

Qualitative Data Analysis Methods 101:
By: Kerryn Warren (PhD) | Reviewed By: Eunice Rautenbach (D.Tech) | May 2020 (Updated April 2023)
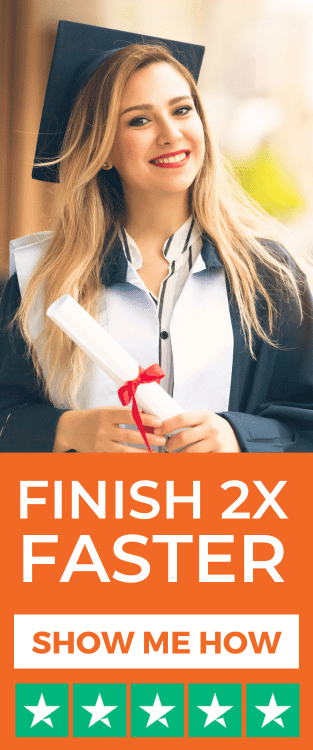
If you’re new to the world of research, qualitative data analysis can look rather intimidating. So much bulky terminology and so many abstract, fluffy concepts. It certainly can be a minefield!
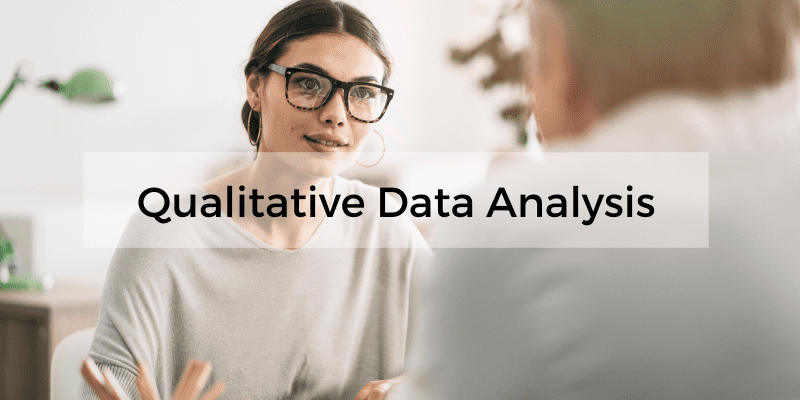
What (exactly) is qualitative data analysis?
To understand qualitative data analysis, we need to first understand qualitative data – so let’s step back and ask the question, “what exactly is qualitative data?”.
Qualitative data refers to pretty much any data that’s “not numbers” . In other words, it’s not the stuff you measure using a fixed scale or complex equipment, nor do you analyse it using complex statistics or mathematics.
So, if it’s not numbers, what is it?
Words, you guessed? Well… sometimes , yes. Qualitative data can, and often does, take the form of interview transcripts, documents and open-ended survey responses – but it can also involve the interpretation of images and videos. In other words, qualitative isn’t just limited to text-based data.
So, how’s that different from quantitative data, you ask?
Simply put, qualitative research focuses on words, descriptions, concepts or ideas – while quantitative research focuses on numbers and statistics . Qualitative research investigates the “softer side” of things to explore and describe , while quantitative research focuses on the “hard numbers”, to measure differences between variables and the relationships between them. If you’re keen to learn more about the differences between qual and quant, we’ve got a detailed post over here .
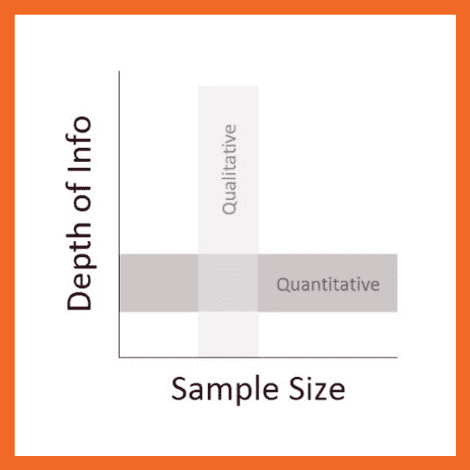
So, qualitative analysis is easier than quantitative, right?
Not quite. In many ways, qualitative data can be challenging and time-consuming to analyse and interpret. At the end of your data collection phase (which itself takes a lot of time), you’ll likely have many pages of text-based data or hours upon hours of audio to work through. You might also have subtle nuances of interactions or discussions that have danced around in your mind, or that you scribbled down in messy field notes. All of this needs to work its way into your analysis.
Making sense of all of this is no small task and you shouldn’t underestimate it. Long story short – qualitative analysis can be a lot of work! Of course, quantitative analysis is no piece of cake either, but it’s important to recognise that qualitative analysis still requires a significant investment in terms of time and effort.
Need a helping hand?
The “Big 6” Qualitative Analysis Methods
There are many different types of qualitative data analysis, all of which serve different purposes and have unique strengths and weaknesses . We’ll start by outlining the analysis methods and then we’ll dive into the details for each.
The 6 most popular methods (or at least the ones we see at Grad Coach) are:
- Content analysis
- Narrative analysis
- Discourse analysis
- Thematic analysis
- Grounded theory (GT)
- Interpretive phenomenological analysis (IPA)
QDA Method #1: Qualitative Content Analysis
Content analysis is possibly the most common and straightforward QDA method. At the simplest level, content analysis is used to evaluate patterns within a piece of content (for example, words, phrases or images) or across multiple pieces of content or sources of communication. For example, a collection of newspaper articles or political speeches.
With content analysis, you could, for instance, identify the frequency with which an idea is shared or spoken about – like the number of times a Kardashian is mentioned on Twitter. Or you could identify patterns of deeper underlying interpretations – for instance, by identifying phrases or words in tourist pamphlets that highlight India as an ancient country.
Because content analysis can be used in such a wide variety of ways, it’s important to go into your analysis with a very specific question and goal, or you’ll get lost in the fog. With content analysis, you’ll group large amounts of text into codes , summarise these into categories, and possibly even tabulate the data to calculate the frequency of certain concepts or variables. Because of this, content analysis provides a small splash of quantitative thinking within a qualitative method.
Naturally, while content analysis is widely useful, it’s not without its drawbacks . One of the main issues with content analysis is that it can be very time-consuming , as it requires lots of reading and re-reading of the texts. Also, because of its multidimensional focus on both qualitative and quantitative aspects, it is sometimes accused of losing important nuances in communication.
Content analysis also tends to concentrate on a very specific timeline and doesn’t take into account what happened before or after that timeline. This isn’t necessarily a bad thing though – just something to be aware of. So, keep these factors in mind if you’re considering content analysis. Every analysis method has its limitations , so don’t be put off by these – just be aware of them ! If you’re interested in learning more about content analysis, the video below provides a good starting point.
QDA Method #2: Narrative Analysis
As the name suggests, narrative analysis is all about listening to people telling stories and analysing what that means . Since stories serve a functional purpose of helping us make sense of the world, we can gain insights into the ways that people deal with and make sense of reality by analysing their stories and the ways they’re told.
You could, for example, use narrative analysis to explore whether how something is being said is important. For instance, the narrative of a prisoner trying to justify their crime could provide insight into their view of the world and the justice system. Similarly, analysing the ways entrepreneurs talk about the struggles in their careers or cancer patients telling stories of hope could provide powerful insights into their mindsets and perspectives . Simply put, narrative analysis is about paying attention to the stories that people tell – and more importantly, the way they tell them.
Of course, the narrative approach has its weaknesses , too. Sample sizes are generally quite small due to the time-consuming process of capturing narratives. Because of this, along with the multitude of social and lifestyle factors which can influence a subject, narrative analysis can be quite difficult to reproduce in subsequent research. This means that it’s difficult to test the findings of some of this research.
Similarly, researcher bias can have a strong influence on the results here, so you need to be particularly careful about the potential biases you can bring into your analysis when using this method. Nevertheless, narrative analysis is still a very useful qualitative analysis method – just keep these limitations in mind and be careful not to draw broad conclusions . If you’re keen to learn more about narrative analysis, the video below provides a great introduction to this qualitative analysis method.
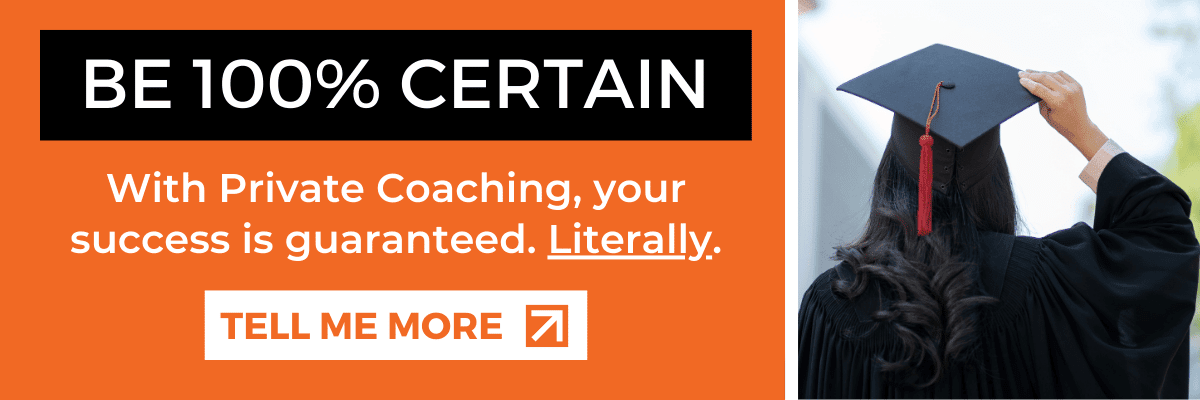
QDA Method #3: Discourse Analysis
Discourse is simply a fancy word for written or spoken language or debate . So, discourse analysis is all about analysing language within its social context. In other words, analysing language – such as a conversation, a speech, etc – within the culture and society it takes place. For example, you could analyse how a janitor speaks to a CEO, or how politicians speak about terrorism.
To truly understand these conversations or speeches, the culture and history of those involved in the communication are important factors to consider. For example, a janitor might speak more casually with a CEO in a company that emphasises equality among workers. Similarly, a politician might speak more about terrorism if there was a recent terrorist incident in the country.
So, as you can see, by using discourse analysis, you can identify how culture , history or power dynamics (to name a few) have an effect on the way concepts are spoken about. So, if your research aims and objectives involve understanding culture or power dynamics, discourse analysis can be a powerful method.
Because there are many social influences in terms of how we speak to each other, the potential use of discourse analysis is vast . Of course, this also means it’s important to have a very specific research question (or questions) in mind when analysing your data and looking for patterns and themes, or you might land up going down a winding rabbit hole.
Discourse analysis can also be very time-consuming as you need to sample the data to the point of saturation – in other words, until no new information and insights emerge. But this is, of course, part of what makes discourse analysis such a powerful technique. So, keep these factors in mind when considering this QDA method. Again, if you’re keen to learn more, the video below presents a good starting point.
QDA Method #4: Thematic Analysis
Thematic analysis looks at patterns of meaning in a data set – for example, a set of interviews or focus group transcripts. But what exactly does that… mean? Well, a thematic analysis takes bodies of data (which are often quite large) and groups them according to similarities – in other words, themes . These themes help us make sense of the content and derive meaning from it.
Let’s take a look at an example.
With thematic analysis, you could analyse 100 online reviews of a popular sushi restaurant to find out what patrons think about the place. By reviewing the data, you would then identify the themes that crop up repeatedly within the data – for example, “fresh ingredients” or “friendly wait staff”.
So, as you can see, thematic analysis can be pretty useful for finding out about people’s experiences , views, and opinions . Therefore, if your research aims and objectives involve understanding people’s experience or view of something, thematic analysis can be a great choice.
Since thematic analysis is a bit of an exploratory process, it’s not unusual for your research questions to develop , or even change as you progress through the analysis. While this is somewhat natural in exploratory research, it can also be seen as a disadvantage as it means that data needs to be re-reviewed each time a research question is adjusted. In other words, thematic analysis can be quite time-consuming – but for a good reason. So, keep this in mind if you choose to use thematic analysis for your project and budget extra time for unexpected adjustments.
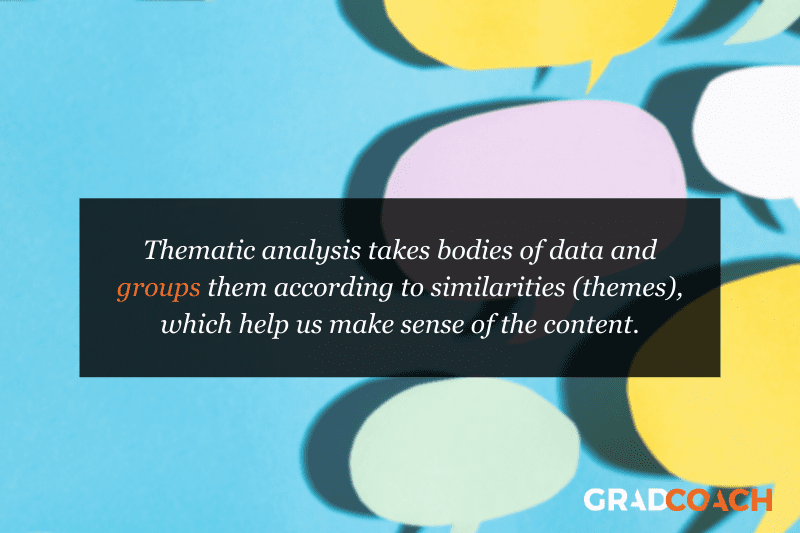
QDA Method #5: Grounded theory (GT)
Grounded theory is a powerful qualitative analysis method where the intention is to create a new theory (or theories) using the data at hand, through a series of “ tests ” and “ revisions ”. Strictly speaking, GT is more a research design type than an analysis method, but we’ve included it here as it’s often referred to as a method.
What’s most important with grounded theory is that you go into the analysis with an open mind and let the data speak for itself – rather than dragging existing hypotheses or theories into your analysis. In other words, your analysis must develop from the ground up (hence the name).
Let’s look at an example of GT in action.
Assume you’re interested in developing a theory about what factors influence students to watch a YouTube video about qualitative analysis. Using Grounded theory , you’d start with this general overarching question about the given population (i.e., graduate students). First, you’d approach a small sample – for example, five graduate students in a department at a university. Ideally, this sample would be reasonably representative of the broader population. You’d interview these students to identify what factors lead them to watch the video.
After analysing the interview data, a general pattern could emerge. For example, you might notice that graduate students are more likely to read a post about qualitative methods if they are just starting on their dissertation journey, or if they have an upcoming test about research methods.
From here, you’ll look for another small sample – for example, five more graduate students in a different department – and see whether this pattern holds true for them. If not, you’ll look for commonalities and adapt your theory accordingly. As this process continues, the theory would develop . As we mentioned earlier, what’s important with grounded theory is that the theory develops from the data – not from some preconceived idea.
So, what are the drawbacks of grounded theory? Well, some argue that there’s a tricky circularity to grounded theory. For it to work, in principle, you should know as little as possible regarding the research question and population, so that you reduce the bias in your interpretation. However, in many circumstances, it’s also thought to be unwise to approach a research question without knowledge of the current literature . In other words, it’s a bit of a “chicken or the egg” situation.
Regardless, grounded theory remains a popular (and powerful) option. Naturally, it’s a very useful method when you’re researching a topic that is completely new or has very little existing research about it, as it allows you to start from scratch and work your way from the ground up .
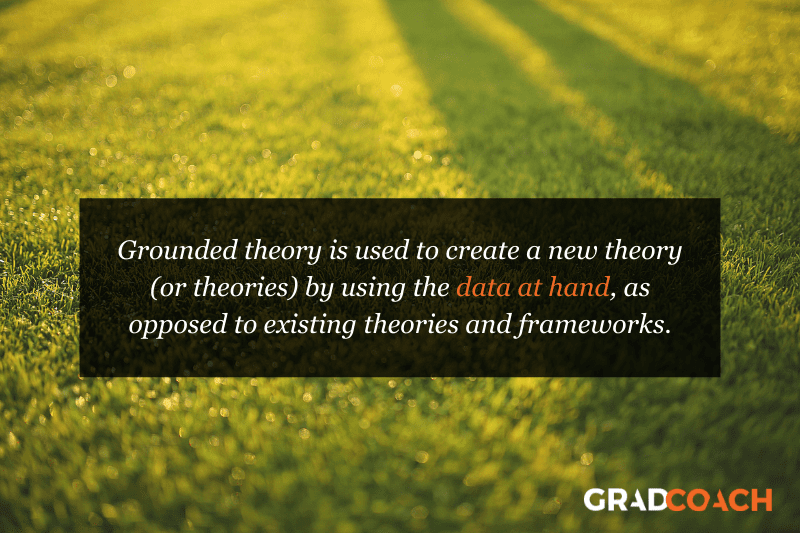
QDA Method #6: Interpretive Phenomenological Analysis (IPA)
Interpretive. Phenomenological. Analysis. IPA . Try saying that three times fast…
Let’s just stick with IPA, okay?
IPA is designed to help you understand the personal experiences of a subject (for example, a person or group of people) concerning a major life event, an experience or a situation . This event or experience is the “phenomenon” that makes up the “P” in IPA. Such phenomena may range from relatively common events – such as motherhood, or being involved in a car accident – to those which are extremely rare – for example, someone’s personal experience in a refugee camp. So, IPA is a great choice if your research involves analysing people’s personal experiences of something that happened to them.
It’s important to remember that IPA is subject – centred . In other words, it’s focused on the experiencer . This means that, while you’ll likely use a coding system to identify commonalities, it’s important not to lose the depth of experience or meaning by trying to reduce everything to codes. Also, keep in mind that since your sample size will generally be very small with IPA, you often won’t be able to draw broad conclusions about the generalisability of your findings. But that’s okay as long as it aligns with your research aims and objectives.
Another thing to be aware of with IPA is personal bias . While researcher bias can creep into all forms of research, self-awareness is critically important with IPA, as it can have a major impact on the results. For example, a researcher who was a victim of a crime himself could insert his own feelings of frustration and anger into the way he interprets the experience of someone who was kidnapped. So, if you’re going to undertake IPA, you need to be very self-aware or you could muddy the analysis.
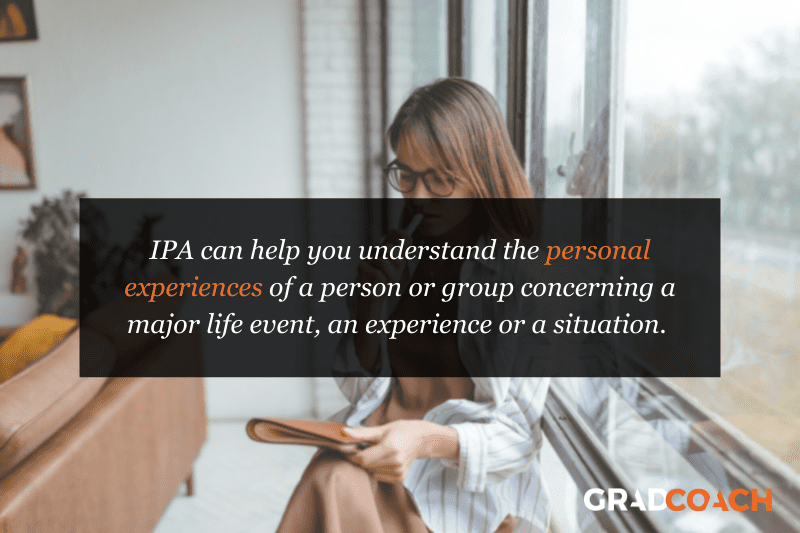
How to choose the right analysis method
In light of all of the qualitative analysis methods we’ve covered so far, you’re probably asking yourself the question, “ How do I choose the right one? ”
Much like all the other methodological decisions you’ll need to make, selecting the right qualitative analysis method largely depends on your research aims, objectives and questions . In other words, the best tool for the job depends on what you’re trying to build. For example:
- Perhaps your research aims to analyse the use of words and what they reveal about the intention of the storyteller and the cultural context of the time.
- Perhaps your research aims to develop an understanding of the unique personal experiences of people that have experienced a certain event, or
- Perhaps your research aims to develop insight regarding the influence of a certain culture on its members.
As you can probably see, each of these research aims are distinctly different , and therefore different analysis methods would be suitable for each one. For example, narrative analysis would likely be a good option for the first aim, while grounded theory wouldn’t be as relevant.
It’s also important to remember that each method has its own set of strengths, weaknesses and general limitations. No single analysis method is perfect . So, depending on the nature of your research, it may make sense to adopt more than one method (this is called triangulation ). Keep in mind though that this will of course be quite time-consuming.
As we’ve seen, all of the qualitative analysis methods we’ve discussed make use of coding and theme-generating techniques, but the intent and approach of each analysis method differ quite substantially. So, it’s very important to come into your research with a clear intention before you decide which analysis method (or methods) to use.
Start by reviewing your research aims , objectives and research questions to assess what exactly you’re trying to find out – then select a qualitative analysis method that fits. Never pick a method just because you like it or have experience using it – your analysis method (or methods) must align with your broader research aims and objectives.
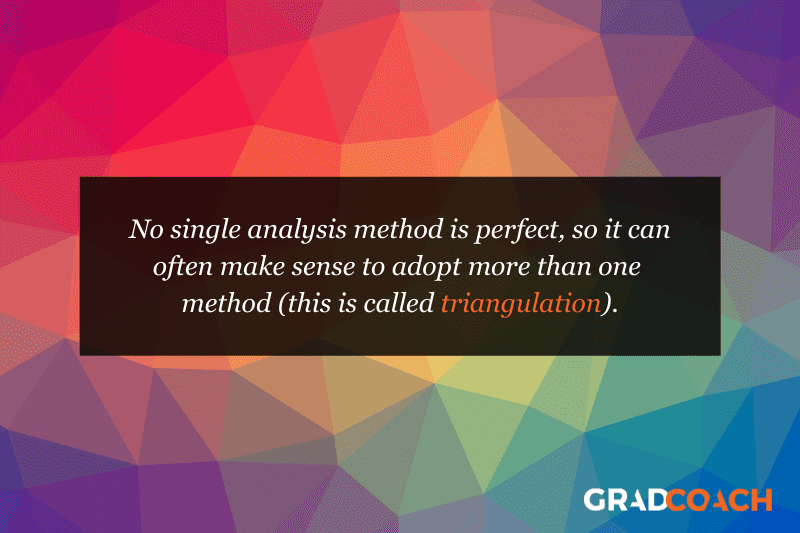
Let’s recap on QDA methods…
In this post, we looked at six popular qualitative data analysis methods:
- First, we looked at content analysis , a straightforward method that blends a little bit of quant into a primarily qualitative analysis.
- Then we looked at narrative analysis , which is about analysing how stories are told.
- Next up was discourse analysis – which is about analysing conversations and interactions.
- Then we moved on to thematic analysis – which is about identifying themes and patterns.
- From there, we went south with grounded theory – which is about starting from scratch with a specific question and using the data alone to build a theory in response to that question.
- And finally, we looked at IPA – which is about understanding people’s unique experiences of a phenomenon.
Of course, these aren’t the only options when it comes to qualitative data analysis, but they’re a great starting point if you’re dipping your toes into qualitative research for the first time.
If you’re still feeling a bit confused, consider our private coaching service , where we hold your hand through the research process to help you develop your best work.
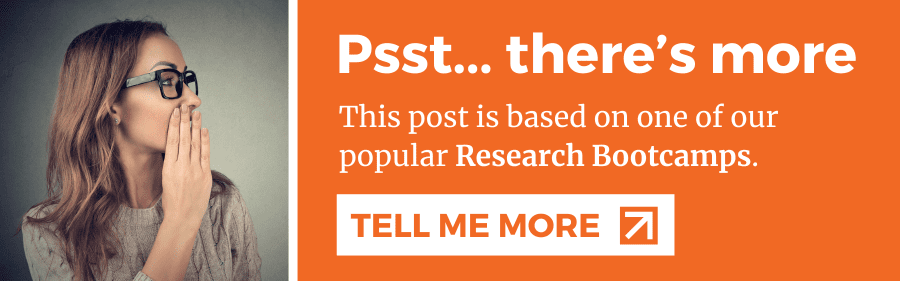
Learn More About Qualitative:
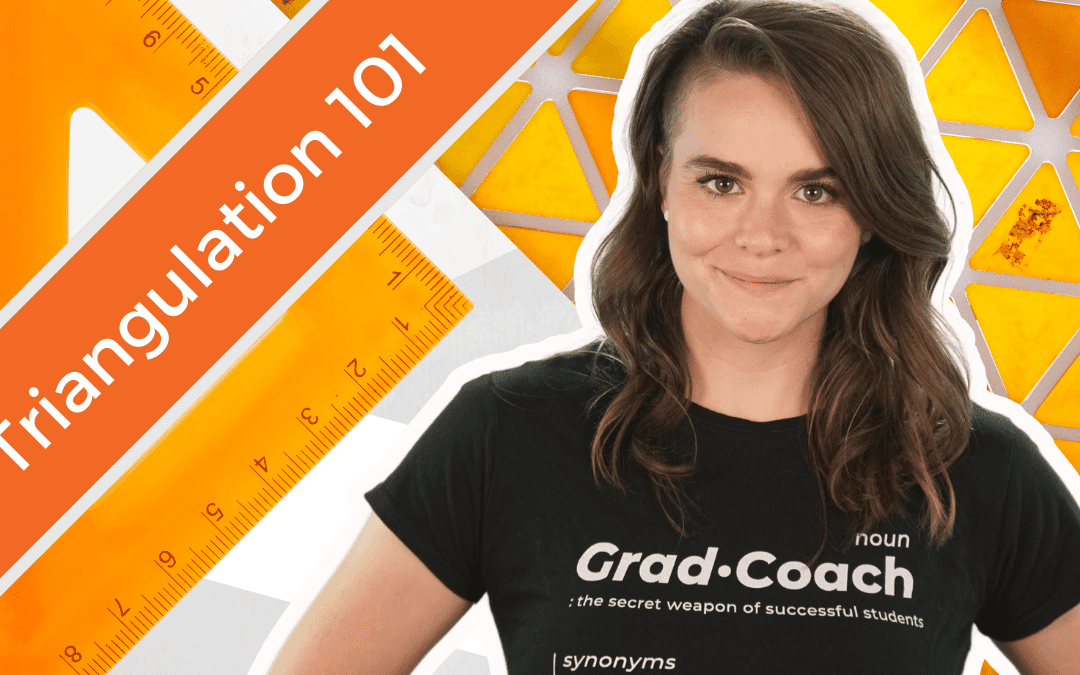
Triangulation: The Ultimate Credibility Enhancer
Triangulation is one of the best ways to enhance the credibility of your research. Learn about the different options here.
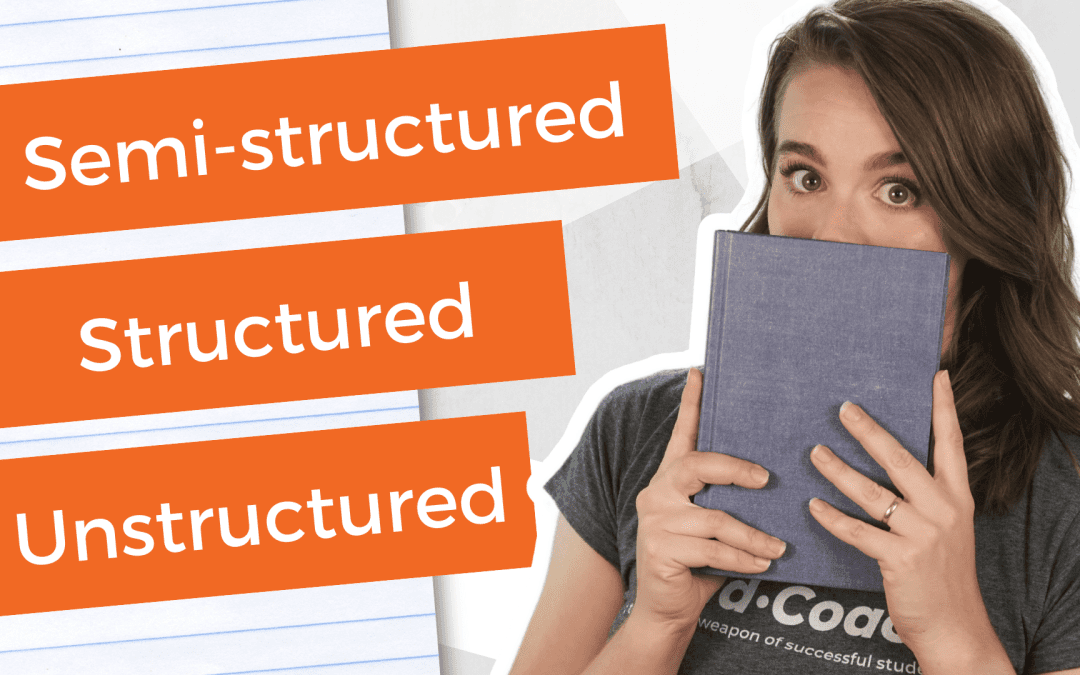
Structured, Semi-Structured & Unstructured Interviews
Learn about the differences (and similarities) between the three interview approaches: structured, semi-structured and unstructured.
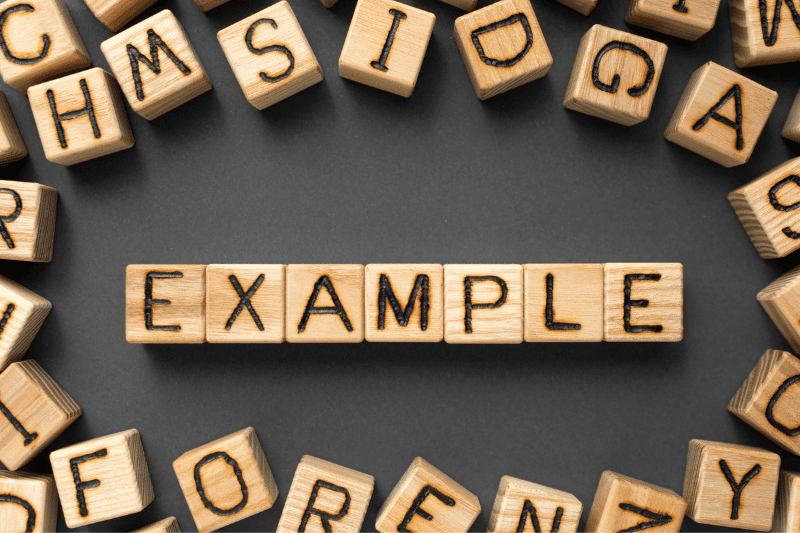
Qualitative Coding Examples: Process, Values & In Vivo Coding
See real-world examples of qualitative data that has been coded using process coding, values coding and in vivo coding.
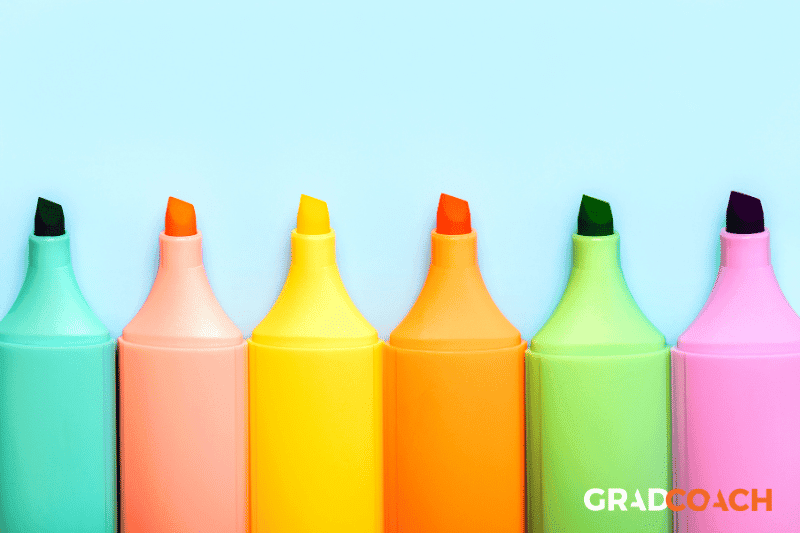
In Vivo Coding 101: Full Explainer With Examples
Learn about in vivo coding, a popular qualitative coding technique ideal for studies where the nuances of language are central to the aims.
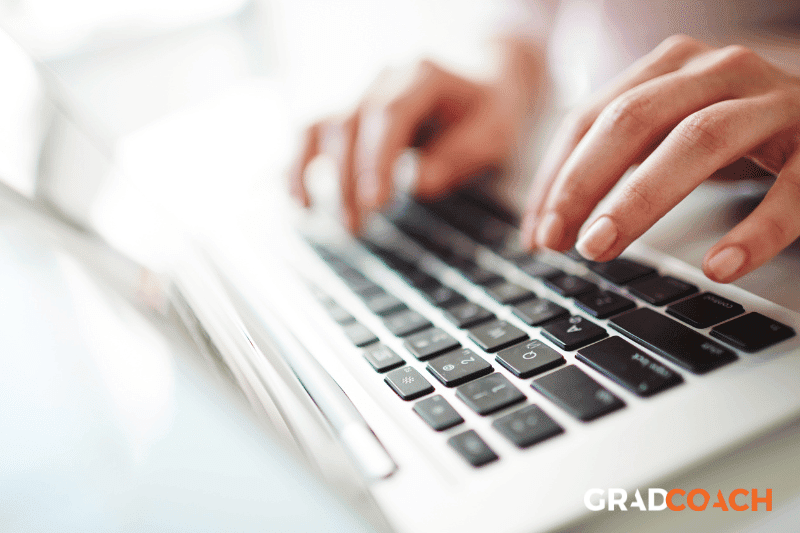
Process Coding 101: Full Explainer With Examples
Learn about process coding, a popular qualitative coding technique ideal for studies exploring processes, actions and changes over time.
📄 FREE TEMPLATES
Research Topic Ideation
Proposal Writing
Literature Review
Methodology & Analysis
Academic Writing
Referencing & Citing
Apps, Tools & Tricks
The Grad Coach Podcast
87 Comments
This has been very helpful. Thank you.
Thank you madam,
Thank you so much for this information
I wonder it so clear for understand and good for me. can I ask additional query?
Very insightful and useful
Good work done with clear explanations. Thank you.
Thanks so much for the write-up, it’s really good.
Thanks madam . It is very important .
thank you very good
Great presentation
This has been very well explained in simple language . It is useful even for a new researcher.
Great to hear that. Good luck with your qualitative data analysis, Pramod!
This is very useful information. And it was very a clear language structured presentation. Thanks a lot.
Thank you so much.
very informative sequential presentation
Precise explanation of method.
Hi, may we use 2 data analysis methods in our qualitative research?
Thanks for your comment. Most commonly, one would use one type of analysis method, but it depends on your research aims and objectives.
You explained it in very simple language, everyone can understand it. Thanks so much.
Thank you very much, this is very helpful. It has been explained in a very simple manner that even a layman understands
Thank nicely explained can I ask is Qualitative content analysis the same as thematic analysis?
Thanks for your comment. No, QCA and thematic are two different types of analysis. This article might help clarify – https://onlinelibrary.wiley.com/doi/10.1111/nhs.12048
This is my first time to come across a well explained data analysis. so helpful.
I have thoroughly enjoyed your explanation of the six qualitative analysis methods. This is very helpful. Thank you!
Thank you very much, this is well explained and useful
i need a citation of your book.
Thanks a lot , remarkable indeed, enlighting to the best
Hi Derek, What other theories/methods would you recommend when the data is a whole speech?
Keep writing useful artikel.
It is important concept about QDA and also the way to express is easily understandable, so thanks for all.
Thank you, this is well explained and very useful.
Very helpful .Thanks.
Hi there! Very well explained. Simple but very useful style of writing. Please provide the citation of the text. warm regards
The session was very helpful and insightful. Thank you
This was very helpful and insightful. Easy to read and understand
As a professional academic writer, this has been so informative and educative. Keep up the good work Grad Coach you are unmatched with quality content for sure.
Keep up the good work Grad Coach you are unmatched with quality content for sure.
Its Great and help me the most. A Million Thanks you Dr.
It is a very nice work
Very insightful. Please, which of this approach could be used for a research that one is trying to elicit students’ misconceptions in a particular concept ?
This is Amazing and well explained, thanks
great overview
What do we call a research data analysis method that one use to advise or determining the best accounting tool or techniques that should be adopted in a company.
Informative video, explained in a clear and simple way. Kudos
Waoo! I have chosen method wrong for my data analysis. But I can revise my work according to this guide. Thank you so much for this helpful lecture.
This has been very helpful. It gave me a good view of my research objectives and how to choose the best method. Thematic analysis it is.
Very helpful indeed. Thanku so much for the insight.
This was incredibly helpful.
Very helpful.
very educative
Nicely written especially for novice academic researchers like me! Thank you.
choosing a right method for a paper is always a hard job for a student, this is a useful information, but it would be more useful personally for me, if the author provide me with a little bit more information about the data analysis techniques in type of explanatory research. Can we use qualitative content analysis technique for explanatory research ? or what is the suitable data analysis method for explanatory research in social studies?
that was very helpful for me. because these details are so important to my research. thank you very much
I learnt a lot. Thank you
Relevant and Informative, thanks !
Well-planned and organized, thanks much! 🙂
I have reviewed qualitative data analysis in a simplest way possible. The content will highly be useful for developing my book on qualitative data analysis methods. Cheers!
Clear explanation on qualitative and how about Case study
This was helpful. Thank you
This was really of great assistance, it was just the right information needed. Explanation very clear and follow.
Wow, Thanks for making my life easy
This was helpful thanks .
Very helpful…. clear and written in an easily understandable manner. Thank you.
This was so helpful as it was easy to understand. I’m a new to research thank you so much.
so educative…. but Ijust want to know which method is coding of the qualitative or tallying done?
Thank you for the great content, I have learnt a lot. So helpful
precise and clear presentation with simple language and thank you for that.
very informative content, thank you.
You guys are amazing on YouTube on this platform. Your teachings are great, educative, and informative. kudos!
Brilliant Delivery. You made a complex subject seem so easy. Well done.
Beautifully explained.
Thanks a lot
Is there a video the captures the practical process of coding using automated applications?
Thanks for the comment. We don’t recommend using automated applications for coding, as they are not sufficiently accurate in our experience.
content analysis can be qualitative research?
THANK YOU VERY MUCH.
Thank you very much for such a wonderful content
do you have any material on Data collection
What a powerful explanation of the QDA methods. Thank you.
Great explanation both written and Video. i have been using of it on a day to day working of my thesis project in accounting and finance. Thank you very much for your support.
very helpful, thank you so much
The tutorial is useful. I benefited a lot.
This is an eye opener for me and very informative, I have used some of your guidance notes on my Thesis, I wonder if you can assist with your 1. name of your book, year of publication, topic etc., this is for citing in my Bibliography,
I certainly hope to hear from you
Submit a Comment Cancel reply
Your email address will not be published. Required fields are marked *
Save my name, email, and website in this browser for the next time I comment.
Submit Comment
- Print Friendly
Have a language expert improve your writing
Run a free plagiarism check in 10 minutes, generate accurate citations for free.
- Knowledge Base
Methodology
- What Is Qualitative Research? | Methods & Examples
What Is Qualitative Research? | Methods & Examples
Published on June 19, 2020 by Pritha Bhandari . Revised on September 5, 2024.
Qualitative research involves collecting and analyzing non-numerical data (e.g., text, video, or audio) to understand concepts, opinions, or experiences. It can be used to gather in-depth insights into a problem or generate new ideas for research.
Qualitative research is the opposite of quantitative research , which involves collecting and analyzing numerical data for statistical analysis.
Qualitative research is commonly used in the humanities and social sciences, in subjects such as anthropology, sociology, education, health sciences, history, etc.
- How does social media shape body image in teenagers?
- How do children and adults interpret healthy eating in the UK?
- What factors influence employee retention in a large organization?
- How is anxiety experienced around the world?
- How can teachers integrate social issues into science curriculums?
Table of contents
Approaches to qualitative research, qualitative research methods, qualitative data analysis, advantages of qualitative research, disadvantages of qualitative research, other interesting articles, frequently asked questions about qualitative research.
Qualitative research is used to understand how people experience the world. While there are many approaches to qualitative research, they tend to be flexible and focus on retaining rich meaning when interpreting data.
Common approaches include grounded theory, ethnography , action research , phenomenological research, and narrative research. They share some similarities, but emphasize different aims and perspectives.
Approach | What does it involve? |
---|---|
Grounded theory | Researchers collect rich data on a topic of interest and develop theories . |
Researchers immerse themselves in groups or organizations to understand their cultures. | |
Action research | Researchers and participants collaboratively link theory to practice to drive social change. |
Phenomenological research | Researchers investigate a phenomenon or event by describing and interpreting participants’ lived experiences. |
Narrative research | Researchers examine how stories are told to understand how participants perceive and make sense of their experiences. |
Note that qualitative research is at risk for certain research biases including the Hawthorne effect , observer bias , recall bias , and social desirability bias . While not always totally avoidable, awareness of potential biases as you collect and analyze your data can prevent them from impacting your work too much.
Prevent plagiarism. Run a free check.
Each of the research approaches involve using one or more data collection methods . These are some of the most common qualitative methods:
- Observations: recording what you have seen, heard, or encountered in detailed field notes.
- Interviews: personally asking people questions in one-on-one conversations.
- Focus groups: asking questions and generating discussion among a group of people.
- Surveys : distributing questionnaires with open-ended questions.
- Secondary research: collecting existing data in the form of texts, images, audio or video recordings, etc.
- You take field notes with observations and reflect on your own experiences of the company culture.
- You distribute open-ended surveys to employees across all the company’s offices by email to find out if the culture varies across locations.
- You conduct in-depth interviews with employees in your office to learn about their experiences and perspectives in greater detail.
Qualitative researchers often consider themselves “instruments” in research because all observations, interpretations and analyses are filtered through their own personal lens.
For this reason, when writing up your methodology for qualitative research, it’s important to reflect on your approach and to thoroughly explain the choices you made in collecting and analyzing the data.
Qualitative data can take the form of texts, photos, videos and audio. For example, you might be working with interview transcripts, survey responses, fieldnotes, or recordings from natural settings.
Most types of qualitative data analysis share the same five steps:
- Prepare and organize your data. This may mean transcribing interviews or typing up fieldnotes.
- Review and explore your data. Examine the data for patterns or repeated ideas that emerge.
- Develop a data coding system. Based on your initial ideas, establish a set of codes that you can apply to categorize your data.
- Assign codes to the data. For example, in qualitative survey analysis, this may mean going through each participant’s responses and tagging them with codes in a spreadsheet. As you go through your data, you can create new codes to add to your system if necessary.
- Identify recurring themes. Link codes together into cohesive, overarching themes.
There are several specific approaches to analyzing qualitative data. Although these methods share similar processes, they emphasize different concepts.
Approach | When to use | Example |
---|---|---|
To describe and categorize common words, phrases, and ideas in qualitative data. | A market researcher could perform content analysis to find out what kind of language is used in descriptions of therapeutic apps. | |
To identify and interpret patterns and themes in qualitative data. | A psychologist could apply thematic analysis to travel blogs to explore how tourism shapes self-identity. | |
To examine the content, structure, and design of texts. | A media researcher could use textual analysis to understand how news coverage of celebrities has changed in the past decade. | |
To study communication and how language is used to achieve effects in specific contexts. | A political scientist could use discourse analysis to study how politicians generate trust in election campaigns. |
Qualitative research often tries to preserve the voice and perspective of participants and can be adjusted as new research questions arise. Qualitative research is good for:
- Flexibility
The data collection and analysis process can be adapted as new ideas or patterns emerge. They are not rigidly decided beforehand.
- Natural settings
Data collection occurs in real-world contexts or in naturalistic ways.
- Meaningful insights
Detailed descriptions of people’s experiences, feelings and perceptions can be used in designing, testing or improving systems or products.
- Generation of new ideas
Open-ended responses mean that researchers can uncover novel problems or opportunities that they wouldn’t have thought of otherwise.
Researchers must consider practical and theoretical limitations in analyzing and interpreting their data. Qualitative research suffers from:
- Unreliability
The real-world setting often makes qualitative research unreliable because of uncontrolled factors that affect the data.
- Subjectivity
Due to the researcher’s primary role in analyzing and interpreting data, qualitative research cannot be replicated . The researcher decides what is important and what is irrelevant in data analysis, so interpretations of the same data can vary greatly.
- Limited generalizability
Small samples are often used to gather detailed data about specific contexts. Despite rigorous analysis procedures, it is difficult to draw generalizable conclusions because the data may be biased and unrepresentative of the wider population .
- Labor-intensive
Although software can be used to manage and record large amounts of text, data analysis often has to be checked or performed manually.
If you want to know more about statistics , methodology , or research bias , make sure to check out some of our other articles with explanations and examples.
- Chi square goodness of fit test
- Degrees of freedom
- Null hypothesis
- Discourse analysis
- Control groups
- Mixed methods research
- Non-probability sampling
- Quantitative research
- Inclusion and exclusion criteria
Research bias
- Rosenthal effect
- Implicit bias
- Cognitive bias
- Selection bias
- Negativity bias
- Status quo bias
Quantitative research deals with numbers and statistics, while qualitative research deals with words and meanings.
Quantitative methods allow you to systematically measure variables and test hypotheses . Qualitative methods allow you to explore concepts and experiences in more detail.
There are five common approaches to qualitative research :
- Grounded theory involves collecting data in order to develop new theories.
- Ethnography involves immersing yourself in a group or organization to understand its culture.
- Narrative research involves interpreting stories to understand how people make sense of their experiences and perceptions.
- Phenomenological research involves investigating phenomena through people’s lived experiences.
- Action research links theory and practice in several cycles to drive innovative changes.
Data collection is the systematic process by which observations or measurements are gathered in research. It is used in many different contexts by academics, governments, businesses, and other organizations.
There are various approaches to qualitative data analysis , but they all share five steps in common:
- Prepare and organize your data.
- Review and explore your data.
- Develop a data coding system.
- Assign codes to the data.
- Identify recurring themes.
The specifics of each step depend on the focus of the analysis. Some common approaches include textual analysis , thematic analysis , and discourse analysis .
Cite this Scribbr article
If you want to cite this source, you can copy and paste the citation or click the “Cite this Scribbr article” button to automatically add the citation to our free Citation Generator.
Bhandari, P. (2024, September 05). What Is Qualitative Research? | Methods & Examples. Scribbr. Retrieved September 27, 2024, from https://www.scribbr.com/methodology/qualitative-research/
Is this article helpful?
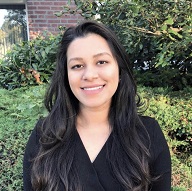
Pritha Bhandari
Other students also liked, qualitative vs. quantitative research | differences, examples & methods, how to do thematic analysis | step-by-step guide & examples, what is your plagiarism score.
- Skip to main content
- Skip to primary sidebar
- Skip to footer
- QuestionPro

- Solutions Industries Gaming Automotive Sports and events Education Government Travel & Hospitality Financial Services Healthcare Cannabis Technology Use Case AskWhy Communities Audience Contactless surveys Mobile LivePolls Member Experience GDPR Positive People Science 360 Feedback Surveys
- Resources Blog eBooks Survey Templates Case Studies Training Help center

Home Market Research
Qualitative Data Analysis: What is it, Methods + Examples
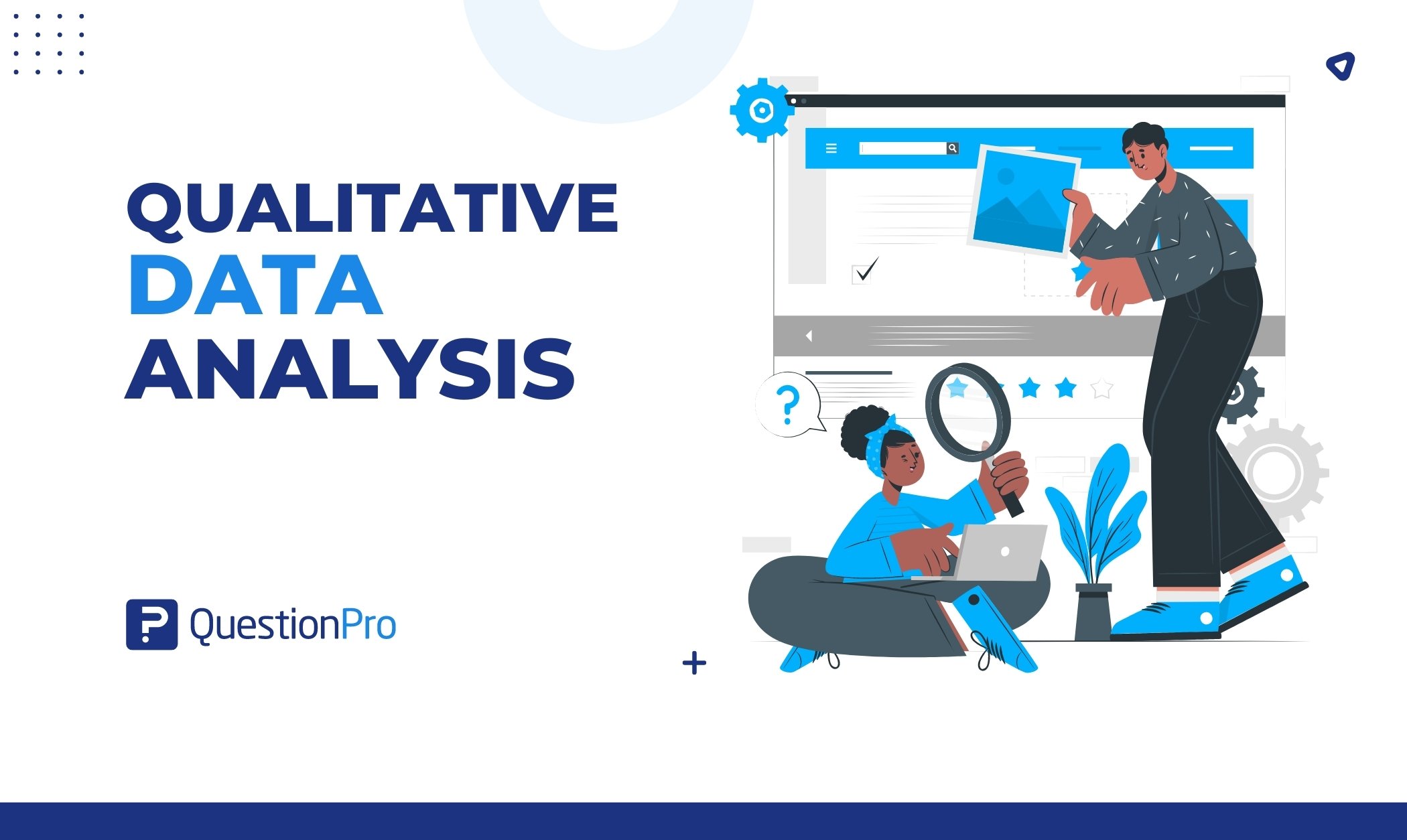
In a world rich with information and narrative, understanding the deeper layers of human experiences requires a unique vision that goes beyond numbers and figures. This is where the power of qualitative data analysis comes to light.
In this blog, we’ll learn about qualitative data analysis, explore its methods, and provide real-life examples showcasing its power in uncovering insights.
What is Qualitative Data Analysis?
Qualitative data analysis is a systematic process of examining non-numerical data to extract meaning, patterns, and insights.
In contrast to quantitative analysis, which focuses on numbers and statistical metrics, the qualitative study focuses on the qualitative aspects of data, such as text, images, audio, and videos. It seeks to understand every aspect of human experiences, perceptions, and behaviors by examining the data’s richness.
Companies frequently conduct this analysis on customer feedback. You can collect qualitative data from reviews, complaints, chat messages, interactions with support centers, customer interviews, case notes, or even social media comments. This kind of data holds the key to understanding customer sentiments and preferences in a way that goes beyond mere numbers.
Importance of Qualitative Data Analysis
Qualitative data analysis plays a crucial role in your research and decision-making process across various disciplines. Let’s explore some key reasons that underline the significance of this analysis:
In-Depth Understanding
It enables you to explore complex and nuanced aspects of a phenomenon, delving into the ‘how’ and ‘why’ questions. This method provides you with a deeper understanding of human behavior, experiences, and contexts that quantitative approaches might not capture fully.
Contextual Insight
You can use this analysis to give context to numerical data. It will help you understand the circumstances and conditions that influence participants’ thoughts, feelings, and actions. This contextual insight becomes essential for generating comprehensive explanations.
Theory Development
You can generate or refine hypotheses via qualitative data analysis. As you analyze the data attentively, you can form hypotheses, concepts, and frameworks that will drive your future research and contribute to theoretical advances.
Participant Perspectives
When performing qualitative research, you can highlight participant voices and opinions. This approach is especially useful for understanding marginalized or underrepresented people, as it allows them to communicate their experiences and points of view.
Exploratory Research
The analysis is frequently used at the exploratory stage of your project. It assists you in identifying important variables, developing research questions, and designing quantitative studies that will follow.
Types of Qualitative Data
When conducting qualitative research, you can use several qualitative data collection methods , and here you will come across many sorts of qualitative data that can provide you with unique insights into your study topic. These data kinds add new views and angles to your understanding and analysis.
Interviews and Focus Groups
Interviews and focus groups will be among your key methods for gathering qualitative data. Interviews are one-on-one talks in which participants can freely share their thoughts, experiences, and opinions.
Focus groups, on the other hand, are discussions in which members interact with one another, resulting in dynamic exchanges of ideas. Both methods provide rich qualitative data and direct access to participant perspectives.
Observations and Field Notes
Observations and field notes are another useful sort of qualitative data. You can immerse yourself in the research environment through direct observation, carefully documenting behaviors, interactions, and contextual factors.
These observations will be recorded in your field notes, providing a complete picture of the environment and the behaviors you’re researching. This data type is especially important for comprehending behavior in their natural setting.
Textual and Visual Data
Textual and visual data include a wide range of resources that can be qualitatively analyzed. Documents, written narratives, and transcripts from various sources, such as interviews or speeches, are examples of textual data.
Photographs, films, and even artwork provide a visual layer to your research. These forms of data allow you to investigate what is spoken and the underlying emotions, details, and symbols expressed by language or pictures.
When to Choose Qualitative Data Analysis over Quantitative Data Analysis
As you begin your research journey, understanding why the analysis of qualitative data is important will guide your approach to understanding complex events. If you analyze qualitative data, it will provide new insights that complement quantitative methodologies, which will give you a broader understanding of your study topic.
It is critical to know when to use qualitative analysis over quantitative procedures. You can prefer qualitative data analysis when:
- Complexity Reigns: When your research questions involve deep human experiences, motivations, or emotions, qualitative research excels at revealing these complexities.
- Exploration is Key: Qualitative analysis is ideal for exploratory research. It will assist you in understanding a new or poorly understood topic before formulating quantitative hypotheses.
- Context Matters: If you want to understand how context affects behaviors or results, qualitative data analysis provides the depth needed to grasp these relationships.
- Unanticipated Findings: When your study provides surprising new viewpoints or ideas, qualitative analysis helps you to delve deeply into these emerging themes.
- Subjective Interpretation is Vital: When it comes to understanding people’s subjective experiences and interpretations, qualitative data analysis is the way to go.
You can make informed decisions regarding the right approach for your research objectives if you understand the importance of qualitative analysis and recognize the situations where it shines.
Qualitative Data Analysis Methods and Examples
Exploring various qualitative data analysis methods will provide you with a wide collection for making sense of your research findings. Once the data has been collected, you can choose from several analysis methods based on your research objectives and the data type you’ve collected.
There are five main methods for analyzing qualitative data. Each method takes a distinct approach to identifying patterns, themes, and insights within your qualitative data. They are:
Method 1: Content Analysis
Content analysis is a methodical technique for analyzing textual or visual data in a structured manner. In this method, you will categorize qualitative data by splitting it into manageable pieces and assigning the manual coding process to these units.
As you go, you’ll notice ongoing codes and designs that will allow you to conclude the content. This method is very beneficial for detecting common ideas, concepts, or themes in your data without losing the context.
Steps to Do Content Analysis
Follow these steps when conducting content analysis:
- Collect and Immerse: Begin by collecting the necessary textual or visual data. Immerse yourself in this data to fully understand its content, context, and complexities.
- Assign Codes and Categories: Assign codes to relevant data sections that systematically represent major ideas or themes. Arrange comparable codes into groups that cover the major themes.
- Analyze and Interpret: Develop a structured framework from the categories and codes. Then, evaluate the data in the context of your research question, investigate relationships between categories, discover patterns, and draw meaning from these connections.
Benefits & Challenges
There are various advantages to using content analysis:
- Structured Approach: It offers a systematic approach to dealing with large data sets and ensures consistency throughout the research.
- Objective Insights: This method promotes objectivity, which helps to reduce potential biases in your study.
- Pattern Discovery: Content analysis can help uncover hidden trends, themes, and patterns that are not always obvious.
- Versatility: You can apply content analysis to various data formats, including text, internet content, images, etc.
However, keep in mind the challenges that arise:
- Subjectivity: Even with the best attempts, a certain bias may remain in coding and interpretation.
- Complexity: Analyzing huge data sets requires time and great attention to detail.
- Contextual Nuances: Content analysis may not capture all of the contextual richness that qualitative data analysis highlights.
Example of Content Analysis
Suppose you’re conducting market research and looking at customer feedback on a product. As you collect relevant data and analyze feedback, you’ll see repeating codes like “price,” “quality,” “customer service,” and “features.” These codes are organized into categories such as “positive reviews,” “negative reviews,” and “suggestions for improvement.”
According to your findings, themes such as “price” and “customer service” stand out and show that pricing and customer service greatly impact customer satisfaction. This example highlights the power of content analysis for obtaining significant insights from large textual data collections.
Method 2: Thematic Analysis
Thematic analysis is a well-structured procedure for identifying and analyzing recurring themes in your data. As you become more engaged in the data, you’ll generate codes or short labels representing key concepts. These codes are then organized into themes, providing a consistent framework for organizing and comprehending the substance of the data.
The analysis allows you to organize complex narratives and perspectives into meaningful categories, which will allow you to identify connections and patterns that may not be visible at first.
Steps to Do Thematic Analysis
Follow these steps when conducting a thematic analysis:
- Code and Group: Start by thoroughly examining the data and giving initial codes that identify the segments. To create initial themes, combine relevant codes.
- Code and Group: Begin by engaging yourself in the data, assigning first codes to notable segments. To construct basic themes, group comparable codes together.
- Analyze and Report: Analyze the data within each theme to derive relevant insights. Organize the topics into a consistent structure and explain your findings, along with data extracts that represent each theme.
Thematic analysis has various benefits:
- Structured Exploration: It is a method for identifying patterns and themes in complex qualitative data.
- Comprehensive knowledge: Thematic analysis promotes an in-depth understanding of the complications and meanings of the data.
- Application Flexibility: This method can be customized to various research situations and data kinds.
However, challenges may arise, such as:
- Interpretive Nature: Interpreting qualitative data in thematic analysis is vital, and it is critical to manage researcher bias.
- Time-consuming: The study can be time-consuming, especially with large data sets.
- Subjectivity: The selection of codes and topics might be subjective.
Example of Thematic Analysis
Assume you’re conducting a thematic analysis on job satisfaction interviews. Following your immersion in the data, you assign initial codes such as “work-life balance,” “career growth,” and “colleague relationships.” As you organize these codes, you’ll notice themes develop, such as “Factors Influencing Job Satisfaction” and “Impact on Work Engagement.”
Further investigation reveals the tales and experiences included within these themes and provides insights into how various elements influence job satisfaction. This example demonstrates how thematic analysis can reveal meaningful patterns and insights in qualitative data.
Method 3: Narrative Analysis
The narrative analysis involves the narratives that people share. You’ll investigate the histories in your data, looking at how stories are created and the meanings they express. This method is excellent for learning how people make sense of their experiences through narrative.
Steps to Do Narrative Analysis
The following steps are involved in narrative analysis:
- Gather and Analyze: Start by collecting narratives, such as first-person tales, interviews, or written accounts. Analyze the stories, focusing on the plot, feelings, and characters.
- Find Themes: Look for recurring themes or patterns in various narratives. Think about the similarities and differences between these topics and personal experiences.
- Interpret and Extract Insights: Contextualize the narratives within their larger context. Accept the subjective nature of each narrative and analyze the narrator’s voice and style. Extract insights from the tales by diving into the emotions, motivations, and implications communicated by the stories.
There are various advantages to narrative analysis:
- Deep Exploration: It lets you look deeply into people’s personal experiences and perspectives.
- Human-Centered: This method prioritizes the human perspective, allowing individuals to express themselves.
However, difficulties may arise, such as:
- Interpretive Complexity: Analyzing narratives requires dealing with the complexities of meaning and interpretation.
- Time-consuming: Because of the richness and complexities of tales, working with them can be time-consuming.
Example of Narrative Analysis
Assume you’re conducting narrative analysis on refugee interviews. As you read the stories, you’ll notice common themes of toughness, loss, and hope. The narratives provide insight into the obstacles that refugees face, their strengths, and the dreams that guide them.
The analysis can provide a deeper insight into the refugees’ experiences and the broader social context they navigate by examining the narratives’ emotional subtleties and underlying meanings. This example highlights how narrative analysis can reveal important insights into human stories.
Method 4: Grounded Theory Analysis
Grounded theory analysis is an iterative and systematic approach that allows you to create theories directly from data without being limited by pre-existing hypotheses. With an open mind, you collect data and generate early codes and labels that capture essential ideas or concepts within the data.
As you progress, you refine these codes and increasingly connect them, eventually developing a theory based on the data. Grounded theory analysis is a dynamic process for developing new insights and hypotheses based on details in your data.
Steps to Do Grounded Theory Analysis
Grounded theory analysis requires the following steps:
- Initial Coding: First, immerse yourself in the data, producing initial codes that represent major concepts or patterns.
- Categorize and Connect: Using axial coding, organize the initial codes, which establish relationships and connections between topics.
- Build the Theory: Focus on creating a core category that connects the codes and themes. Regularly refine the theory by comparing and integrating new data, ensuring that it evolves organically from the data.
Grounded theory analysis has various benefits:
- Theory Generation: It provides a one-of-a-kind opportunity to generate hypotheses straight from data and promotes new insights.
- In-depth Understanding: The analysis allows you to deeply analyze the data and reveal complex relationships and patterns.
- Flexible Process: This method is customizable and ongoing, which allows you to enhance your research as you collect additional data.
However, challenges might arise with:
- Time and Resources: Because grounded theory analysis is a continuous process, it requires a large commitment of time and resources.
- Theoretical Development: Creating a grounded theory involves a thorough understanding of qualitative data analysis software and theoretical concepts.
- Interpretation of Complexity: Interpreting and incorporating a newly developed theory into existing literature can be intellectually hard.
Example of Grounded Theory Analysis
Assume you’re performing a grounded theory analysis on workplace collaboration interviews. As you open code the data, you will discover notions such as “communication barriers,” “team dynamics,” and “leadership roles.” Axial coding demonstrates links between these notions, emphasizing the significance of efficient communication in developing collaboration.
You create the core “Integrated Communication Strategies” category through selective coding, which unifies new topics.
This theory-driven category serves as the framework for understanding how numerous aspects contribute to effective team collaboration. This example shows how grounded theory analysis allows you to generate a theory directly from the inherent nature of the data.
Method 5: Discourse Analysis
Discourse analysis focuses on language and communication. You’ll look at how language produces meaning and how it reflects power relations, identities, and cultural influences. This strategy examines what is said and how it is said; the words, phrasing, and larger context of communication.
The analysis is precious when investigating power dynamics, identities, and cultural influences encoded in language. By evaluating the language used in your data, you can identify underlying assumptions, cultural standards, and how individuals negotiate meaning through communication.
Steps to Do Discourse Analysis
Conducting discourse analysis entails the following steps:
- Select Discourse: For analysis, choose language-based data such as texts, speeches, or media content.
- Analyze Language: Immerse yourself in the conversation, examining language choices, metaphors, and underlying assumptions.
- Discover Patterns: Recognize the dialogue’s reoccurring themes, ideologies, and power dynamics. To fully understand the effects of these patterns, put them in their larger context.
There are various advantages of using discourse analysis:
- Understanding Language: It provides an extensive understanding of how language builds meaning and influences perceptions.
- Uncovering Power Dynamics: The analysis reveals how power dynamics appear via language.
- Cultural Insights: This method identifies cultural norms, beliefs, and ideologies stored in communication.
However, the following challenges may arise:
- Complexity of Interpretation: Language analysis involves navigating multiple levels of nuance and interpretation.
- Subjectivity: Interpretation can be subjective, so controlling researcher bias is important.
- Time-Intensive: Discourse analysis can take a lot of time because careful linguistic study is required in this analysis.
Example of Discourse Analysis
Consider doing discourse analysis on media coverage of a political event. You notice repeating linguistic patterns in news articles that depict the event as a conflict between opposing parties. Through deconstruction, you can expose how this framing supports particular ideologies and power relations.
You can illustrate how language choices influence public perceptions and contribute to building the narrative around the event by analyzing the speech within the broader political and social context. This example shows how discourse analysis can reveal hidden power dynamics and cultural influences on communication.
How to do Qualitative Data Analysis with the QuestionPro Research suite?
QuestionPro is a popular survey and research platform that offers tools for collecting and analyzing qualitative and quantitative data. Follow these general steps for conducting qualitative data analysis using the QuestionPro Research Suite:
- Collect Qualitative Data: Set up your survey to capture qualitative responses. It might involve open-ended questions, text boxes, or comment sections where participants can provide detailed responses.
- Export Qualitative Responses: Export the responses once you’ve collected qualitative data through your survey. QuestionPro typically allows you to export survey data in various formats, such as Excel or CSV.
- Prepare Data for Analysis: Review the exported data and clean it if necessary. Remove irrelevant or duplicate entries to ensure your data is ready for analysis.
- Code and Categorize Responses: Segment and label data, letting new patterns emerge naturally, then develop categories through axial coding to structure the analysis.
- Identify Themes: Analyze the coded responses to identify recurring themes, patterns, and insights. Look for similarities and differences in participants’ responses.
- Generate Reports and Visualizations: Utilize the reporting features of QuestionPro to create visualizations, charts, and graphs that help communicate the themes and findings from your qualitative research.
- Interpret and Draw Conclusions: Interpret the themes and patterns you’ve identified in the qualitative data. Consider how these findings answer your research questions or provide insights into your study topic.
- Integrate with Quantitative Data (if applicable): If you’re also conducting quantitative research using QuestionPro, consider integrating your qualitative findings with quantitative results to provide a more comprehensive understanding.
Qualitative data analysis is vital in uncovering various human experiences, views, and stories. If you’re ready to transform your research journey and apply the power of qualitative analysis, now is the moment to do it. Book a demo with QuestionPro today and begin your journey of exploration.
LEARN MORE FREE TRIAL
MORE LIKE THIS
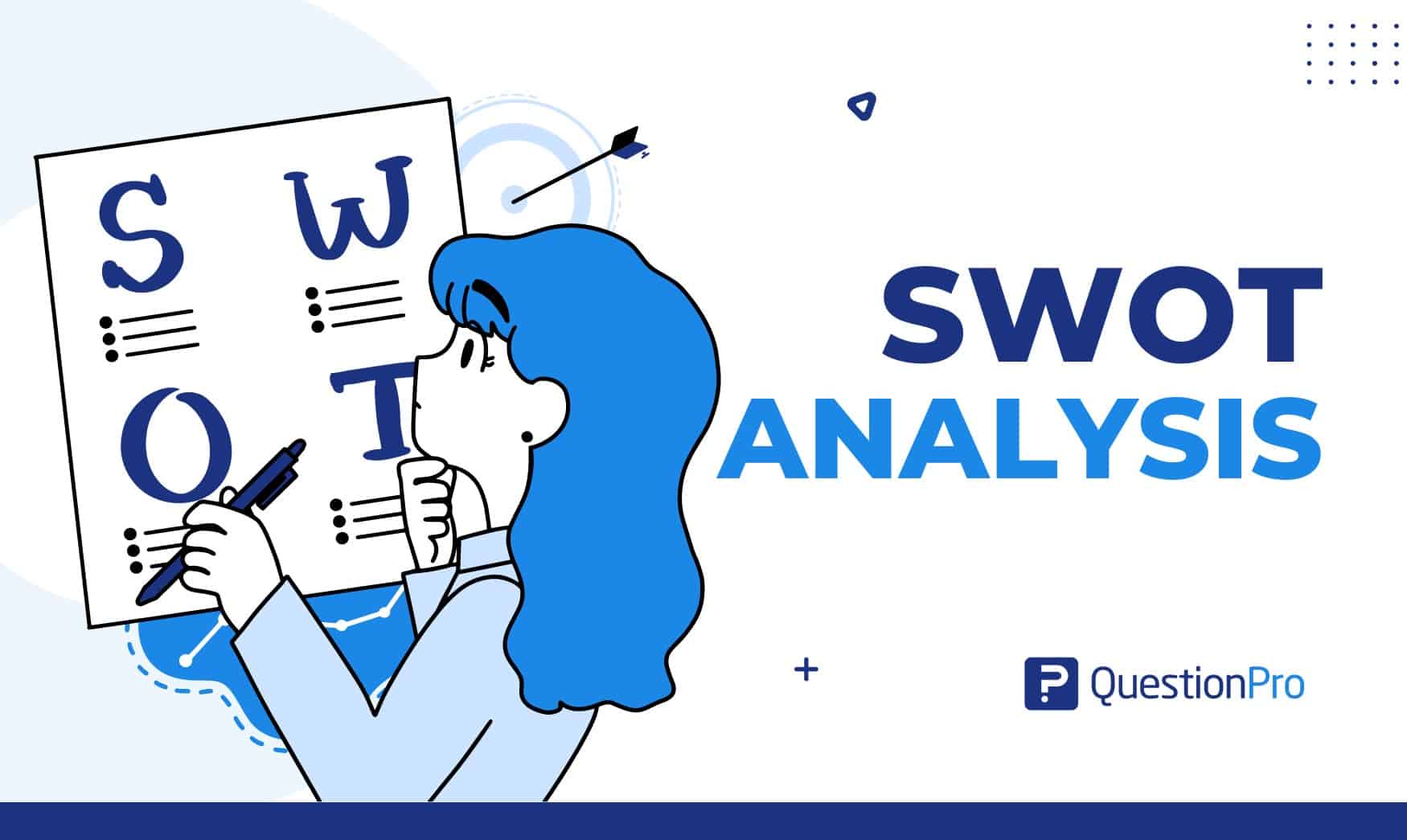
SWOT Analysis: What It Is And How To Do It?
Sep 27, 2024
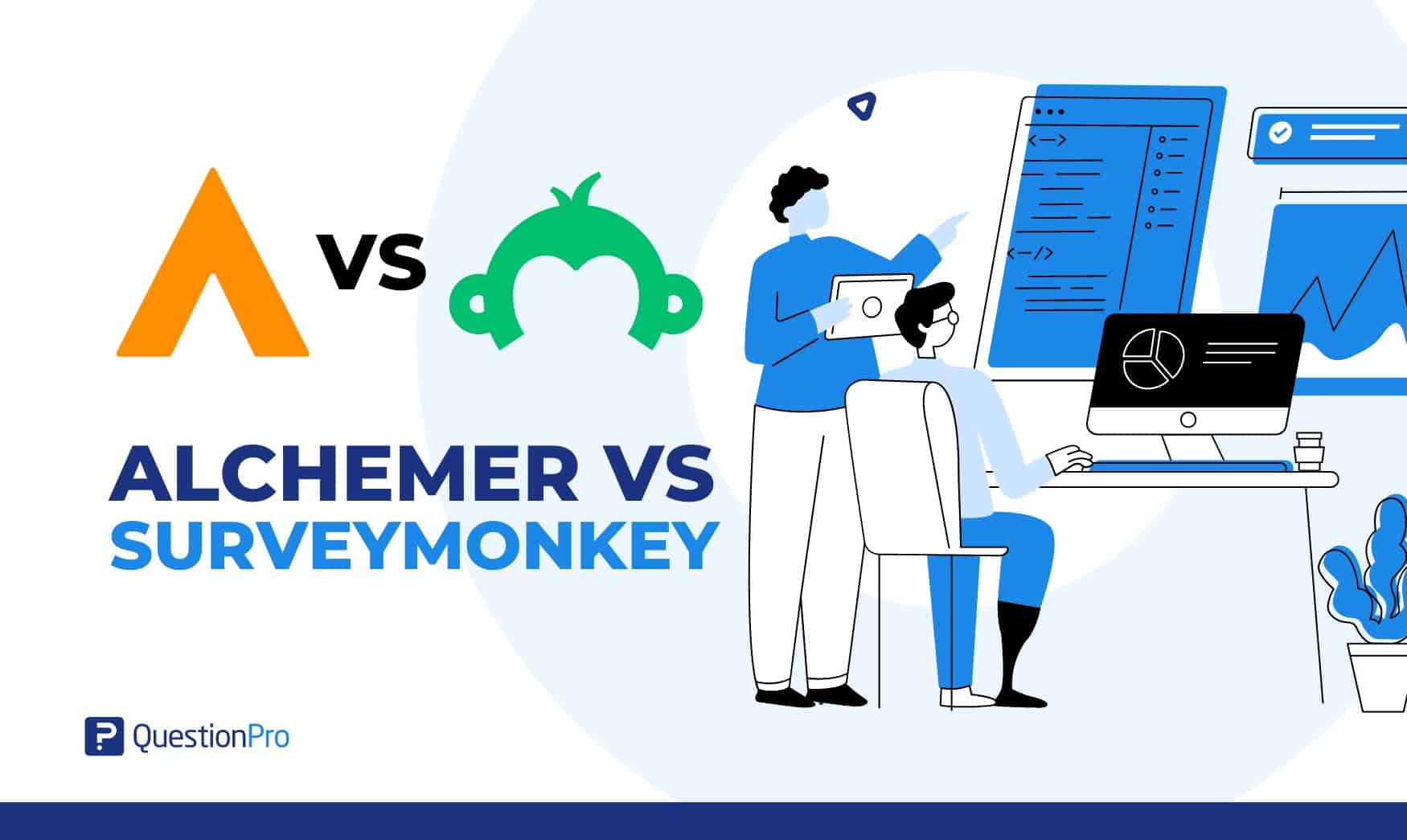
Alchemer vs SurveyMonkey: Which Survey Tool Is Best for You
Sep 26, 2024
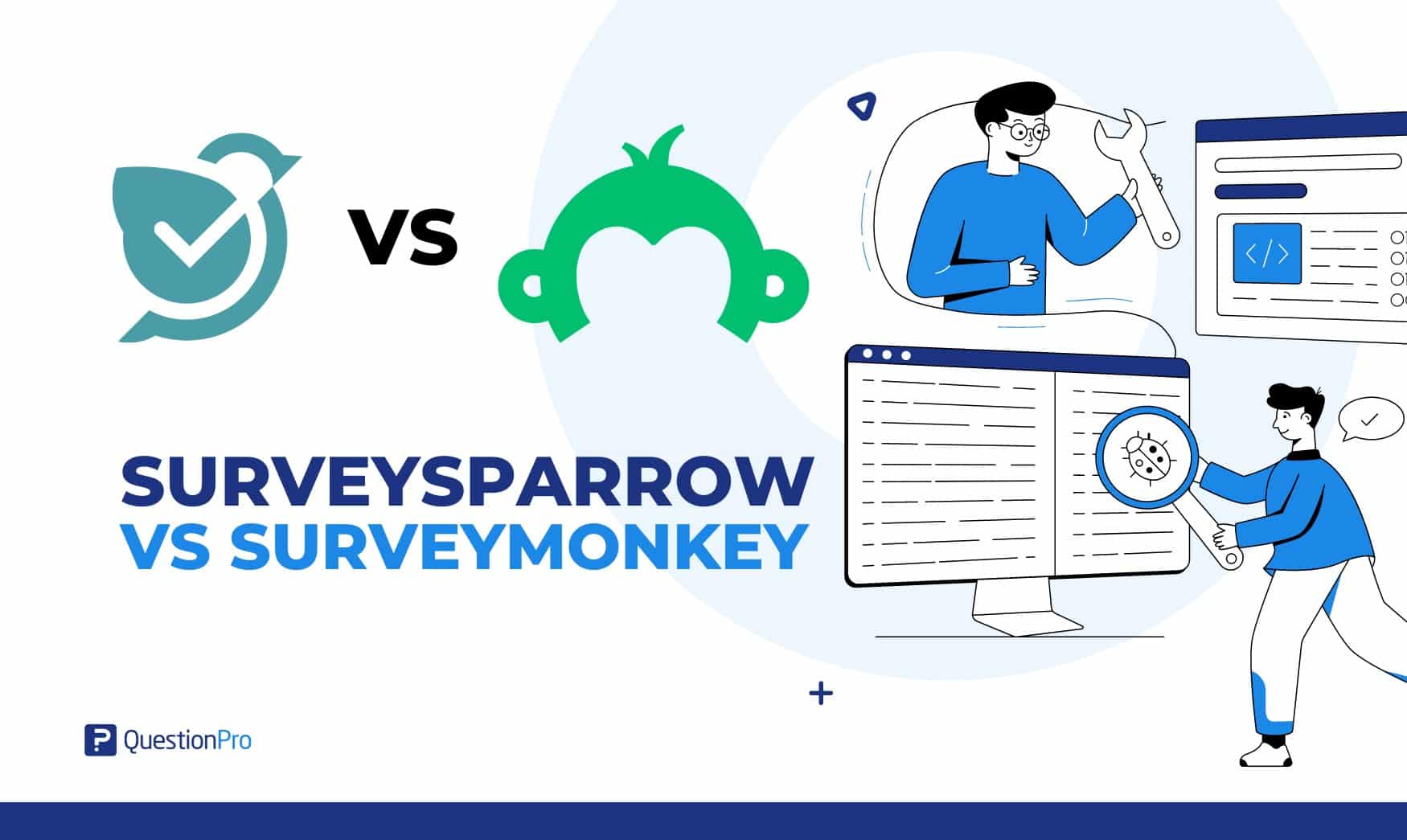
SurveySparrow vs SurveyMonkey: Choosing the Right Survey Tool
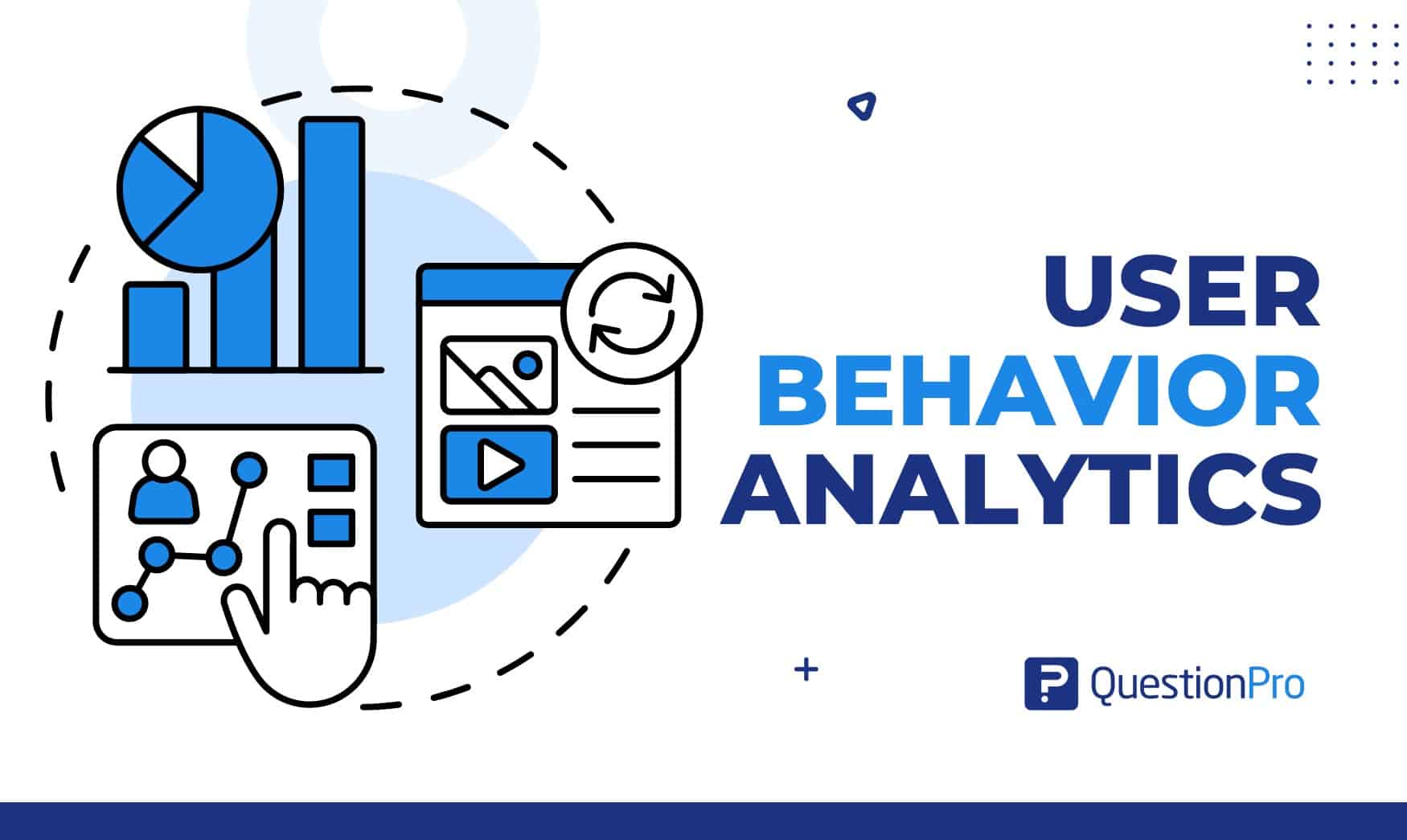
User Behavior Analytics: What it is, Importance, Uses & Tools
Other categories.
- Academic Research
- Artificial Intelligence
- Assessments
- Brand Awareness
- Case Studies
- Communities
- Consumer Insights
- Customer effort score
- Customer Engagement
- Customer Experience
- Customer Loyalty
- Customer Research
- Customer Satisfaction
- Employee Benefits
- Employee Engagement
- Employee Retention
- Friday Five
- General Data Protection Regulation
- Insights Hub
- Life@QuestionPro
- Market Research
- Mobile diaries
- Mobile Surveys
- New Features
- Online Communities
- Question Types
- Questionnaire
- QuestionPro Products
- Release Notes
- Research Tools and Apps
- Revenue at Risk
- Survey Templates
- Training Tips
- Tuesday CX Thoughts (TCXT)
- Uncategorized
- What’s Coming Up
- Workforce Intelligence

The Ultimate Guide to Qualitative Research - Part 2: Handling Qualitative Data
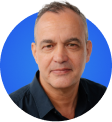
- Handling qualitative data
- Transcripts
- Field notes
- Survey data and responses
- Visual and audio data
- Data organization
- Data coding
- Coding frame
- Auto and smart coding
- Organizing codes
- Introduction
What is qualitative data analysis?
Qualitative data analysis methods, how do you analyze qualitative data, content analysis, thematic analysis.
- Thematic analysis vs. content analysis
- Narrative research
Phenomenological research
Discourse analysis, grounded theory.
- Deductive reasoning
- Inductive reasoning
- Inductive vs. deductive reasoning
- Qualitative data interpretation
- Qualitative data analysis software
Qualitative data analysis
Analyzing qualitative data is the next step after you have completed the use of qualitative data collection methods . The qualitative analysis process aims to identify themes and patterns that emerge across the data.
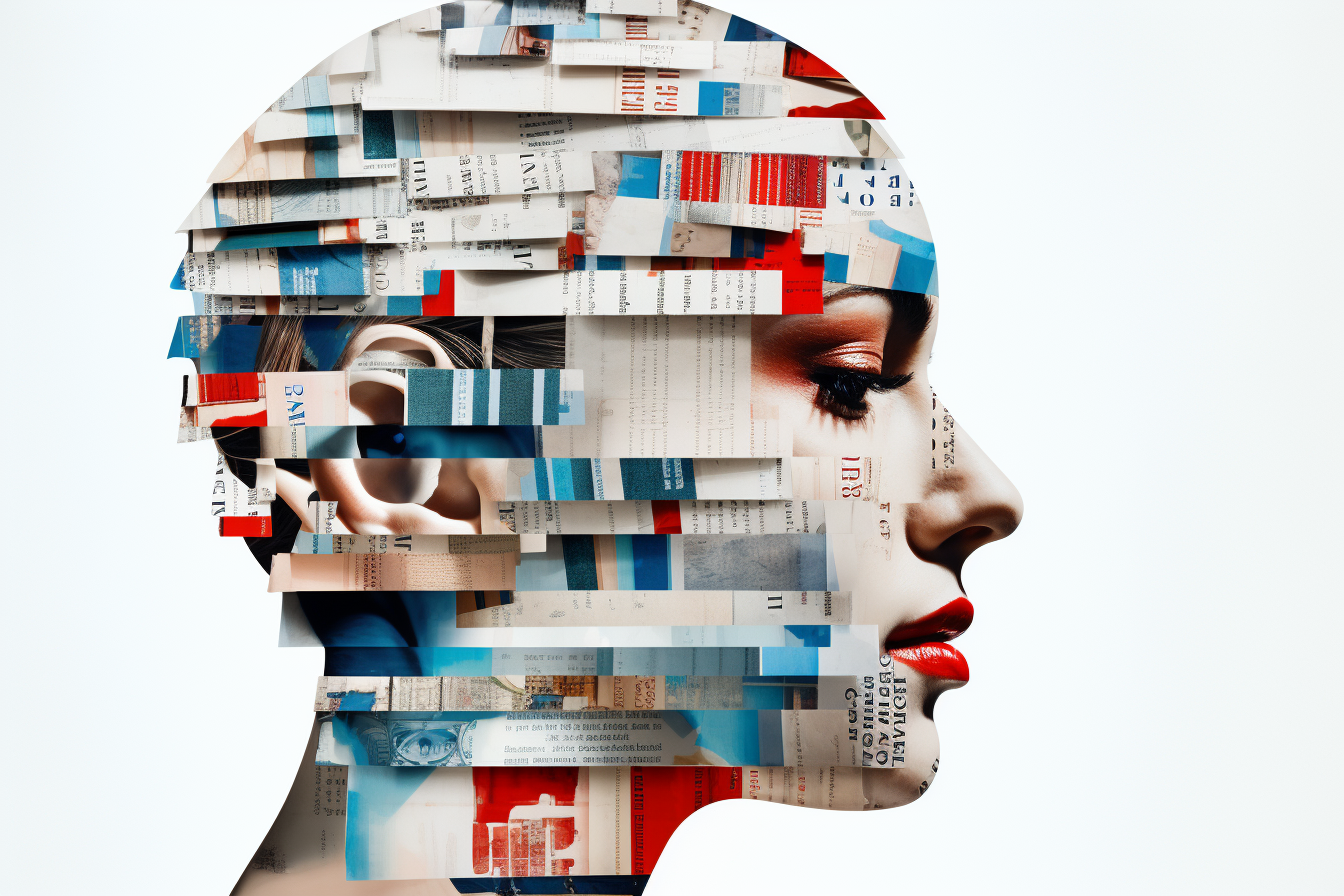
In simplified terms, qualitative research methods involve non-numerical data collection followed by an explanation based on the attributes of the data . For example, if you are asked to explain in qualitative terms a thermal image displayed in multiple colors, then you would explain the color differences rather than the heat's numerical value. If you have a large amount of data (e.g., of group discussions or observations of real-life situations), the next step is to transcribe and prepare the raw data for subsequent analysis.
Researchers can conduct studies fully based on qualitative methodology, or researchers can preface a quantitative research study with a qualitative study to identify issues that were not originally envisioned but are important to the study. Quantitative researchers may also collect and analyze qualitative data following their quantitative analyses to better understand the meanings behind their statistical results.
Conducting qualitative research can especially help build an understanding of how and why certain outcomes were achieved (in addition to what was achieved). For example, qualitative data analysis is often used for policy and program evaluation research since it can answer certain important questions more efficiently and effectively than quantitative approaches.
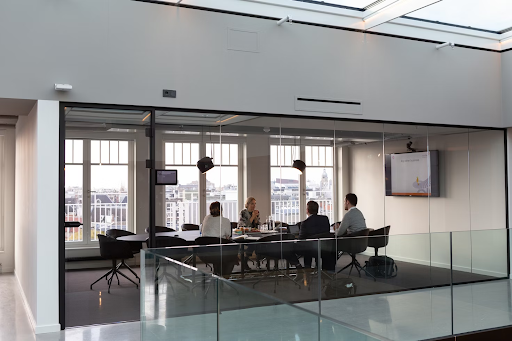
Qualitative data analysis can also answer important questions about the relevance, unintended effects, and impact of programs, such as:
- Were expectations reasonable?
- Did processes operate as expected?
- Were key players able to carry out their duties?
- Were there any unintended effects of the program?
The importance of qualitative data analysis
Qualitative approaches have the advantage of allowing for more diversity in responses and the capacity to adapt to new developments or issues during the research process itself. While qualitative data analysis can be demanding and time-consuming to conduct, many fields of research utilize qualitative software tools that have been specifically developed to provide more succinct, cost-efficient, and timely results.
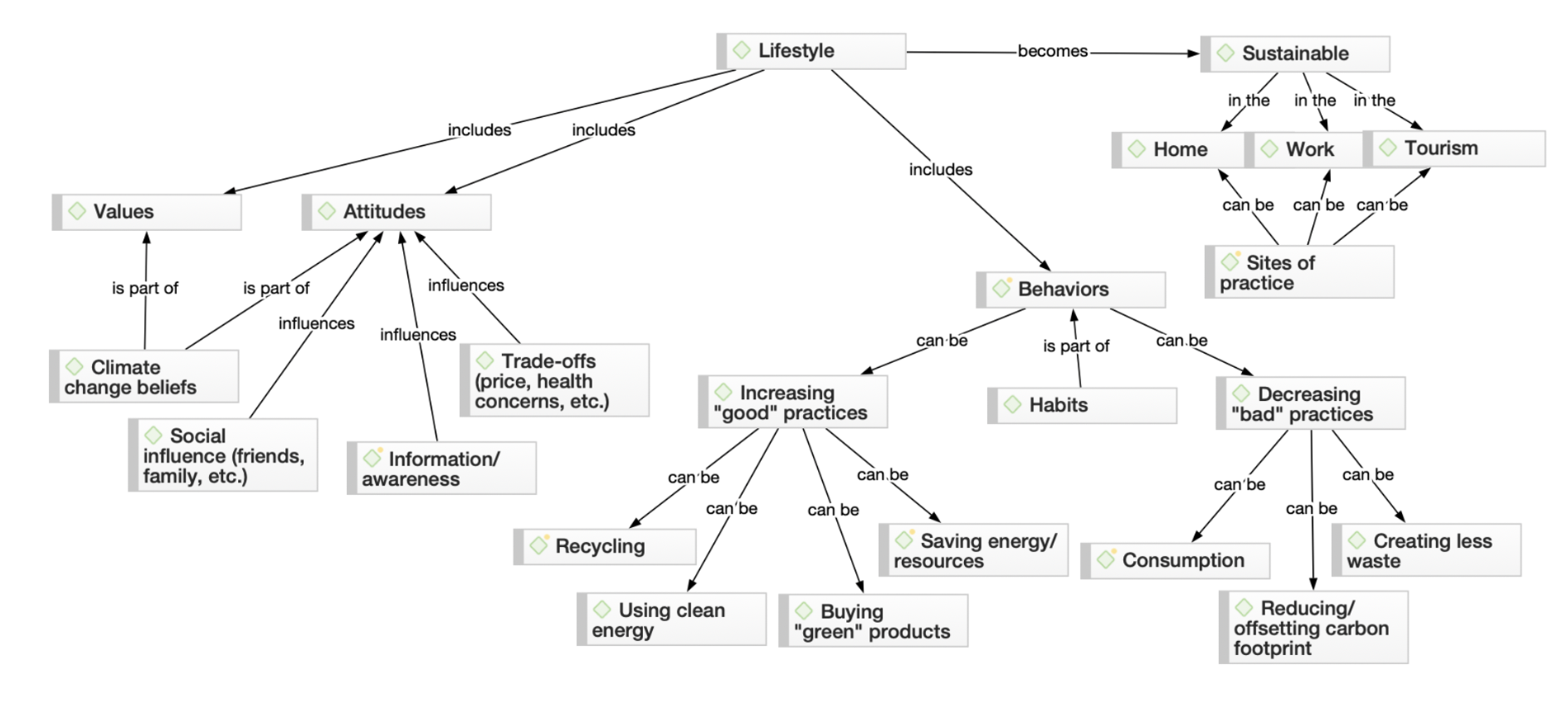
Qualitative data analysis is an important part of research and building greater understanding across fields for a number of reasons. First, cases for qualitative data analysis can be selected purposefully according to whether they typify certain characteristics or contextual locations. In other words, qualitative data permits deep immersion into a topic, phenomenon, or area of interest. Rather than seeking generalizability to the population the sample of participants represent, qualitative research aims to construct an in-depth and nuanced understanding of the research topic.
Secondly, the role or position of the researcher in qualitative data analysis is given greater critical attention. This is because, in qualitative data analysis, the possibility of the researcher taking a ‘neutral' or transcendent position is seen as more problematic in practical and/or philosophical terms. Hence, qualitative researchers are often exhorted to reflect on their role in the research process and make this clear in the analysis.
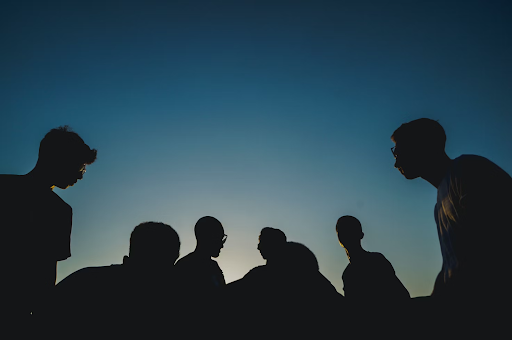
Thirdly, while qualitative data analysis can take a wide variety of forms, it largely differs from quantitative research in the focus on language, signs, experiences, and meaning. In addition, qualitative approaches to analysis are often holistic and contextual rather than analyzing the data in a piecemeal fashion or removing the data from its context. Qualitative approaches thus allow researchers to explore inquiries from directions that could not be accessed with only numerical quantitative data.
Establishing research rigor
Systematic and transparent approaches to the analysis of qualitative data are essential for rigor . For example, many qualitative research methods require researchers to carefully code data and discern and document themes in a consistent and credible way.
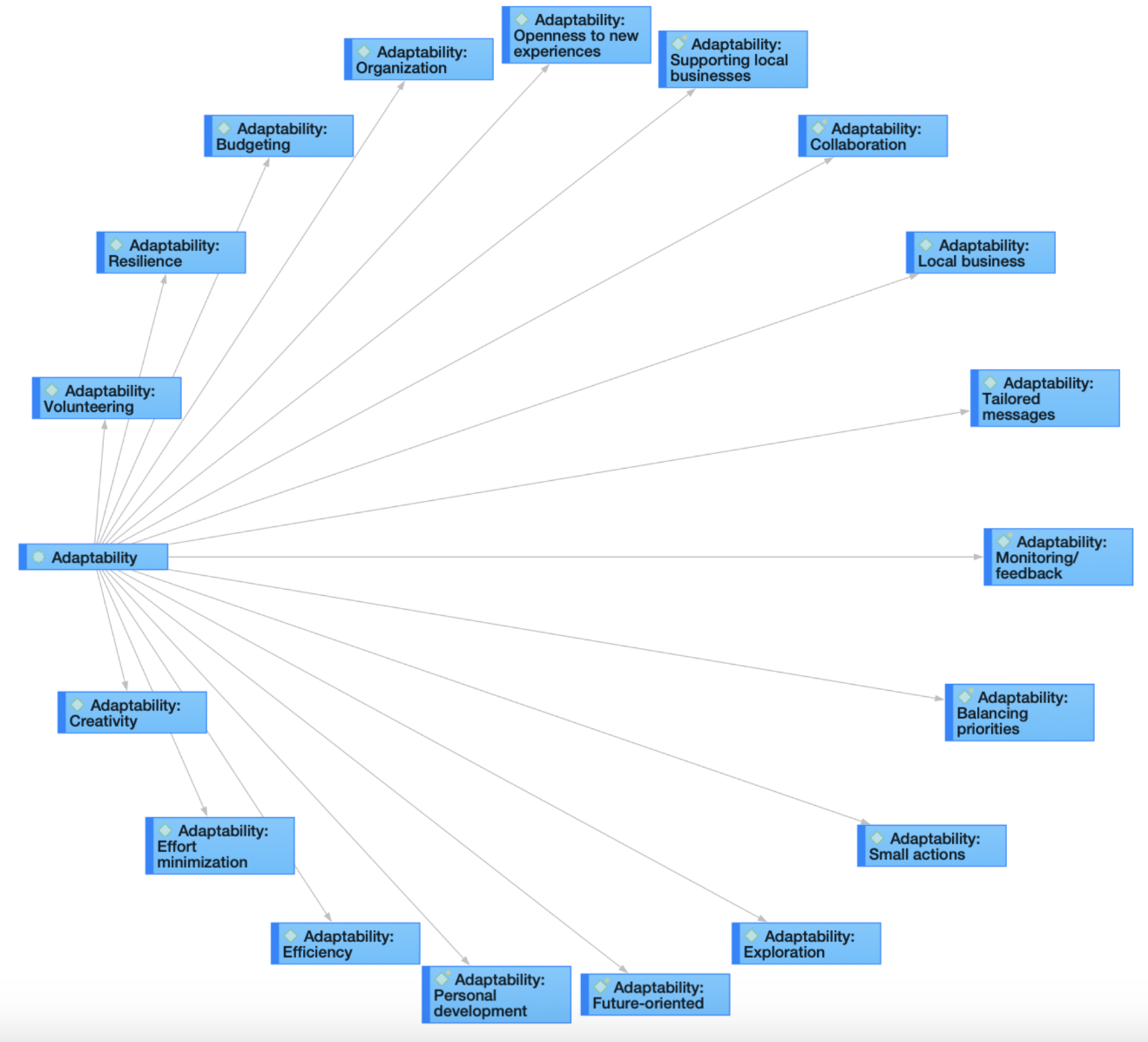
Perhaps the most traditional division in the way qualitative and quantitative research have been used in the social sciences is for qualitative methods to be used for exploratory purposes (e.g., to generate new theory or propositions) or to explain puzzling quantitative results, while quantitative methods are used to test hypotheses .
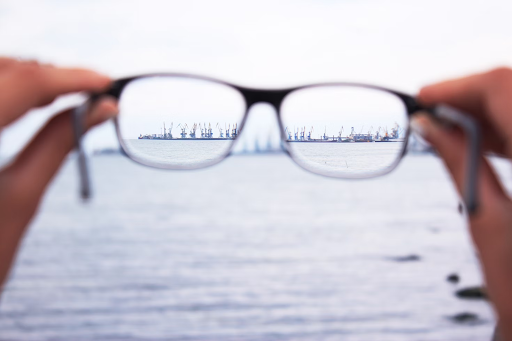
After you’ve collected relevant data , what is the best way to look at your data ? As always, it will depend on your research question . For instance, if you employed an observational research method to learn about a group’s shared practices, an ethnographic approach could be appropriate to explain the various dimensions of culture. If you collected textual data to understand how people talk about something, then a discourse analysis approach might help you generate key insights about language and communication.
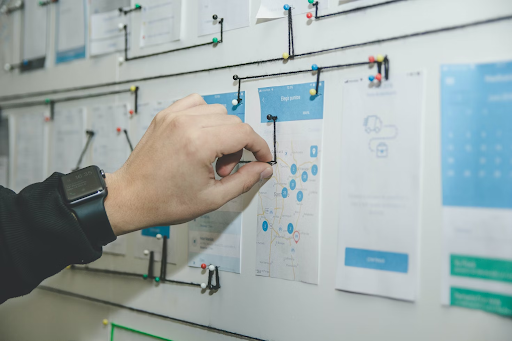
The qualitative data coding process involves iterative categorization and recategorization, ensuring the evolution of the analysis to best represent the data. The procedure typically concludes with the interpretation of patterns and trends identified through the coding process.
To start off, let’s look at two broad approaches to data analysis.
Deductive analysis
Deductive analysis is guided by pre-existing theories or ideas. It starts with a theoretical framework , which is then used to code the data. The researcher can thus use this theoretical framework to interpret their data and answer their research question .
The key steps include coding the data based on the predetermined concepts or categories and using the theory to guide the interpretation of patterns among the codings. Deductive analysis is particularly useful when researchers aim to verify or extend an existing theory within a new context.
Inductive analysis
Inductive analysis involves the generation of new theories or ideas based on the data. The process starts without any preconceived theories or codes, and patterns, themes, and categories emerge out of the data.
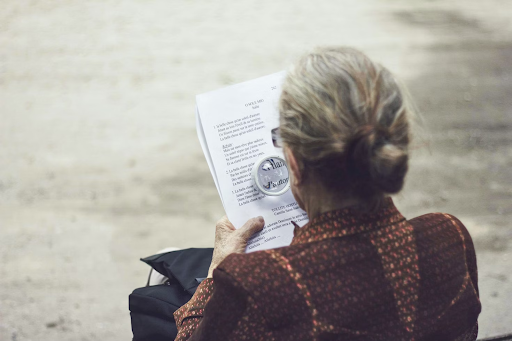
The researcher codes the data to capture any concepts or patterns that seem interesting or important to the research question . These codes are then compared and linked, leading to the formation of broader categories or themes. The main goal of inductive analysis is to allow the data to 'speak for itself' rather than imposing pre-existing expectations or ideas onto the data.
Deductive and inductive approaches can be seen as sitting on opposite poles, and all research falls somewhere within that spectrum. Most often, qualitative data analysis approaches blend both deductive and inductive elements to contribute to the existing conversation around a topic while remaining open to potential unexpected findings. To help you make informed decisions about which qualitative data analysis approach fits with your research objectives, let's look at some of the common approaches for qualitative data analysis.
Content analysis is a research method used to identify patterns and themes within qualitative data. This approach involves systematically coding and categorizing specific aspects of the content in the data to uncover trends and patterns. An often important part of content analysis is quantifying frequencies and patterns of words or characteristics present in the data .
It is a highly flexible technique that can be adapted to various data types , including text, images, and audiovisual content . While content analysis can be exploratory in nature, it is also common to use pre-established theories and follow a more deductive approach to categorizing and quantifying the qualitative data.
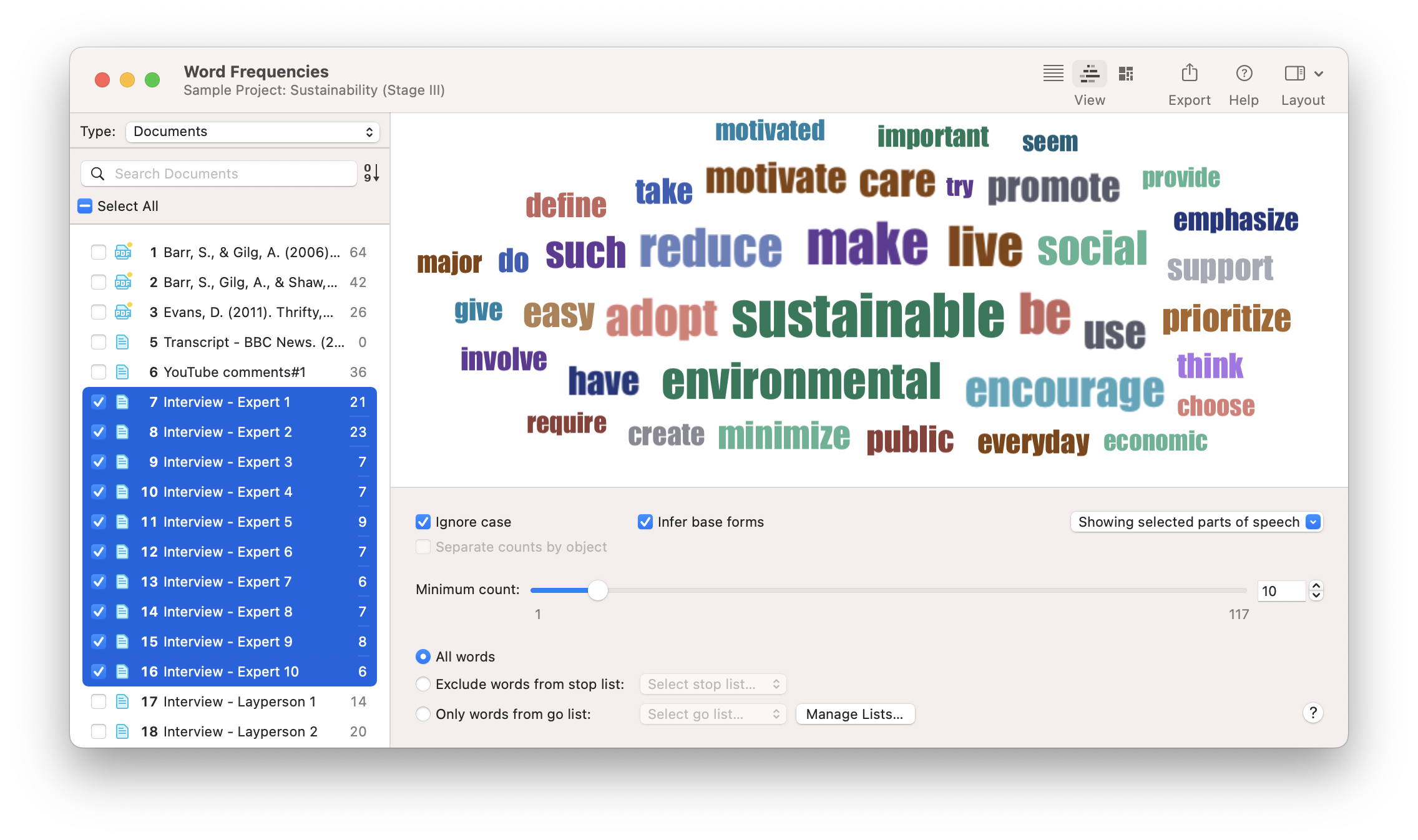
Thematic analysis is a method used to identify, analyze, and report patterns or themes within the data. This approach moves beyond counting explicit words or phrases and focuses on also identifying implicit concepts and themes within the data.
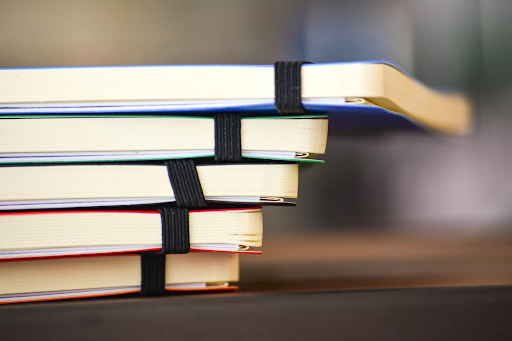
Researchers conduct detailed coding of the data to ascertain repeated themes or patterns of meaning. Codes can be categorized into themes, and the researcher can analyze how the themes relate to one another. Thematic analysis is flexible in terms of the research framework, allowing for both inductive (data-driven) and deductive (theory-driven) approaches. The outcome is a rich, detailed, and complex account of the data.
Grounded theory is a systematic qualitative research methodology that is used to inductively generate theory that is 'grounded' in the data itself. Analysis takes place simultaneously with data collection , and researchers iterate between data collection and analysis until a comprehensive theory is developed.
Grounded theory is characterized by simultaneous data collection and analysis, the development of theoretical codes from the data, purposeful sampling of participants, and the constant comparison of data with emerging categories and concepts. The ultimate goal is to create a theoretical explanation that fits the data and answers the research question .
Discourse analysis is a qualitative research approach that emphasizes the role of language in social contexts. It involves examining communication and language use beyond the level of the sentence, considering larger units of language such as texts or conversations.
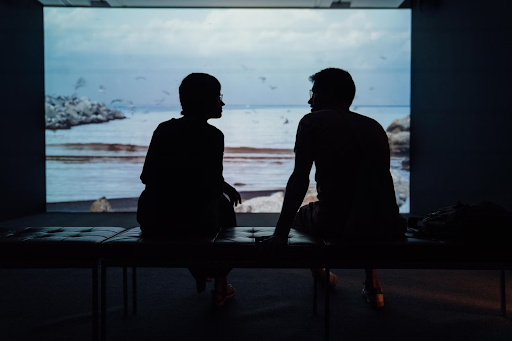
Discourse analysts typically investigate how social meanings and understandings are constructed in different contexts, emphasizing the connection between language and power. It can be applied to texts of all kinds, including interviews , documents, case studies , and social media posts.
Phenomenological research focuses on exploring how human beings make sense of an experience and delves into the essence of this experience. It strives to understand people's perceptions, perspectives, and understandings of a particular situation or phenomenon.
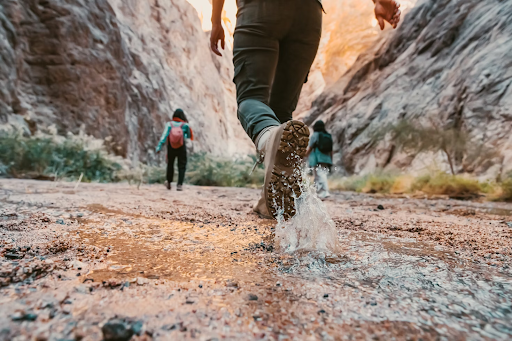
It involves in-depth engagement with participants, often through interviews or conversations, to explore their lived experiences. The goal is to derive detailed descriptions of the essence of the experience and to interpret what insights or implications this may bear on our understanding of this phenomenon.
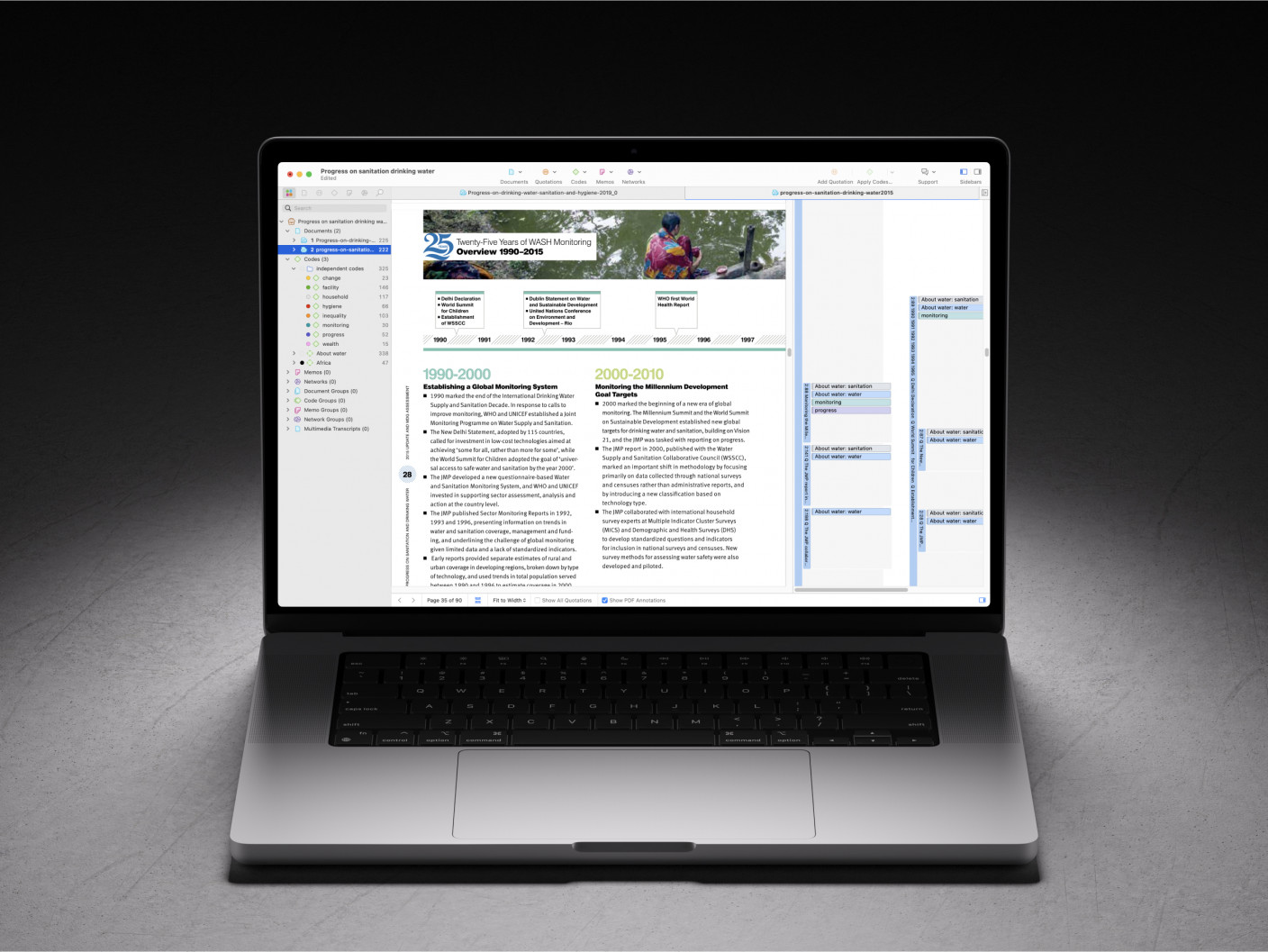
Whatever your data analysis approach, start with ATLAS.ti
Qualitative data analysis done quickly and intuitively with ATLAS.ti. Download a free trial today.
Now that we've summarized the major approaches to data analysis, let's look at the broader process of research and data analysis. Suppose you need to do some research to find answers to any kind of research question, be it an academic inquiry, business problem, or policy decision. In that case, you need to collect some data. There are many methods of collecting data: you can collect primary data yourself by conducting interviews, focus groups , or a survey , for instance. Another option is to use secondary data sources. These are data previously collected for other projects, historical records, reports, statistics – basically everything that exists already and can be relevant to your research.
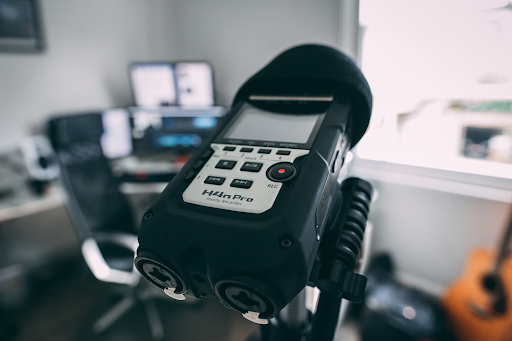
The data you collect should always be a good fit for your research question . For example, if you are interested in how many people in your target population like your brand compared to others, it is no use to conduct interviews or a few focus groups . The sample will be too small to get a representative picture of the population. If your questions are about "how many….", "what is the spread…" etc., you need to conduct quantitative research . If you are interested in why people like different brands, their motives, and their experiences, then conducting qualitative research can provide you with the answers you are looking for.
Let's describe the important steps involved in conducting research.
Step 1: Planning the research
As the saying goes: "Garbage in, garbage out." Suppose you find out after you have collected data that
- you talked to the wrong people
- asked the wrong questions
- a couple of focus groups sessions would have yielded better results because of the group interaction, or
- a survey including a few open-ended questions sent to a larger group of people would have been sufficient and required less effort.
Think thoroughly about sampling, the questions you will be asking, and in which form. If you conduct a focus group or an interview, you are the research instrument, and your data collection will only be as good as you are. If you have never done it before, seek some training and practice. If you have other people do it, make sure they have the skills.
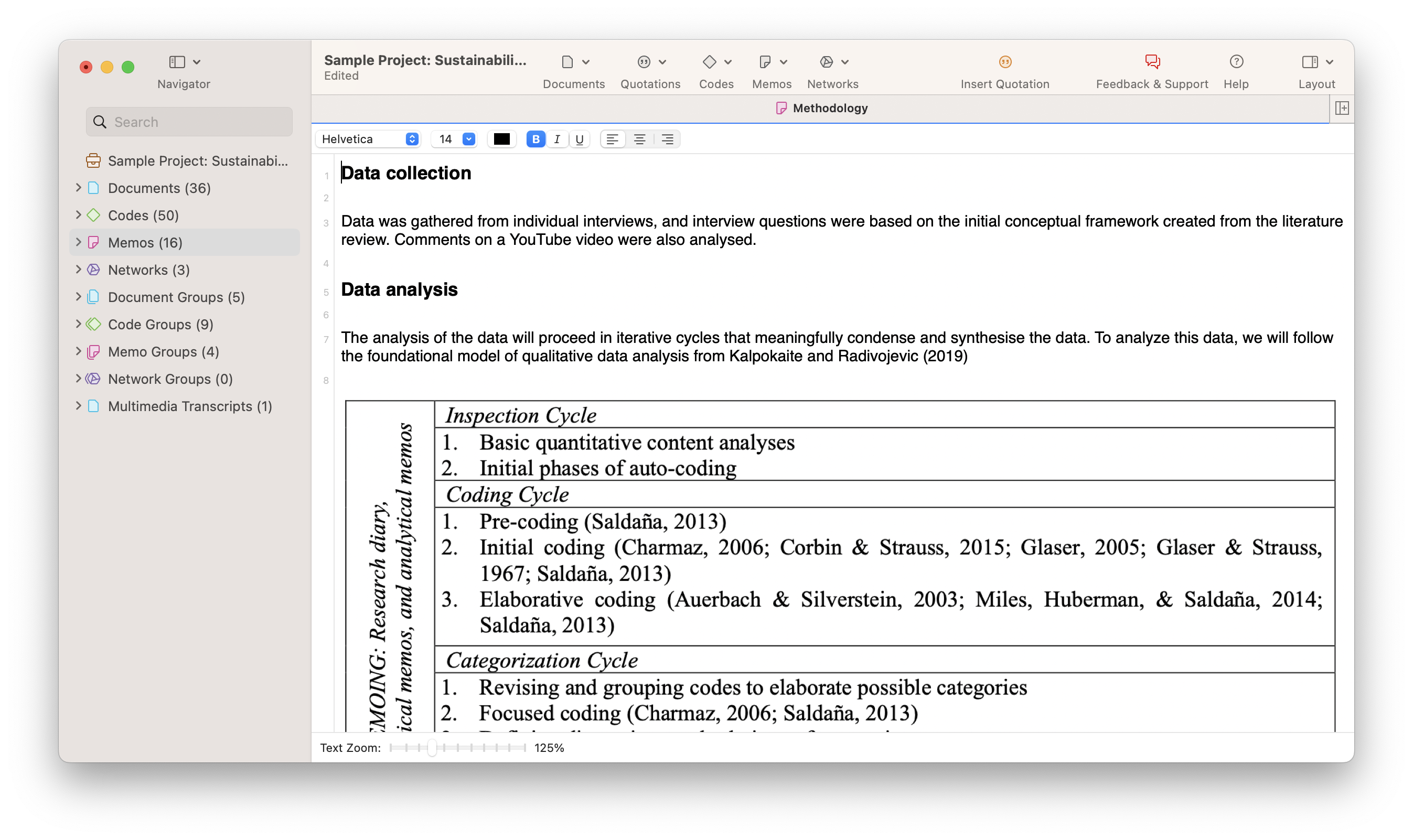
Step 2: Preparing the data
When you conduct focus groups or interviews, think about how to transcribe them. Do you want to run them online or offline? If online, check out which tools can serve your needs, both in terms of functionality and cost. For any audio or video recordings , you can consider using automatic transcription software or services. Automatically generated transcripts can save you time and money, but they still need to be checked. If you don't do this yourself, make sure that you instruct the person doing it on how to prepare the data.
- How should the final transcript be formatted for later analysis?
- Which names and locations should be anonymized?
- What kind of speaker IDs to use?
What about survey data ? Some survey data programs will immediately provide basic descriptive-level analysis of the responses. ATLAS.ti will support you with the analysis of the open-ended questions. For this, you need to export your data as an Excel file. ATLAS.ti's survey import wizard will guide you through the process.
Other kinds of data such as images, videos, audio recordings, text, and more can be imported to ATLAS.ti. You can organize all your data into groups and write comments on each source of data to maintain a systematic organization and documentation of your data.
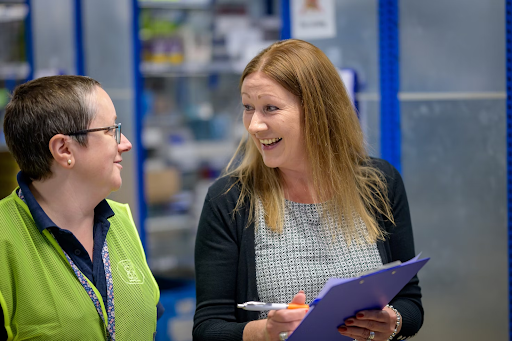
Step 3: Exploratory data analysis
You can run a few simple exploratory analyses to get to know your data. For instance, you can create a word list or word cloud of all your text data or compare and contrast the words in different documents. You can also let ATLAS.ti find relevant concepts for you. There are many tools available that can automatically code your text data, so you can also use these codings to explore your data and refine your coding.
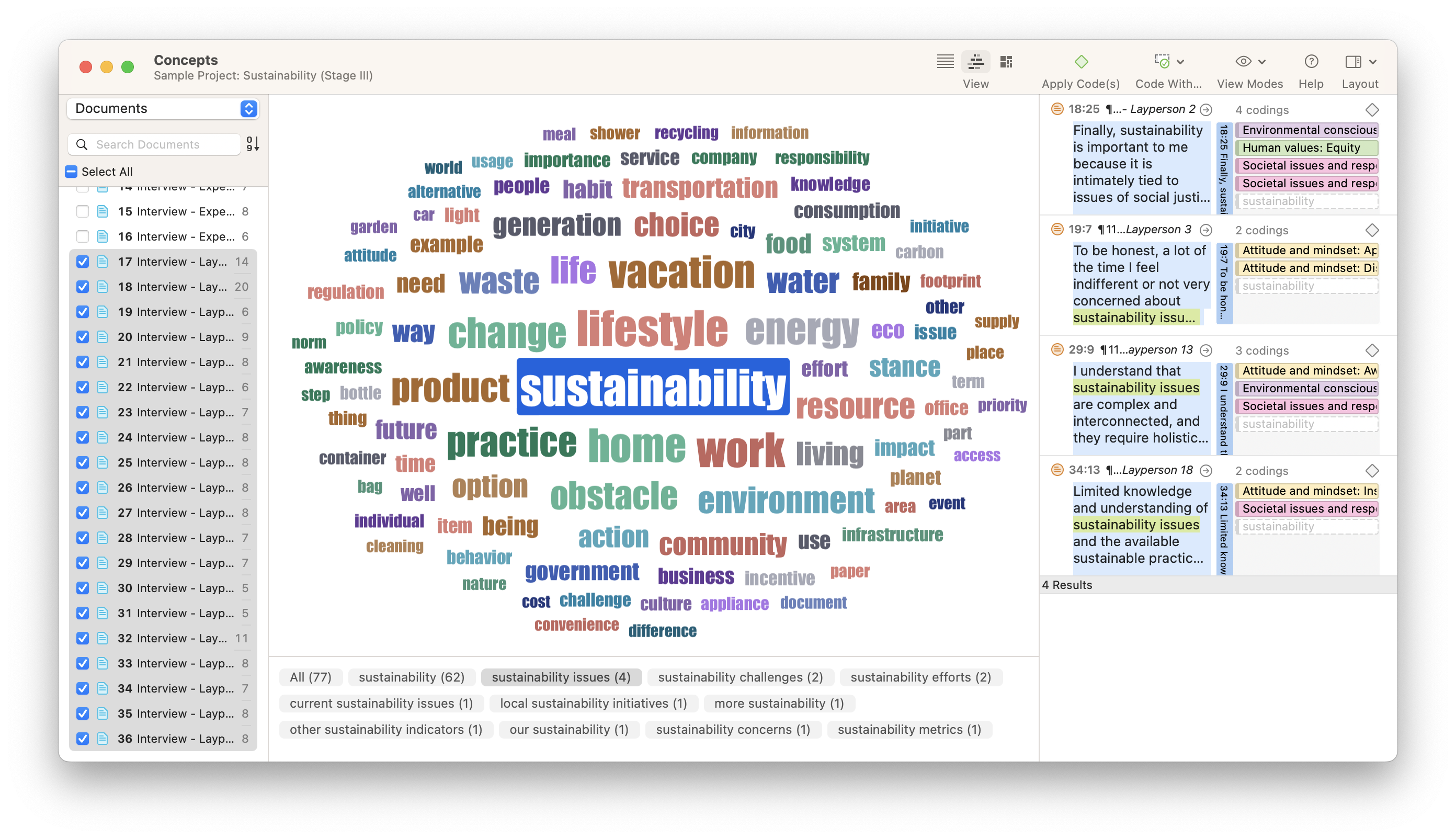
For instance, you can get a feeling for the sentiments expressed in the data. Who is more optimistic, pessimistic, or neutral in their responses? ATLAS.ti can auto-code the positive, negative, and neutral sentiments in your data. Naturally, you can also simply browse through your data and highlight relevant segments that catch your attention or attach codes to begin condensing the data.
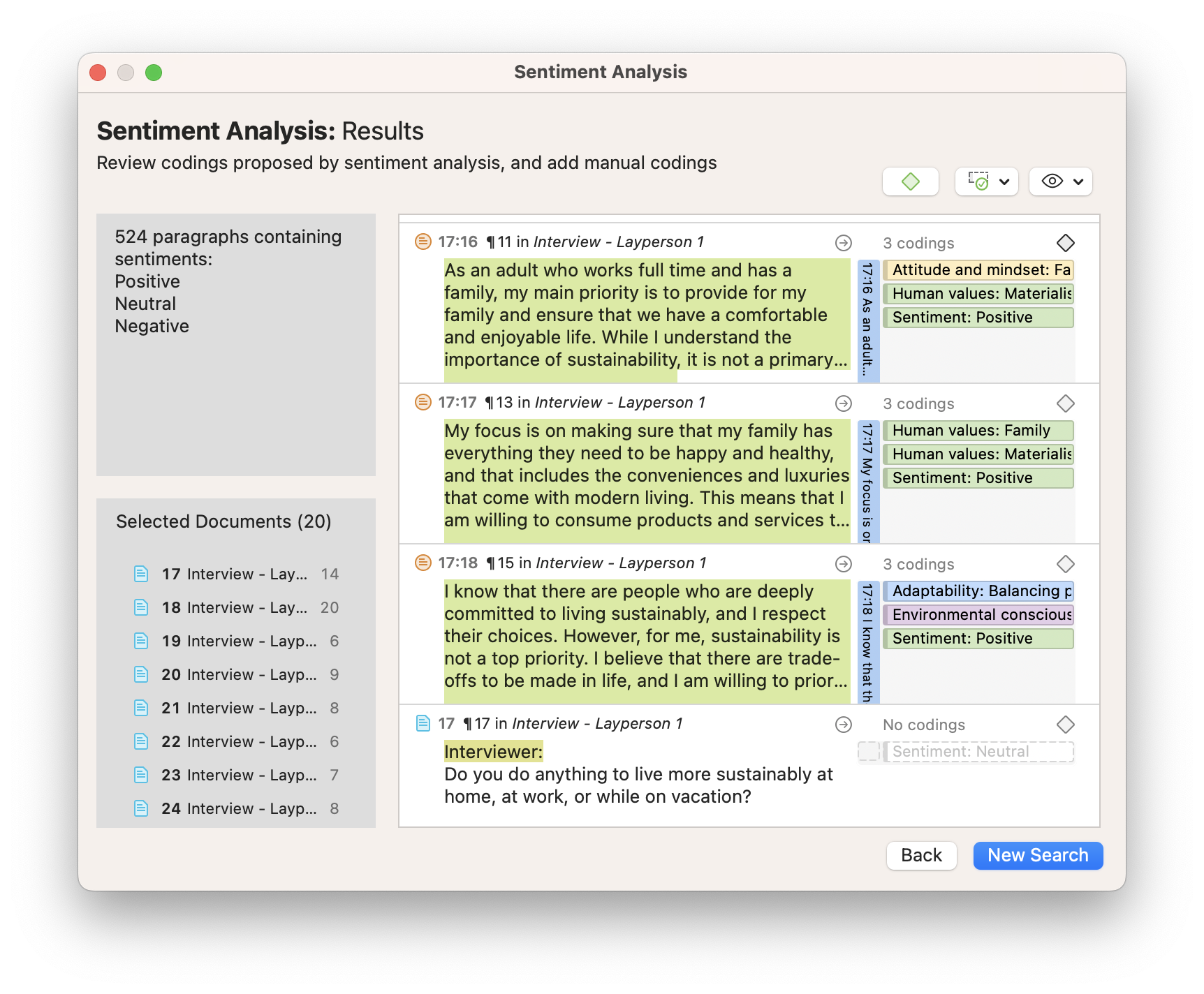
Step 4: Build a code system
Whether you start with auto-coding or manual coding, after having generated some first codes, you need to get some order in your code system to develop a cohesive understanding. You can build your code system by sorting codes into groups and creating categories and subcodes. As this process requires reading and re-reading your data, you will become very familiar with your data. Counting on a tool like ATLAS.ti qualitative data analysis software will support you in the process and make it easier to review your data, modify codings if necessary, change code labels, and write operational definitions to explain what each code means.
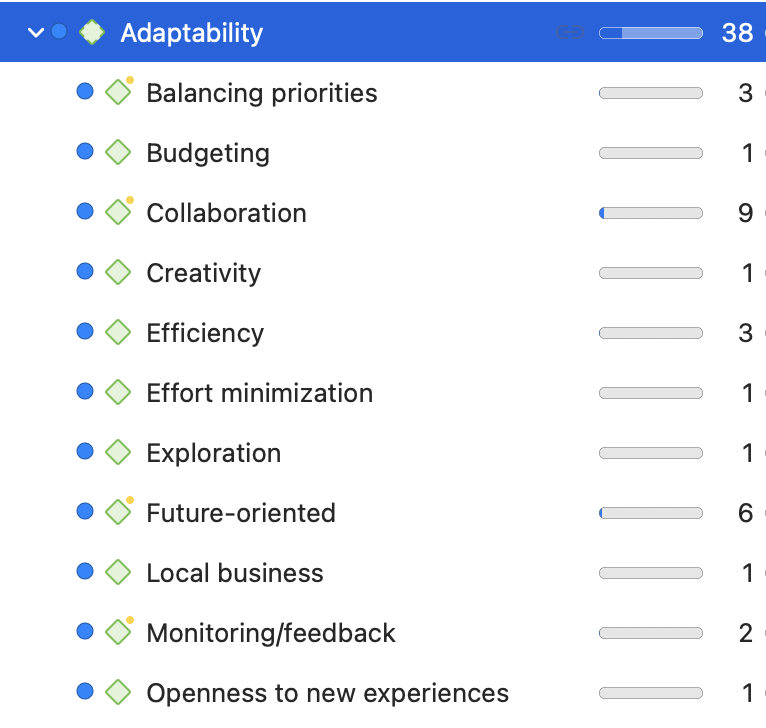
Step 5: Query your coded data and write up the analysis
Once you have coded your data, it is time to take the analysis a step further. When using software for qualitative data analysis , it is easy to compare and contrast subsets in your data, such as groups of participants or sets of themes.
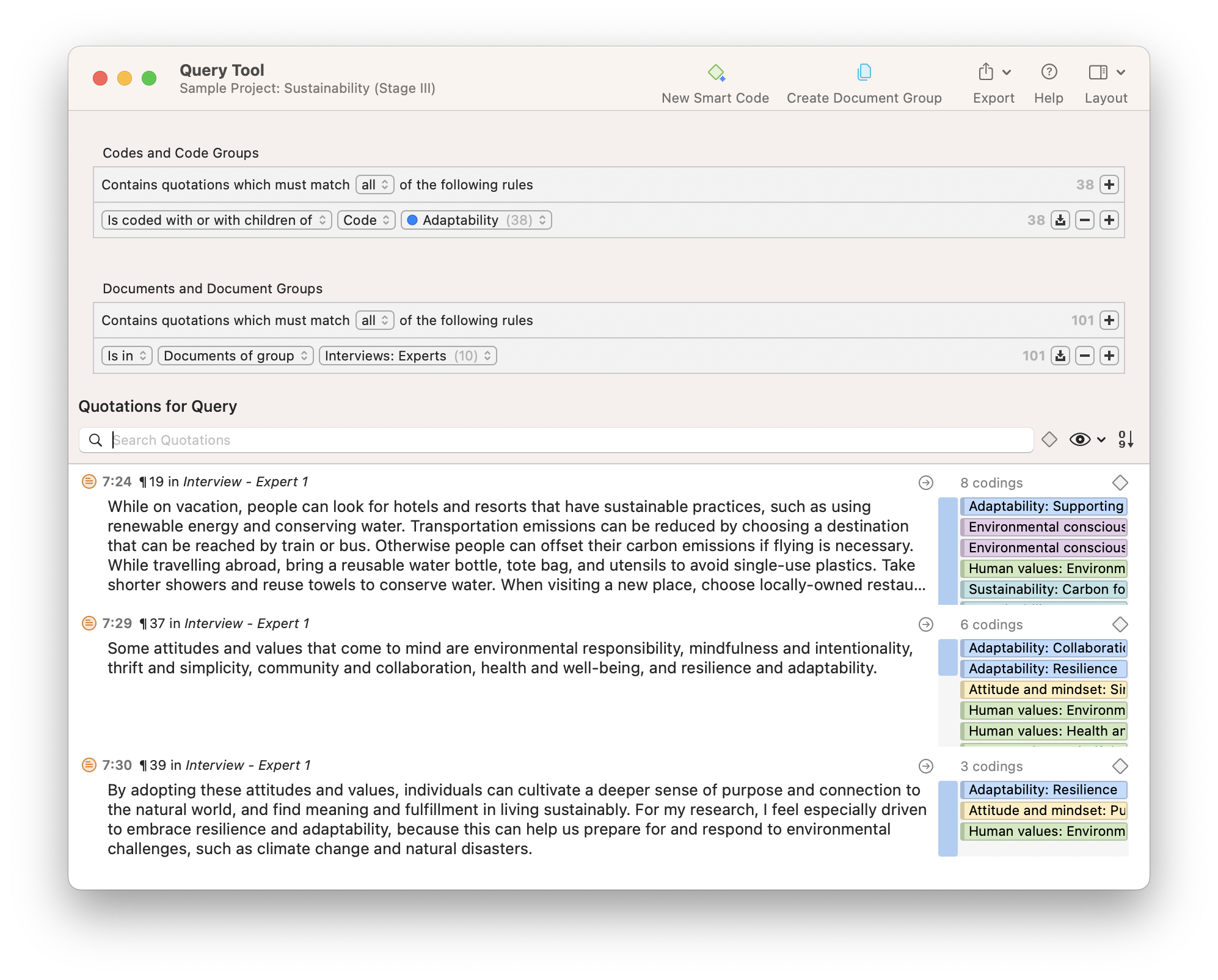
For instance, you can query the various opinions of female vs. male respondents. Is there a difference between consumers from rural or urban areas or among different age groups or educational levels? Which codes occur together throughout the data set? Are there relationships between various concepts, and if so, why?
Step 6: Data visualization
Data visualization brings your data to life. It is a powerful way of seeing patterns and relationships in your data. For instance, diagrams allow you to see how your codes are distributed across documents or specific subpopulations in your data.
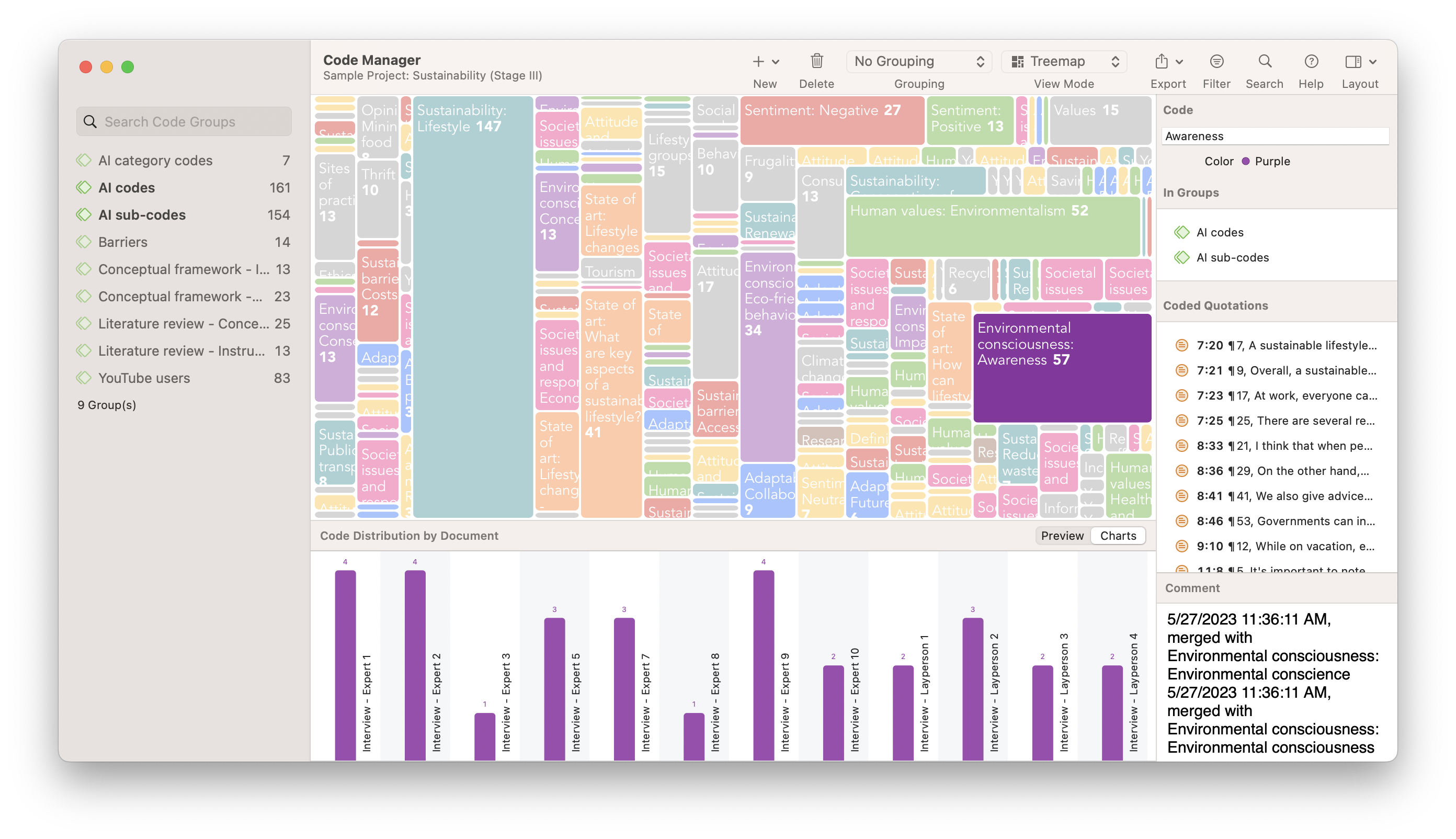
Exploring coded data on a canvas, moving around code labels in a virtual space, linking codes and other elements of your data set, and thinking about how they are related and why – all of these will advance your analysis and spur further insights. Visuals are also great for communicating results to others.
Step 7: Data presentation
The final step is to summarize the analysis in a written report . You can now put together the memos you have written about the various topics, select some salient quotes that illustrate your writing, and add visuals such as tables and diagrams. If you follow the steps above, you will already have all the building blocks, and you just have to put them together in a report or presentation.
When preparing a report or a presentation, keep your audience in mind. Does your audience better understand numbers than long sections of detailed interpretations? If so, add more tables, charts, and short supportive data quotes to your report or presentation. If your audience loves a good interpretation, add your full-length memos and walk your audience through your conceptual networks and illustrative data quotes.
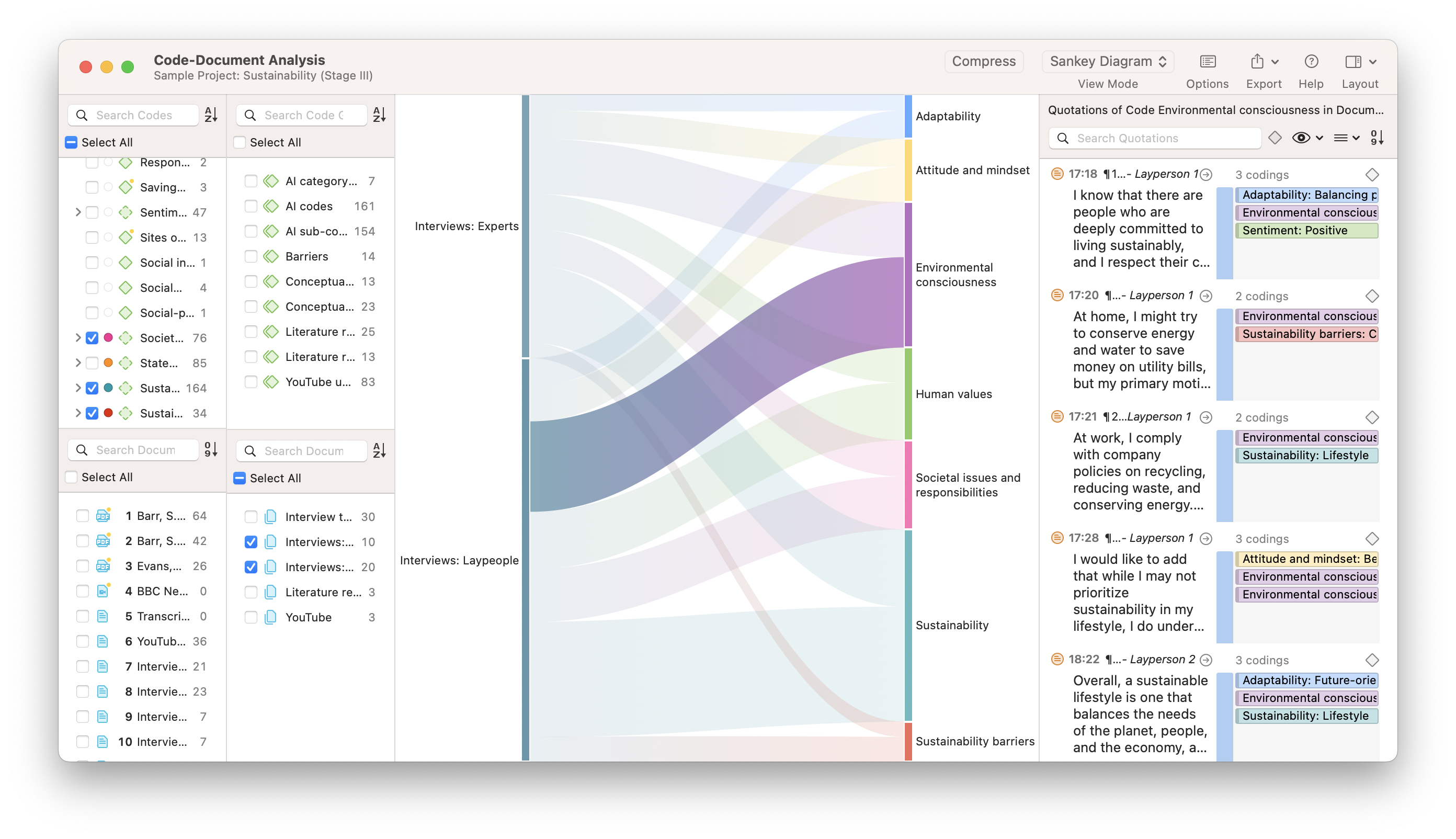
Qualitative data analysis begins with ATLAS.ti
For tools that can make the most out of your data, check out ATLAS.ti with a free trial.
- Search Menu
Sign in through your institution
- Browse content in Arts and Humanities
- Browse content in Archaeology
- Anglo-Saxon and Medieval Archaeology
- Archaeological Methodology and Techniques
- Archaeology by Region
- Archaeology of Religion
- Archaeology of Trade and Exchange
- Biblical Archaeology
- Contemporary and Public Archaeology
- Environmental Archaeology
- Historical Archaeology
- History and Theory of Archaeology
- Industrial Archaeology
- Landscape Archaeology
- Mortuary Archaeology
- Prehistoric Archaeology
- Underwater Archaeology
- Urban Archaeology
- Zooarchaeology
- Browse content in Architecture
- Architectural Structure and Design
- History of Architecture
- Residential and Domestic Buildings
- Theory of Architecture
- Browse content in Art
- Art Subjects and Themes
- History of Art
- Industrial and Commercial Art
- Theory of Art
- Biographical Studies
- Byzantine Studies
- Browse content in Classical Studies
- Classical History
- Classical Philosophy
- Classical Mythology
- Classical Numismatics
- Classical Literature
- Classical Reception
- Classical Art and Architecture
- Classical Oratory and Rhetoric
- Greek and Roman Papyrology
- Greek and Roman Epigraphy
- Greek and Roman Law
- Greek and Roman Archaeology
- Late Antiquity
- Religion in the Ancient World
- Social History
- Digital Humanities
- Browse content in History
- Colonialism and Imperialism
- Diplomatic History
- Environmental History
- Genealogy, Heraldry, Names, and Honours
- Genocide and Ethnic Cleansing
- Historical Geography
- History by Period
- History of Emotions
- History of Agriculture
- History of Education
- History of Gender and Sexuality
- Industrial History
- Intellectual History
- International History
- Labour History
- Legal and Constitutional History
- Local and Family History
- Maritime History
- Military History
- National Liberation and Post-Colonialism
- Oral History
- Political History
- Public History
- Regional and National History
- Revolutions and Rebellions
- Slavery and Abolition of Slavery
- Social and Cultural History
- Theory, Methods, and Historiography
- Urban History
- World History
- Browse content in Language Teaching and Learning
- Language Learning (Specific Skills)
- Language Teaching Theory and Methods
- Browse content in Linguistics
- Applied Linguistics
- Cognitive Linguistics
- Computational Linguistics
- Forensic Linguistics
- Grammar, Syntax and Morphology
- Historical and Diachronic Linguistics
- History of English
- Language Evolution
- Language Reference
- Language Acquisition
- Language Variation
- Language Families
- Lexicography
- Linguistic Anthropology
- Linguistic Theories
- Linguistic Typology
- Phonetics and Phonology
- Psycholinguistics
- Sociolinguistics
- Translation and Interpretation
- Writing Systems
- Browse content in Literature
- Bibliography
- Children's Literature Studies
- Literary Studies (Romanticism)
- Literary Studies (American)
- Literary Studies (Asian)
- Literary Studies (European)
- Literary Studies (Eco-criticism)
- Literary Studies (Modernism)
- Literary Studies - World
- Literary Studies (1500 to 1800)
- Literary Studies (19th Century)
- Literary Studies (20th Century onwards)
- Literary Studies (African American Literature)
- Literary Studies (British and Irish)
- Literary Studies (Early and Medieval)
- Literary Studies (Fiction, Novelists, and Prose Writers)
- Literary Studies (Gender Studies)
- Literary Studies (Graphic Novels)
- Literary Studies (History of the Book)
- Literary Studies (Plays and Playwrights)
- Literary Studies (Poetry and Poets)
- Literary Studies (Postcolonial Literature)
- Literary Studies (Queer Studies)
- Literary Studies (Science Fiction)
- Literary Studies (Travel Literature)
- Literary Studies (War Literature)
- Literary Studies (Women's Writing)
- Literary Theory and Cultural Studies
- Mythology and Folklore
- Shakespeare Studies and Criticism
- Browse content in Media Studies
- Browse content in Music
- Applied Music
- Dance and Music
- Ethics in Music
- Ethnomusicology
- Gender and Sexuality in Music
- Medicine and Music
- Music Cultures
- Music and Media
- Music and Religion
- Music and Culture
- Music Education and Pedagogy
- Music Theory and Analysis
- Musical Scores, Lyrics, and Libretti
- Musical Structures, Styles, and Techniques
- Musicology and Music History
- Performance Practice and Studies
- Race and Ethnicity in Music
- Sound Studies
- Browse content in Performing Arts
- Browse content in Philosophy
- Aesthetics and Philosophy of Art
- Epistemology
- Feminist Philosophy
- History of Western Philosophy
- Meta-Philosophy
- Metaphysics
- Moral Philosophy
- Non-Western Philosophy
- Philosophy of Language
- Philosophy of Mind
- Philosophy of Perception
- Philosophy of Science
- Philosophy of Action
- Philosophy of Law
- Philosophy of Religion
- Philosophy of Mathematics and Logic
- Practical Ethics
- Social and Political Philosophy
- Browse content in Religion
- Biblical Studies
- Christianity
- East Asian Religions
- History of Religion
- Judaism and Jewish Studies
- Qumran Studies
- Religion and Education
- Religion and Health
- Religion and Politics
- Religion and Science
- Religion and Law
- Religion and Art, Literature, and Music
- Religious Studies
- Browse content in Society and Culture
- Cookery, Food, and Drink
- Cultural Studies
- Customs and Traditions
- Ethical Issues and Debates
- Hobbies, Games, Arts and Crafts
- Natural world, Country Life, and Pets
- Popular Beliefs and Controversial Knowledge
- Sports and Outdoor Recreation
- Technology and Society
- Travel and Holiday
- Visual Culture
- Browse content in Law
- Arbitration
- Browse content in Company and Commercial Law
- Commercial Law
- Company Law
- Browse content in Comparative Law
- Systems of Law
- Competition Law
- Browse content in Constitutional and Administrative Law
- Government Powers
- Judicial Review
- Local Government Law
- Military and Defence Law
- Parliamentary and Legislative Practice
- Construction Law
- Contract Law
- Browse content in Criminal Law
- Criminal Procedure
- Criminal Evidence Law
- Sentencing and Punishment
- Employment and Labour Law
- Environment and Energy Law
- Browse content in Financial Law
- Banking Law
- Insolvency Law
- History of Law
- Human Rights and Immigration
- Intellectual Property Law
- Browse content in International Law
- Private International Law and Conflict of Laws
- Public International Law
- IT and Communications Law
- Jurisprudence and Philosophy of Law
- Law and Politics
- Law and Society
- Browse content in Legal System and Practice
- Courts and Procedure
- Legal Skills and Practice
- Legal System - Costs and Funding
- Primary Sources of Law
- Regulation of Legal Profession
- Medical and Healthcare Law
- Browse content in Policing
- Criminal Investigation and Detection
- Police and Security Services
- Police Procedure and Law
- Police Regional Planning
- Browse content in Property Law
- Personal Property Law
- Restitution
- Study and Revision
- Terrorism and National Security Law
- Browse content in Trusts Law
- Wills and Probate or Succession
- Browse content in Medicine and Health
- Browse content in Allied Health Professions
- Arts Therapies
- Clinical Science
- Dietetics and Nutrition
- Occupational Therapy
- Operating Department Practice
- Physiotherapy
- Radiography
- Speech and Language Therapy
- Browse content in Anaesthetics
- General Anaesthesia
- Clinical Neuroscience
- Browse content in Clinical Medicine
- Acute Medicine
- Cardiovascular Medicine
- Clinical Genetics
- Clinical Pharmacology and Therapeutics
- Dermatology
- Endocrinology and Diabetes
- Gastroenterology
- Genito-urinary Medicine
- Geriatric Medicine
- Infectious Diseases
- Medical Toxicology
- Medical Oncology
- Pain Medicine
- Palliative Medicine
- Rehabilitation Medicine
- Respiratory Medicine and Pulmonology
- Rheumatology
- Sleep Medicine
- Sports and Exercise Medicine
- Community Medical Services
- Critical Care
- Emergency Medicine
- Forensic Medicine
- Haematology
- History of Medicine
- Browse content in Medical Skills
- Clinical Skills
- Communication Skills
- Nursing Skills
- Surgical Skills
- Browse content in Medical Dentistry
- Oral and Maxillofacial Surgery
- Paediatric Dentistry
- Restorative Dentistry and Orthodontics
- Surgical Dentistry
- Medical Ethics
- Medical Statistics and Methodology
- Browse content in Neurology
- Clinical Neurophysiology
- Neuropathology
- Nursing Studies
- Browse content in Obstetrics and Gynaecology
- Gynaecology
- Occupational Medicine
- Ophthalmology
- Otolaryngology (ENT)
- Browse content in Paediatrics
- Neonatology
- Browse content in Pathology
- Chemical Pathology
- Clinical Cytogenetics and Molecular Genetics
- Histopathology
- Medical Microbiology and Virology
- Patient Education and Information
- Browse content in Pharmacology
- Psychopharmacology
- Browse content in Popular Health
- Caring for Others
- Complementary and Alternative Medicine
- Self-help and Personal Development
- Browse content in Preclinical Medicine
- Cell Biology
- Molecular Biology and Genetics
- Reproduction, Growth and Development
- Primary Care
- Professional Development in Medicine
- Browse content in Psychiatry
- Addiction Medicine
- Child and Adolescent Psychiatry
- Forensic Psychiatry
- Learning Disabilities
- Old Age Psychiatry
- Psychotherapy
- Browse content in Public Health and Epidemiology
- Epidemiology
- Public Health
- Browse content in Radiology
- Clinical Radiology
- Interventional Radiology
- Nuclear Medicine
- Radiation Oncology
- Reproductive Medicine
- Browse content in Surgery
- Cardiothoracic Surgery
- Gastro-intestinal and Colorectal Surgery
- General Surgery
- Neurosurgery
- Paediatric Surgery
- Peri-operative Care
- Plastic and Reconstructive Surgery
- Surgical Oncology
- Transplant Surgery
- Trauma and Orthopaedic Surgery
- Vascular Surgery
- Browse content in Science and Mathematics
- Browse content in Biological Sciences
- Aquatic Biology
- Biochemistry
- Bioinformatics and Computational Biology
- Developmental Biology
- Ecology and Conservation
- Evolutionary Biology
- Genetics and Genomics
- Microbiology
- Molecular and Cell Biology
- Natural History
- Plant Sciences and Forestry
- Research Methods in Life Sciences
- Structural Biology
- Systems Biology
- Zoology and Animal Sciences
- Browse content in Chemistry
- Analytical Chemistry
- Computational Chemistry
- Crystallography
- Environmental Chemistry
- Industrial Chemistry
- Inorganic Chemistry
- Materials Chemistry
- Medicinal Chemistry
- Mineralogy and Gems
- Organic Chemistry
- Physical Chemistry
- Polymer Chemistry
- Study and Communication Skills in Chemistry
- Theoretical Chemistry
- Browse content in Computer Science
- Artificial Intelligence
- Computer Architecture and Logic Design
- Game Studies
- Human-Computer Interaction
- Mathematical Theory of Computation
- Programming Languages
- Software Engineering
- Systems Analysis and Design
- Virtual Reality
- Browse content in Computing
- Business Applications
- Computer Security
- Computer Games
- Computer Networking and Communications
- Digital Lifestyle
- Graphical and Digital Media Applications
- Operating Systems
- Browse content in Earth Sciences and Geography
- Atmospheric Sciences
- Environmental Geography
- Geology and the Lithosphere
- Maps and Map-making
- Meteorology and Climatology
- Oceanography and Hydrology
- Palaeontology
- Physical Geography and Topography
- Regional Geography
- Soil Science
- Urban Geography
- Browse content in Engineering and Technology
- Agriculture and Farming
- Biological Engineering
- Civil Engineering, Surveying, and Building
- Electronics and Communications Engineering
- Energy Technology
- Engineering (General)
- Environmental Science, Engineering, and Technology
- History of Engineering and Technology
- Mechanical Engineering and Materials
- Technology of Industrial Chemistry
- Transport Technology and Trades
- Browse content in Environmental Science
- Applied Ecology (Environmental Science)
- Conservation of the Environment (Environmental Science)
- Environmental Sustainability
- Environmentalist Thought and Ideology (Environmental Science)
- Management of Land and Natural Resources (Environmental Science)
- Natural Disasters (Environmental Science)
- Nuclear Issues (Environmental Science)
- Pollution and Threats to the Environment (Environmental Science)
- Social Impact of Environmental Issues (Environmental Science)
- History of Science and Technology
- Browse content in Materials Science
- Ceramics and Glasses
- Composite Materials
- Metals, Alloying, and Corrosion
- Nanotechnology
- Browse content in Mathematics
- Applied Mathematics
- Biomathematics and Statistics
- History of Mathematics
- Mathematical Education
- Mathematical Finance
- Mathematical Analysis
- Numerical and Computational Mathematics
- Probability and Statistics
- Pure Mathematics
- Browse content in Neuroscience
- Cognition and Behavioural Neuroscience
- Development of the Nervous System
- Disorders of the Nervous System
- History of Neuroscience
- Invertebrate Neurobiology
- Molecular and Cellular Systems
- Neuroendocrinology and Autonomic Nervous System
- Neuroscientific Techniques
- Sensory and Motor Systems
- Browse content in Physics
- Astronomy and Astrophysics
- Atomic, Molecular, and Optical Physics
- Biological and Medical Physics
- Classical Mechanics
- Computational Physics
- Condensed Matter Physics
- Electromagnetism, Optics, and Acoustics
- History of Physics
- Mathematical and Statistical Physics
- Measurement Science
- Nuclear Physics
- Particles and Fields
- Plasma Physics
- Quantum Physics
- Relativity and Gravitation
- Semiconductor and Mesoscopic Physics
- Browse content in Psychology
- Affective Sciences
- Clinical Psychology
- Cognitive Psychology
- Cognitive Neuroscience
- Criminal and Forensic Psychology
- Developmental Psychology
- Educational Psychology
- Evolutionary Psychology
- Health Psychology
- History and Systems in Psychology
- Music Psychology
- Neuropsychology
- Organizational Psychology
- Psychological Assessment and Testing
- Psychology of Human-Technology Interaction
- Psychology Professional Development and Training
- Research Methods in Psychology
- Social Psychology
- Browse content in Social Sciences
- Browse content in Anthropology
- Anthropology of Religion
- Human Evolution
- Medical Anthropology
- Physical Anthropology
- Regional Anthropology
- Social and Cultural Anthropology
- Theory and Practice of Anthropology
- Browse content in Business and Management
- Business Ethics
- Business Strategy
- Business History
- Business and Technology
- Business and Government
- Business and the Environment
- Comparative Management
- Corporate Governance
- Corporate Social Responsibility
- Entrepreneurship
- Health Management
- Human Resource Management
- Industrial and Employment Relations
- Industry Studies
- Information and Communication Technologies
- International Business
- Knowledge Management
- Management and Management Techniques
- Operations Management
- Organizational Theory and Behaviour
- Pensions and Pension Management
- Public and Nonprofit Management
- Social Issues in Business and Management
- Strategic Management
- Supply Chain Management
- Browse content in Criminology and Criminal Justice
- Criminal Justice
- Criminology
- Forms of Crime
- International and Comparative Criminology
- Youth Violence and Juvenile Justice
- Development Studies
- Browse content in Economics
- Agricultural, Environmental, and Natural Resource Economics
- Asian Economics
- Behavioural Finance
- Behavioural Economics and Neuroeconomics
- Econometrics and Mathematical Economics
- Economic History
- Economic Systems
- Economic Methodology
- Economic Development and Growth
- Financial Markets
- Financial Institutions and Services
- General Economics and Teaching
- Health, Education, and Welfare
- History of Economic Thought
- International Economics
- Labour and Demographic Economics
- Law and Economics
- Macroeconomics and Monetary Economics
- Microeconomics
- Public Economics
- Urban, Rural, and Regional Economics
- Welfare Economics
- Browse content in Education
- Adult Education and Continuous Learning
- Care and Counselling of Students
- Early Childhood and Elementary Education
- Educational Equipment and Technology
- Educational Strategies and Policy
- Higher and Further Education
- Organization and Management of Education
- Philosophy and Theory of Education
- Schools Studies
- Secondary Education
- Teaching of a Specific Subject
- Teaching of Specific Groups and Special Educational Needs
- Teaching Skills and Techniques
- Browse content in Environment
- Applied Ecology (Social Science)
- Climate Change
- Conservation of the Environment (Social Science)
- Environmentalist Thought and Ideology (Social Science)
- Management of Land and Natural Resources (Social Science)
- Natural Disasters (Environment)
- Pollution and Threats to the Environment (Social Science)
- Social Impact of Environmental Issues (Social Science)
- Sustainability
- Browse content in Human Geography
- Cultural Geography
- Economic Geography
- Political Geography
- Browse content in Interdisciplinary Studies
- Communication Studies
- Museums, Libraries, and Information Sciences
- Browse content in Politics
- African Politics
- Asian Politics
- Chinese Politics
- Comparative Politics
- Conflict Politics
- Elections and Electoral Studies
- Environmental Politics
- Ethnic Politics
- European Union
- Foreign Policy
- Gender and Politics
- Human Rights and Politics
- Indian Politics
- International Relations
- International Organization (Politics)
- Irish Politics
- Latin American Politics
- Middle Eastern Politics
- Political Behaviour
- Political Economy
- Political Institutions
- Political Methodology
- Political Communication
- Political Philosophy
- Political Sociology
- Political Theory
- Politics and Law
- Politics of Development
- Public Policy
- Public Administration
- Qualitative Political Methodology
- Quantitative Political Methodology
- Regional Political Studies
- Russian Politics
- Security Studies
- State and Local Government
- UK Politics
- US Politics
- Browse content in Regional and Area Studies
- African Studies
- Asian Studies
- East Asian Studies
- Japanese Studies
- Latin American Studies
- Middle Eastern Studies
- Native American Studies
- Scottish Studies
- Browse content in Research and Information
- Research Methods
- Browse content in Social Work
- Addictions and Substance Misuse
- Adoption and Fostering
- Care of the Elderly
- Child and Adolescent Social Work
- Couple and Family Social Work
- Direct Practice and Clinical Social Work
- Emergency Services
- Human Behaviour and the Social Environment
- International and Global Issues in Social Work
- Mental and Behavioural Health
- Social Justice and Human Rights
- Social Policy and Advocacy
- Social Work and Crime and Justice
- Social Work Macro Practice
- Social Work Practice Settings
- Social Work Research and Evidence-based Practice
- Welfare and Benefit Systems
- Browse content in Sociology
- Childhood Studies
- Community Development
- Comparative and Historical Sociology
- Disability Studies
- Economic Sociology
- Gender and Sexuality
- Gerontology and Ageing
- Health, Illness, and Medicine
- Marriage and the Family
- Migration Studies
- Occupations, Professions, and Work
- Organizations
- Population and Demography
- Race and Ethnicity
- Social Theory
- Social Movements and Social Change
- Social Research and Statistics
- Social Stratification, Inequality, and Mobility
- Sociology of Religion
- Sociology of Education
- Sport and Leisure
- Urban and Rural Studies
- Browse content in Warfare and Defence
- Defence Strategy, Planning, and Research
- Land Forces and Warfare
- Military Administration
- Military Life and Institutions
- Naval Forces and Warfare
- Other Warfare and Defence Issues
- Peace Studies and Conflict Resolution
- Weapons and Equipment

The Oxford Handbook of Qualitative Research (2nd edn)
Patricia Leavy Independent Scholar Kennebunk, ME, USA
- Cite Icon Cite
- Permissions Icon Permissions
The Oxford Handbook of Qualitative Research, second edition, presents a comprehensive retrospective and prospective review of the field of qualitative research. Original, accessible chapters written by interdisciplinary leaders in the field make this a critical reference work. Filled with robust examples from real-world research; ample discussion of the historical, theoretical, and methodological foundations of the field; and coverage of key issues including data collection, interpretation, representation, assessment, and teaching, this handbook aims to be a valuable text for students, professors, and researchers. This newly revised and expanded edition features up-to-date examples and topics, including seven new chapters on duoethnography, team research, writing ethnographically, creative approaches to writing, writing for performance, writing for the public, and teaching qualitative research.
Personal account
- Sign in with email/username & password
- Get email alerts
- Save searches
- Purchase content
- Activate your purchase/trial code
- Add your ORCID iD
Institutional access
Sign in with a library card.
- Sign in with username/password
- Recommend to your librarian
- Institutional account management
- Get help with access
Access to content on Oxford Academic is often provided through institutional subscriptions and purchases. If you are a member of an institution with an active account, you may be able to access content in one of the following ways:
IP based access
Typically, access is provided across an institutional network to a range of IP addresses. This authentication occurs automatically, and it is not possible to sign out of an IP authenticated account.
Choose this option to get remote access when outside your institution. Shibboleth/Open Athens technology is used to provide single sign-on between your institution’s website and Oxford Academic.
- Click Sign in through your institution.
- Select your institution from the list provided, which will take you to your institution's website to sign in.
- When on the institution site, please use the credentials provided by your institution. Do not use an Oxford Academic personal account.
- Following successful sign in, you will be returned to Oxford Academic.
If your institution is not listed or you cannot sign in to your institution’s website, please contact your librarian or administrator.
Enter your library card number to sign in. If you cannot sign in, please contact your librarian.
Society Members
Society member access to a journal is achieved in one of the following ways:
Sign in through society site
Many societies offer single sign-on between the society website and Oxford Academic. If you see ‘Sign in through society site’ in the sign in pane within a journal:
- Click Sign in through society site.
- When on the society site, please use the credentials provided by that society. Do not use an Oxford Academic personal account.
If you do not have a society account or have forgotten your username or password, please contact your society.
Sign in using a personal account
Some societies use Oxford Academic personal accounts to provide access to their members. See below.
A personal account can be used to get email alerts, save searches, purchase content, and activate subscriptions.
Some societies use Oxford Academic personal accounts to provide access to their members.
Viewing your signed in accounts
Click the account icon in the top right to:
- View your signed in personal account and access account management features.
- View the institutional accounts that are providing access.
Signed in but can't access content
Oxford Academic is home to a wide variety of products. The institutional subscription may not cover the content that you are trying to access. If you believe you should have access to that content, please contact your librarian.
For librarians and administrators, your personal account also provides access to institutional account management. Here you will find options to view and activate subscriptions, manage institutional settings and access options, access usage statistics, and more.
Our books are available by subscription or purchase to libraries and institutions.
Month: | Total Views: |
---|---|
October 2022 | 6 |
October 2022 | 10 |
October 2022 | 53 |
October 2022 | 93 |
October 2022 | 31 |
October 2022 | 57 |
October 2022 | 88 |
October 2022 | 28 |
October 2022 | 11 |
October 2022 | 32 |
October 2022 | 30 |
October 2022 | 216 |
October 2022 | 100 |
October 2022 | 100 |
October 2022 | 39 |
October 2022 | 74 |
October 2022 | 115 |
October 2022 | 27 |
October 2022 | 41 |
October 2022 | 18 |
October 2022 | 20 |
October 2022 | 23 |
October 2022 | 99 |
October 2022 | 146 |
October 2022 | 22 |
October 2022 | 38 |
October 2022 | 5 |
October 2022 | 28 |
October 2022 | 33 |
October 2022 | 97 |
October 2022 | 102 |
October 2022 | 115 |
October 2022 | 217 |
October 2022 | 131 |
October 2022 | 65 |
October 2022 | 36 |
October 2022 | 37 |
October 2022 | 33 |
October 2022 | 7 |
October 2022 | 48 |
October 2022 | 68 |
October 2022 | 71 |
October 2022 | 11 |
October 2022 | 49 |
October 2022 | 94 |
October 2022 | 19 |
October 2022 | 27 |
November 2022 | 22 |
November 2022 | 20 |
November 2022 | 30 |
November 2022 | 65 |
November 2022 | 107 |
November 2022 | 53 |
November 2022 | 34 |
November 2022 | 229 |
November 2022 | 89 |
November 2022 | 25 |
November 2022 | 117 |
November 2022 | 56 |
November 2022 | 9 |
November 2022 | 12 |
November 2022 | 89 |
November 2022 | 28 |
November 2022 | 24 |
November 2022 | 306 |
November 2022 | 19 |
November 2022 | 137 |
November 2022 | 93 |
November 2022 | 31 |
November 2022 | 36 |
November 2022 | 42 |
November 2022 | 1 |
November 2022 | 13 |
November 2022 | 49 |
November 2022 | 77 |
November 2022 | 14 |
November 2022 | 29 |
November 2022 | 15 |
November 2022 | 22 |
November 2022 | 102 |
November 2022 | 87 |
November 2022 | 110 |
November 2022 | 71 |
November 2022 | 34 |
November 2022 | 39 |
November 2022 | 26 |
November 2022 | 53 |
November 2022 | 61 |
November 2022 | 8 |
November 2022 | 51 |
November 2022 | 42 |
November 2022 | 97 |
November 2022 | 16 |
November 2022 | 35 |
December 2022 | 90 |
December 2022 | 30 |
December 2022 | 109 |
December 2022 | 50 |
December 2022 | 102 |
December 2022 | 98 |
December 2022 | 57 |
December 2022 | 292 |
December 2022 | 73 |
December 2022 | 22 |
December 2022 | 7 |
December 2022 | 86 |
December 2022 | 61 |
December 2022 | 23 |
December 2022 | 40 |
December 2022 | 154 |
December 2022 | 49 |
December 2022 | 21 |
December 2022 | 48 |
December 2022 | 102 |
December 2022 | 207 |
December 2022 | 53 |
December 2022 | 68 |
December 2022 | 23 |
December 2022 | 47 |
December 2022 | 81 |
December 2022 | 22 |
December 2022 | 27 |
December 2022 | 29 |
December 2022 | 27 |
December 2022 | 32 |
December 2022 | 83 |
December 2022 | 60 |
December 2022 | 72 |
December 2022 | 20 |
December 2022 | 86 |
December 2022 | 63 |
December 2022 | 55 |
December 2022 | 32 |
December 2022 | 44 |
December 2022 | 41 |
December 2022 | 28 |
December 2022 | 95 |
December 2022 | 102 |
December 2022 | 41 |
December 2022 | 26 |
December 2022 | 66 |
January 2023 | 191 |
January 2023 | 32 |
January 2023 | 64 |
January 2023 | 50 |
January 2023 | 71 |
January 2023 | 329 |
January 2023 | 89 |
January 2023 | 83 |
January 2023 | 7 |
January 2023 | 29 |
January 2023 | 28 |
January 2023 | 4 |
January 2023 | 48 |
January 2023 | 50 |
January 2023 | 79 |
January 2023 | 70 |
January 2023 | 8 |
January 2023 | 31 |
January 2023 | 23 |
January 2023 | 4 |
January 2023 | 74 |
January 2023 | 48 |
January 2023 | 178 |
January 2023 | 35 |
January 2023 | 36 |
January 2023 | 26 |
January 2023 | 17 |
January 2023 | 65 |
January 2023 | 9 |
January 2023 | 59 |
January 2023 | 26 |
January 2023 | 35 |
January 2023 | 213 |
January 2023 | 25 |
January 2023 | 31 |
January 2023 | 12 |
January 2023 | 65 |
January 2023 | 27 |
January 2023 | 88 |
January 2023 | 112 |
January 2023 | 23 |
January 2023 | 98 |
January 2023 | 69 |
January 2023 | 150 |
January 2023 | 48 |
January 2023 | 192 |
January 2023 | 74 |
February 2023 | 99 |
February 2023 | 27 |
February 2023 | 141 |
February 2023 | 66 |
February 2023 | 126 |
February 2023 | 40 |
February 2023 | 51 |
February 2023 | 83 |
February 2023 | 293 |
February 2023 | 175 |
February 2023 | 9 |
February 2023 | 48 |
February 2023 | 105 |
February 2023 | 11 |
February 2023 | 35 |
February 2023 | 44 |
February 2023 | 103 |
February 2023 | 28 |
February 2023 | 157 |
February 2023 | 45 |
February 2023 | 39 |
February 2023 | 11 |
February 2023 | 40 |
February 2023 | 7 |
February 2023 | 91 |
February 2023 | 239 |
February 2023 | 64 |
February 2023 | 10 |
February 2023 | 45 |
February 2023 | 27 |
February 2023 | 60 |
February 2023 | 27 |
February 2023 | 42 |
February 2023 | 84 |
February 2023 | 87 |
February 2023 | 31 |
February 2023 | 56 |
February 2023 | 152 |
February 2023 | 35 |
February 2023 | 35 |
February 2023 | 129 |
February 2023 | 9 |
February 2023 | 106 |
February 2023 | 87 |
February 2023 | 59 |
February 2023 | 93 |
February 2023 | 97 |
March 2023 | 80 |
March 2023 | 21 |
March 2023 | 60 |
March 2023 | 154 |
March 2023 | 174 |
March 2023 | 74 |
March 2023 | 84 |
March 2023 | 228 |
March 2023 | 111 |
March 2023 | 383 |
March 2023 | 51 |
March 2023 | 6 |
March 2023 | 19 |
March 2023 | 13 |
March 2023 | 46 |
March 2023 | 85 |
March 2023 | 219 |
March 2023 | 51 |
March 2023 | 15 |
March 2023 | 40 |
March 2023 | 48 |
March 2023 | 12 |
March 2023 | 20 |
March 2023 | 81 |
March 2023 | 39 |
March 2023 | 83 |
March 2023 | 83 |
March 2023 | 12 |
March 2023 | 313 |
March 2023 | 101 |
March 2023 | 43 |
March 2023 | 20 |
March 2023 | 214 |
March 2023 | 101 |
March 2023 | 26 |
March 2023 | 27 |
March 2023 | 63 |
March 2023 | 21 |
March 2023 | 80 |
March 2023 | 82 |
March 2023 | 9 |
March 2023 | 49 |
March 2023 | 151 |
March 2023 | 108 |
March 2023 | 106 |
March 2023 | 28 |
March 2023 | 36 |
April 2023 | 65 |
April 2023 | 110 |
April 2023 | 18 |
April 2023 | 58 |
April 2023 | 173 |
April 2023 | 74 |
April 2023 | 126 |
April 2023 | 43 |
April 2023 | 393 |
April 2023 | 41 |
April 2023 | 82 |
April 2023 | 12 |
April 2023 | 53 |
April 2023 | 39 |
April 2023 | 30 |
April 2023 | 53 |
April 2023 | 2 |
April 2023 | 16 |
April 2023 | 24 |
April 2023 | 22 |
April 2023 | 33 |
April 2023 | 8 |
April 2023 | 11 |
April 2023 | 33 |
April 2023 | 15 |
April 2023 | 37 |
April 2023 | 95 |
April 2023 | 29 |
April 2023 | 254 |
April 2023 | 45 |
April 2023 | 24 |
April 2023 | 166 |
April 2023 | 94 |
April 2023 | 40 |
April 2023 | 7 |
April 2023 | 32 |
April 2023 | 69 |
April 2023 | 31 |
April 2023 | 6 |
April 2023 | 124 |
April 2023 | 46 |
April 2023 | 18 |
April 2023 | 101 |
April 2023 | 152 |
April 2023 | 93 |
April 2023 | 29 |
April 2023 | 58 |
May 2023 | 90 |
May 2023 | 202 |
May 2023 | 60 |
May 2023 | 28 |
May 2023 | 90 |
May 2023 | 27 |
May 2023 | 59 |
May 2023 | 139 |
May 2023 | 51 |
May 2023 | 100 |
May 2023 | 68 |
May 2023 | 324 |
May 2023 | 36 |
May 2023 | 8 |
May 2023 | 67 |
May 2023 | 75 |
May 2023 | 14 |
May 2023 | 25 |
May 2023 | 30 |
May 2023 | 73 |
May 2023 | 34 |
May 2023 | 45 |
May 2023 | 38 |
May 2023 | 141 |
May 2023 | 13 |
May 2023 | 93 |
May 2023 | 84 |
May 2023 | 270 |
May 2023 | 9 |
May 2023 | 39 |
May 2023 | 18 |
May 2023 | 36 |
May 2023 | 32 |
May 2023 | 13 |
May 2023 | 68 |
May 2023 | 36 |
May 2023 | 66 |
May 2023 | 180 |
May 2023 | 112 |
May 2023 | 58 |
May 2023 | 26 |
May 2023 | 42 |
May 2023 | 7 |
May 2023 | 113 |
May 2023 | 109 |
May 2023 | 129 |
May 2023 | 22 |
June 2023 | 42 |
June 2023 | 25 |
June 2023 | 87 |
June 2023 | 93 |
June 2023 | 108 |
June 2023 | 85 |
June 2023 | 42 |
June 2023 | 54 |
June 2023 | 181 |
June 2023 | 83 |
June 2023 | 34 |
June 2023 | 31 |
June 2023 | 67 |
June 2023 | 9 |
June 2023 | 87 |
June 2023 | 40 |
June 2023 | 13 |
June 2023 | 28 |
June 2023 | 33 |
June 2023 | 13 |
June 2023 | 44 |
June 2023 | 27 |
June 2023 | 30 |
June 2023 | 13 |
June 2023 | 51 |
June 2023 | 33 |
June 2023 | 158 |
June 2023 | 73 |
June 2023 | 29 |
June 2023 | 13 |
June 2023 | 28 |
June 2023 | 80 |
June 2023 | 87 |
June 2023 | 36 |
June 2023 | 30 |
June 2023 | 33 |
June 2023 | 65 |
June 2023 | 63 |
June 2023 | 45 |
June 2023 | 66 |
June 2023 | 76 |
June 2023 | 98 |
June 2023 | 89 |
June 2023 | 24 |
June 2023 | 36 |
June 2023 | 55 |
June 2023 | 10 |
July 2023 | 9 |
July 2023 | 11 |
July 2023 | 44 |
July 2023 | 36 |
July 2023 | 14 |
July 2023 | 56 |
July 2023 | 75 |
July 2023 | 51 |
July 2023 | 103 |
July 2023 | 27 |
July 2023 | 155 |
July 2023 | 55 |
July 2023 | 62 |
July 2023 | 33 |
July 2023 | 22 |
July 2023 | 27 |
July 2023 | 5 |
July 2023 | 9 |
July 2023 | 33 |
July 2023 | 35 |
July 2023 | 27 |
July 2023 | 16 |
July 2023 | 11 |
July 2023 | 99 |
July 2023 | 17 |
July 2023 | 35 |
July 2023 | 10 |
July 2023 | 20 |
July 2023 | 21 |
July 2023 | 17 |
July 2023 | 32 |
July 2023 | 23 |
July 2023 | 76 |
July 2023 | 49 |
July 2023 | 43 |
July 2023 | 25 |
July 2023 | 59 |
July 2023 | 80 |
July 2023 | 159 |
July 2023 | 18 |
July 2023 | 63 |
July 2023 | 14 |
July 2023 | 20 |
July 2023 | 34 |
July 2023 | 44 |
July 2023 | 48 |
July 2023 | 32 |
August 2023 | 49 |
August 2023 | 27 |
August 2023 | 99 |
August 2023 | 52 |
August 2023 | 181 |
August 2023 | 49 |
August 2023 | 68 |
August 2023 | 90 |
August 2023 | 223 |
August 2023 | 43 |
August 2023 | 7 |
August 2023 | 54 |
August 2023 | 26 |
August 2023 | 19 |
August 2023 | 39 |
August 2023 | 26 |
August 2023 | 69 |
August 2023 | 45 |
August 2023 | 25 |
August 2023 | 32 |
August 2023 | 10 |
August 2023 | 42 |
August 2023 | 14 |
August 2023 | 36 |
August 2023 | 71 |
August 2023 | 177 |
August 2023 | 65 |
August 2023 | 23 |
August 2023 | 77 |
August 2023 | 28 |
August 2023 | 79 |
August 2023 | 43 |
August 2023 | 60 |
August 2023 | 23 |
August 2023 | 17 |
August 2023 | 30 |
August 2023 | 128 |
August 2023 | 80 |
August 2023 | 182 |
August 2023 | 59 |
August 2023 | 28 |
August 2023 | 41 |
August 2023 | 12 |
August 2023 | 80 |
August 2023 | 22 |
August 2023 | 148 |
August 2023 | 127 |
September 2023 | 24 |
September 2023 | 60 |
September 2023 | 75 |
September 2023 | 57 |
September 2023 | 26 |
September 2023 | 100 |
September 2023 | 107 |
September 2023 | 190 |
September 2023 | 345 |
September 2023 | 277 |
September 2023 | 76 |
September 2023 | 98 |
September 2023 | 5 |
September 2023 | 51 |
September 2023 | 102 |
September 2023 | 48 |
September 2023 | 30 |
September 2023 | 75 |
September 2023 | 51 |
September 2023 | 39 |
September 2023 | 23 |
September 2023 | 210 |
September 2023 | 61 |
September 2023 | 118 |
September 2023 | 257 |
September 2023 | 28 |
September 2023 | 23 |
September 2023 | 51 |
September 2023 | 43 |
September 2023 | 77 |
September 2023 | 119 |
September 2023 | 50 |
September 2023 | 40 |
September 2023 | 30 |
September 2023 | 168 |
September 2023 | 39 |
September 2023 | 53 |
September 2023 | 24 |
September 2023 | 108 |
September 2023 | 110 |
September 2023 | 31 |
September 2023 | 93 |
September 2023 | 129 |
September 2023 | 39 |
September 2023 | 43 |
September 2023 | 38 |
September 2023 | 31 |
October 2023 | 83 |
October 2023 | 15 |
October 2023 | 45 |
October 2023 | 92 |
October 2023 | 109 |
October 2023 | 154 |
October 2023 | 79 |
October 2023 | 34 |
October 2023 | 208 |
October 2023 | 2 |
October 2023 | 27 |
October 2023 | 11 |
October 2023 | 16 |
October 2023 | 20 |
October 2023 | 30 |
October 2023 | 31 |
October 2023 | 86 |
October 2023 | 30 |
October 2023 | 152 |
October 2023 | 118 |
October 2023 | 64 |
October 2023 | 152 |
October 2023 | 18 |
October 2023 | 12 |
October 2023 | 102 |
October 2023 | 97 |
October 2023 | 54 |
October 2023 | 13 |
October 2023 | 18 |
October 2023 | 45 |
October 2023 | 94 |
October 2023 | 159 |
October 2023 | 257 |
October 2023 | 80 |
October 2023 | 30 |
October 2023 | 38 |
October 2023 | 13 |
October 2023 | 14 |
October 2023 | 23 |
October 2023 | 107 |
October 2023 | 50 |
October 2023 | 139 |
October 2023 | 16 |
October 2023 | 41 |
October 2023 | 29 |
October 2023 | 13 |
October 2023 | 29 |
November 2023 | 9 |
November 2023 | 109 |
November 2023 | 96 |
November 2023 | 14 |
November 2023 | 25 |
November 2023 | 89 |
November 2023 | 21 |
November 2023 | 50 |
November 2023 | 129 |
November 2023 | 44 |
November 2023 | 106 |
November 2023 | 52 |
November 2023 | 21 |
November 2023 | 265 |
November 2023 | 109 |
November 2023 | 13 |
November 2023 | 32 |
November 2023 | 9 |
November 2023 | 1 |
November 2023 | 61 |
November 2023 | 37 |
November 2023 | 24 |
November 2023 | 49 |
November 2023 | 37 |
November 2023 | 9 |
November 2023 | 77 |
November 2023 | 313 |
November 2023 | 46 |
November 2023 | 14 |
November 2023 | 11 |
November 2023 | 25 |
November 2023 | 24 |
November 2023 | 139 |
November 2023 | 12 |
November 2023 | 21 |
November 2023 | 80 |
November 2023 | 34 |
November 2023 | 2 |
November 2023 | 10 |
November 2023 | 52 |
November 2023 | 99 |
November 2023 | 11 |
November 2023 | 59 |
November 2023 | 71 |
November 2023 | 69 |
November 2023 | 136 |
November 2023 | 106 |
December 2023 | 59 |
December 2023 | 18 |
December 2023 | 72 |
December 2023 | 44 |
December 2023 | 103 |
December 2023 | 267 |
December 2023 | 58 |
December 2023 | 4 |
December 2023 | 61 |
December 2023 | 36 |
December 2023 | 36 |
December 2023 | 12 |
December 2023 | 42 |
December 2023 | 32 |
December 2023 | 15 |
December 2023 | 57 |
December 2023 | 63 |
December 2023 | 114 |
December 2023 | 18 |
December 2023 | 35 |
December 2023 | 5 |
December 2023 | 52 |
December 2023 | 20 |
December 2023 | 22 |
December 2023 | 84 |
December 2023 | 29 |
December 2023 | 68 |
December 2023 | 90 |
December 2023 | 206 |
December 2023 | 22 |
December 2023 | 53 |
December 2023 | 18 |
December 2023 | 41 |
December 2023 | 49 |
December 2023 | 83 |
December 2023 | 24 |
December 2023 | 5 |
December 2023 | 76 |
December 2023 | 40 |
December 2023 | 72 |
December 2023 | 32 |
December 2023 | 35 |
December 2023 | 11 |
December 2023 | 15 |
December 2023 | 102 |
December 2023 | 13 |
December 2023 | 9 |
January 2024 | 39 |
January 2024 | 76 |
January 2024 | 65 |
January 2024 | 65 |
January 2024 | 79 |
January 2024 | 74 |
January 2024 | 43 |
January 2024 | 40 |
January 2024 | 15 |
January 2024 | 116 |
January 2024 | 25 |
January 2024 | 88 |
January 2024 | 124 |
January 2024 | 110 |
January 2024 | 127 |
January 2024 | 302 |
January 2024 | 107 |
January 2024 | 74 |
January 2024 | 9 |
January 2024 | 86 |
January 2024 | 61 |
January 2024 | 36 |
January 2024 | 42 |
January 2024 | 75 |
January 2024 | 261 |
January 2024 | 173 |
January 2024 | 24 |
January 2024 | 31 |
January 2024 | 114 |
January 2024 | 12 |
January 2024 | 91 |
January 2024 | 67 |
January 2024 | 17 |
January 2024 | 90 |
January 2024 | 216 |
January 2024 | 42 |
January 2024 | 21 |
January 2024 | 79 |
January 2024 | 45 |
January 2024 | 84 |
January 2024 | 35 |
January 2024 | 16 |
January 2024 | 31 |
January 2024 | 32 |
January 2024 | 20 |
January 2024 | 25 |
January 2024 | 88 |
February 2024 | 353 |
February 2024 | 3 |
February 2024 | 73 |
February 2024 | 7 |
February 2024 | 37 |
February 2024 | 71 |
February 2024 | 12 |
February 2024 | 124 |
February 2024 | 96 |
February 2024 | 27 |
February 2024 | 63 |
February 2024 | 192 |
February 2024 | 81 |
February 2024 | 16 |
February 2024 | 127 |
February 2024 | 97 |
February 2024 | 33 |
February 2024 | 42 |
February 2024 | 7 |
February 2024 | 19 |
February 2024 | 28 |
February 2024 | 85 |
February 2024 | 45 |
February 2024 | 127 |
February 2024 | 39 |
February 2024 | 238 |
February 2024 | 13 |
February 2024 | 30 |
February 2024 | 17 |
February 2024 | 13 |
February 2024 | 24 |
February 2024 | 111 |
February 2024 | 48 |
February 2024 | 7 |
February 2024 | 131 |
February 2024 | 8 |
February 2024 | 71 |
February 2024 | 12 |
February 2024 | 14 |
February 2024 | 98 |
February 2024 | 53 |
February 2024 | 56 |
February 2024 | 35 |
February 2024 | 107 |
February 2024 | 5 |
February 2024 | 34 |
February 2024 | 126 |
March 2024 | 26 |
March 2024 | 86 |
March 2024 | 80 |
March 2024 | 48 |
March 2024 | 52 |
March 2024 | 8 |
March 2024 | 34 |
March 2024 | 223 |
March 2024 | 481 |
March 2024 | 3 |
March 2024 | 36 |
March 2024 | 232 |
March 2024 | 73 |
March 2024 | 166 |
March 2024 | 11 |
March 2024 | 22 |
March 2024 | 45 |
March 2024 | 9 |
March 2024 | 29 |
March 2024 | 39 |
March 2024 | 26 |
March 2024 | 86 |
March 2024 | 57 |
March 2024 | 33 |
March 2024 | 194 |
March 2024 | 99 |
March 2024 | 27 |
March 2024 | 129 |
March 2024 | 151 |
March 2024 | 51 |
March 2024 | 65 |
March 2024 | 240 |
March 2024 | 108 |
March 2024 | 25 |
March 2024 | 88 |
March 2024 | 44 |
March 2024 | 45 |
March 2024 | 8 |
March 2024 | 27 |
March 2024 | 153 |
March 2024 | 32 |
March 2024 | 104 |
March 2024 | 9 |
March 2024 | 49 |
March 2024 | 114 |
March 2024 | 173 |
March 2024 | 111 |
April 2024 | 105 |
April 2024 | 25 |
April 2024 | 92 |
April 2024 | 140 |
April 2024 | 96 |
April 2024 | 32 |
April 2024 | 99 |
April 2024 | 91 |
April 2024 | 149 |
April 2024 | 91 |
April 2024 | 16 |
April 2024 | 21 |
April 2024 | 100 |
April 2024 | 148 |
April 2024 | 19 |
April 2024 | 88 |
April 2024 | 83 |
April 2024 | 495 |
April 2024 | 157 |
April 2024 | 49 |
April 2024 | 69 |
April 2024 | 13 |
April 2024 | 236 |
April 2024 | 188 |
April 2024 | 18 |
April 2024 | 31 |
April 2024 | 9 |
April 2024 | 11 |
April 2024 | 286 |
April 2024 | 61 |
April 2024 | 128 |
April 2024 | 76 |
April 2024 | 271 |
April 2024 | 21 |
April 2024 | 23 |
April 2024 | 44 |
April 2024 | 36 |
April 2024 | 50 |
April 2024 | 169 |
April 2024 | 42 |
April 2024 | 29 |
April 2024 | 187 |
April 2024 | 39 |
April 2024 | 143 |
April 2024 | 194 |
April 2024 | 13 |
April 2024 | 37 |
May 2024 | 18 |
May 2024 | 135 |
May 2024 | 67 |
May 2024 | 57 |
May 2024 | 131 |
May 2024 | 123 |
May 2024 | 182 |
May 2024 | 57 |
May 2024 | 58 |
May 2024 | 88 |
May 2024 | 410 |
May 2024 | 65 |
May 2024 | 10 |
May 2024 | 19 |
May 2024 | 47 |
May 2024 | 14 |
May 2024 | 82 |
May 2024 | 45 |
May 2024 | 26 |
May 2024 | 12 |
May 2024 | 201 |
May 2024 | 49 |
May 2024 | 32 |
May 2024 | 19 |
May 2024 | 121 |
May 2024 | 28 |
May 2024 | 81 |
May 2024 | 15 |
May 2024 | 297 |
May 2024 | 33 |
May 2024 | 69 |
May 2024 | 35 |
May 2024 | 31 |
May 2024 | 21 |
May 2024 | 123 |
May 2024 | 20 |
May 2024 | 166 |
May 2024 | 56 |
May 2024 | 73 |
May 2024 | 30 |
May 2024 | 11 |
May 2024 | 39 |
May 2024 | 179 |
May 2024 | 132 |
May 2024 | 133 |
May 2024 | 17 |
May 2024 | 110 |
June 2024 | 38 |
June 2024 | 11 |
June 2024 | 77 |
June 2024 | 52 |
June 2024 | 102 |
June 2024 | 35 |
June 2024 | 87 |
June 2024 | 32 |
June 2024 | 78 |
June 2024 | 37 |
June 2024 | 205 |
June 2024 | 2 |
June 2024 | 7 |
June 2024 | 26 |
June 2024 | 13 |
June 2024 | 41 |
June 2024 | 39 |
June 2024 | 22 |
June 2024 | 27 |
June 2024 | 114 |
June 2024 | 5 |
June 2024 | 39 |
June 2024 | 14 |
June 2024 | 2 |
June 2024 | 20 |
June 2024 | 26 |
June 2024 | 48 |
June 2024 | 21 |
June 2024 | 146 |
June 2024 | 4 |
June 2024 | 49 |
June 2024 | 8 |
June 2024 | 62 |
June 2024 | 8 |
June 2024 | 98 |
June 2024 | 34 |
June 2024 | 19 |
June 2024 | 68 |
June 2024 | 20 |
June 2024 | 13 |
June 2024 | 100 |
June 2024 | 3 |
June 2024 | 18 |
June 2024 | 21 |
June 2024 | 62 |
June 2024 | 68 |
June 2024 | 50 |
July 2024 | 35 |
July 2024 | 18 |
July 2024 | 43 |
July 2024 | 89 |
July 2024 | 30 |
July 2024 | 145 |
July 2024 | 84 |
July 2024 | 231 |
July 2024 | 88 |
July 2024 | 28 |
July 2024 | 81 |
July 2024 | 12 |
July 2024 | 34 |
July 2024 | 21 |
July 2024 | 14 |
July 2024 | 67 |
July 2024 | 39 |
July 2024 | 10 |
July 2024 | 31 |
July 2024 | 48 |
July 2024 | 28 |
July 2024 | 64 |
July 2024 | 5 |
July 2024 | 141 |
July 2024 | 14 |
July 2024 | 60 |
July 2024 | 65 |
July 2024 | 9 |
July 2024 | 122 |
July 2024 | 18 |
July 2024 | 22 |
July 2024 | 143 |
July 2024 | 9 |
July 2024 | 55 |
July 2024 | 11 |
July 2024 | 6 |
July 2024 | 60 |
July 2024 | 99 |
July 2024 | 119 |
July 2024 | 178 |
July 2024 | 96 |
July 2024 | 53 |
July 2024 | 35 |
July 2024 | 38 |
July 2024 | 3 |
July 2024 | 28 |
July 2024 | 79 |
August 2024 | 18 |
August 2024 | 102 |
August 2024 | 81 |
August 2024 | 105 |
August 2024 | 49 |
August 2024 | 39 |
August 2024 | 21 |
August 2024 | 121 |
August 2024 | 56 |
August 2024 | 97 |
August 2024 | 33 |
August 2024 | 58 |
August 2024 | 215 |
August 2024 | 94 |
August 2024 | 5 |
August 2024 | 24 |
August 2024 | 58 |
August 2024 | 20 |
August 2024 | 30 |
August 2024 | 68 |
August 2024 | 19 |
August 2024 | 36 |
August 2024 | 16 |
August 2024 | 31 |
August 2024 | 138 |
August 2024 | 19 |
August 2024 | 21 |
August 2024 | 47 |
August 2024 | 73 |
August 2024 | 30 |
August 2024 | 137 |
August 2024 | 37 |
August 2024 | 62 |
August 2024 | 75 |
August 2024 | 20 |
August 2024 | 85 |
August 2024 | 18 |
August 2024 | 22 |
August 2024 | 54 |
August 2024 | 18 |
August 2024 | 101 |
August 2024 | 26 |
August 2024 | 93 |
August 2024 | 21 |
August 2024 | 43 |
August 2024 | 69 |
August 2024 | 36 |
September 2024 | 43 |
September 2024 | 19 |
September 2024 | 80 |
September 2024 | 94 |
September 2024 | 128 |
September 2024 | 28 |
September 2024 | 108 |
September 2024 | 151 |
September 2024 | 274 |
September 2024 | 33 |
September 2024 | 17 |
September 2024 | 33 |
September 2024 | 29 |
September 2024 | 81 |
September 2024 | 20 |
September 2024 | 48 |
September 2024 | 29 |
September 2024 | 15 |
September 2024 | 168 |
September 2024 | 27 |
September 2024 | 163 |
September 2024 | 42 |
September 2024 | 20 |
September 2024 | 38 |
September 2024 | 53 |
September 2024 | 51 |
September 2024 | 28 |
September 2024 | 120 |
September 2024 | 78 |
September 2024 | 30 |
September 2024 | 20 |
September 2024 | 13 |
September 2024 | 53 |
September 2024 | 83 |
September 2024 | 134 |
September 2024 | 58 |
September 2024 | 12 |
September 2024 | 65 |
September 2024 | 196 |
September 2024 | 39 |
September 2024 | 22 |
September 2024 | 76 |
September 2024 | 74 |
September 2024 | 5 |
September 2024 | 37 |
September 2024 | 66 |
September 2024 | 20 |
- About Oxford Academic
- Publish journals with us
- University press partners
- What we publish
- New features
- Open access
- Rights and permissions
- Accessibility
- Advertising
- Media enquiries
- Oxford University Press
- Oxford Languages
- University of Oxford
Oxford University Press is a department of the University of Oxford. It furthers the University's objective of excellence in research, scholarship, and education by publishing worldwide
- Copyright © 2024 Oxford University Press
- Cookie settings
- Cookie policy
- Privacy policy
- Legal notice
This Feature Is Available To Subscribers Only
Sign In or Create an Account
This PDF is available to Subscribers Only
For full access to this pdf, sign in to an existing account, or purchase an annual subscription.
- AI & Tech
- Case Studies
- Churn & Loyalty
- Customer Experience
- Customer Journeys
- Data analytics
- Feedback Analysis
- Product Experience
- Product Updates
- Sentiment Analysis
- Surveys & Feedback Collection
- Text Analytics
- Thematic vs Others
- Using Thematic
- Voice of Customer
- Try Thematic
- Data Analytics
- Thematic vs others
- AI and tech
Welcome to the community
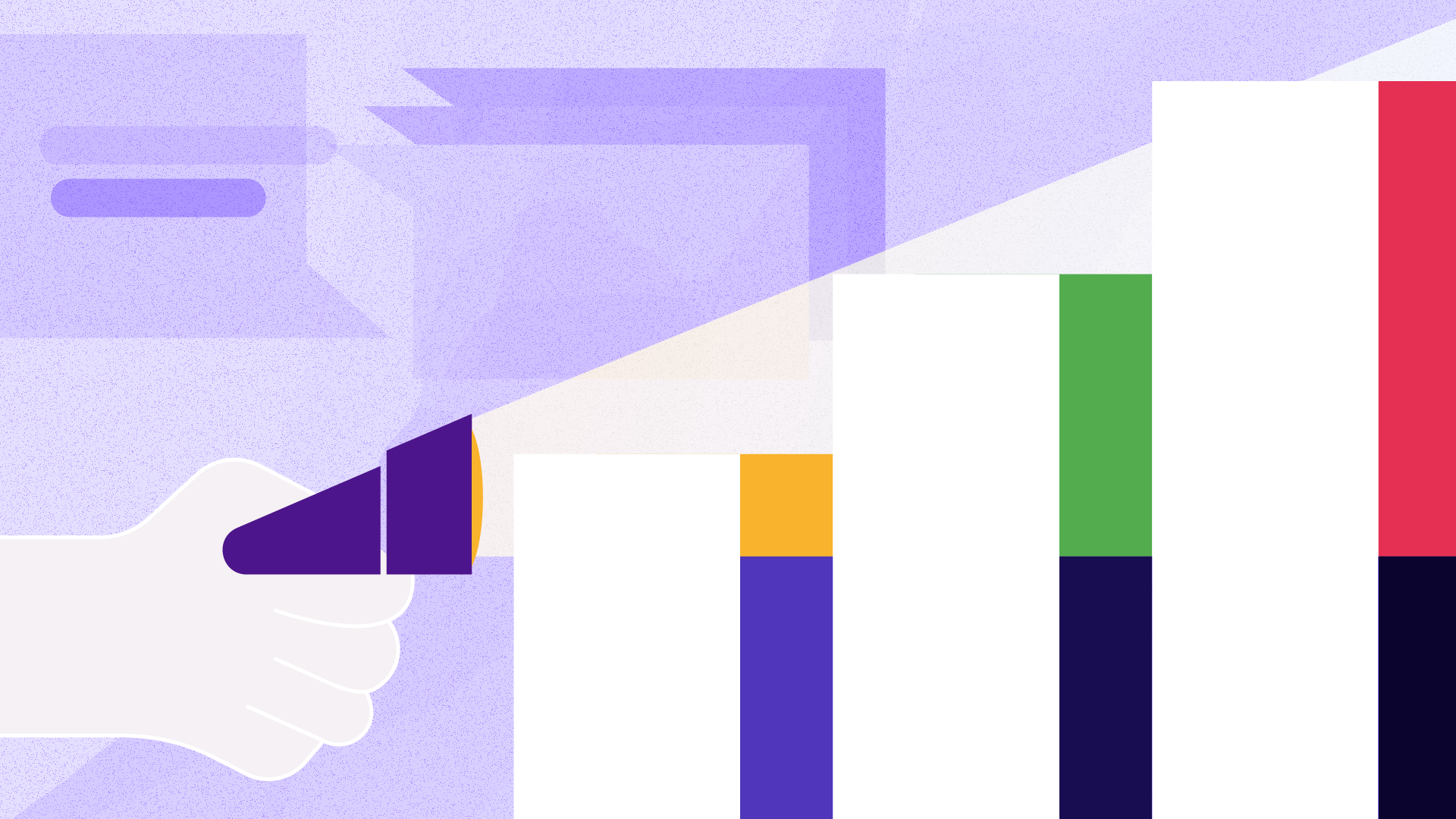
The Primary Methods of Qualitative Data Analysis
In academic research as well as in the business landscape, qualitative data analysis plays a crucial role in understanding and interpreting non-numerical data.
Qualitative data analysis helps us make sense of the stories and personal narratives. In the business context, qualitative data analysis turns customer feedback into an in-depth understanding of what matters to customers. Sharing the insights from this analysis with decision-makers helps them drive initiatives that improve customer experiences.
While quantitative data analysis focuses on numerical measurement and statistical analysis, qualitative data analysis delves into the rich and complex nature of human experiences and perceptions. When analyzed effectively, customer feedback can be transformed into actionable insights for every team across the company.
This guide will provide an in-depth exploration of the different methods employed in qualitative data analysis, as well as the steps involved and challenges encountered. We’ll also have a look at what QDA means in the business context and how to turn it into a high-powered tool for CX and product teams.
Understanding Qualitative Data Analysis Methods
Definition and importance of qualitative data analysis.
Qualitative data analysis refers to the systematic process of examining and interpreting non-numerical data to gain meaningful insights and generate new knowledge. It’s what happens when you put a year’s worth of Amazon reviews into a thematic analysis engine, and end up with a thorough understanding of how users interact with your product (and half a dozen actionable insights to boot).
It involves dissecting text, images, videos, and other forms of qualitative data to identify patterns, themes, and relationships.
By capturing the nuances and depth of human experiences, the qualitative data analysis approach allows researchers to explore complex social phenomena that quantitative approaches cannot fully capture. It provides a rich and detailed understanding of social contexts, individual perspectives, and subjective experiences.
Qualitative data analysis methods offer an in-depth exploration of the hows and whys behind social phenomena, enabling researchers to gain a comprehensive understanding of complex social issues. It is incredibly valuable in fields such as sociology, anthropology, psychology, and education, where human behavior and social interactions are studied.
In these fields, researchers often seek to understand the intricacies of human experiences, and qualitative data analysis allows them to capture the complexity of these phenomena.
In the world of business & product development, qualitative data analysis methods can work to improve user experiences. Suddenly, you’ve got the opportunity to reach a comprehensive understanding of just what your products mean on the social landscape.
User feedback gets transformed into big-picture knowledge that offers a 360-degree view of how a product performs in the real world. Product teams get a solid, reliable basis on which to make decisions , and guesswork becomes a thing of the past.
Key Principles of Qualitative Data Analysis
Before delving into the various methods of qualitative data analysis, let’s look at the key principles that underpin these analysis techniques. Qualitative data analysis is guided by the following principles:
- Inductive Reasoning: Qualitative research focuses on specific observations and gradually develops broader interpretations and theories. It allows for the discovery of new patterns and relationships through an iterative process of data investigation.
- Contextual Understanding: Qualitative data analysis emphasizes the importance of understanding the research context and the social, cultural, and historical factors that shape it. Context provides meaning and helps researchers identify themes as well as interpret and make sense of the data.
- Subjectivity and Reflexivity: When research is human-led, the researchers acknowledge and critically reflect upon their own beliefs, biases, and experiences throughout the qualitative data analysis process. Where research is AI-driven, humans get a chance to view the actual data each insight is based on and check to see if it makes objective sense.
- Active Engagement: A qualitative data analysis method is an active and dynamic process that involves constant engagement with the data. Thematic analysis works most effectively as an ongoing process, thoroughly examining and interpreting all available data, while continually questioning and refining the research questions and analysis as new data points are added.
Inductive reasoning is a fundamental principle of qualitative data analysis. It allows researchers to start with specific observations and gradually develop broader interpretations and theories. Through this iterative process of data investigation, new patterns and relationships can be discovered. When you’ve got AI-driven data analysis software, this inductive reasoning is going on under the hood.
Contextual understanding is another key principle of the qualitative analysis process. It emphasizes the importance of understanding the research context and the social, cultural, and historical factors that shape it.
By considering the context when analyzing qualitative data, researchers can gain a deeper understanding of the data and interpret it more accurately. Well-designed thematic analysis software has this built in.
Subjectivity and reflexivity are essential principles in qualitative data analysis. Qualitative data analysis research must be repeatable if it is to be relied on, and there should always be ways to check just what qualitative feedback particular trends and insights come from. When qualitative data analysis is done right, transparency and rigor can be maintained throughout the process, from the initial selection of research questions and gathering of raw data to final analysis techniques.
Active engagement is a crucial aspect of qualitative feedback interpretation. It involves constant engagement with the data, as researchers thoroughly examine and interpret it. This active and dynamic process allows researchers to continually question and refine their qualitative analysis, ensuring a comprehensive understanding of the data.
Different Qualitative Data Analysis Methods
Just how does qualitative analysis work out in practice? In this article, we will explore five commonly used qualitative analysis methods: content analysis, narrative analysis, discourse analysis, grounded theory, and thematic analysis.
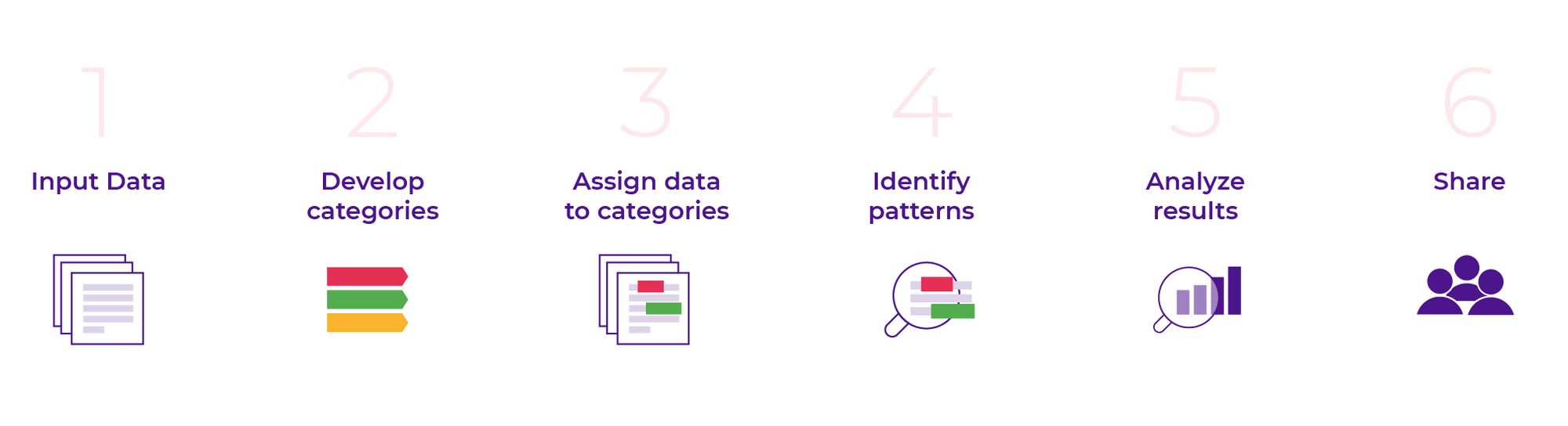
- Content Analysis
Content analysis is a systematic and objective approach to analyzing data by categorizing, coding, and quantifying specific words, themes, or concepts within a text. It involves identifying patterns, frequencies, and relationships in the content, which can be textual, visual, or auditory.
Researchers can employ content analysis techniques to examine interviews, focus group discussions, newspaper articles, social media posts, and other forms of textual data. By assigning codes to different segments of the text, researchers can identify recurring themes, sentiments, or messages.
This same qualitative data analysis approach can be used by CX and product teams to analyze customer feedback or support tickets.
For example, in an analysis of public response to a new product, a PX team might use content analysis to analyze social media posts discussing the topic.
By categorizing the posts based on their stance (e.g., positive, negative, neutral) and identifying recurring themes (e.g., user experience, look and feel), a company could gain insights into the dominant narratives and public perceptions surrounding the product launch.
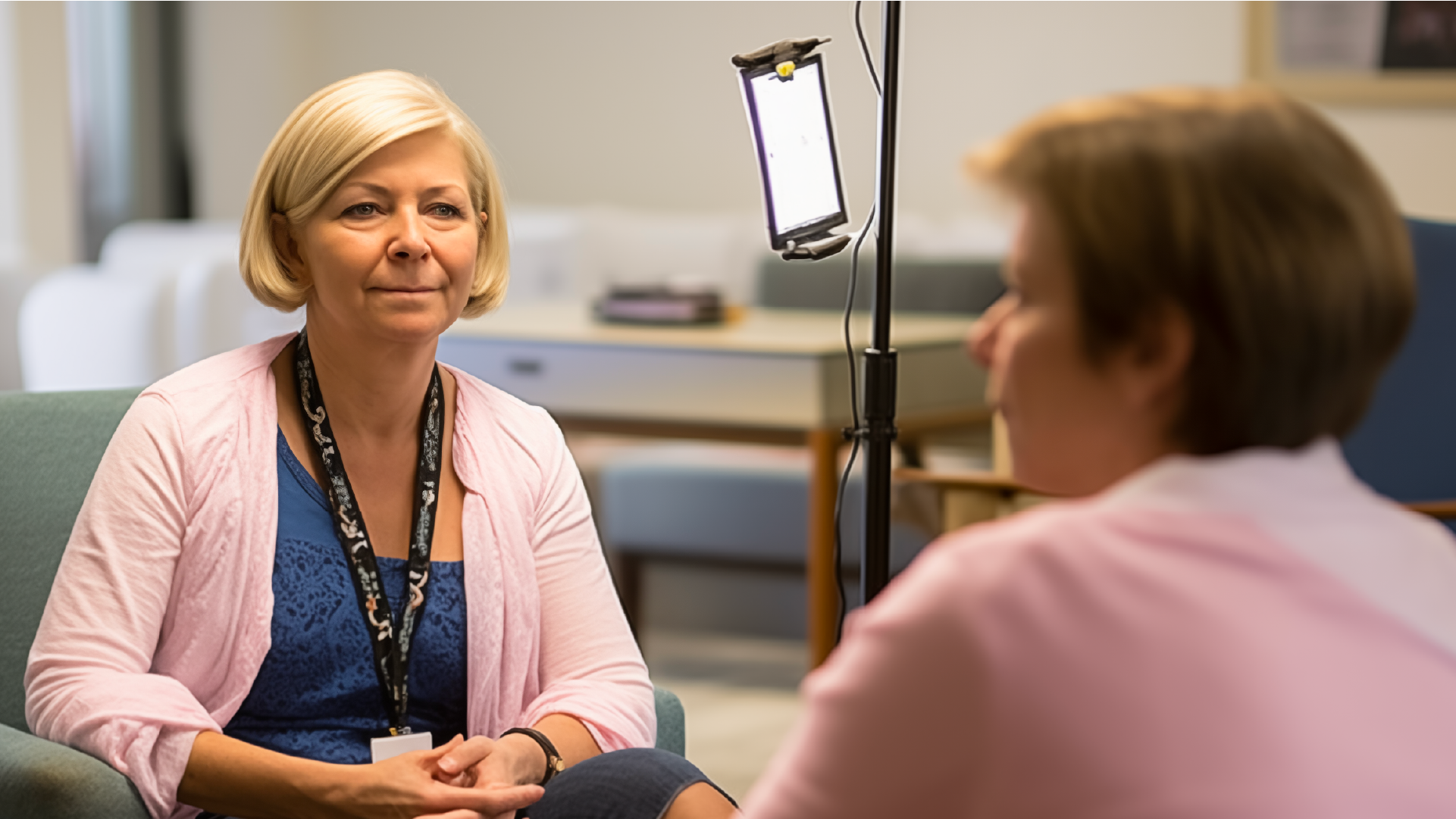
- Narrative Analysis
Narrative analysis focuses on interpreting and understanding the stories and personal narratives shared by individuals. Researchers analyze the structure, content, and meaning of these narratives to gain insights into how individuals make sense of their experiences, construct identities, and communicate their perspectives.
Through narrative analysis techniques, qualitative researchers explore the plot, characters, setting, and themes within a narrative. They examine how the narrator constructs meaning, conveys emotions, and positions themselves within the story.
This same narrative analysis method is often used in psychology, sociology, and anthropology to understand identity formation, life histories, and personal narratives. It can be used in a business setting to analyze long-form responses and user interviews or descriptions of user behavior.
For instance, in a study on the experiences of cancer survivors, researchers may conduct narrative analysis on interviews with survivors. By examining the narratives, researchers can identify common themes such as coping strategies, support systems, and personal growth.
This qualitative analysis process can provide valuable insights into the lived experiences of cancer survivors and inform interventions and support programs.
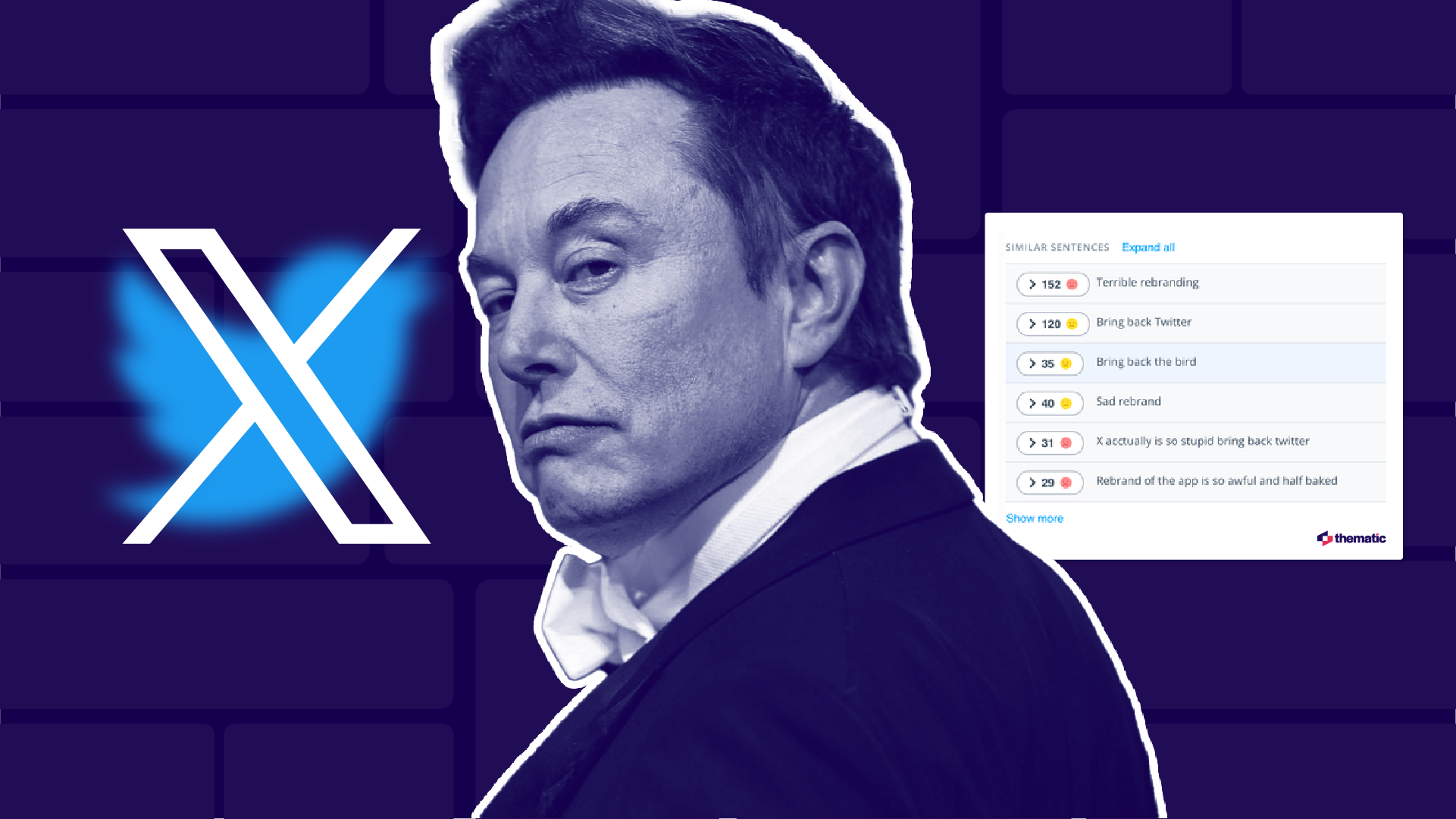
- Discourse Analysis
Discourse analysis examines the social, cultural, and power relations that shape language use in different contexts. It focuses on the ways in which language constructs and reflects social reality, identities, and ideologies.
Researchers employing discourse analysis analyze data that includes spoken or written language, including interviews, speeches, media articles, and conversations.
They examine linguistic features such as metaphors, power dynamics, framing, and silences to uncover underlying social structures and processes.
For example, in a study on gender representation in media, researchers may use discourse analysis to analyze television advertisements. By examining the language, visual cues, and narratives used in the advertisements, researchers can identify how gender roles and stereotypes are constructed and reinforced.
It can shed light on the ways in which media perpetuates or challenges societal norms and expectations.
Another example might be using discourse analysis to analyze Tik Tok and YouTube videos to understand the societal responses to a rebranding; for instance, that from Twitter to X. Customer interviews are another good source for this analysis method.
- Grounded Theory
Grounded theory is an approach to qualitative analysis that aims to develop theories and concepts grounded in data. It involves iterative data collection and analysis to develop an inductive theory that emerges from the unstructured data itself.
Researchers using grounded theory analyze interviews, observations, and textual data to generate concepts and categories.
These concepts are continually refined and developed through theoretical sampling and constant comparison. Grounded theory analysis is particularly useful when exploring complex social phenomena where existing theories may be limited.
For instance, in a study on the experiences of individuals living with chronic pain, researchers may use grounded theory to analyze interviews with participants. Through iterative analysis, researchers can identify key concepts such as pain management strategies, social support networks, and psychological coping mechanisms.
These concepts can then be used to develop a theoretical framework within grounded theory that captures the multidimensional nature of living with chronic pain.
Although historically grounded theory analysis has been primarily used in the social sciences, grounded theory has also been used successfully for business inquiry.
- Thematic Analysis
Thematic analysis is a widely used method in qualitative data analysis that involves identifying, analyzing, and reporting patterns or themes within data. It is a flexible approach that can be applied across a variety of qualitative data, such as interview transcripts, survey responses, and observational notes.
When thematic analysis is done manually, researchers initially familiarize themselves with the raw data, reading through the material multiple times to gain a deep understanding.
Following this, they begin manual coding. The first step is to generate initial codes, which are tags or labels that identify important features of the data relevant to the research question.
These codes are then collated into potential themes, which are broader patterns that emerge across the data set.
Each theme is then reviewed and refined to ensure it accurately represents the coded data and the overall data set. The final step involves defining and naming the themes, during which researchers provide detailed analysis, including how themes relate to each other and to the research question.
Sound complicated? The great news is that advances in artificial intelligence mean we no longer have to do all that by hand.
Thematic analysis software can process thousands of pieces of consumer feedback in a matter of minutes, providing a user-friendly view of the themes and trends in the customer data pool.
What’s more, this type of software can be programmed to do content analysis, discourse analysis, and narrative analysis at the same time. The best comprehensive business solution for thematic analysis today is Thematic; a comprehensive feedback analysis that is designed for customer-centric businesses. It makes qualitative user analysis accessible to anyone, and is able to process feedback at scale.
Across disciplines, thematic analysis is particularly valued for its ability to provide a rich and detailed, yet complex account of data. It's a method that is accessible to researchers across different levels of qualitative research experience and can be applied to a variety of theoretical and epistemological approaches, making it a versatile tool in qualitative work.
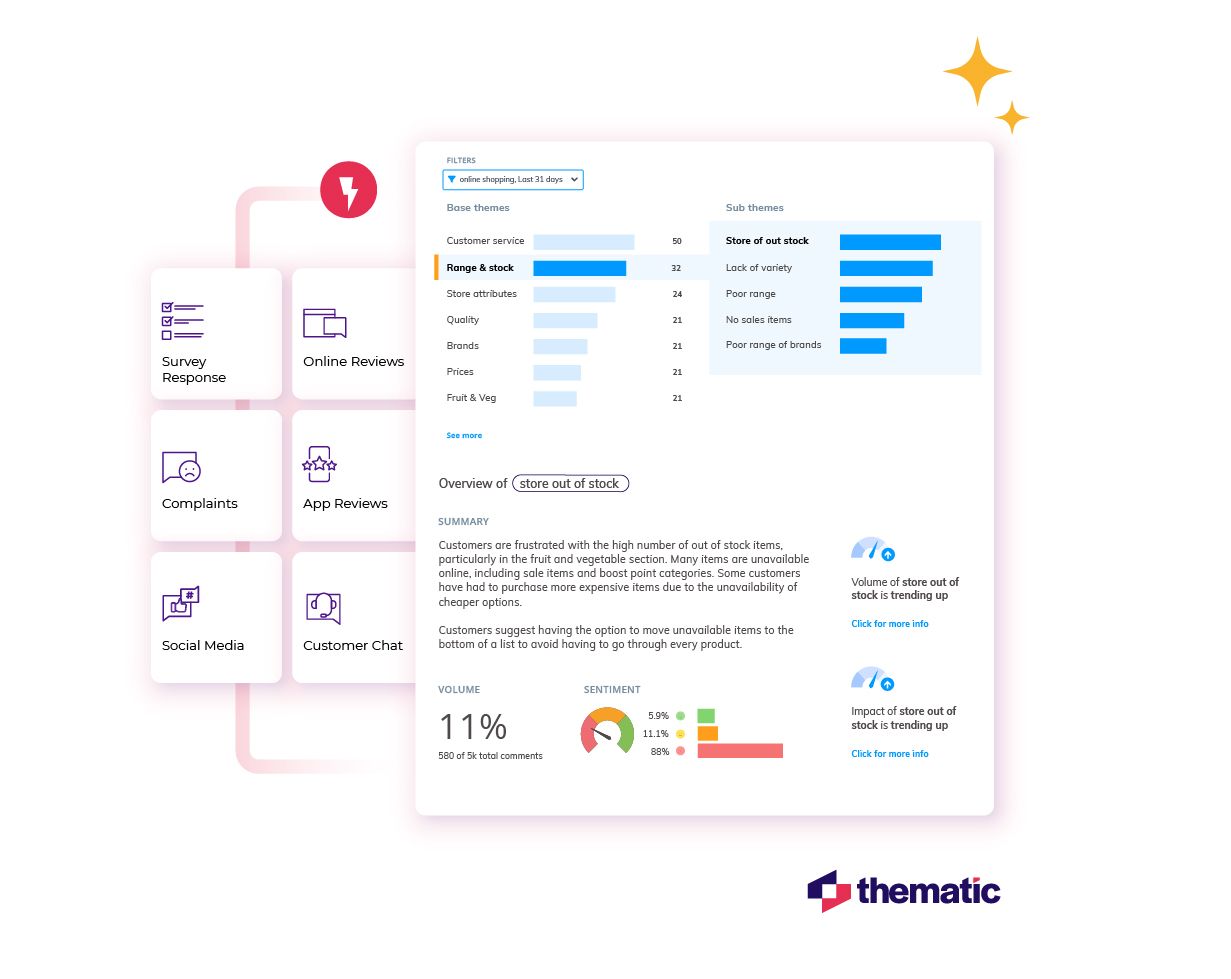
Steps in Qualitative Data Analysis
Data collection.
Data collection is the initial phase of qualitative research and data analysis. It involves selecting appropriate methods to gather data such as interviews, observations, focus groups, or archival research.
Researchers may employ various techniques to collect data. These can include developing interview protocols, conducting observations, or collecting data using audio-visual recording devices.
They may need to consider ethical considerations, ensure informed consent, and establish rapport with participants to obtain rich and reliable data. The goal is to gather qualitative data that is relevant, comprehensive, and representative of the research topic.
Qualitative research questions can be more open-ended than those used for gathering quantitative data, and the research findings have the potential to be far more extensive.
In a business context, much of the work is done for you by customers who provide feedback in reviews, on support tickets, and on social media. Customer interviews are another possible source of rich data.
Data Coding
Data coding is the process of categorizing and organizing qualitative data into meaningful segments. When this is done manually, researchers assign codes to different parts of the data based on the emerging patterns, themes, or concepts identified during analysis. This coding process helps researchers manage and make sense of large amounts of qualitative data.
There are different types of codes used in analyzing raw data, including descriptive codes, interpretive codes, and conceptual codes.
Descriptive codes capture the content and surface-level meaning of all the data, while interpretive codes delve deeper into the underlying meanings and interpretations. A conceptual coding system further abstracts the research data by identifying broader concepts or theories.
Data Interpretation
Data interpretation involves making sense of the coded data and exploring the relationships, themes, and patterns that emerge from the analysis. Researchers critically examine the data, compare different codes, and then identify themes and connections between categories and concepts.
During data interpretation, researchers may engage in constant comparison, where they continually compare new data to existing codes and categories. This iterative process helps refine the analysis and identify theoretical insights.
It involves synthesizing the findings of qualitative and quantitative data and crafting a narrative that presents a comprehensive understanding of the research phenomenon.
Both data coding and data interpretation can be done by your qualitative analytics software, either in a research or business setting. In a corporate setting, CX /PX teams and customer service can then use information gained through the data interpretation step to drive favourable outcomes.
Performing Qualitative Data Analysis with Generative AI and LLM
Running manual grounded theory analysis or content analysis on a large amount of consumer feedback has never been a practical option. But that doesn’t mean qualitative research doesn’t make sense in a business context.
Generative AI, based on large language models (LLMs) can work with qualitative data at scale, analyze it, and derive the themes, connections and insights that can inform business decisions.
An LLM is a powerful machine learning model, based on deep learning and neural networks. It’s able to process and identify the complex relationships in natural language, and it can also understand user questions and moods and even generate text.
A natural languague processing LLM, trained on huge amounts of text data, could do all the work of a QDA researcher with the added benefits of easily verifiable, repeatable results.
Companies with extensive in-house talent may be able to build an in-house AI engine to analyze customer feedback and make sense of it— on a small scale. Those who are serious about getting real insights, though, will want to go with professional tools that have been trained on massive amounts of data and give reliable, dependable results.
Thematic is probably the best example of such a tool. Built to make sense of any amount of feedback data, it works in a highly transparent way that will leave you confident in every insight you derive.
It’s also incredibly user-friendly, with helpful visualizations and an easy-to-use dashboard that enables you to keep constant tabs on exactly what your users feel about the company. It’s never been easier to transform your user experience.
Modern Methods of Qualitative Data Analysis in Action: A Case Study
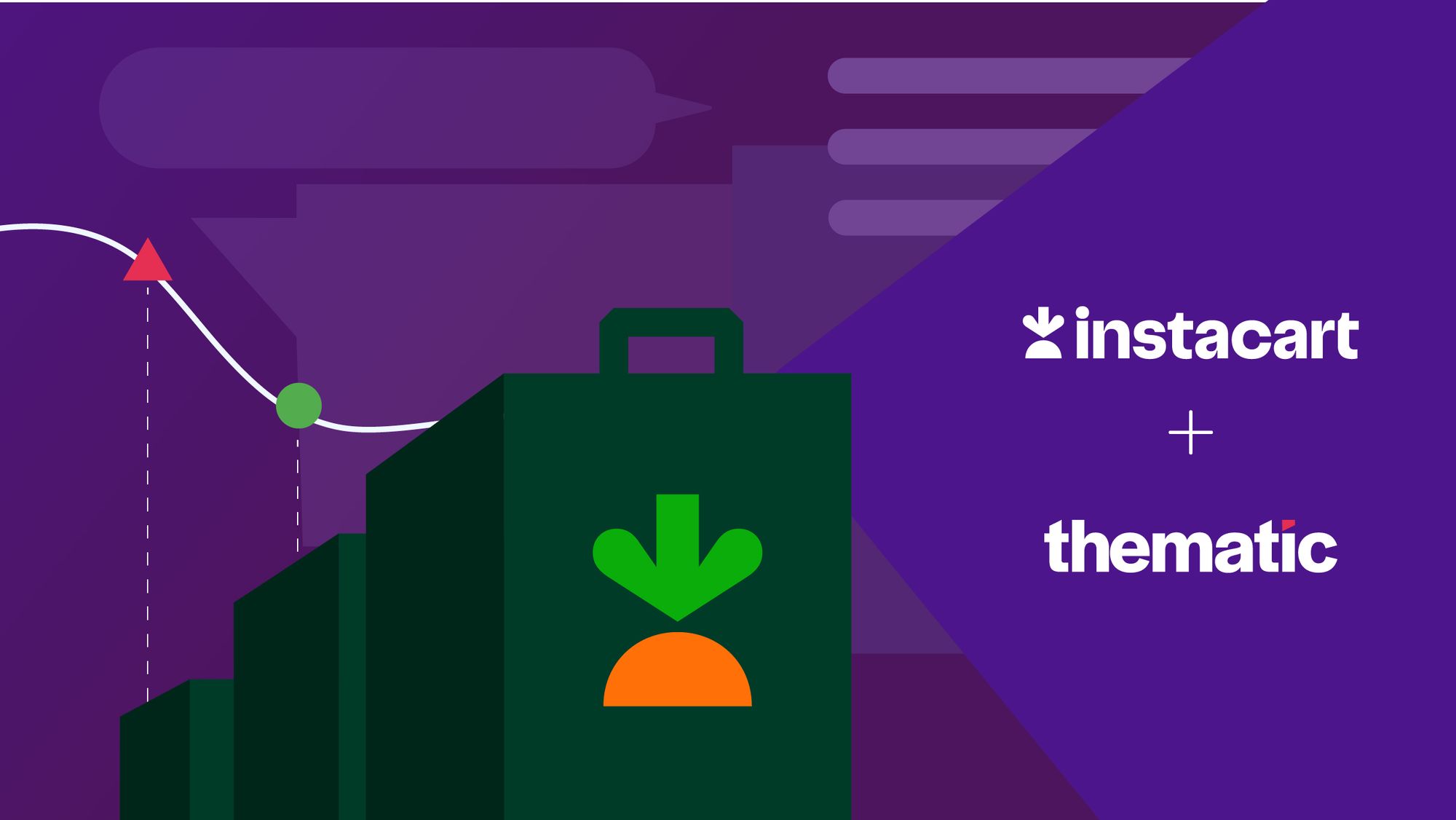
Instacart is one example of a company that discovered the power of qualitative data analysis. This company has 10 million end users, 500,000 personal shoppers, and more than 40,000 retailers. Processing all this qualitative data the traditional way would have been impossible, but Ant Marty, product operations team manager, found a method that worked.
Plugging data from the app into Thematic, she got real time information on everything happening among those millions of users: trends, themes, and deep understanding of what mattered to the people who made the company run.
Data collection is easy when you have an app with numerous feedback collection options. Data coding is automated by Thematic. And Thematic makes the first move in interpretation as well, providing insights that can be transformed by product teams into action plans and even a long-term vision.
Challenges Facing Qualitative Data Analysis Methods
Ensuring data validity and reliability.
One of the main challenges to a qualitative approach is ensuring the validity and reliability of the findings. Validity refers to the accuracy, truthfulness, and credibility of the data collected and analysis, while reliability refers to the consistency and replicability of the research process and findings.
Researchers address these challenges by employing rigorous data collection methods, ensuring data saturation, conducting member checks, and establishing inter-rater reliability. They also maintain reflexivity by critically reflecting on their assumptions, biases, and interpretations throughout the analysis process.
If you are a business using software to conduct qualitative research, your data validation check may be somewhat different, but it’s just as important. Some software, like Thematic, has validation built in, and the whole process is so transparent you can easily check and double-check where each insight comes from .
With other software options, you may have to run manual checks to ensure every piece of information provided has a firm basis.
Dealing with Subjectivity and Bias
Subjectivity and bias used to be considered inherent to qualitative research methods due to the interpretive nature of the process. Researchers bring their own perspectives, beliefs, and experiences, which can influence the analysis and interpretations.
To mitigate subjectivity and bias, researchers maintain transparency in their analytical processes by documenting their decision-making, providing detailed justifications for their interpretations, and engaging in peer debriefing and member checking. Using multiple researchers or an expert panel can also increase the credibility and reliability of the analysis.
Another way to decrease subjectivity is through thematic analysis software, which produces results that are repeatable and verifiable.
When it is all said and done, qualitative analysis offers a powerful and nuanced examination of human experiences and social phenomena. By employing diverse methods, adhering to key principles, and addressing potential limitations, researchers can harness the full potential of qualitative data to uncover rich insights and contribute to the advancement of knowledge.
Benefits of Qualitative Data Analysis Methods
Rich, in-depth insights.
A primary benefit of qualitative research techniques is their ability to provide rich, in-depth insights into complex phenomena. These methods delve deeply into human experiences, emotions, beliefs, and behaviors, offering a comprehensive understanding that is often unattainable through quantitative methods.
By exploring the nuances and subtleties of social interactions and personal experiences, qualitative analysis can uncover the layers of meaning that underpin human behavior. This depth of understanding is particularly valuable in fields like psychology, sociology, and anthropology, where the intricacies of human experience are central to the research question.
It is even more important for customer-focused businesses and enables them to create a product and a CX that meets their customer’s needs and desires. Quantitative analysis can provide a one-dimensional understanding of user behavior based on quantitative data, but when analysing qualitative data you get the why to every what.
Flexibility and Contextual Understanding
Another significant advantage of these analysis techniques is their inherent flexibility and capacity to provide contextual understanding. Unlike quantitative research, which relies on rigid structures and predefined hypotheses, qualitative research is adaptable to the evolving nature of the study.
This flexibility allows researchers to explore unexpected themes and patterns that emerge during the data collection process. Qualitative analysis is how businesses like Atlassian have created infinite customer feedback loops and powered their own infinitely evolving products.
Additionally, qualitative methods are sensitive to the context in which the data is collected, acknowledging and incorporating the environmental, cultural, and social factors that influence the data. The context-rich approach used to collect qualitative data ensures a more holistic understanding of the subject matter, making it particularly useful in cross-cultural studies, community research, and exploratory investigations.
Your product may have global reach, and users in different areas may interact with it in different ways– but qualitative techniques can take all that into account.
This considered, it should be no surprise that qualitative analysis techniques have become powerful tools for researchers seeking to understand the complexities of human behavior and social phenomena. Their ability to provide depth, context, and rich narrative data makes them indispensable tools in the arsenal of social science research, and there’s no better way to gain solid information to guide your business decisions.
Whether you’re a researcher keen on analyzing and interpreting qualitative data or an entrepreneur keen on making your business more customer-centric, this research method is likely to become your next best friend.
If you’re in academia, you may want to do it all manually, and that’s totally okay. But if it’s business intelligence you’re after— try out Thematic. Your future self will thank you, as will everyone else who views the end-of-year reports.
What are the five methods to analyze qualitative data?
The five chief methods of qualitative data analysis are:
The right analysis method for your use case will depend on what context, your research questions, and the form of data available to you.
What are good sources of data for qualitative data analysis?
In a business context user reviews, support tickets, customer surveys and social media posts are all great sources of data for qualitative analysis. In a research project, gathering qualitative data may mean conducting interviews, surveys, or focus groups.
What are the benefits of qualitative data analysis?
Two big benefits of qualitative data analysis include:
- Rich, in-depth insights
- Flexibility and contextual understanding
In a business context, this translates into a loyal, well-satisfied user base, a successful product, and an upwards-ticking revenue curve. Research objectives for social sciences may include a better understanding of social dynamics or human relations.
What are the challenges of qualitative data analysis?
The two prime challenges of qualitative data analysis techniques are:
- Ensuring data validity and reliability
- Dealing with subjectivity and bias
What is the best tool for qualitative data analysis?
While a number of other options do exist, the best comprehensive software for qualitative data analysis in a business context today is Thematic.
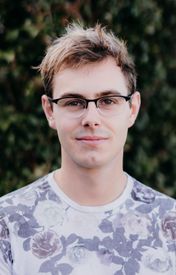
Digital Marketing
Tyler manages Thematic's content & aims to create useful & informative content for CX, Product & Insight professionals.
We make it easy to discover the customer and product issues that matter.
Unlock the value of feedback at scale, in one platform. Try it for free now!
- Questions to ask your Feedback Analytics vendor
- How to end customer churn for good
- Scalable analysis of NPS verbatims
- 5 Text analytics approaches
- How to calculate the ROI of CX
Our experts will show you how Thematic works, how to discover pain points and track the ROI of decisions. To access your free trial, book a personal demo today.
Recent posts
Become a qualitative theming pro! Creating a perfect code frame is hard, but thematic analysis software makes the process much easier.
Discover the power of thematic analysis to unlock insights from qualitative data. Learn about manual vs. AI-powered approaches, best practices, and how Thematic software can revolutionize your analysis workflow.
Customer feedback doesn't have all the answers. But it has critical insights for strategy and prioritization. Thematic is a B2B SaaS company. We aren't swimming in feedback. Every piece of feedback counts. Collecting and analyzing this feedback requires a different approach. We receive feedback from many places: * our in-product NPS
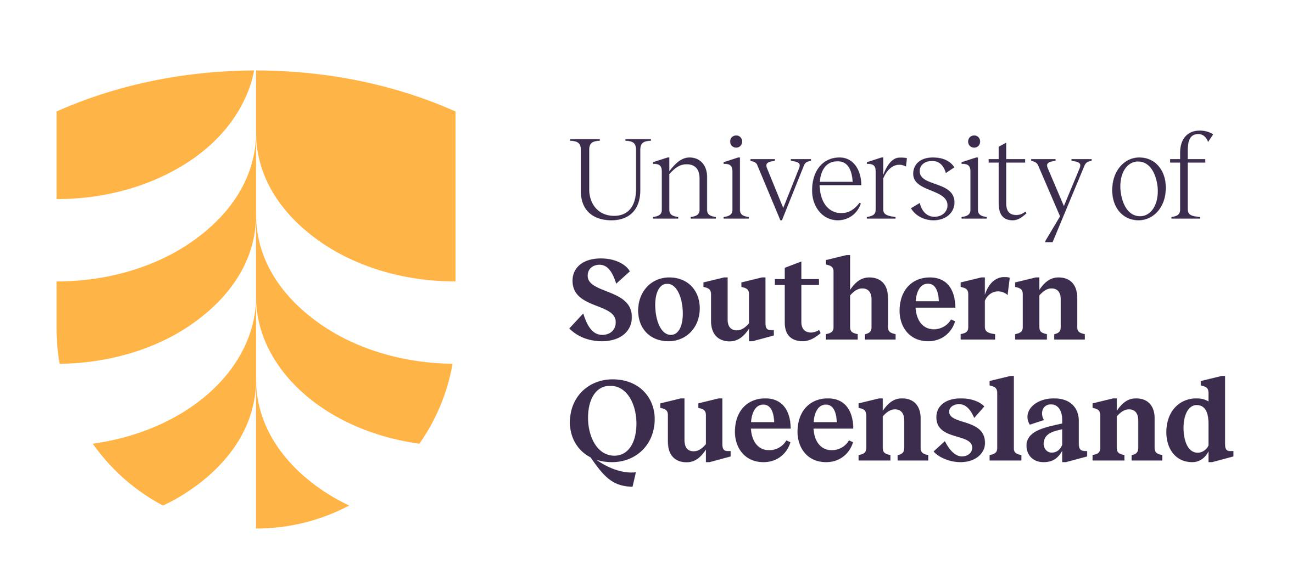
Want to create or adapt books like this? Learn more about how Pressbooks supports open publishing practices.
13 Qualitative analysis
Qualitative analysis is the analysis of qualitative data such as text data from interview transcripts. Unlike quantitative analysis, which is statistics driven and largely independent of the researcher, qualitative analysis is heavily dependent on the researcher’s analytic and integrative skills and personal knowledge of the social context where the data is collected. The emphasis in qualitative analysis is ‘sense making’ or understanding a phenomenon, rather than predicting or explaining. A creative and investigative mindset is needed for qualitative analysis, based on an ethically enlightened and participant-in-context attitude, and a set of analytic strategies. This chapter provides a brief overview of some of these qualitative analysis strategies. Interested readers are referred to more authoritative and detailed references such as Miles and Huberman’s (1984) [1] seminal book on this topic.
Grounded theory
How can you analyse a vast set of qualitative data acquired through participant observation, in-depth interviews, focus groups, narratives of audio/video recordings, or secondary documents? One of these techniques for analysing text data is grounded theory —an inductive technique of interpreting recorded data about a social phenomenon to build theories about that phenomenon. The technique was developed by Glaser and Strauss (1967) [2] in their method of constant comparative analysis of grounded theory research, and further refined by Strauss and Corbin (1990) [3] to further illustrate specific coding techniques—a process of classifying and categorising text data segments into a set of codes (concepts), categories (constructs), and relationships. The interpretations are ‘grounded in’ (or based on) observed empirical data, hence the name. To ensure that the theory is based solely on observed evidence, the grounded theory approach requires that researchers suspend any pre-existing theoretical expectations or biases before data analysis, and let the data dictate the formulation of the theory.
Strauss and Corbin (1998) describe three coding techniques for analysing text data: open, axial, and selective. Open coding is a process aimed at identifying concepts or key ideas that are hidden within textual data, which are potentially related to the phenomenon of interest. The researcher examines the raw textual data line by line to identify discrete events, incidents, ideas, actions, perceptions, and interactions of relevance that are coded as concepts (hence called in vivo codes ). Each concept is linked to specific portions of the text (coding unit) for later validation. Some concepts may be simple, clear, and unambiguous, while others may be complex, ambiguous, and viewed differently by different participants. The coding unit may vary with the concepts being extracted. Simple concepts such as ‘organisational size’ may include just a few words of text, while complex ones such as ‘organizational mission’ may span several pages. Concepts can be named using the researcher’s own naming convention, or standardised labels taken from the research literature. Once a basic set of concepts are identified, these concepts can then be used to code the remainder of the data, while simultaneously looking for new concepts and refining old concepts. While coding, it is important to identify the recognisable characteristics of each concept, such as its size, colour, or level—e.g., high or low—so that similar concepts can be grouped together later . This coding technique is called ‘open’ because the researcher is open to and actively seeking new concepts relevant to the phenomenon of interest.
Next, similar concepts are grouped into higher order categories . While concepts may be context-specific, categories tend to be broad and generalisable, and ultimately evolve into constructs in a grounded theory. Categories are needed to reduce the amount of concepts the researcher must work with and to build a ‘big picture’ of the issues salient to understanding a social phenomenon. Categorisation can be done in phases, by combining concepts into subcategories, and then subcategories into higher order categories. Constructs from the existing literature can be used to name these categories, particularly if the goal of the research is to extend current theories. However, caution must be taken while using existing constructs, as such constructs may bring with them commonly held beliefs and biases. For each category, its characteristics (or properties) and the dimensions of each characteristic should be identified. The dimension represents a value of a characteristic along a continuum. For example, a ‘communication media’ category may have a characteristic called ‘speed’, which can be dimensionalised as fast, medium, or slow . Such categorisation helps differentiate between different kinds of communication media, and enables researchers to identify patterns in the data, such as which communication media is used for which types of tasks.
The second phase of grounded theory is axial coding , where the categories and subcategories are assembled into causal relationships or hypotheses that can tentatively explain the phenomenon of interest. Although distinct from open coding, axial coding can be performed simultaneously with open coding. The relationships between categories may be clearly evident in the data, or may be more subtle and implicit. In the latter instance, researchers may use a coding scheme (often called a ‘coding paradigm’, but different from the paradigms discussed in Chapter 3) to understand which categories represent conditions (the circumstances in which the phenomenon is embedded), actions/interactions (the responses of individuals to events under these conditions), and consequences (the outcomes of actions/interactions). As conditions, actions/interactions, and consequences are identified, theoretical propositions start to emerge, and researchers can start explaining why a phenomenon occurs, under what conditions, and with what consequences.
The third and final phase of grounded theory is selective coding , which involves identifying a central category or a core variable, and systematically and logically relating this central category to other categories. The central category can evolve from existing categories or can be a higher order category that subsumes previously coded categories. New data is selectively sampled to validate the central category, and its relationships to other categories—i.e., the tentative theory. Selective coding limits the range of analysis, and makes it move fast. At the same time, the coder must watch out for other categories that may emerge from the new data that could be related to the phenomenon of interest (open coding), which may lead to further refinement of the initial theory. Hence, open, axial, and selective coding may proceed simultaneously. Coding of new data and theory refinement continues until theoretical saturation is reached—i.e., when additional data does not yield any marginal change in the core categories or the relationships.
The ‘constant comparison’ process implies continuous rearrangement, aggregation, and refinement of categories, relationships, and interpretations based on increasing depth of understanding, and an iterative interplay of four stages of activities: comparing incidents/texts assigned to each category to validate the category), integrating categories and their properties, delimiting the theory by focusing on the core concepts and ignoring less relevant concepts, and writing theory using techniques like memoing, storylining, and diagramming. Having a central category does not necessarily mean that all other categories can be integrated nicely around it. In order to identify key categories that are conditions, action/interactions, and consequences of the core category, Strauss and Corbin (1990) recommend several integration techniques, such as storylining, memoing, or concept mapping, which are discussed here. In storylining , categories and relationships are used to explicate and/or refine a story of the observed phenomenon. Memos are theorised write-ups of ideas about substantive concepts and their theoretically coded relationships as they evolve during ground theory analysis, and are important tools to keep track of and refine ideas that develop during the analysis. Memoing is the process of using these memos to discover patterns and relationships between categories using two-by-two tables, diagrams, or figures, or other illustrative displays. Concept mapping is a graphical representation of concepts and relationships between those concepts—e.g., using boxes and arrows. The major concepts are typically laid out on one or more sheets of paper, blackboards, or using graphical software programs, linked to each other using arrows, and readjusted to best fit the observed data.
After a grounded theory is generated, it must be refined for internal consistency and logic. Researchers must ensure that the central construct has the stated characteristics and dimensions, and if not, the data analysis may be repeated. Researcher must then ensure that the characteristics and dimensions of all categories show variation. For example, if behaviour frequency is one such category, then the data must provide evidence of both frequent performers and infrequent performers of the focal behaviour. Finally, the theory must be validated by comparing it with raw data. If the theory contradicts with observed evidence, the coding process may need to be repeated to reconcile such contradictions or unexplained variations.
Content analysis
Content analysis is the systematic analysis of the content of a text—e.g., who says what, to whom, why, and to what extent and with what effect—in a quantitative or qualitative manner. Content analysis is typically conducted as follows. First, when there are many texts to analyse—e.g., newspaper stories, financial reports, blog postings, online reviews, etc.—the researcher begins by sampling a selected set of texts from the population of texts for analysis. This process is not random, but instead, texts that have more pertinent content should be chosen selectively. Second, the researcher identifies and applies rules to divide each text into segments or ‘chunks’ that can be treated as separate units of analysis. This process is called unitising . For example, assumptions, effects, enablers, and barriers in texts may constitute such units. Third, the researcher constructs and applies one or more concepts to each unitised text segment in a process called coding . For coding purposes, a coding scheme is used based on the themes the researcher is searching for or uncovers as they classify the text. Finally, the coded data is analysed, often both quantitatively and qualitatively, to determine which themes occur most frequently, in what contexts, and how they are related to each other.
A simple type of content analysis is sentiment analysis —a technique used to capture people’s opinion or attitude toward an object, person, or phenomenon. Reading online messages about a political candidate posted on an online forum and classifying each message as positive, negative, or neutral is an example of such an analysis. In this case, each message represents one unit of analysis. This analysis will help identify whether the sample as a whole is positively or negatively disposed, or neutral towards that candidate. Examining the content of online reviews in a similar manner is another example. Though this analysis can be done manually, for very large datasets—e.g., millions of text records—natural language processing and text analytics based software programs are available to automate the coding process, and maintain a record of how people’s sentiments fluctuate with time.
A frequent criticism of content analysis is that it lacks a set of systematic procedures that would allow the analysis to be replicated by other researchers. Schilling (2006) [4] addressed this criticism by organising different content analytic procedures into a spiral model. This model consists of five levels or phases in interpreting text: convert recorded tapes into raw text data or transcripts for content analysis, convert raw data into condensed protocols, convert condensed protocols into a preliminary category system, use the preliminary category system to generate coded protocols, and analyse coded protocols to generate interpretations about the phenomenon of interest.
Content analysis has several limitations. First, the coding process is restricted to the information available in text form. For instance, if a researcher is interested in studying people’s views on capital punishment, but no such archive of text documents is available, then the analysis cannot be done. Second, sampling must be done carefully to avoid sampling bias. For instance, if your population is the published research literature on a given topic, then you have systematically omitted unpublished research or the most recent work that is yet to be published.
Hermeneutic analysis
Hermeneutic analysis is a special type of content analysis where the researcher tries to ‘interpret’ the subjective meaning of a given text within its sociohistoric context. Unlike grounded theory or content analysis—which ignores the context and meaning of text documents during the coding process—hermeneutic analysis is a truly interpretive technique for analysing qualitative data. This method assumes that written texts narrate an author’s experience within a sociohistoric context, and should be interpreted as such within that context. Therefore, the researcher continually iterates between singular interpretation of the text (the part) and a holistic understanding of the context (the whole) to develop a fuller understanding of the phenomenon in its situated context, which German philosopher Martin Heidegger called the hermeneutic circle . The word hermeneutic (singular) refers to one particular method or strand of interpretation.
More generally, hermeneutics is the study of interpretation and the theory and practice of interpretation. Derived from religious studies and linguistics, traditional hermeneutics—such as biblical hermeneutics —refers to the interpretation of written texts, especially in the areas of literature, religion and law—such as the Bible. In the twentieth century, Heidegger suggested that a more direct, non-mediated, and authentic way of understanding social reality is to experience it, rather than simply observe it, and proposed philosophical hermeneutics , where the focus shifted from interpretation to existential understanding. Heidegger argued that texts are the means by which readers can not only read about an author’s experience, but also relive the author’s experiences. Contemporary or modern hermeneutics, developed by Heidegger’s students such as Hans-Georg Gadamer, further examined the limits of written texts for communicating social experiences, and went on to propose a framework of the interpretive process, encompassing all forms of communication, including written, verbal, and non-verbal, and exploring issues that restrict the communicative ability of written texts, such as presuppositions, language structures (e.g., grammar, syntax, etc.), and semiotics—the study of written signs such as symbolism, metaphor, analogy, and sarcasm. The term hermeneutics is sometimes used interchangeably and inaccurately with exegesis , which refers to the interpretation or critical explanation of written text only, and especially religious texts.
Finally, standard software programs, such as ATLAS.ti.5, NVivo, and QDA Miner, can be used to automate coding processes in qualitative research methods. These programs can quickly and efficiently organise, search, sort, and process large volumes of text data using user-defined rules. To guide such automated analysis, a coding schema should be created, specifying the keywords or codes to search for in the text, based on an initial manual examination of sample text data. The schema can be arranged in a hierarchical manner to organise codes into higher-order codes or constructs. The coding schema should be validated using a different sample of texts for accuracy and adequacy. However, if the coding schema is biased or incorrect, the resulting analysis of the entire population of texts may be flawed and non-interpretable. However, software programs cannot decipher the meaning behind certain words or phrases or the context within which these words or phrases are used—such sarcasm or metaphors—which may lead to significant misinterpretation in large scale qualitative analysis.
- Miles, M. B., & Huberman, A. M. (1984). Qualitative data analysis: A sourcebook of new methods . Newbury Park, CA: Sage Publications. ↵
- Glaser, B., & Strauss, A. (1967). The discovery of grounded theory: Strategies for qualitative research . New York: Aldine Pub Co. ↵
- Strauss, A., & Corbin, J. (1990). Basics of qualitative research: Grounded theory procedures and techniques , Beverly Hills: Sage Publications. ↵
- Schiling, J. (2006). On the pragmatics of qualitative assessment: Designing the process for content analysis. European Journal of Psychological Assessment , 22(1), 28–37. ↵
Social Science Research: Principles, Methods and Practices (Revised edition) Copyright © 2019 by Anol Bhattacherjee is licensed under a Creative Commons Attribution-NonCommercial-ShareAlike 4.0 International License , except where otherwise noted.
Share This Book
Qualitative Research : Definition
Qualitative research is the naturalistic study of social meanings and processes, using interviews, observations, and the analysis of texts and images. In contrast to quantitative researchers, whose statistical methods enable broad generalizations about populations (for example, comparisons of the percentages of U.S. demographic groups who vote in particular ways), qualitative researchers use in-depth studies of the social world to analyze how and why groups think and act in particular ways (for instance, case studies of the experiences that shape political views).
Events and Workshops
- Introduction to NVivo Have you just collected your data and wondered what to do next? Come join us for an introductory session on utilizing NVivo to support your analytical process. This session will only cover features of the software and how to import your records. Please feel free to attend any of the following sessions below: April 25th, 2024 12:30 pm - 1:45 pm Green Library - SVA Conference Room 125 May 9th, 2024 12:30 pm - 1:45 pm Green Library - SVA Conference Room 125
- Next: Choose an approach >>
- Choose an approach
- Find studies
- Learn methods
- Getting Started
- Get software
- Get data for secondary analysis
- Network with researchers

- Last Updated: Aug 9, 2024 2:09 PM
- URL: https://guides.library.stanford.edu/qualitative_research
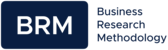
Qualitative Data Analysis
Qualitative data refers to non-numeric information such as interview transcripts, notes, video and audio recordings, images and text documents. Qualitative data analysis can be divided into the following five categories:
1. Content analysis . This refers to the process of categorizing verbal or behavioural data to classify, summarize and tabulate the data.
2. Narrative analysis . This method involves the reformulation of stories presented by respondents taking into account context of each case and different experiences of each respondent. In other words, narrative analysis is the revision of primary qualitative data by researcher.
3. Discourse analysis . A method of analysis of naturally occurring talk and all types of written text.
4. Framework analysis . This is more advanced method that consists of several stages such as familiarization, identifying a thematic framework, coding, charting, mapping and interpretation.
5. Grounded theory . This method of qualitative data analysis starts with an analysis of a single case to formulate a theory. Then, additional cases are examined to see if they contribute to the theory.
Qualitative data analysis can be conducted through the following three steps:
Step 1: Developing and Applying Codes . Coding can be explained as categorization of data. A ‘code’ can be a word or a short phrase that represents a theme or an idea. All codes need to be assigned meaningful titles. A wide range of non-quantifiable elements such as events, behaviours, activities, meanings etc. can be coded.
There are three types of coding:
- Open coding . The initial organization of raw data to try to make sense of it.
- Axial coding . Interconnecting and linking the categories of codes.
- Selective coding . Formulating the story through connecting the categories.
Coding can be done manually or using qualitative data analysis software such as
NVivo, Atlas ti 6.0, HyperRESEARCH 2.8, Max QDA and others.
When using manual coding you can use folders, filing cabinets, wallets etc. to gather together materials that are examples of similar themes or analytic ideas. Manual method of coding in qualitative data analysis is rightly considered as labour-intensive, time-consuming and outdated.
In computer-based coding, on the other hand, physical files and cabinets are replaced with computer based directories and files. When choosing software for qualitative data analysis you need to consider a wide range of factors such as the type and amount of data you need to analyse, time required to master the software and cost considerations.
Moreover, it is important to get confirmation from your dissertation supervisor prior to application of any specific qualitative data analysis software.
The following table contains examples of research titles, elements to be coded and identification of relevant codes:
Born or bred: revising The Great Man theory of leadership in the 21 century | Leadership practice | Born leaders Made leaders Leadership effectiveness |
A study into advantages and disadvantages of various entry strategies to Chinese market
|
Market entry strategies | Wholly-owned subsidiaries Joint-ventures Franchising Exporting Licensing |
Impacts of CSR programs and initiative on brand image: a case study of Coca-Cola Company UK. |
Activities, phenomenon | Philanthropy Supporting charitable courses Ethical behaviour Brand awareness Brand value |
An investigation into the ways of customer relationship management in mobile marketing environment |
Tactics | Viral messages Customer retention Popularity of social networking sites |
Qualitative data coding
Step 2: Identifying themes, patterns and relationships . Unlike quantitative methods , in qualitative data analysis there are no universally applicable techniques that can be applied to generate findings. Analytical and critical thinking skills of researcher plays significant role in data analysis in qualitative studies. Therefore, no qualitative study can be repeated to generate the same results.
Nevertheless, there is a set of techniques that you can use to identify common themes, patterns and relationships within responses of sample group members in relation to codes that have been specified in the previous stage.
Specifically, the most popular and effective methods of qualitative data interpretation include the following:
- Word and phrase repetitions – scanning primary data for words and phrases most commonly used by respondents, as well as, words and phrases used with unusual emotions;
- Primary and secondary data comparisons – comparing the findings of interview/focus group/observation/any other qualitative data collection method with the findings of literature review and discussing differences between them;
- Search for missing information – discussions about which aspects of the issue was not mentioned by respondents, although you expected them to be mentioned;
- Metaphors and analogues – comparing primary research findings to phenomena from a different area and discussing similarities and differences.
Step 3: Summarizing the data . At this last stage you need to link research findings to hypotheses or research aim and objectives. When writing data analysis chapter, you can use noteworthy quotations from the transcript in order to highlight major themes within findings and possible contradictions.
It is important to note that the process of qualitative data analysis described above is general and different types of qualitative studies may require slightly different methods of data analysis.
My e-book, The Ultimate Guide to Writing a Dissertation in Business Studies: a step by step approach contains a detailed, yet simple explanation of qualitative data analysis methods . The e-book explains all stages of the research process starting from the selection of the research area to writing personal reflection. Important elements of dissertations such as research philosophy, research approach, research design, methods of data collection and data analysis are explained in simple words. John Dudovskiy
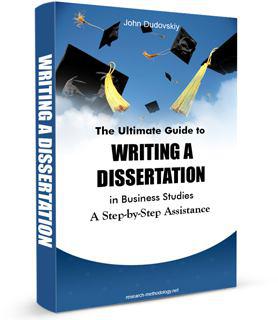
- Privacy Policy

Home » Qualitative Data – Types, Methods and Examples
Qualitative Data – Types, Methods and Examples
Table of Contents


Qualitative Data
Definition:
Qualitative data is a type of data that is collected and analyzed in a non-numerical form, such as words, images, or observations. It is generally used to gain an in-depth understanding of complex phenomena, such as human behavior, attitudes, and beliefs.
Types of Qualitative Data
There are various types of qualitative data that can be collected and analyzed, including:
- Interviews : These involve in-depth, face-to-face conversations with individuals or groups to gather their perspectives, experiences, and opinions on a particular topic.
- Focus Groups: These are group discussions where a facilitator leads a discussion on a specific topic, allowing participants to share their views and experiences.
- Observations : These involve observing and recording the behavior and interactions of individuals or groups in a particular setting.
- Case Studies: These involve in-depth analysis of a particular individual, group, or organization, usually over an extended period.
- Document Analysis : This involves examining written or recorded materials, such as newspaper articles, diaries, or public records, to gain insight into a particular topic.
- Visual Data : This involves analyzing images or videos to understand people’s experiences or perspectives on a particular topic.
- Online Data: This involves analyzing data collected from social media platforms, forums, or online communities to understand people’s views and opinions on a particular topic.
Qualitative Data Formats
Qualitative data can be collected and presented in various formats. Some common formats include:
- Textual data: This includes written or transcribed data from interviews, focus groups, or observations. It can be analyzed using various techniques such as thematic analysis or content analysis.
- Audio data: This includes recordings of interviews or focus groups, which can be transcribed and analyzed using software such as NVivo.
- Visual data: This includes photographs, videos, or drawings, which can be analyzed using techniques such as visual analysis or semiotics.
- Mixed media data : This includes data collected in different formats, such as audio and text. This can be analyzed using mixed methods research, which combines both qualitative and quantitative research methods.
- Field notes: These are notes taken by researchers during observations, which can include descriptions of the setting, behaviors, and interactions of participants.
Qualitative Data Analysis Methods
Qualitative data analysis refers to the process of systematically analyzing and interpreting qualitative data to identify patterns, themes, and relationships. Here are some common methods of analyzing qualitative data:
- Thematic analysis: This involves identifying and analyzing patterns or themes within the data. It involves coding the data into themes and subthemes and organizing them into a coherent narrative.
- Content analysis: This involves analyzing the content of the data, such as the words, phrases, or images used. It involves identifying patterns and themes in the data and examining the relationships between them.
- Discourse analysis: This involves analyzing the language and communication used in the data, such as the meaning behind certain words or phrases. It involves examining how the language constructs and shapes social reality.
- Grounded theory: This involves developing a theory or framework based on the data. It involves identifying patterns and themes in the data and using them to develop a theory that explains the phenomenon being studied.
- Narrative analysis : This involves analyzing the stories and narratives present in the data. It involves examining how the stories are constructed and how they contribute to the overall understanding of the phenomenon being studied.
- Ethnographic analysis : This involves analyzing the culture and social practices present in the data. It involves examining how the cultural and social practices contribute to the phenomenon being studied.
Qualitative Data Collection Guide
Here are some steps to guide the collection of qualitative data:
- Define the research question : Start by clearly defining the research question that you want to answer. This will guide the selection of data collection methods and help to ensure that the data collected is relevant to the research question.
- Choose data collection methods : Select the most appropriate data collection methods based on the research question, the research design, and the resources available. Common methods include interviews, focus groups, observations, document analysis, and participatory research.
- Develop a data collection plan : Develop a plan for data collection that outlines the specific procedures, timelines, and resources needed for each data collection method. This plan should include details such as how to recruit participants, how to conduct interviews or focus groups, and how to record and store data.
- Obtain ethical approval : Obtain ethical approval from an institutional review board or ethics committee before beginning data collection. This is particularly important when working with human participants to ensure that their rights and interests are protected.
- Recruit participants: Recruit participants based on the research question and the data collection methods chosen. This may involve purposive sampling, snowball sampling, or random sampling.
- Collect data: Collect data using the chosen data collection methods. This may involve conducting interviews, facilitating focus groups, observing participants, or analyzing documents.
- Transcribe and store data : Transcribe and store the data in a secure location. This may involve transcribing audio or video recordings, organizing field notes, or scanning documents.
- Analyze data: Analyze the data using appropriate qualitative data analysis methods, such as thematic analysis or content analysis.
- I nterpret findings : Interpret the findings of the data analysis in the context of the research question and the relevant literature. This may involve developing new theories or frameworks, or validating existing ones.
- Communicate results: Communicate the results of the research in a clear and concise manner, using appropriate language and visual aids where necessary. This may involve writing a report, presenting at a conference, or publishing in a peer-reviewed journal.
Qualitative Data Examples
Some examples of qualitative data in different fields are as follows:
- Sociology : In sociology, qualitative data is used to study social phenomena such as culture, norms, and social relationships. For example, a researcher might conduct interviews with members of a community to understand their beliefs and practices.
- Psychology : In psychology, qualitative data is used to study human behavior, emotions, and attitudes. For example, a researcher might conduct a focus group to explore how individuals with anxiety cope with their symptoms.
- Education : In education, qualitative data is used to study learning processes and educational outcomes. For example, a researcher might conduct observations in a classroom to understand how students interact with each other and with their teacher.
- Marketing : In marketing, qualitative data is used to understand consumer behavior and preferences. For example, a researcher might conduct in-depth interviews with customers to understand their purchasing decisions.
- Anthropology : In anthropology, qualitative data is used to study human cultures and societies. For example, a researcher might conduct participant observation in a remote community to understand their customs and traditions.
- Health Sciences: In health sciences, qualitative data is used to study patient experiences, beliefs, and preferences. For example, a researcher might conduct interviews with cancer patients to understand how they cope with their illness.
Application of Qualitative Data
Qualitative data is used in a variety of fields and has numerous applications. Here are some common applications of qualitative data:
- Exploratory research: Qualitative data is often used in exploratory research to understand a new or unfamiliar topic. Researchers use qualitative data to generate hypotheses and develop a deeper understanding of the research question.
- Evaluation: Qualitative data is often used to evaluate programs or interventions. Researchers use qualitative data to understand the impact of a program or intervention on the people who participate in it.
- Needs assessment: Qualitative data is often used in needs assessments to understand the needs of a specific population. Researchers use qualitative data to identify the most pressing needs of the population and develop strategies to address those needs.
- Case studies: Qualitative data is often used in case studies to understand a particular case in detail. Researchers use qualitative data to understand the context, experiences, and perspectives of the people involved in the case.
- Market research: Qualitative data is often used in market research to understand consumer behavior and preferences. Researchers use qualitative data to gain insights into consumer attitudes, opinions, and motivations.
- Social and cultural research : Qualitative data is often used in social and cultural research to understand social phenomena such as culture, norms, and social relationships. Researchers use qualitative data to understand the experiences, beliefs, and practices of individuals and communities.
Purpose of Qualitative Data
The purpose of qualitative data is to gain a deeper understanding of social phenomena that cannot be captured by numerical or quantitative data. Qualitative data is collected through methods such as observation, interviews, and focus groups, and it provides descriptive information that can shed light on people’s experiences, beliefs, attitudes, and behaviors.
Qualitative data serves several purposes, including:
- Generating hypotheses: Qualitative data can be used to generate hypotheses about social phenomena that can be further tested with quantitative data.
- Providing context : Qualitative data provides a rich and detailed context for understanding social phenomena that cannot be captured by numerical data alone.
- Exploring complex phenomena : Qualitative data can be used to explore complex phenomena such as culture, social relationships, and the experiences of marginalized groups.
- Evaluating programs and intervention s: Qualitative data can be used to evaluate the impact of programs and interventions on the people who participate in them.
- Enhancing understanding: Qualitative data can be used to enhance understanding of the experiences, beliefs, and attitudes of individuals and communities, which can inform policy and practice.
When to use Qualitative Data
Qualitative data is appropriate when the research question requires an in-depth understanding of complex social phenomena that cannot be captured by numerical or quantitative data.
Here are some situations when qualitative data is appropriate:
- Exploratory research : Qualitative data is often used in exploratory research to generate hypotheses and develop a deeper understanding of a research question.
- Understanding social phenomena : Qualitative data is appropriate when the research question requires an in-depth understanding of social phenomena such as culture, social relationships, and experiences of marginalized groups.
- Program evaluation: Qualitative data is often used in program evaluation to understand the impact of a program on the people who participate in it.
- Needs assessment: Qualitative data is often used in needs assessments to understand the needs of a specific population.
- Market research: Qualitative data is often used in market research to understand consumer behavior and preferences.
- Case studies: Qualitative data is often used in case studies to understand a particular case in detail.
Characteristics of Qualitative Data
Here are some characteristics of qualitative data:
- Descriptive : Qualitative data provides a rich and detailed description of the social phenomena under investigation.
- Contextual : Qualitative data is collected in the context in which the social phenomena occur, which allows for a deeper understanding of the phenomena.
- Subjective : Qualitative data reflects the subjective experiences, beliefs, attitudes, and behaviors of the individuals and communities under investigation.
- Flexible : Qualitative data collection methods are flexible and can be adapted to the specific needs of the research question.
- Emergent : Qualitative data analysis is often an iterative process, where new themes and patterns emerge as the data is analyzed.
- Interpretive : Qualitative data analysis involves interpretation of the data, which requires the researcher to be reflexive and aware of their own biases and assumptions.
- Non-standardized: Qualitative data collection methods are often non-standardized, which means that the data is not collected in a standardized or uniform way.
Advantages of Qualitative Data
Some advantages of qualitative data are as follows:
- Richness : Qualitative data provides a rich and detailed description of the social phenomena under investigation, allowing for a deeper understanding of the phenomena.
- Flexibility : Qualitative data collection methods are flexible and can be adapted to the specific needs of the research question, allowing for a more nuanced exploration of social phenomena.
- Contextualization : Qualitative data is collected in the context in which the social phenomena occur, which allows for a deeper understanding of the phenomena and their cultural and social context.
- Subjectivity : Qualitative data reflects the subjective experiences, beliefs, attitudes, and behaviors of the individuals and communities under investigation, allowing for a more holistic understanding of the phenomena.
- New insights : Qualitative data can generate new insights and hypotheses that can be further tested with quantitative data.
- Participant voice : Qualitative data collection methods often involve direct participation by the individuals and communities under investigation, allowing for their voices to be heard.
- Ethical considerations: Qualitative data collection methods often prioritize ethical considerations such as informed consent, confidentiality, and respect for the autonomy of the participants.
Limitations of Qualitative Data
Here are some limitations of qualitative data:
- Subjectivity : Qualitative data is subjective, and the interpretation of the data depends on the researcher’s own biases, assumptions, and perspectives.
- Small sample size: Qualitative data collection methods often involve a small sample size, which limits the generalizability of the findings.
- Time-consuming: Qualitative data collection and analysis can be time-consuming, as it requires in-depth engagement with the data and often involves iterative processes.
- Limited statistical analysis: Qualitative data is often not suitable for statistical analysis, which limits the ability to draw quantitative conclusions from the data.
- Limited comparability: Qualitative data collection methods are often non-standardized, which makes it difficult to compare findings across different studies or contexts.
- Social desirability bias : Qualitative data collection methods often rely on self-reporting by the participants, which can be influenced by social desirability bias.
- Researcher bias: The researcher’s own biases, assumptions, and perspectives can influence the data collection and analysis, which can limit the objectivity of the findings.
About the author
Muhammad Hassan
Researcher, Academic Writer, Web developer
You may also like

Research Data – Types Methods and Examples

Quantitative Data – Types, Methods and Examples

Primary Data – Types, Methods and Examples

Secondary Data – Types, Methods and Examples

Information in Research – Types and Examples
- Open access
- Published: 27 May 2020
How to use and assess qualitative research methods
- Loraine Busetto ORCID: orcid.org/0000-0002-9228-7875 1 ,
- Wolfgang Wick 1 , 2 &
- Christoph Gumbinger 1
Neurological Research and Practice volume 2 , Article number: 14 ( 2020 ) Cite this article
784k Accesses
375 Citations
90 Altmetric
Metrics details
This paper aims to provide an overview of the use and assessment of qualitative research methods in the health sciences. Qualitative research can be defined as the study of the nature of phenomena and is especially appropriate for answering questions of why something is (not) observed, assessing complex multi-component interventions, and focussing on intervention improvement. The most common methods of data collection are document study, (non-) participant observations, semi-structured interviews and focus groups. For data analysis, field-notes and audio-recordings are transcribed into protocols and transcripts, and coded using qualitative data management software. Criteria such as checklists, reflexivity, sampling strategies, piloting, co-coding, member-checking and stakeholder involvement can be used to enhance and assess the quality of the research conducted. Using qualitative in addition to quantitative designs will equip us with better tools to address a greater range of research problems, and to fill in blind spots in current neurological research and practice.
The aim of this paper is to provide an overview of qualitative research methods, including hands-on information on how they can be used, reported and assessed. This article is intended for beginning qualitative researchers in the health sciences as well as experienced quantitative researchers who wish to broaden their understanding of qualitative research.
What is qualitative research?
Qualitative research is defined as “the study of the nature of phenomena”, including “their quality, different manifestations, the context in which they appear or the perspectives from which they can be perceived” , but excluding “their range, frequency and place in an objectively determined chain of cause and effect” [ 1 ]. This formal definition can be complemented with a more pragmatic rule of thumb: qualitative research generally includes data in form of words rather than numbers [ 2 ].
Why conduct qualitative research?
Because some research questions cannot be answered using (only) quantitative methods. For example, one Australian study addressed the issue of why patients from Aboriginal communities often present late or not at all to specialist services offered by tertiary care hospitals. Using qualitative interviews with patients and staff, it found one of the most significant access barriers to be transportation problems, including some towns and communities simply not having a bus service to the hospital [ 3 ]. A quantitative study could have measured the number of patients over time or even looked at possible explanatory factors – but only those previously known or suspected to be of relevance. To discover reasons for observed patterns, especially the invisible or surprising ones, qualitative designs are needed.
While qualitative research is common in other fields, it is still relatively underrepresented in health services research. The latter field is more traditionally rooted in the evidence-based-medicine paradigm, as seen in " research that involves testing the effectiveness of various strategies to achieve changes in clinical practice, preferably applying randomised controlled trial study designs (...) " [ 4 ]. This focus on quantitative research and specifically randomised controlled trials (RCT) is visible in the idea of a hierarchy of research evidence which assumes that some research designs are objectively better than others, and that choosing a "lesser" design is only acceptable when the better ones are not practically or ethically feasible [ 5 , 6 ]. Others, however, argue that an objective hierarchy does not exist, and that, instead, the research design and methods should be chosen to fit the specific research question at hand – "questions before methods" [ 2 , 7 , 8 , 9 ]. This means that even when an RCT is possible, some research problems require a different design that is better suited to addressing them. Arguing in JAMA, Berwick uses the example of rapid response teams in hospitals, which he describes as " a complex, multicomponent intervention – essentially a process of social change" susceptible to a range of different context factors including leadership or organisation history. According to him, "[in] such complex terrain, the RCT is an impoverished way to learn. Critics who use it as a truth standard in this context are incorrect" [ 8 ] . Instead of limiting oneself to RCTs, Berwick recommends embracing a wider range of methods , including qualitative ones, which for "these specific applications, (...) are not compromises in learning how to improve; they are superior" [ 8 ].
Research problems that can be approached particularly well using qualitative methods include assessing complex multi-component interventions or systems (of change), addressing questions beyond “what works”, towards “what works for whom when, how and why”, and focussing on intervention improvement rather than accreditation [ 7 , 9 , 10 , 11 , 12 ]. Using qualitative methods can also help shed light on the “softer” side of medical treatment. For example, while quantitative trials can measure the costs and benefits of neuro-oncological treatment in terms of survival rates or adverse effects, qualitative research can help provide a better understanding of patient or caregiver stress, visibility of illness or out-of-pocket expenses.
How to conduct qualitative research?
Given that qualitative research is characterised by flexibility, openness and responsivity to context, the steps of data collection and analysis are not as separate and consecutive as they tend to be in quantitative research [ 13 , 14 ]. As Fossey puts it : “sampling, data collection, analysis and interpretation are related to each other in a cyclical (iterative) manner, rather than following one after another in a stepwise approach” [ 15 ]. The researcher can make educated decisions with regard to the choice of method, how they are implemented, and to which and how many units they are applied [ 13 ]. As shown in Fig. 1 , this can involve several back-and-forth steps between data collection and analysis where new insights and experiences can lead to adaption and expansion of the original plan. Some insights may also necessitate a revision of the research question and/or the research design as a whole. The process ends when saturation is achieved, i.e. when no relevant new information can be found (see also below: sampling and saturation). For reasons of transparency, it is essential for all decisions as well as the underlying reasoning to be well-documented.
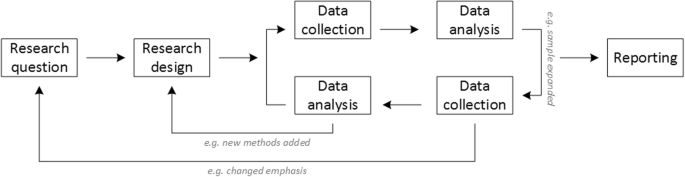
Iterative research process
While it is not always explicitly addressed, qualitative methods reflect a different underlying research paradigm than quantitative research (e.g. constructivism or interpretivism as opposed to positivism). The choice of methods can be based on the respective underlying substantive theory or theoretical framework used by the researcher [ 2 ].
Data collection
The methods of qualitative data collection most commonly used in health research are document study, observations, semi-structured interviews and focus groups [ 1 , 14 , 16 , 17 ].
Document study
Document study (also called document analysis) refers to the review by the researcher of written materials [ 14 ]. These can include personal and non-personal documents such as archives, annual reports, guidelines, policy documents, diaries or letters.
Observations
Observations are particularly useful to gain insights into a certain setting and actual behaviour – as opposed to reported behaviour or opinions [ 13 ]. Qualitative observations can be either participant or non-participant in nature. In participant observations, the observer is part of the observed setting, for example a nurse working in an intensive care unit [ 18 ]. In non-participant observations, the observer is “on the outside looking in”, i.e. present in but not part of the situation, trying not to influence the setting by their presence. Observations can be planned (e.g. for 3 h during the day or night shift) or ad hoc (e.g. as soon as a stroke patient arrives at the emergency room). During the observation, the observer takes notes on everything or certain pre-determined parts of what is happening around them, for example focusing on physician-patient interactions or communication between different professional groups. Written notes can be taken during or after the observations, depending on feasibility (which is usually lower during participant observations) and acceptability (e.g. when the observer is perceived to be judging the observed). Afterwards, these field notes are transcribed into observation protocols. If more than one observer was involved, field notes are taken independently, but notes can be consolidated into one protocol after discussions. Advantages of conducting observations include minimising the distance between the researcher and the researched, the potential discovery of topics that the researcher did not realise were relevant and gaining deeper insights into the real-world dimensions of the research problem at hand [ 18 ].
Semi-structured interviews
Hijmans & Kuyper describe qualitative interviews as “an exchange with an informal character, a conversation with a goal” [ 19 ]. Interviews are used to gain insights into a person’s subjective experiences, opinions and motivations – as opposed to facts or behaviours [ 13 ]. Interviews can be distinguished by the degree to which they are structured (i.e. a questionnaire), open (e.g. free conversation or autobiographical interviews) or semi-structured [ 2 , 13 ]. Semi-structured interviews are characterized by open-ended questions and the use of an interview guide (or topic guide/list) in which the broad areas of interest, sometimes including sub-questions, are defined [ 19 ]. The pre-defined topics in the interview guide can be derived from the literature, previous research or a preliminary method of data collection, e.g. document study or observations. The topic list is usually adapted and improved at the start of the data collection process as the interviewer learns more about the field [ 20 ]. Across interviews the focus on the different (blocks of) questions may differ and some questions may be skipped altogether (e.g. if the interviewee is not able or willing to answer the questions or for concerns about the total length of the interview) [ 20 ]. Qualitative interviews are usually not conducted in written format as it impedes on the interactive component of the method [ 20 ]. In comparison to written surveys, qualitative interviews have the advantage of being interactive and allowing for unexpected topics to emerge and to be taken up by the researcher. This can also help overcome a provider or researcher-centred bias often found in written surveys, which by nature, can only measure what is already known or expected to be of relevance to the researcher. Interviews can be audio- or video-taped; but sometimes it is only feasible or acceptable for the interviewer to take written notes [ 14 , 16 , 20 ].
Focus groups
Focus groups are group interviews to explore participants’ expertise and experiences, including explorations of how and why people behave in certain ways [ 1 ]. Focus groups usually consist of 6–8 people and are led by an experienced moderator following a topic guide or “script” [ 21 ]. They can involve an observer who takes note of the non-verbal aspects of the situation, possibly using an observation guide [ 21 ]. Depending on researchers’ and participants’ preferences, the discussions can be audio- or video-taped and transcribed afterwards [ 21 ]. Focus groups are useful for bringing together homogeneous (to a lesser extent heterogeneous) groups of participants with relevant expertise and experience on a given topic on which they can share detailed information [ 21 ]. Focus groups are a relatively easy, fast and inexpensive method to gain access to information on interactions in a given group, i.e. “the sharing and comparing” among participants [ 21 ]. Disadvantages include less control over the process and a lesser extent to which each individual may participate. Moreover, focus group moderators need experience, as do those tasked with the analysis of the resulting data. Focus groups can be less appropriate for discussing sensitive topics that participants might be reluctant to disclose in a group setting [ 13 ]. Moreover, attention must be paid to the emergence of “groupthink” as well as possible power dynamics within the group, e.g. when patients are awed or intimidated by health professionals.
Choosing the “right” method
As explained above, the school of thought underlying qualitative research assumes no objective hierarchy of evidence and methods. This means that each choice of single or combined methods has to be based on the research question that needs to be answered and a critical assessment with regard to whether or to what extent the chosen method can accomplish this – i.e. the “fit” between question and method [ 14 ]. It is necessary for these decisions to be documented when they are being made, and to be critically discussed when reporting methods and results.
Let us assume that our research aim is to examine the (clinical) processes around acute endovascular treatment (EVT), from the patient’s arrival at the emergency room to recanalization, with the aim to identify possible causes for delay and/or other causes for sub-optimal treatment outcome. As a first step, we could conduct a document study of the relevant standard operating procedures (SOPs) for this phase of care – are they up-to-date and in line with current guidelines? Do they contain any mistakes, irregularities or uncertainties that could cause delays or other problems? Regardless of the answers to these questions, the results have to be interpreted based on what they are: a written outline of what care processes in this hospital should look like. If we want to know what they actually look like in practice, we can conduct observations of the processes described in the SOPs. These results can (and should) be analysed in themselves, but also in comparison to the results of the document analysis, especially as regards relevant discrepancies. Do the SOPs outline specific tests for which no equipment can be observed or tasks to be performed by specialized nurses who are not present during the observation? It might also be possible that the written SOP is outdated, but the actual care provided is in line with current best practice. In order to find out why these discrepancies exist, it can be useful to conduct interviews. Are the physicians simply not aware of the SOPs (because their existence is limited to the hospital’s intranet) or do they actively disagree with them or does the infrastructure make it impossible to provide the care as described? Another rationale for adding interviews is that some situations (or all of their possible variations for different patient groups or the day, night or weekend shift) cannot practically or ethically be observed. In this case, it is possible to ask those involved to report on their actions – being aware that this is not the same as the actual observation. A senior physician’s or hospital manager’s description of certain situations might differ from a nurse’s or junior physician’s one, maybe because they intentionally misrepresent facts or maybe because different aspects of the process are visible or important to them. In some cases, it can also be relevant to consider to whom the interviewee is disclosing this information – someone they trust, someone they are otherwise not connected to, or someone they suspect or are aware of being in a potentially “dangerous” power relationship to them. Lastly, a focus group could be conducted with representatives of the relevant professional groups to explore how and why exactly they provide care around EVT. The discussion might reveal discrepancies (between SOPs and actual care or between different physicians) and motivations to the researchers as well as to the focus group members that they might not have been aware of themselves. For the focus group to deliver relevant information, attention has to be paid to its composition and conduct, for example, to make sure that all participants feel safe to disclose sensitive or potentially problematic information or that the discussion is not dominated by (senior) physicians only. The resulting combination of data collection methods is shown in Fig. 2 .

Possible combination of data collection methods
Attributions for icons: “Book” by Serhii Smirnov, “Interview” by Adrien Coquet, FR, “Magnifying Glass” by anggun, ID, “Business communication” by Vectors Market; all from the Noun Project
The combination of multiple data source as described for this example can be referred to as “triangulation”, in which multiple measurements are carried out from different angles to achieve a more comprehensive understanding of the phenomenon under study [ 22 , 23 ].
Data analysis
To analyse the data collected through observations, interviews and focus groups these need to be transcribed into protocols and transcripts (see Fig. 3 ). Interviews and focus groups can be transcribed verbatim , with or without annotations for behaviour (e.g. laughing, crying, pausing) and with or without phonetic transcription of dialects and filler words, depending on what is expected or known to be relevant for the analysis. In the next step, the protocols and transcripts are coded , that is, marked (or tagged, labelled) with one or more short descriptors of the content of a sentence or paragraph [ 2 , 15 , 23 ]. Jansen describes coding as “connecting the raw data with “theoretical” terms” [ 20 ]. In a more practical sense, coding makes raw data sortable. This makes it possible to extract and examine all segments describing, say, a tele-neurology consultation from multiple data sources (e.g. SOPs, emergency room observations, staff and patient interview). In a process of synthesis and abstraction, the codes are then grouped, summarised and/or categorised [ 15 , 20 ]. The end product of the coding or analysis process is a descriptive theory of the behavioural pattern under investigation [ 20 ]. The coding process is performed using qualitative data management software, the most common ones being InVivo, MaxQDA and Atlas.ti. It should be noted that these are data management tools which support the analysis performed by the researcher(s) [ 14 ].
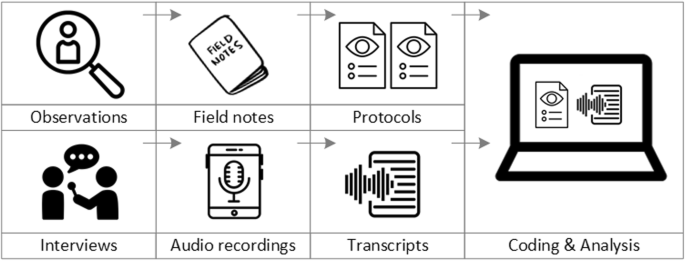
From data collection to data analysis
Attributions for icons: see Fig. 2 , also “Speech to text” by Trevor Dsouza, “Field Notes” by Mike O’Brien, US, “Voice Record” by ProSymbols, US, “Inspection” by Made, AU, and “Cloud” by Graphic Tigers; all from the Noun Project
How to report qualitative research?
Protocols of qualitative research can be published separately and in advance of the study results. However, the aim is not the same as in RCT protocols, i.e. to pre-define and set in stone the research questions and primary or secondary endpoints. Rather, it is a way to describe the research methods in detail, which might not be possible in the results paper given journals’ word limits. Qualitative research papers are usually longer than their quantitative counterparts to allow for deep understanding and so-called “thick description”. In the methods section, the focus is on transparency of the methods used, including why, how and by whom they were implemented in the specific study setting, so as to enable a discussion of whether and how this may have influenced data collection, analysis and interpretation. The results section usually starts with a paragraph outlining the main findings, followed by more detailed descriptions of, for example, the commonalities, discrepancies or exceptions per category [ 20 ]. Here it is important to support main findings by relevant quotations, which may add information, context, emphasis or real-life examples [ 20 , 23 ]. It is subject to debate in the field whether it is relevant to state the exact number or percentage of respondents supporting a certain statement (e.g. “Five interviewees expressed negative feelings towards XYZ”) [ 21 ].
How to combine qualitative with quantitative research?
Qualitative methods can be combined with other methods in multi- or mixed methods designs, which “[employ] two or more different methods [ …] within the same study or research program rather than confining the research to one single method” [ 24 ]. Reasons for combining methods can be diverse, including triangulation for corroboration of findings, complementarity for illustration and clarification of results, expansion to extend the breadth and range of the study, explanation of (unexpected) results generated with one method with the help of another, or offsetting the weakness of one method with the strength of another [ 1 , 17 , 24 , 25 , 26 ]. The resulting designs can be classified according to when, why and how the different quantitative and/or qualitative data strands are combined. The three most common types of mixed method designs are the convergent parallel design , the explanatory sequential design and the exploratory sequential design. The designs with examples are shown in Fig. 4 .

Three common mixed methods designs
In the convergent parallel design, a qualitative study is conducted in parallel to and independently of a quantitative study, and the results of both studies are compared and combined at the stage of interpretation of results. Using the above example of EVT provision, this could entail setting up a quantitative EVT registry to measure process times and patient outcomes in parallel to conducting the qualitative research outlined above, and then comparing results. Amongst other things, this would make it possible to assess whether interview respondents’ subjective impressions of patients receiving good care match modified Rankin Scores at follow-up, or whether observed delays in care provision are exceptions or the rule when compared to door-to-needle times as documented in the registry. In the explanatory sequential design, a quantitative study is carried out first, followed by a qualitative study to help explain the results from the quantitative study. This would be an appropriate design if the registry alone had revealed relevant delays in door-to-needle times and the qualitative study would be used to understand where and why these occurred, and how they could be improved. In the exploratory design, the qualitative study is carried out first and its results help informing and building the quantitative study in the next step [ 26 ]. If the qualitative study around EVT provision had shown a high level of dissatisfaction among the staff members involved, a quantitative questionnaire investigating staff satisfaction could be set up in the next step, informed by the qualitative study on which topics dissatisfaction had been expressed. Amongst other things, the questionnaire design would make it possible to widen the reach of the research to more respondents from different (types of) hospitals, regions, countries or settings, and to conduct sub-group analyses for different professional groups.
How to assess qualitative research?
A variety of assessment criteria and lists have been developed for qualitative research, ranging in their focus and comprehensiveness [ 14 , 17 , 27 ]. However, none of these has been elevated to the “gold standard” in the field. In the following, we therefore focus on a set of commonly used assessment criteria that, from a practical standpoint, a researcher can look for when assessing a qualitative research report or paper.
Assessors should check the authors’ use of and adherence to the relevant reporting checklists (e.g. Standards for Reporting Qualitative Research (SRQR)) to make sure all items that are relevant for this type of research are addressed [ 23 , 28 ]. Discussions of quantitative measures in addition to or instead of these qualitative measures can be a sign of lower quality of the research (paper). Providing and adhering to a checklist for qualitative research contributes to an important quality criterion for qualitative research, namely transparency [ 15 , 17 , 23 ].
Reflexivity
While methodological transparency and complete reporting is relevant for all types of research, some additional criteria must be taken into account for qualitative research. This includes what is called reflexivity, i.e. sensitivity to the relationship between the researcher and the researched, including how contact was established and maintained, or the background and experience of the researcher(s) involved in data collection and analysis. Depending on the research question and population to be researched this can be limited to professional experience, but it may also include gender, age or ethnicity [ 17 , 27 ]. These details are relevant because in qualitative research, as opposed to quantitative research, the researcher as a person cannot be isolated from the research process [ 23 ]. It may influence the conversation when an interviewed patient speaks to an interviewer who is a physician, or when an interviewee is asked to discuss a gynaecological procedure with a male interviewer, and therefore the reader must be made aware of these details [ 19 ].
Sampling and saturation
The aim of qualitative sampling is for all variants of the objects of observation that are deemed relevant for the study to be present in the sample “ to see the issue and its meanings from as many angles as possible” [ 1 , 16 , 19 , 20 , 27 ] , and to ensure “information-richness [ 15 ]. An iterative sampling approach is advised, in which data collection (e.g. five interviews) is followed by data analysis, followed by more data collection to find variants that are lacking in the current sample. This process continues until no new (relevant) information can be found and further sampling becomes redundant – which is called saturation [ 1 , 15 ] . In other words: qualitative data collection finds its end point not a priori , but when the research team determines that saturation has been reached [ 29 , 30 ].
This is also the reason why most qualitative studies use deliberate instead of random sampling strategies. This is generally referred to as “ purposive sampling” , in which researchers pre-define which types of participants or cases they need to include so as to cover all variations that are expected to be of relevance, based on the literature, previous experience or theory (i.e. theoretical sampling) [ 14 , 20 ]. Other types of purposive sampling include (but are not limited to) maximum variation sampling, critical case sampling or extreme or deviant case sampling [ 2 ]. In the above EVT example, a purposive sample could include all relevant professional groups and/or all relevant stakeholders (patients, relatives) and/or all relevant times of observation (day, night and weekend shift).
Assessors of qualitative research should check whether the considerations underlying the sampling strategy were sound and whether or how researchers tried to adapt and improve their strategies in stepwise or cyclical approaches between data collection and analysis to achieve saturation [ 14 ].
Good qualitative research is iterative in nature, i.e. it goes back and forth between data collection and analysis, revising and improving the approach where necessary. One example of this are pilot interviews, where different aspects of the interview (especially the interview guide, but also, for example, the site of the interview or whether the interview can be audio-recorded) are tested with a small number of respondents, evaluated and revised [ 19 ]. In doing so, the interviewer learns which wording or types of questions work best, or which is the best length of an interview with patients who have trouble concentrating for an extended time. Of course, the same reasoning applies to observations or focus groups which can also be piloted.
Ideally, coding should be performed by at least two researchers, especially at the beginning of the coding process when a common approach must be defined, including the establishment of a useful coding list (or tree), and when a common meaning of individual codes must be established [ 23 ]. An initial sub-set or all transcripts can be coded independently by the coders and then compared and consolidated after regular discussions in the research team. This is to make sure that codes are applied consistently to the research data.
Member checking
Member checking, also called respondent validation , refers to the practice of checking back with study respondents to see if the research is in line with their views [ 14 , 27 ]. This can happen after data collection or analysis or when first results are available [ 23 ]. For example, interviewees can be provided with (summaries of) their transcripts and asked whether they believe this to be a complete representation of their views or whether they would like to clarify or elaborate on their responses [ 17 ]. Respondents’ feedback on these issues then becomes part of the data collection and analysis [ 27 ].
Stakeholder involvement
In those niches where qualitative approaches have been able to evolve and grow, a new trend has seen the inclusion of patients and their representatives not only as study participants (i.e. “members”, see above) but as consultants to and active participants in the broader research process [ 31 , 32 , 33 ]. The underlying assumption is that patients and other stakeholders hold unique perspectives and experiences that add value beyond their own single story, making the research more relevant and beneficial to researchers, study participants and (future) patients alike [ 34 , 35 ]. Using the example of patients on or nearing dialysis, a recent scoping review found that 80% of clinical research did not address the top 10 research priorities identified by patients and caregivers [ 32 , 36 ]. In this sense, the involvement of the relevant stakeholders, especially patients and relatives, is increasingly being seen as a quality indicator in and of itself.
How not to assess qualitative research
The above overview does not include certain items that are routine in assessments of quantitative research. What follows is a non-exhaustive, non-representative, experience-based list of the quantitative criteria often applied to the assessment of qualitative research, as well as an explanation of the limited usefulness of these endeavours.
Protocol adherence
Given the openness and flexibility of qualitative research, it should not be assessed by how well it adheres to pre-determined and fixed strategies – in other words: its rigidity. Instead, the assessor should look for signs of adaptation and refinement based on lessons learned from earlier steps in the research process.
Sample size
For the reasons explained above, qualitative research does not require specific sample sizes, nor does it require that the sample size be determined a priori [ 1 , 14 , 27 , 37 , 38 , 39 ]. Sample size can only be a useful quality indicator when related to the research purpose, the chosen methodology and the composition of the sample, i.e. who was included and why.
Randomisation
While some authors argue that randomisation can be used in qualitative research, this is not commonly the case, as neither its feasibility nor its necessity or usefulness has been convincingly established for qualitative research [ 13 , 27 ]. Relevant disadvantages include the negative impact of a too large sample size as well as the possibility (or probability) of selecting “ quiet, uncooperative or inarticulate individuals ” [ 17 ]. Qualitative studies do not use control groups, either.
Interrater reliability, variability and other “objectivity checks”
The concept of “interrater reliability” is sometimes used in qualitative research to assess to which extent the coding approach overlaps between the two co-coders. However, it is not clear what this measure tells us about the quality of the analysis [ 23 ]. This means that these scores can be included in qualitative research reports, preferably with some additional information on what the score means for the analysis, but it is not a requirement. Relatedly, it is not relevant for the quality or “objectivity” of qualitative research to separate those who recruited the study participants and collected and analysed the data. Experiences even show that it might be better to have the same person or team perform all of these tasks [ 20 ]. First, when researchers introduce themselves during recruitment this can enhance trust when the interview takes place days or weeks later with the same researcher. Second, when the audio-recording is transcribed for analysis, the researcher conducting the interviews will usually remember the interviewee and the specific interview situation during data analysis. This might be helpful in providing additional context information for interpretation of data, e.g. on whether something might have been meant as a joke [ 18 ].
Not being quantitative research
Being qualitative research instead of quantitative research should not be used as an assessment criterion if it is used irrespectively of the research problem at hand. Similarly, qualitative research should not be required to be combined with quantitative research per se – unless mixed methods research is judged as inherently better than single-method research. In this case, the same criterion should be applied for quantitative studies without a qualitative component.
The main take-away points of this paper are summarised in Table 1 . We aimed to show that, if conducted well, qualitative research can answer specific research questions that cannot to be adequately answered using (only) quantitative designs. Seeing qualitative and quantitative methods as equal will help us become more aware and critical of the “fit” between the research problem and our chosen methods: I can conduct an RCT to determine the reasons for transportation delays of acute stroke patients – but should I? It also provides us with a greater range of tools to tackle a greater range of research problems more appropriately and successfully, filling in the blind spots on one half of the methodological spectrum to better address the whole complexity of neurological research and practice.
Availability of data and materials
Not applicable.
Abbreviations
Endovascular treatment
Randomised Controlled Trial
Standard Operating Procedure
Standards for Reporting Qualitative Research
Philipsen, H., & Vernooij-Dassen, M. (2007). Kwalitatief onderzoek: nuttig, onmisbaar en uitdagend. In L. PLBJ & H. TCo (Eds.), Kwalitatief onderzoek: Praktische methoden voor de medische praktijk . [Qualitative research: useful, indispensable and challenging. In: Qualitative research: Practical methods for medical practice (pp. 5–12). Houten: Bohn Stafleu van Loghum.
Chapter Google Scholar
Punch, K. F. (2013). Introduction to social research: Quantitative and qualitative approaches . London: Sage.
Kelly, J., Dwyer, J., Willis, E., & Pekarsky, B. (2014). Travelling to the city for hospital care: Access factors in country aboriginal patient journeys. Australian Journal of Rural Health, 22 (3), 109–113.
Article Google Scholar
Nilsen, P., Ståhl, C., Roback, K., & Cairney, P. (2013). Never the twain shall meet? - a comparison of implementation science and policy implementation research. Implementation Science, 8 (1), 1–12.
Howick J, Chalmers I, Glasziou, P., Greenhalgh, T., Heneghan, C., Liberati, A., Moschetti, I., Phillips, B., & Thornton, H. (2011). The 2011 Oxford CEBM evidence levels of evidence (introductory document) . Oxford Center for Evidence Based Medicine. https://www.cebm.net/2011/06/2011-oxford-cebm-levels-evidence-introductory-document/ .
Eakin, J. M. (2016). Educating critical qualitative health researchers in the land of the randomized controlled trial. Qualitative Inquiry, 22 (2), 107–118.
May, A., & Mathijssen, J. (2015). Alternatieven voor RCT bij de evaluatie van effectiviteit van interventies!? Eindrapportage. In Alternatives for RCTs in the evaluation of effectiveness of interventions!? Final report .
Google Scholar
Berwick, D. M. (2008). The science of improvement. Journal of the American Medical Association, 299 (10), 1182–1184.
Article CAS Google Scholar
Christ, T. W. (2014). Scientific-based research and randomized controlled trials, the “gold” standard? Alternative paradigms and mixed methodologies. Qualitative Inquiry, 20 (1), 72–80.
Lamont, T., Barber, N., Jd, P., Fulop, N., Garfield-Birkbeck, S., Lilford, R., Mear, L., Raine, R., & Fitzpatrick, R. (2016). New approaches to evaluating complex health and care systems. BMJ, 352:i154.
Drabble, S. J., & O’Cathain, A. (2015). Moving from Randomized Controlled Trials to Mixed Methods Intervention Evaluation. In S. Hesse-Biber & R. B. Johnson (Eds.), The Oxford Handbook of Multimethod and Mixed Methods Research Inquiry (pp. 406–425). London: Oxford University Press.
Chambers, D. A., Glasgow, R. E., & Stange, K. C. (2013). The dynamic sustainability framework: Addressing the paradox of sustainment amid ongoing change. Implementation Science : IS, 8 , 117.
Hak, T. (2007). Waarnemingsmethoden in kwalitatief onderzoek. In L. PLBJ & H. TCo (Eds.), Kwalitatief onderzoek: Praktische methoden voor de medische praktijk . [Observation methods in qualitative research] (pp. 13–25). Houten: Bohn Stafleu van Loghum.
Russell, C. K., & Gregory, D. M. (2003). Evaluation of qualitative research studies. Evidence Based Nursing, 6 (2), 36–40.
Fossey, E., Harvey, C., McDermott, F., & Davidson, L. (2002). Understanding and evaluating qualitative research. Australian and New Zealand Journal of Psychiatry, 36 , 717–732.
Yanow, D. (2000). Conducting interpretive policy analysis (Vol. 47). Thousand Oaks: Sage University Papers Series on Qualitative Research Methods.
Shenton, A. K. (2004). Strategies for ensuring trustworthiness in qualitative research projects. Education for Information, 22 , 63–75.
van der Geest, S. (2006). Participeren in ziekte en zorg: meer over kwalitatief onderzoek. Huisarts en Wetenschap, 49 (4), 283–287.
Hijmans, E., & Kuyper, M. (2007). Het halfopen interview als onderzoeksmethode. In L. PLBJ & H. TCo (Eds.), Kwalitatief onderzoek: Praktische methoden voor de medische praktijk . [The half-open interview as research method (pp. 43–51). Houten: Bohn Stafleu van Loghum.
Jansen, H. (2007). Systematiek en toepassing van de kwalitatieve survey. In L. PLBJ & H. TCo (Eds.), Kwalitatief onderzoek: Praktische methoden voor de medische praktijk . [Systematics and implementation of the qualitative survey (pp. 27–41). Houten: Bohn Stafleu van Loghum.
Pv, R., & Peremans, L. (2007). Exploreren met focusgroepgesprekken: de ‘stem’ van de groep onder de loep. In L. PLBJ & H. TCo (Eds.), Kwalitatief onderzoek: Praktische methoden voor de medische praktijk . [Exploring with focus group conversations: the “voice” of the group under the magnifying glass (pp. 53–64). Houten: Bohn Stafleu van Loghum.
Carter, N., Bryant-Lukosius, D., DiCenso, A., Blythe, J., & Neville, A. J. (2014). The use of triangulation in qualitative research. Oncology Nursing Forum, 41 (5), 545–547.
Boeije H: Analyseren in kwalitatief onderzoek: Denken en doen, [Analysis in qualitative research: Thinking and doing] vol. Den Haag Boom Lemma uitgevers; 2012.
Hunter, A., & Brewer, J. (2015). Designing Multimethod Research. In S. Hesse-Biber & R. B. Johnson (Eds.), The Oxford Handbook of Multimethod and Mixed Methods Research Inquiry (pp. 185–205). London: Oxford University Press.
Archibald, M. M., Radil, A. I., Zhang, X., & Hanson, W. E. (2015). Current mixed methods practices in qualitative research: A content analysis of leading journals. International Journal of Qualitative Methods, 14 (2), 5–33.
Creswell, J. W., & Plano Clark, V. L. (2011). Choosing a Mixed Methods Design. In Designing and Conducting Mixed Methods Research . Thousand Oaks: SAGE Publications.
Mays, N., & Pope, C. (2000). Assessing quality in qualitative research. BMJ, 320 (7226), 50–52.
O'Brien, B. C., Harris, I. B., Beckman, T. J., Reed, D. A., & Cook, D. A. (2014). Standards for reporting qualitative research: A synthesis of recommendations. Academic Medicine : Journal of the Association of American Medical Colleges, 89 (9), 1245–1251.
Saunders, B., Sim, J., Kingstone, T., Baker, S., Waterfield, J., Bartlam, B., Burroughs, H., & Jinks, C. (2018). Saturation in qualitative research: Exploring its conceptualization and operationalization. Quality and Quantity, 52 (4), 1893–1907.
Moser, A., & Korstjens, I. (2018). Series: Practical guidance to qualitative research. Part 3: Sampling, data collection and analysis. European Journal of General Practice, 24 (1), 9–18.
Marlett, N., Shklarov, S., Marshall, D., Santana, M. J., & Wasylak, T. (2015). Building new roles and relationships in research: A model of patient engagement research. Quality of Life Research : an international journal of quality of life aspects of treatment, care and rehabilitation, 24 (5), 1057–1067.
Demian, M. N., Lam, N. N., Mac-Way, F., Sapir-Pichhadze, R., & Fernandez, N. (2017). Opportunities for engaging patients in kidney research. Canadian Journal of Kidney Health and Disease, 4 , 2054358117703070–2054358117703070.
Noyes, J., McLaughlin, L., Morgan, K., Roberts, A., Stephens, M., Bourne, J., Houlston, M., Houlston, J., Thomas, S., Rhys, R. G., et al. (2019). Designing a co-productive study to overcome known methodological challenges in organ donation research with bereaved family members. Health Expectations . 22(4):824–35.
Piil, K., Jarden, M., & Pii, K. H. (2019). Research agenda for life-threatening cancer. European Journal Cancer Care (Engl), 28 (1), e12935.
Hofmann, D., Ibrahim, F., Rose, D., Scott, D. L., Cope, A., Wykes, T., & Lempp, H. (2015). Expectations of new treatment in rheumatoid arthritis: Developing a patient-generated questionnaire. Health Expectations : an international journal of public participation in health care and health policy, 18 (5), 995–1008.
Jun, M., Manns, B., Laupacis, A., Manns, L., Rehal, B., Crowe, S., & Hemmelgarn, B. R. (2015). Assessing the extent to which current clinical research is consistent with patient priorities: A scoping review using a case study in patients on or nearing dialysis. Canadian Journal of Kidney Health and Disease, 2 , 35.
Elsie Baker, S., & Edwards, R. (2012). How many qualitative interviews is enough? In National Centre for Research Methods Review Paper . National Centre for Research Methods. http://eprints.ncrm.ac.uk/2273/4/how_many_interviews.pdf .
Sandelowski, M. (1995). Sample size in qualitative research. Research in Nursing & Health, 18 (2), 179–183.
Sim, J., Saunders, B., Waterfield, J., & Kingstone, T. (2018). Can sample size in qualitative research be determined a priori? International Journal of Social Research Methodology, 21 (5), 619–634.
Download references
Acknowledgements
no external funding.
Author information
Authors and affiliations.
Department of Neurology, Heidelberg University Hospital, Im Neuenheimer Feld 400, 69120, Heidelberg, Germany
Loraine Busetto, Wolfgang Wick & Christoph Gumbinger
Clinical Cooperation Unit Neuro-Oncology, German Cancer Research Center, Heidelberg, Germany
Wolfgang Wick
You can also search for this author in PubMed Google Scholar
Contributions
LB drafted the manuscript; WW and CG revised the manuscript; all authors approved the final versions.
Corresponding author
Correspondence to Loraine Busetto .
Ethics declarations
Ethics approval and consent to participate, consent for publication, competing interests.
The authors declare no competing interests.
Additional information
Publisher’s note.
Springer Nature remains neutral with regard to jurisdictional claims in published maps and institutional affiliations.
Rights and permissions
Open Access This article is licensed under a Creative Commons Attribution 4.0 International License, which permits use, sharing, adaptation, distribution and reproduction in any medium or format, as long as you give appropriate credit to the original author(s) and the source, provide a link to the Creative Commons licence, and indicate if changes were made. The images or other third party material in this article are included in the article's Creative Commons licence, unless indicated otherwise in a credit line to the material. If material is not included in the article's Creative Commons licence and your intended use is not permitted by statutory regulation or exceeds the permitted use, you will need to obtain permission directly from the copyright holder. To view a copy of this licence, visit http://creativecommons.org/licenses/by/4.0/ .
Reprints and permissions
About this article
Cite this article.
Busetto, L., Wick, W. & Gumbinger, C. How to use and assess qualitative research methods. Neurol. Res. Pract. 2 , 14 (2020). https://doi.org/10.1186/s42466-020-00059-z
Download citation
Received : 30 January 2020
Accepted : 22 April 2020
Published : 27 May 2020
DOI : https://doi.org/10.1186/s42466-020-00059-z
Share this article
Anyone you share the following link with will be able to read this content:
Sorry, a shareable link is not currently available for this article.
Provided by the Springer Nature SharedIt content-sharing initiative
- Qualitative research
- Mixed methods
- Quality assessment
Neurological Research and Practice
ISSN: 2524-3489
- Submission enquiries: Access here and click Contact Us
- General enquiries: [email protected]
How to Conduct Qualitative Data Analysis? (+The Best Tool to Use)
12 min read
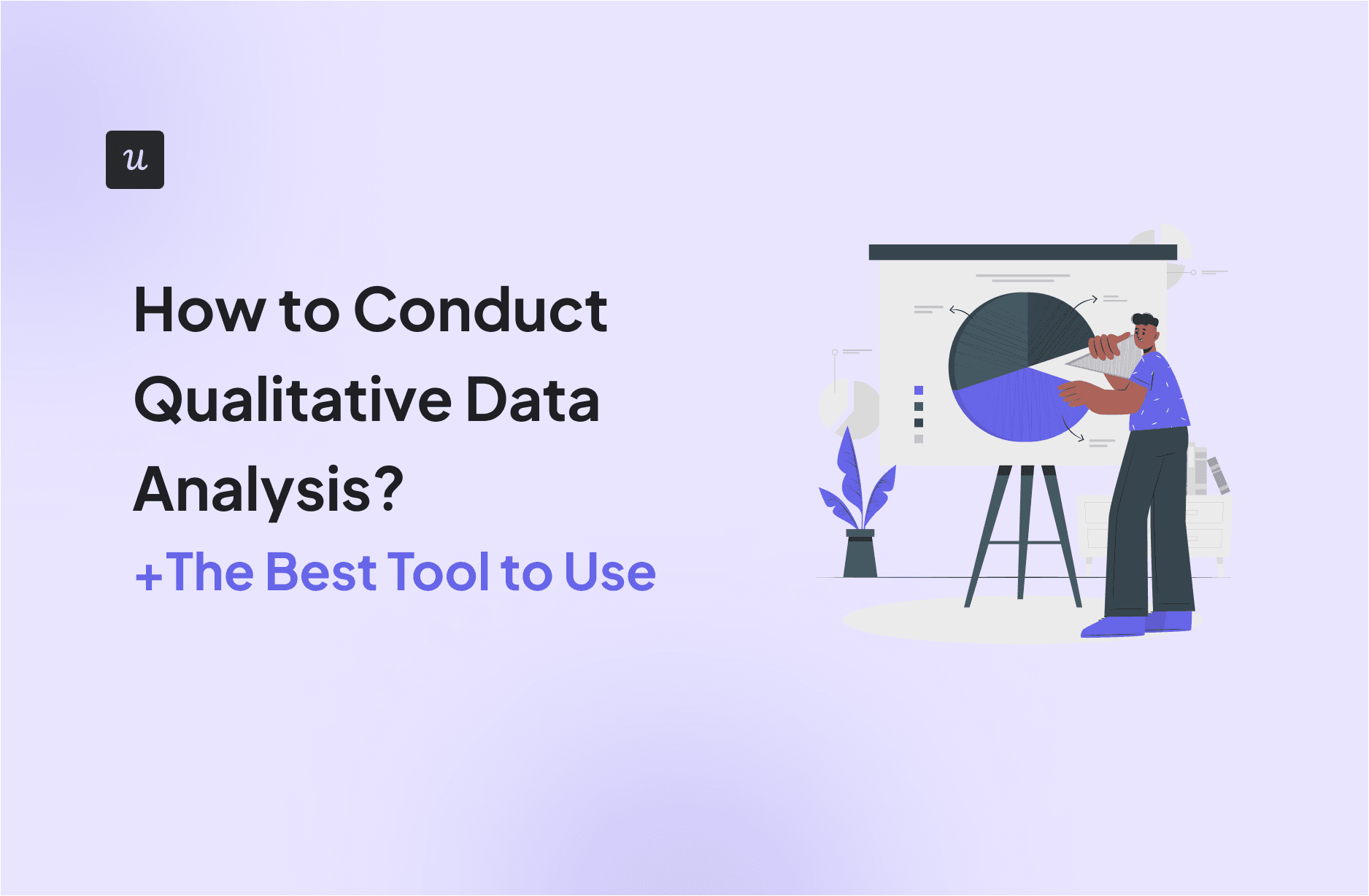
Let’s face it: qualitative data analysis is vital to understanding why users act in a particular way and how they feel about your product in a way that quantitative product analytics can’t.
This article will teach you how to analyze qualitative data to inform product development and improve the product experience.
You will discover:
- Five qualitative data analysis methods.
- A six-step analysis process and how to streamline it with Userpilot.
- How to act on your research findings.
What is qualitative data analysis?
Qualitative data analysis (QDA) involves organizing, examining, and interpreting non-numerical data collected from customers . For example, their survey responses or session recordings.
The purpose?
To understand their interactions with the product and gain in-depth insights into user pain points , unmet needs, motivations, and expectations.
Quantitative data analysis vs. qualitative data analysis
Quantitative and qualitative data analyses have different objectives and use different data types, methods, and tools to achieve them.
- The aim of quantitative research is to illustrate objectively what happens inside the product, while qualitative research focuses on the why . For example, quantitative data can reveal the percentage of users who drop off at a particular touchpoint, whereas qualitative data explains the reasons for that.
- Quantitative data analysis uses numerical and measurable data, such as event occurrences. In contrast, qualitative data analysis relies on non-numerical and descriptive data, like open-ended survey responses .
- To collect quantitative data, you use closed-ended survey questions and analytics tools to track user actions . Qualitative data, on the other hand, comes from open-ended survey questions , interviews, focus groups, phone calls and chats, and session recordings.
- Analyzing quantitative data involves visualizing it in charts and graphs and conducting statistical operations. Qualitative analysis is about finding themes and patterns in data, often manually.
Benefits of qualitative data analysis
Here are the key advantages of qualitative data analysis that underscore its importance in customer and market research :
- Deep insights : Qualitative analysis helps understand complex patterns by looking at reasons and perspectives behind the data.
- Flexibility : It allows researchers to adjust their approach as they continuously discover new information or themes.
- Contextual understanding : It explores contextual factors, which adds depth to number-based findings and reveals hidden connections.
- Participant voice : It highlights what participants actually say, experience, and feel and uses their input to shape the analysis and the results.
Challenges of qualitative data analysis
Let’s get it straight: qualitative data analysis comes with its challenges. The key ones include:
- Data overload and management : Qualitative data analysis involves tons of text or multimedia, which is challenging to organize, manage, and analyze.
- Reliability and validity : Ensuring the reliability and validity of qualitative findings is difficult because the process isn’t as standardized as quantitative analysis. Personal biases can skew the results.
- Time-intensive nature : Qualitative data analysis is resource-intensive and time-consuming. It involves iterative processes of coding, categorizing, and synthesizing data.
What are the 5 qualitative data analysis methods?
There are 5 main methods of qualitative research studies:
- Content analysis.
- Narrative analysis.
- Discourse analysis.
- Thematic analysis.
- Grounded theory analysis.
Mind you, the differences between them aren’t always clear-cut, and there is always some overlap.

1. Content analysis
Content analysis is a qualitative data analysis method that systematically evaluates a text to identify specific features or patterns. This could be anything from a customer interview transcript to survey responses, social media posts, or customer success calls.
The first step in the content analysis research method is data coding, or labeling and categorizing.
For example, if you are looking at customer feedback , you might code all mentions of “price” as “P,” all mentions of “quality” as “Q,” and so on.
Once manual coding is done, start looking for patterns and trends in the codes.
While it’s a qualitative approach, it often aims to quantify the content, for example, by counting how many times a word or theme appears.
One of the main advantages of content analysis is its relative simplicity – anyone with a good understanding of the data can do it.

The advantages of the content analysis process
- Content analysis can provide rich insights into how customers feel about your product, what their unmet needs are, and their motives.
- Once you have developed a coding system, content analysis is relatively quick and easy because it’s a systematic process.
- Content analysis requires very little investment since all you need is a good understanding of the data, and it doesn’t require any specialist qualitative research data analysis software .
The disadvantages of the content analysis process
- Coding data takes time, particularly if you have large amounts to analyze.
- Content analysis can ignore the context in which the data was collected. This may lead to misinterpretations.
- Some people argue that content analysis is a reductive approach to qualitative data because it involves breaking the data down into smaller pieces and quantifying, which can lead to oversimplifications.
2. Narrative analysis
Narrative analysis involves identifying, analyzing, and interpreting customer stories, for example, in the form of customer interviews or testimonials.
This kind of analysis helps product managers understand customers’ feelings toward the product, identify trends in customer behavior, and personalize their in-app experiences .
The advantages of narrative analysis
- The stories people tell give a deep understanding of customers’ needs and pain points.
- It collects unique, in-depth data based on customer stories.
The disadvantages of narrative analysis
- Hard to implement in large-scale studies.
- Transcribing customer interviews or testimonials is labor-intensive.
- Impossible to replicate as it relies on unique customer stories and your ability to interpret them.
3. Discourse analysis
Discourse analysis is about understanding how people communicate with each other. You can use it to analyze written or spoken language.
For instance, product teams can use discourse analysis to understand how customers talk about their products on the web.
The advantages of discourse analysis
- Uncovers emotions behind customers’ words.
- Gives insights into customer data.
The disadvantages of disclosure analysis
- Takes a lot of time and effort as the process is highly specialized and requires training and practice. There’s no “right” way to do it.
- Focuses solely on language.
4. Thematic analysis
Thematic analysis is similar to content analysis.
It also looks for patterns and themes in qualitative data and involves labeling and categorizing the data.
The difference?
It focuses on the meaning of the data and how the themes relate to the research questions .
You can pair it with sentiment analysis to determine whether a piece of writing is positive, negative, or neutral. This is done using a lexicon (i.e., a list of words and their associated sentiment scores).
SaaS companies use thematic analysis to analyze qualitative NPS survey responses to identify patterns among their customer base.
The advantages of thematic analysis
- Anyone with little training on how to label the data can perform thematic analysis.
- Survey or customer interview raw data can be easily converted into insights and quantitative data with the help of labeling.
- If done automatically, it’s an effective way to process large amounts of data (you need AI tools for this).
The disadvantages of thematic analysis
- If the data isn’t coded correctly, it can be difficult to identify themes since it’s a phrase-based method.
- It’s difficult to implement from scratch because balancing the themes and categories is tricky. They shouldn’t be too generic or too large.
5. Grounded theory analysis
Grounded theory analysis is a method used by qualitative researchers when there is little existing theory on the topic. Instead of starting with hypotheses and testing them through research, it involves generating them from the data on the fly as it emerges.
The grounded theory approach is useful for product managers who want to understand how customers interact with their products. You can also use it to generate hypotheses about how customers will behave in the future.
Suppose product teams want to understand the reasons behind the high churn rate . They can use customer surveys and grounded theory to analyze responses and develop hypotheses about why users churn and how to reengage inactive ones .
The advantages of grounded theory analysis
- It reduces bias because it doesn’t rely on assumptions.
- It’s great for analyzing poorly researched topics.
The disadvantages of grounded theory analysis
- It can come across as overly theoretical.
- It requires a lot of objectivity, creativity, and critical thinking.
Which qualitative data analysis method should you choose?
The choice of the appropriate qualitative data analysis method depends on various factors, including:
- Research question : Different qualitative methods are suitable for different research questions. For example, you can use content analysis to categorize and quantify customer feedback from support tickets to prioritize areas for improvement. Contrastingly, discourse analysis will show you how users feel about your product.
- Nature of data : Choose qualitative data analysis techniques that align with the data’s characteristics. For instance, content analysis is versatile and can be applied to various types of qualitative data, while narrative analysis focuses specifically on stories and narratives.
- Researcher expertise : Consider your own skills and expertise in qualitative analysis techniques. Some methods may require specialized training or familiarity with specific software tools . Choose a method that you feel comfortable with and confident in applying effectively.
- Research goals and resources : Evaluate your research goals, timeline, and resources available for analysis. Consider the balance between the depth of analysis and practical constraints. For example, narrative analysis is more time-consuming or resource-intensive than others.

Take Advantage of Userpilot’s Qualitative Data Analysis Today
- 14 Day Trial
- No Credit Card Required
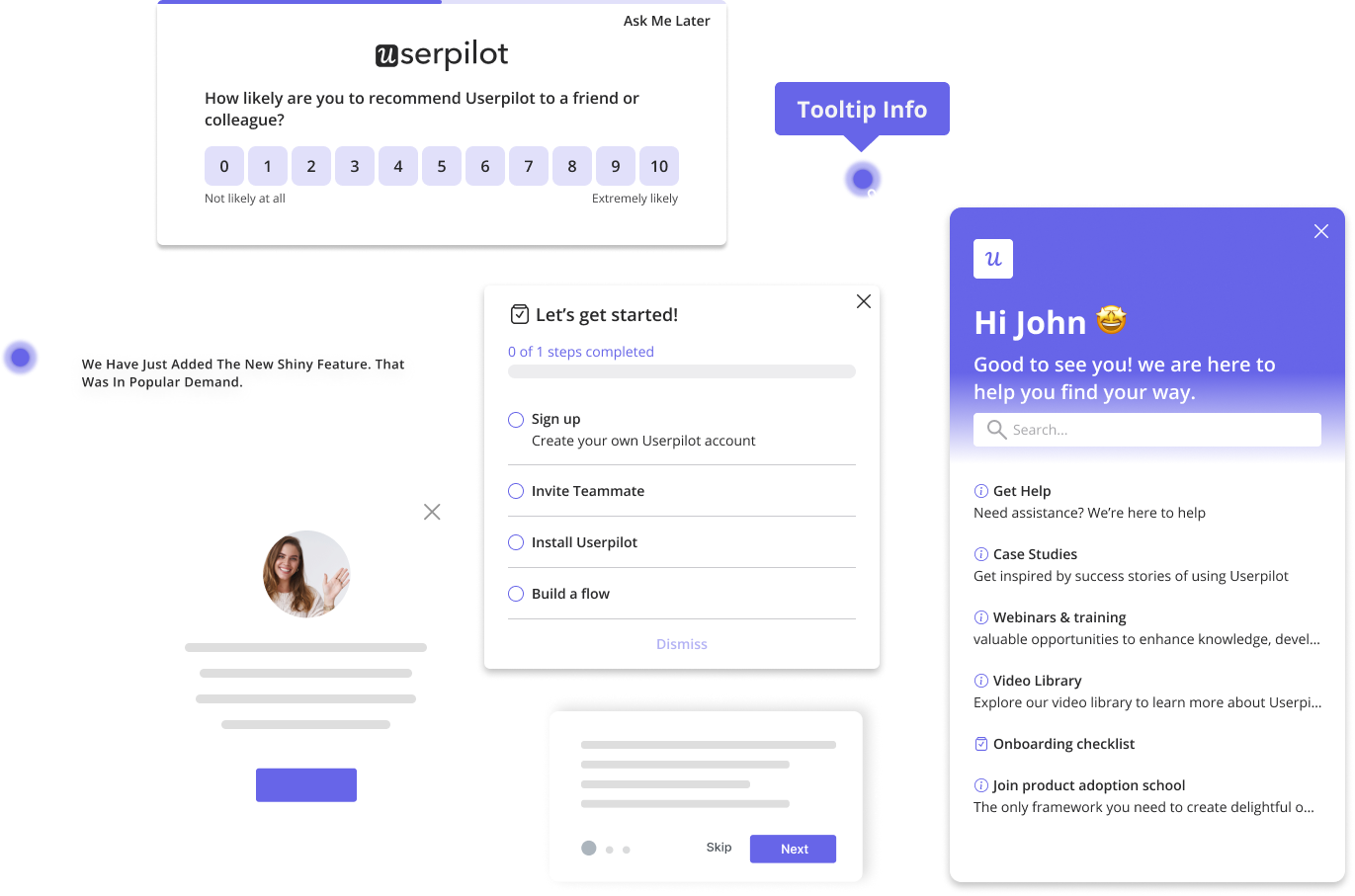
How to perform qualitative data analysis? A step-by-step process
With all that qualitative research methods covered, it’s time for our step-by-step guide to qualitative data analysis.
Step 1: Define your qualitative research questions
It’s important to be as specific as possible with the questions, as this will guide how you collect qualitative research data and the rest of your analysis.
Examples are:
- What are the primary reasons for customer dissatisfaction with our product?
- How does X group of users feel about our new feature?
- What are our customers’ needs, and how do they vary by segment?
- How do our products fit into our customers’ lives?
- What factors influence the low usage rate of the new feature ?
Step 2: Gather your qualitative customer data
Now, you decide what type of data collection to use based on previously defined goals.
Here are 5 methods to collect qualitative data for product companies:
- In-app surveys : triggered at a specific time or contextually, when the user completes an action, they allow you to reach product users (not account managers) and to collect qualitative data at scale. They should include both closed-ended and open-ended follow-up questions.
- Email surveys : delivered to users’ mailboxes, these surveys tend to have lower response rates than in-app surveys, but they’re sometimes the only way to reach inactive users .

- Review sites : Customer reviews on sites like G2, Capterra, or Clutch are often unfiltered and give you honest insights into customer sentiment and how to improve the product .

- User interviews : one-to-one conversations with customers give you the flexibility that no survey offers. A skilled interviewer can follow up on responses to help users get to the root cause of an issue, even if they’re not very good at articulating their thoughts or reflecting on their behaviors.

- Focus groups : Just like interviews, these moderated group discussions can offer valuable insights about your product and customer preferences . They aren’t very scalable, though.
Pro tip : Use a mix of in-app surveys and in-person interviews to collect qualitative data. For example, send regular NPS surveys to customers to track their sentiment over time. Segment your detractors and invite them to interviews to better understand why they’re dissatisfied with the product.
Step 3: Organize and categorize collected data
Before analyzing customer feedback and assigning any value, organize the unstructured feedback data in a single place. This is to easily detect patterns and similar themes.
One way to do this is to create a spreadsheet with all the data organized by research questions. Then, arrange the data by theme or category within each research question.
Or use Userpilot’s NPS response tagging to group similar responses. For example, you can tag all feedback about technical issues with “bug.”

Leverage Userpilot’s Qualitative Data Analysis Today
Step 4: use qualitative data coding to identify themes and patterns.
Themes in data analysis help you organize and make sense of your information.
In SaaS products, common themes from customer feedback might include:
- Product issues ( bugs or defects).
- Pricing concerns.
- Customer service experiences.
- Usability problems.
- User interface (UI) design.
- User experience (UX) issues.
- Missing features.
Identifying specific themes is just a start. You also need to identify their patterns: how often they occur, when, and who is affected. For example, if users complain about a missing feature, find out how many and what their JTBDs are.
You can detect those patterns using survey analytics .

Step 5: Act on the insights to improve product metrics
Once you understand the nature of the problem, look for ways to resolve it.
How you act on feedback depends on the issues.
Imagine your users request a feature . But it turns out you have a similar feature that allows them to complete the same task – they just haven’t found it. So, the solution is redesigning your onboarding process to improve feature discovery .
And if you have to build the feature, don’t just blindly follow customer requests or what competitors are doing – look for innovative solutions to get the edge.

Step 6: Continuously analyze qualitative data to stay ahead of changing customer needs
Qualitative data analysis isn’t a one-off exercise. As customer needs constantly evolve, you must always keep your hand on the pulse.
Collect customer feedback in-app at regular intervals and make a habit of interviewing customers all the time, even if you’re not planning to launch new features or products.

Qualitative data analysis example: How Unolo reduced customer churn with Userpilot NPS survey
One of our customers, Unolo, faced a challenge: a high month-on-month churn rate of around 3%.
They implemented in-app NPS surveys to analyze qualitative and quantitative responses about why their customers were leaving.
In addition to collecting the feedback in-app, their customer success team also reached out to the dissatisfied customers directly.
What was the outcome?
UX and product experience improvements that reduced the churn by up to 1%!

How to perform qualitative data analysis with Userpilot?
Userpilot is a product growth platform with advanced feedback, analytics, and engagement features. So you can use it to collect qualitative and quantitative data and act on the insights to improve user experience .

Collect qualitative feedback from users with in-app surveys
You can collect qualitative user data in Userpilot through in-app surveys .
Thanks to the template library and visual editor, creating customized surveys takes minutes.
Once ready, there are two ways to trigger them.
One is by setting the date, time, and page where the user should see it. Great for regular surveys, like CSAT or NPS .
The other is through event-based triggering. That’s when you link the survey to another user action in-app, for example, using a feature. That’s how you can measure their satisfaction with specific product aspects and collect ideas on how to improve them.
In both instances, you can choose to send the survey to specific user segments .
Finally, Userpilot offers localization functionality, so you can automatically translate the survey into multiple languages for users around the globe.
Analyze data no-code using survey analytics
Once you collect the data, you can easily analyze it without any coding.
Userpilot offers you survey analytics where you can quickly view the results and analyze user engagement with the surveys.
And let’s not forget about the NPS dashboard , which provides a comprehensive overview of your NPS scores over time, allowing you to track changes and trends in user loyalty and advocacy.
And don’t worry, Userpilot does all the NPS analysis for you: calculates the overall score and segments users into detractors, passives , and promoters.
You can also tag the NPS responses to identify common themes.

Gather customer insights via chatbots
With Userpilot, you can embed a chatbot in the resource center .
This serves two purposes:
- It allows you to support your users in-app and help them overcome issues that could lower their satisfaction and potentially lead to churn .
- You can also use it to collect qualitative customer feedback .

Build different in-app experiences based on the insights from qualitative data analysis
By analyzing qualitative feedback collected through in-app surveys , you can segment users based on these insights and create targeted in-app experiences designed to address specific user concerns or enhance key workflows.
For example, you can personalize the onboarding experiences for different user personas so that they discover the most relevant features as quickly as possible. Or use interactive walkthroughs to guide them through challenging processes.

The qualitative data analysis process is iterative and should be revisited as new data is collected. The goal is to constantly refine your understanding of your customer base and how they interact with your product.
Want to get started with qualitative analysis? Get a Userpilot demo and automate the qualitative data collection process. Save time on mundane work and understand your customers better!
Build a Qualitative Data Analysis Process Code-Free with Userpilot
Leave a comment cancel reply.
Save my name, email, and website in this browser for the next time I comment.
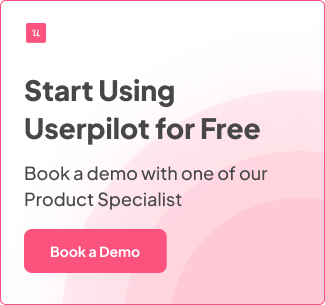
Get The Insights!
The fastest way to learn about Product Growth,Management & Trends.
The coolest way to learn about Product Growth, Management & Trends. Delivered fresh to your inbox, weekly.

The fastest way to learn about Product Growth, Management & Trends.
You might also be interested in ...
Best customer feedback examples from saas companies.
Userpilot Content Team
80+ Good Survey Questions & Question Types for Collecting Insights
11 types of survey questions for collecting actionable insights.
Aazar Ali Shad
We Trust in Human Precision
20,000+ Professional Language Experts Ready to Help. Expertise in a variety of Niches.
API Solutions
- API Pricing
- Cost estimate
- Customer loyalty program
- Educational Discount
- Non-Profit Discount
- Green Initiative Discount1
Value-Driven Pricing
Unmatched expertise at affordable rates tailored for your needs. Our services empower you to boost your productivity.

- Special Discounts
- Enterprise transcription solutions
- Enterprise translation solutions
- Transcription/Caption API
- AI Transcription Proofreading API
Trusted by Global Leaders
GoTranscript is the chosen service for top media organizations, universities, and Fortune 50 companies.
GoTranscript
One of the Largest Online Transcription and Translation Agencies in the World. Founded in 2005.
Speaker 1: In this video, we're going to look at the ever popular qualitative analysis method, thematic analysis. We'll unpack what thematic analysis is, explore its strengths and weaknesses, and explain when and when not to use it. By the end of the video, you'll have a clearer understanding of thematic analysis so that you can approach your research project with confidence. By the way, if you're currently working on a dissertation or thesis or research project, be sure to grab our free dissertation templates to help fast-track your write-up. These tried and tested templates provide a detailed roadmap to guide you through each chapter, section by section. If that sounds helpful, you can find the link in the description down below. So, first things first, what is thematic analysis? Well, as the name suggests, thematic analysis, or TA for short, is a qualitative analysis method focused on identifying patterns, themes, and meanings within a data set. Breaking that down a little, TA involves interpreting language-based data to uncover categories or themes that relate to the research aims and research questions of the study. This data could be taken from interview transcripts, open-ended survey responses, or even social media posts. In other words, thematic analysis can be used on both primary and secondary data. Let's look at an example to make things a little more tangible. Assume you're researching customer sentiment toward a newly launched product line. Using thematic analysis, you could review open-ended survey responses from a sample of consumers looking for similarities, patterns, and categories in the data. These patterns would form a foundation for the development of an initial set of themes. You'd then reduce and synthesize these themes by filtering them through the lens of your specific research aims until you have a small number of key themes that help answer your research questions. By the way, if you're not familiar with the concept of research aims and research questions, be sure to check out our primer video covering that. Link in the description. Now that we've defined what thematic analysis is, let's unpack the different forms that TA can take, specifically inductive and deductive. Your choice of approach will make a big difference to the analysis process, so it's important to understand the difference. Let's take a look at each of them. First up is inductive thematic analysis. This type of TA is completely bottom-up, inductive in terms of approach. In other words, the codes and themes will emerge exclusively from your analysis of the data as you work through it rather than being determined beforehand. This makes it a relatively flexible approach as you can adjust, remove, or add codes and themes as you become more familiar with your data. For example, you could use inductive TA to conduct research on staff experiences of a new office space. In this case, you'd conduct interviews and begin developing codes based on the initial patterns you observe. You could then adjust or change these codes on an iterative basis as you become more familiar with the full data set, following which you develop your themes. By the way, if you're not familiar with the process of qualitative coding, we've got a dedicated video covering that. As always, the link is in the description. Next up, we've got deductive thematic analysis. Contrasted to the inductive option, deductive TA uses predetermined, tightly defined codes. These codes, often referred to as a priori codes, are typically drawn from the study's theoretical framework, as well as empirical research and the researcher's knowledge of the situation. Typically, these codes would be compiled into a codebook where each code would be clearly defined and scoped. As an example, your research might aim to assess constituent opinions regarding local government policy. Applying deductive thematic analysis here would involve developing a list of tightly defined codes in advance based on existing theory and knowledge. Those codes would then be compiled into a codebook and applied to interview data collected from constituents. Importantly, throughout the coding and analysis process, those codes and their descriptions would remain fixed. It's worth mentioning that deductive thematic analysis can be undertaken both individually or by multiple researchers. The latter is referred to as coding reliability TA. As the name suggests, this approach aims to achieve a high level of reliability with regard to the application of codes. By having multiple researchers apply the same set of codes to the same data set, inconsistencies in interpretation can be ironed out and a higher level of reliability can be reached. By the way, qualitative coding is something that we regularly help students with here at Grad Coach, so if you'd like a helping hand with your research project, be sure to check out the link that's down in the description. All right, we've covered quite a lot here. To recap, thematic analysis can be conducted using either an inductive approach where your codes naturally emerge from the data or a deductive approach where your codes are independently or collaboratively developed before analyzing the data. So now that we've unpacked the different types of thematic analysis, it's important to understand the broader strengths and weaknesses of this method so that you know when and when not to use it. One of the main strengths of thematic analysis is the relative simplicity with which you can derive codes and themes and, by extension, conclusions. Whether you take an inductive or a deductive approach, identifying codes and themes can be an easier process with thematic analysis than with some other methods. Discourse analysis, for example, requires both an in-depth analysis of the data and a strong understanding of the context in which that data was collected, demanding a significant time investment. Flexibility is another major strength of thematic analysis. The relatively generic focus on identifying patterns and themes allows TA to be used on a broad range of research topics and data types. Whether you're undertaking a small sociological study with a handful of participants or a large market research project with hundreds of participants, thematic analysis can be equally effective. Given these attributes, thematic analysis is best used in projects where the research aims involve identifying similarities and patterns across a wide range of data. This makes it particularly useful for research topics centered on understanding patterns of meaning expressed in thoughts, beliefs, and opinions. For example, research focused on identifying the thoughts and feelings of an audience in response to a new ad campaign might utilize TA to find patterns in participant responses. All that said, just like any analysis method, thematic analysis has its shortcomings and isn't suitable for every project. First, the inherent flexibility of TA also means that results can at times be kind of vague and imprecise. In other words, the broad applicability of this method means that the patterns and themes you draw from your data can potentially lack the sensitivity to incorporate text and contradiction. Second is the problem of inconsistency and lack of rigor. Put another way, the simplicity of thematic analysis can sometimes mean it's a little too crude for more delicate research aims. Specifically, the focus on identifying patterns and themes can lead to results that lack nuance. For example, even an inductive thematic analysis applied to a sample of just 10 participants might overlook some of the subtle nuances of participant responses in favor of identifying generalized themes. It could also miss fine details in language and expression that might reveal counterintuitive but more accurate implications. All that said, thematic analysis is still a useful method in many cases, but it's important to assess whether it fits your needs. So think carefully about what you're trying to achieve with your research project. In other words, your research aims and research questions. And be sure to explore all the options before choosing an analysis method. If you need some inspiration, we've got a video that unpacks the most popular qualitative analysis methods. Link is in the description. If you're enjoying this video so far, please help us out by hitting that like button. You can also subscribe for loads of plain language actionable advice. If you're new to research, check out our free dissertation writing course, which covers everything you need to get started on your research project. As always, links in the description. Okay, that was a lot. So let's do a quick recap. Thematic analysis is a qualitative analysis method focused on identifying patterns of meaning as themes within data, whether primary or secondary. As we've discussed, there are two overarching types of thematic analysis. Inductive TA, in which the codes emerge from an initial review of the data itself and are revised as you become increasingly familiar with the data. And deductive TA, in which the codes are determined beforehand based on a combination of the theoretical and or conceptual framework, empirical studies, and prior knowledge. As with all things, thematic analysis has its strengths and weaknesses and based on those is generally most appropriate for research focused on identifying patterns in data and drawing conclusions in relation to those. If you liked the video, please hit that like button to help more students find this content. For more videos like this one, check out the Grad Coach channel and make sure you subscribe for plain language, actionable research tips and advice every week. Also, if you're looking for one-on-one support with your dissertation, thesis, or research project, be sure to check out our private coaching service where we hold your hand throughout the research process step by step. You can learn more about that and book a free initial consultation at gradcoach.com.

Ohio State nav bar
The Ohio State University
- BuckeyeLink
- Find People
- Search Ohio State

- Ohio State University Libraries
- Research Guides
- Qualitative Research
- General Library Information
- Databases and Websites
- Color Theory
- Sustainability & Design
- Trend Forecasting
- Career Information for Designers
- Citing Sources
Analysis Tools
- dedoose No OSU subscription acess, but is an inexpensive web-based option, particularly good for collaborative projects.
- NVivo NVivo, produced by Lumivero, is a computer-assisted qualitative data analysis software (CAQDAS) program. It is one of the CAQDAS programs popular among education researchers.
- Taguette Free and open source data analysis tool.
- Transana Transana is a computer-assisted qualitative data analysis software (CAQDAS) program. It is specifically designed to analyze digital video or audio data.
Intro to Qualitative Research
"Qualitative inquiries are framed by interpretive approaches that include a growing and necessary interest in critical theories, such as feminism, critical race, queer, disability, postcolonial, posthuman, and materiality studies, among others (Denzin & Lincoln, 2018). Qualitative research often focuses on transformation and social change (Creswell & Poth, 2018; Denzin & Lincoln, 2018; Mertens, 2019)."
Patricia Leavy in An Introductory Guide to Qualitative Research in Art Museums , p. 7
Some methods of qualitative research :
- Arts-Based Research (ABR)
- Observation
- Focus Groups
- Case Studies
Resources for Qualitative Research at Ohio State
- OSU QualLab
- OSU Institutional Review Board (IRB)
- University Libraries Research Commons (18th Avenue Library)
Overview of Qualitative Methods in Collecting Research Data

Table Source : Duke University's Guide to Qualitative Research Methods , Accessed Sept. 2024
- The Sage Encyclopedia of Qualitative Research Methods by Lisa M. Given, editor Publication Date: 2008
- << Previous: Trend Forecasting
- Next: Career Information for Designers >>

© The Ohio State University - University Libraries 1858 Neil Avenue Mall, Columbus, OH 43210 Phone: (614) 292-OSUL (6785) | Fax: (614) 292-9101
Request an alternate format of this page | Accessibility | Privacy Policy
Print Page Login to LibApps

An official website of the United States government
The .gov means it’s official. Federal government websites often end in .gov or .mil. Before sharing sensitive information, make sure you’re on a federal government site.
The site is secure. The https:// ensures that you are connecting to the official website and that any information you provide is encrypted and transmitted securely.
- Publications
- Account settings
The PMC website is updating on October 15, 2024. Learn More or Try it out now .
- Advanced Search
- Journal List
- Malays Fam Physician
- v.3(1); 2008
Data Analysis in Qualitative Research: A Brief Guide to Using Nvivo
MSc, PhD, Faculty of Medicine, University of Malaya, Kuala Lumpur, Malaysia
Qualitative data is often subjective, rich, and consists of in-depth information normally presented in the form of words. Analysing qualitative data entails reading a large amount of transcripts looking for similarities or differences, and subsequently finding themes and developing categories. Traditionally, researchers ‘cut and paste’ and use coloured pens to categorise data. Recently, the use of software specifically designed for qualitative data management greatly reduces technical sophistication and eases the laborious task, thus making the process relatively easier. A number of computer software packages has been developed to mechanise this ‘coding’ process as well as to search and retrieve data. This paper illustrates the ways in which NVivo can be used in the qualitative data analysis process. The basic features and primary tools of NVivo which assist qualitative researchers in managing and analysing their data are described.
QUALITATIVE RESEARCH IN MEDICINE
Qualitative research has seen an increased popularity in the last two decades and is becoming widely accepted across a wide range of medical and health disciplines, including health services research, health technology assessment, nursing, and allied health. 1 There has also been a corresponding rise in the reporting of qualitative research studies in medical and health related journals. 2
The increasing popularity of qualitative methods is a result of failure of quantitative methods to provide insight into in-depth information about the attitudes, beliefs, motives, or behaviours of people, for example in understanding the emotions, perceptions and actions of people who suffer from a medical condition. Qualitative methods explore the perspective and meaning of experiences, seek insight and identify the social structures or processes that explain people”s behavioural meaning. 1 , 3 Most importantly, qualitative research relies on extensive interaction with the people being studied, and often allows researchers to uncover unexpected or unanticipated information, which is not possible in the quantitative methods. In medical research, it is particularly useful, for example, in a health behaviour study whereby health or education policies can be effectively developed if reasons for behaviours are clearly understood when observed or investigated using qualitative methods. 4
ANALYSING QUALITATIVE DATA
Qualitative research yields mainly unstructured text-based data. These textual data could be interview transcripts, observation notes, diary entries, or medical and nursing records. In some cases, qualitative data can also include pictorial display, audio or video clips (e.g. audio and visual recordings of patients, radiology film, and surgery videos), or other multimedia materials. Data analysis is the part of qualitative research that most distinctively differentiates from quantitative research methods. It is not a technical exercise as in quantitative methods, but more of a dynamic, intuitive and creative process of inductive reasoning, thinking and theorising. 5 In contrast to quantitative research, which uses statistical methods, qualitative research focuses on the exploration of values, meanings, beliefs, thoughts, experiences, and feelings characteristic of the phenomenon under investigation. 6
Data analysis in qualitative research is defined as the process of systematically searching and arranging the interview transcripts, observation notes, or other non-textual materials that the researcher accumulates to increase the understanding of the phenomenon. 7 The process of analysing qualitative data predominantly involves coding or categorising the data. Basically it involves making sense of huge amounts of data by reducing the volume of raw information, followed by identifying significant patterns, and finally drawing meaning from data and subsequently building a logical chain of evidence. 8
Coding or categorising the data is the most important stage in the qualitative data analysis process. Coding and data analysis are not synonymous, though coding is a crucial aspect of the qualitative data analysis process. Coding merely involves subdividing the huge amount of raw information or data, and subsequently assigning them into categories. 9 In simple terms, codes are tags or labels for allocating identified themes or topics from the data compiled in the study. Traditionally, coding was done manually, with the use of coloured pens to categorise data, and subsequently cutting and sorting the data. Given the advancement of software technology, electronic methods of coding data are increasingly used by qualitative researchers.
Nevertheless, the computer does not do the analysis for the researchers. Users still have to create the categories, code, decide what to collate, identify the patterns and draw meaning from the data. The use of computer software in qualitative data analysis is limited due to the nature of qualitative research itself in terms of the complexity of its unstructured data, the richness of the data and the way in which findings and theories emerge from the data. 10 The programme merely takes over the marking, cutting, and sorting tasks that qualitative researchers used to do with a pair of scissors, paper and note cards. It helps to maximise efficiency and speed up the process of grouping data according to categories and retrieving coded themes. Ultimately, the researcher still has to synthesise the data and interpret the meanings that were extracted from the data. Therefore, the use of computers in qualitative analysis merely made organisation, reduction and storage of data more efficient and manageable. The qualitative data analysis process is illustrated in Figure 1 .

Qualitative data analysis flowchart
USING NVIVO IN QUALITATIVE DATA ANALYSIS
NVivo is one of the computer-assisted qualitative data analysis softwares (CAQDAS) developed by QSR International (Melbourne, Australia), the world’s largest qualitative research software developer. This software allows for qualitative inquiry beyond coding, sorting and retrieval of data. It was also designed to integrate coding with qualitative linking, shaping and modelling. The following sections discuss the fundamentals of the NVivo software (version 2.0) and illustrates the primary tools in NVivo which assist qualitative researchers in managing their data.
Key features of NVivo
To work with NVivo, first and foremost, the researcher has to create a Project to hold the data or study information. Once a project is created, the Project pad appears ( Figure 2 ). The project pad of NVivo has two main menus: Document browser and Node browser . In any project in NVivo, the researcher can create and explore documents and nodes, when the data is browsed, linked and coded. Both document and node browsers have an Attribute feature, which helps researchers to refer the characteristics of the data such as age, gender, marital status, ethnicity, etc.

Project pad with documents tab selected
The document browser is the main work space for coding documents ( Figure 3 ). Documents in NVivo can be created inside the NVivo project or imported from MS Word or WordPad in a rich text (.rtf) format into the project. It can also be imported as a plain text file (.txt) from any word processor. Transcripts of interview data and observation notes are examples of documents that can be saved as individual documents in NVivo. In the document browser all the documents can be viewed in a database with short descriptions of each document.

Document browser with coder and coding stripe activated
NVivo is also designed to allow the researcher to place a Hyperlink to other files (for example audio, video and image files, web pages, etc.) in the documents to capture conceptual links which are observed during the analysis. The readers can click on it and be taken to another part of the same document, or a separate file. A hyperlink is very much like a footnote.
The second menu is Node explorer ( Figure 4 ), which represents categories throughout the data. The codes are saved within the NVivo database as nodes. Nodes created in NVivo are equivalent to sticky notes that the researcher places on the document to indicate that a particular passage belongs to a certain theme or topic. Unlike sticky notes, the nodes in NVivo are retrievable, easily organised, and give flexibility to the researcher to either create, delete, alter or merge at any stage. There are two most common types of node: tree nodes (codes that are organised in a hierarchical structure) and free nodes (free standing and not associated with a structured framework of themes or concepts). Once the coding process is complete, the researcher can browse the nodes. To view all the quotes on a particular Node, select the particular node on the Node Explorer and click the Browse button ( Figure 5 ).

Node explorer with a tree node highlighted

Browsing a node
Coding in NVivo using Coder
Coding is done in the document browser. Coding involves the desegregation of textual data into segments, examining the data similarities and differences, and grouping together conceptually similar data in the respective nodes. 11 The organised list of nodes will appear with a click on the Coder button at the bottom of document browser window.
To code a segment of the text in a project document under a particular node, highlight the particular segment and drag the highlighted text to the desired node in the coder window ( Figure 3 ). The segments that have been coded to a particular node are highlighted in colours and nodes that have attached to a document turns bold. Multiple codes can be assigned to the same segment of text using the same process. Coding Stripes can be activated to view the quotes that are associated with the particular nodes. With the guide of highlighted text and coding stripes, the researcher can return to the data to do further coding or refine the coding.
Coding can be done with pre-constructed coding schemes where the nodes are first created using the Node explorer followed by coding using the coder. Alternatively, a bottom-up approach can be used where the researcher reads the documents and creates nodes when themes arise from the data as he or she codes.
Making and using memos
In analysing qualitative data, pieces of reflective thinking, ideas, theories, and concepts often emerge as the researcher reads through the data. NVivo allows the user the flexibility to record ideas about the research as they emerge in the Memos . Memos can be seen as add-on documents, treated as full status data and coded like any other documents. 12 Memos can be placed in a document or at a node. A memo itself can have memos (e.g. documents or nodes) linked to it, using DocLinks and NodeLinks .
Creating attributes
Attributes are characteristics (e.g. age, marital status, ethnicity, educational level, etc.) that the researcher associates with a document or node. Attributes have different values (for example, the values of the attribute for ethnicity are ‘Malay’, ‘Chinese’ and ‘Indian’). NVivo makes it possible to assign attributes to either document or node. Items in attributes can be added, removed or rearranged to help the researcher in making comparisons. Attributes are also integrated with the searching process; for example, linking the attributes to documents will enable the researcher to conduct searches pertaining to documents with specified characteristics ( Figure 6 ).

Document attribute explorer
Search operation
The three most useful types of searches in NVivo are Single item (text, node, or attribute value), Boolean and Proximity searches. Single item search is particularly important, for example, if researchers want to ensure that every mention of the word ‘cure’ has been coded under the ‘Curability of cervical cancer’ tree node. Every paragraph in which this word is used can be viewed. The results of the search can also be compiled into a single document in the node browser and by viewing the coding stripe. The researcher can check whether each of the resulting passages has been coded under a particular node. This is particularly useful for the researcher to further determine whether conducting further coding is necessary.
Boolean searches combine codes using the logical terms like ‘and’, ‘or’ and ‘not’. Common Boolean searches are ‘or’ (also referred to as ‘combination’ or ‘union’) and ‘and’ (also called ‘intersection’). For example, the researcher may wish to search for a node and an attributed value, such as ‘ever screened for cervical cancer’ and ‘primary educated’. Search results can be displayed in matrix form and it is possible for the researcher to perform quantitative interpretations or simple counts to provide useful summaries of some aspects of the analysis. 13 Proximity searches are used to find places where two items (e.g. text patterns, attribute values, nodes) appear near each other in the text.
Using models to show relationships
Models or visualisations are an essential way to describe and explore relationships in qualitative research. NVivo provides a Modeler designated for visual exploration and explanation of relationships between various nodes and documents. In Model Explorer, the researcher can create, label and connect ideas or concepts. NVivo allows the user to create a model over time and have any number of layers to track the progress of theory development to enable the researcher to examine the stages in the model-building over time ( Figure 7 ). Any documents, nodes or attributes can be placed in a model and clicking on the item will enable the researcher to inspect its properties.

Model explorer showing the perceived risk factors of cervical cancer
NVivo has clear advantages and can greatly enhance research quality as outlined above. It can ease the laborious task of data analysis which would otherwise be performed manually. The software certainly removes the tremendous amount of manual tasks and allows more time for the researcher to explore trends, identify themes, and make conclusions. Ultimately, analysis of qualitative data is now more systematic and much easier. In addition, NVivo is ideal for researchers working in a team as the software has a Merge tool that enables researchers that work in separate teams to bring their work together into one project.
The NVivo software has been revolutionised and enhanced recently. The newly released NVivo 7 (released March 2006) and NVivo 8 (released March 2008) are even more sophisticated, flexible, and enable more fluid analysis. These new softwares come with a more user-friendly interface that resembles the Microsoft Windows XP applications. Furthermore, they have new data handling capacities such as to enable tables or images embedded in rich text files to be imported and coded as well. In addition, the user can also import and work on rich text files in character based languages such as Chinese or Arabic.
To sum up, qualitative research undoubtedly has been advanced greatly by the development of CAQDAS. The use of qualitative methods in medical and health care research is postulated to grow exponentially in years to come with the further development of CAQDAS.
More information about the NVivo software
Detailed information about NVivo’s functionality is available at http://www.qsrinternational.com . The website also carries information about the latest versions of NVivo. Free demonstrations and tutorials are available for download.
ACKNOWLEDGEMENT
The examples in this paper were adapted from the data of the study funded by the Ministry of Science, Technology and Environment, Malaysia under the Intensification of Research in Priority Areas (IRPA) 06-02-1032 PR0024/09-06.
TERMINOLOGY
Attributes : An attribute is a property of a node, case or document. It is equivalent to a variable in quantitative analysis. An attribute (e.g. ethnicity) may have several values (e.g. Malay, Chinese, Indian, etc.). Any particular node, case or document may be assigned one value for each attribute. Similarities within or differences between groups can be identified using attributes. Attribute Explorer displays a table of all attributes assigned to a document, node or set.
CAQDAS : Computer Aided Qualitative Data Analysis. The CAQDAS programme assists data management and supports coding processes. The software does not really analyse data, but rather supports the qualitative analysis process. NVivo is one of the CAQDAS programmes; others include NUDIST, ATLAS-ti, AQUAD, ETHNOGRAPH and MAXQDA.
Code : A term that represents an idea, theme, theory, dimension, characteristic, etc., of the data.
Coder : A tool used to code a passage of text in a document under a particular node. The coder can be accessed from the Document or Node Browser .
Coding : The action of identifying a passage of text in a document that exemplifies ideas or concepts and connecting it to a node that represents that idea or concept. Multiple codes can be assigned to the same segment of text in a document.
Coding stripes : Coloured vertical lines displayed at the right-hand pane of a Document ; each is named with title of the node at which the text is coded.
DataLinks : A tool for linking the information in a document or node to the information outside the project, or between project documents. DocLinks , NodeLinks and DataBite Links are all forms of DataLink .
Document : A document in an NVivo project is an editable rich text or plain text file. It may be a transcription of project data or it may be a summary of such data or memos, notes or passages written by the researcher. The text in a document can be coded, may be given values of document attributes and may be linked (via DataLinks ) to other related documents, annotations, or external computer files. The Document Explorer shows the list of all project documents.
Memo : A document containing the researcher”s commentary flagged (linked) on any text in a Document or Node. Any files (text, audio or video, or picture data) can be linked via MemoLink .
Model : NVivo models are made up of symbols, usually representing items in the project, which are joined by lines or arrows, designed to represent the relationship between key elements in a field of study. Models are constructed in the Modeller .
Node : Relevant passages in the project”s documents are coded at nodes. A Node represents a code, theme, or idea about the data in a project. Nodes can be kept as Free Nodes (without organisation) or may be organised hierarchically in Trees (of categories and subcategories). Free nodes are free-standing and are not associated to themes or concepts. Early on in the project, tentative ideas may be stored in the Free Nodes area. Free nodes can be kept in a simple list and can be moved to a logical place in the Tree Node when higher levels of categories are discovered. Nodes can be given values of attributes according to the features of what they represent, and can be grouped in sets. Nodes can be organised (created, edited) in Node Explorer (a window listing all the project nodes and node sets). The Node Browser displays the node”s coding and allow the researcher to change the coding.
Project : Collection of all the files, documents, codes, nodes, attributes, etc. associated with a research project. The Project pad is a window in NVivo when a project is open which gives access to all the main functions of the programme.
Sets : Sets in NVivo hold shortcuts to any nodes or documents, as a way of holding those items together without actually combining them. Sets are used primarily as a way of indicating items that in some way are related conceptually or theoretically. It provides different ways of sorting and managing data.
Tree Node : Nodes organised hierarchically into trees to catalogue categories and subcategories.

COMMENTS
QDA Method #1: Qualitative Content Analysis. Content analysis is possibly the most common and straightforward QDA method. At the simplest level, content analysis is used to evaluate patterns within a piece of content (for example, words, phrases or images) or across multiple pieces of content or sources of communication. For example, a collection of newspaper articles or political speeches.
Qualitative Research. Qualitative research is a type of research methodology that focuses on exploring and understanding people's beliefs, attitudes, behaviors, and experiences through the collection and analysis of non-numerical data. It seeks to answer research questions through the examination of subjective data, such as interviews, focus ...
Qualitative research is the opposite of quantitative research, which involves collecting and analyzing numerical data for statistical analysis. Qualitative research is commonly used in the humanities and social sciences, in subjects such as anthropology, sociology, education, health sciences, history, etc. Qualitative research question examples
Qualitative data analysis is a systematic process of examining non-numerical data to extract meaning, patterns, and insights. In contrast to quantitative analysis, which focuses on numbers and statistical metrics, the qualitative study focuses on the qualitative aspects of data, such as text, images, audio, and videos.
The SAGE Handbook of. tive Data AnalysisUwe FlickMapping the FieldData analys. s is the central step in qualitative research. Whatever the data are, it is their analysis that, in a de. isive way, forms the outcomes of the research. Sometimes, data collection is limited to recording and docu-menting naturally occurring ph.
Through a critical lens and the power of synthesis, 2 this guide navigates the complexities of qualitative research to provide a clear and structured pathway from conceptualization to implementation. This guide underscores the importance, necessity, and relevance of qualitative methods in addressing real-world issues, and emphasizes the urgency of equipping the next generation of researchers ...
Jessica Nina Lester is an associate professor of Counseling and Educational Psychology at Indiana University. She received her PhD from the University of Tennessee, Knoxville. Her research strand focuses on the study and development of qualitative research methodologies and methods at a theoretical, conceptual, and technical level.
Qualitative data analysis is an important part of research and building greater understanding across fields for a number of reasons. First, cases for qualitative data analysis can be selected purposefully according to whether they typify certain characteristics or contextual locations. In other words, qualitative data permits deep immersion into a topic, phenomenon, or area of interest.
How to conduct qualitative research? Given that qualitative research is characterised by flexibility, openness and responsivity to context, the steps of data collection and analysis are not as separate and consecutive as they tend to be in quantitative research [13, 14].As Fossey puts it: "sampling, data collection, analysis and interpretation are related to each other in a cyclical ...
Abstract. The Oxford Handbook of Qualitative Research, second edition, presents a comprehensive retrospective and prospective review of the field of qualitative research. Original, accessible chapters written by interdisciplinary leaders in the field make this a critical reference work. Filled with robust examples from real-world research ...
While many books and articles guide various qualitative research methods and analyses, there is currently no concise resource that explains and differentiates among the most common qualitative approaches. We believe novice qualitative researchers, students planning the design of a qualitative study or taking an introductory qualitative research course, and faculty teaching such courses can ...
Qualitative Data Analysis methods. Once all the data has been captured, there are a variety of analysis techniques available and the choice is determined by your specific research objectives and the kind of data you've gathered. Common qualitative data analysis methods include: Content Analysis. This is a popular approach to qualitative data ...
INTRODUCTION. Qualitative research methods refer to techniques of investigation that rely on nonstatistical and nonnumerical methods of data collection, analysis, and evidence production. Qualitative research techniques provide a lens for learning about nonquantifiable phenomena such as people's experiences, languages, histories, and cultures.
Qualitative research is a systematic inquiry method used to explore and understand social phenomena by examining the subjective experiences, behaviors, and perspectives of individuals or groups. Unlike quantitative research, which focuses on quantifiable variables and statistical analysis, qualitative research seeks to uncover rich, context ...
Qualitative data analysis research must be repeatable if it is to be relied on, and there should always be ways to check just what qualitative feedback particular trends and insights come from. When qualitative data analysis is done right, transparency and rigor can be maintained throughout the process, from the initial selection of research ...
Content analysis is the systematic analysis of the content of a text—e.g., who says what, to whom, why, and to what extent and with what effect—in a quantitative or qualitative manner. Content analysis is typically conducted as follows. First, when there are many texts to analyse—e.g., newspaper stories, financial reports, blog postings ...
Qualitative research is a type of research that explores and provides deeper insights into real-world problems.[1] Instead of collecting numerical data points or intervening or introducing treatments just like in quantitative research, qualitative research helps generate hypothenar to further investigate and understand quantitative data. Qualitative research gathers participants' experiences ...
Qualitative research is the naturalistic study of social meanings and processes, using interviews, observations, and the analysis of texts and images. In contrast to quantitative researchers, whose statistical methods enable broad generalizations about populations (for example, comparisons of the percentages of U.S. demographic groups who vote in particular ways), qualitative researchers use ...
This method of qualitative data analysis starts with an analysis of a single case to formulate a theory. Then, additional cases are examined to see if they contribute to the theory. Qualitative data analysis can be conducted through the following three steps: Step 1: Developing and Applying Codes. Coding can be explained as categorization of data.
This can be analyzed using mixed methods research, which combines both qualitative and quantitative research methods. Field notes: These are notes taken by researchers during observations, which can include descriptions of the setting, behaviors, and interactions of participants. Qualitative Data Analysis Methods
Given that qualitative research is characterised by flexibility, openness and responsivity to context, the steps of data collection and analysis are not as separate and consecutive as they tend to be in quantitative research [13, 14].As Fossey puts it: "sampling, data collection, analysis and interpretation are related to each other in a cyclical (iterative) manner, rather than following one ...
With all that qualitative research methods covered, it's time for our step-by-step guide to qualitative data analysis. Step 1: Define your qualitative research questions. It's important to be as specific as possible with the questions, as this will guide how you collect qualitative research data and the rest of your analysis. Examples are:
INTRODUCTION. In an earlier paper, 1 we presented an introduction to using qualitative research methods in pharmacy practice. In this article, we review some principles of the collection, analysis, and management of qualitative data to help pharmacists interested in doing research in their practice to continue their learning in this area.
Well, as the name suggests, thematic analysis, or TA for short, is a qualitative analysis method focused on identifying patterns, themes, and meanings within a data set. Breaking that down a little, TA involves interpreting language-based data to uncover categories or themes that relate to the research aims and research questions of the study.
Qualitative research often focuses on transformation and social change (Creswell & Poth, 2018; Denzin & Lincoln, 2018; Mertens, 2019)." Patricia Leavy in An Introductory Guide to Qualitative Research in Art Museums, p. 7. Some methods of qualitative research: Arts-Based Research (ABR) Surveys; Interviews; Observation; Focus Groups; Case Studies
In part due to misunderstandings about what qualitative psychological research is and the marginalisation of qualitative methods within the field of psychology (e.g., Riley et al., 2019), qualitative scholars have made significant efforts in producing guidance for qualitative researchers to improve the quality of qualitative research.It is recognized that identifying appropriate quality ...
This 2-day (12-hour) workshop provides an overview of how qualitative researchers think (research design) and what they do (research methods). Participants will gain hands-on experience with qualitative research by working through exercises related to their own current, past, or possible future projects. Group experiences are punctuated by brief discussions of key ideas in qualitative research.
In some cases, qualitative data can also include pictorial display, audio or video clips (e.g. audio and visual recordings of patients, radiology film, and surgery videos), or other multimedia materials. Data analysis is the part of qualitative research that most distinctively differentiates from quantitative research methods.