush Faculty Fellowship, N00014-17-1-3022.
Author information
Authors and affiliations.
FLOW, Engineering Mechanics, KTH Royal Institute of Technology, Stockholm, Sweden
- Ricardo Vinuesa
Swedish e-Science Research Centre (SeRC), Stockholm, Sweden
Department of Mechanical Engineering, University of Washington, Seattle, WA, USA
Steven L. Brunton
Department of Mechanical Engineering, Stanford University, Stanford, CA, USA
Beverley J. McKeon
You can also search for this author in PubMed Google Scholar
Contributions
All authors contributed to all aspects of the manuscript.
Corresponding author
Correspondence to Ricardo Vinuesa .
Ethics declarations
Competing interests.
The authors declare no competing interests.
Peer review
Peer review information.
Nature Reviews Physics thanks Hui Tang and the other, anonymous, reviewer(s) for their contribution to the peer review of this work.
Additional information
Publisher’s note Springer Nature remains neutral with regard to jurisdictional claims in published maps and institutional affiliations.
Rights and permissions
Springer Nature or its licensor (e.g. a society or other partner) holds exclusive rights to this article under a publishing agreement with the author(s) or other rightsholder(s); author self-archiving of the accepted manuscript version of this article is solely governed by the terms of such publishing agreement and applicable law.
Reprints and permissions
About this article
Cite this article.
Vinuesa, R., Brunton, S.L. & McKeon, B.J. The transformative potential of machine learning for experiments in fluid mechanics. Nat Rev Phys 5 , 536–545 (2023). https://doi.org/10.1038/s42254-023-00622-y
Download citation
Accepted : 11 July 2023
Published : 10 August 2023
Issue Date : September 2023
DOI : https://doi.org/10.1038/s42254-023-00622-y
Share this article
Anyone you share the following link with will be able to read this content:
Sorry, a shareable link is not currently available for this article.
Provided by the Springer Nature SharedIt content-sharing initiative
This article is cited by
Identifying regions of importance in wall-bounded turbulence through explainable deep learning.
- Andrés Cremades
- Sergio Hoyas
Nature Communications (2024)
State-of-the-art review on the use of AI-enhanced computational mechanics in geotechnical engineering
- Hongchen Liu
- Daniel Dias-da-Costa
Artificial Intelligence Review (2024)
Computational Sensing, Understanding, and Reasoning: An Artificial Intelligence Approach to Physics-Informed World Modeling
- Beatriz Moya
- Alberto Badías
- Elias Cueto
Archives of Computational Methods in Engineering (2024)
Predicting the soliton dynamics and system parameters in optical fiber couplers
- Aocheng Yang
- Yunzhou Sun
Nonlinear Dynamics (2024)
Quick links
- Explore articles by subject
- Guide to authors
- Editorial policies
Sign up for the Nature Briefing: AI and Robotics newsletter — what matters in AI and robotics research, free to your inbox weekly.


IMAGES
VIDEO