- Skip to main content
- Skip to primary sidebar
- Skip to footer
- QuestionPro

- Solutions Industries Gaming Automotive Sports and events Education Government Travel & Hospitality Financial Services Healthcare Cannabis Technology Use Case AskWhy Communities Audience Contactless surveys Mobile LivePolls Member Experience GDPR Positive People Science 360 Feedback Surveys
- Resources Blog eBooks Survey Templates Case Studies Training Help center

Home Market Research Research Tools and Apps

Quasi-experimental Research: What It Is, Types & Examples
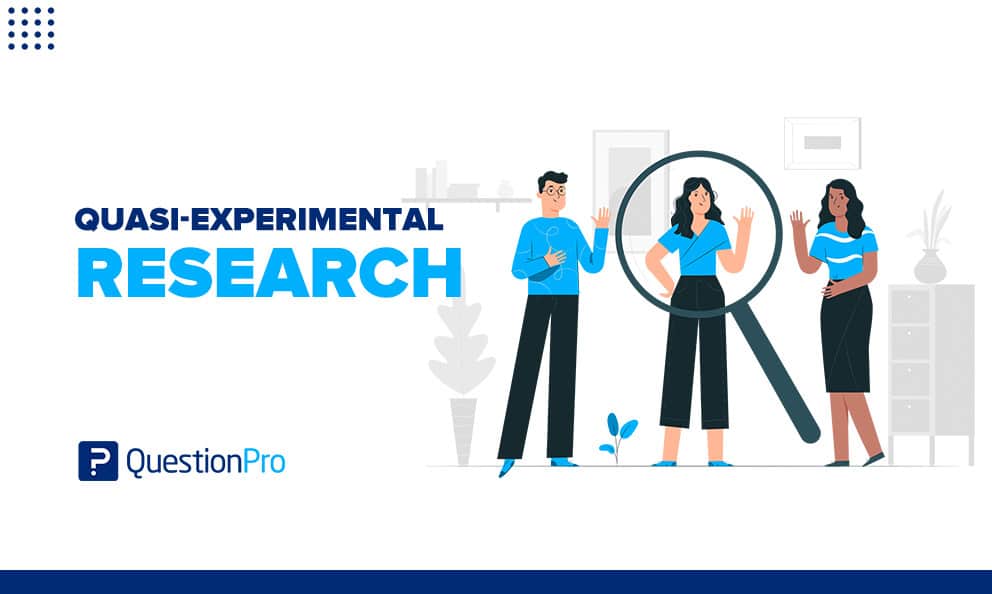
Much like an actual experiment, quasi-experimental research tries to demonstrate a cause-and-effect link between a dependent and an independent variable. A quasi-experiment, on the other hand, does not depend on random assignment, unlike an actual experiment. The subjects are sorted into groups based on non-random variables.
What is Quasi-Experimental Research?
“Resemblance” is the definition of “quasi.” Individuals are not randomly allocated to conditions or orders of conditions, even though the regression analysis is changed. As a result, quasi-experimental research is research that appears to be experimental but is not.
The directionality problem is avoided in quasi-experimental research since the regression analysis is altered before the multiple regression is assessed. However, because individuals are not randomized at random, there are likely to be additional disparities across conditions in quasi-experimental research.
As a result, in terms of internal consistency, quasi-experiments fall somewhere between correlational research and actual experiments.
The key component of a true experiment is randomly allocated groups. This means that each person has an equivalent chance of being assigned to the experimental group or the control group, depending on whether they are manipulated or not.
Simply put, a quasi-experiment is not a real experiment. A quasi-experiment does not feature randomly allocated groups since the main component of a real experiment is randomly assigned groups. Why is it so crucial to have randomly allocated groups, given that they constitute the only distinction between quasi-experimental and actual experimental research ?
Let’s use an example to illustrate our point. Let’s assume we want to discover how new psychological therapy affects depressed patients. In a genuine trial, you’d split half of the psych ward into treatment groups, With half getting the new psychotherapy therapy and the other half receiving standard depression treatment .
And the physicians compare the outcomes of this treatment to the results of standard treatments to see if this treatment is more effective. Doctors, on the other hand, are unlikely to agree with this genuine experiment since they believe it is unethical to treat one group while leaving another untreated.
A quasi-experimental study will be useful in this case. Instead of allocating these patients at random, you uncover pre-existing psychotherapist groups in the hospitals. Clearly, there’ll be counselors who are eager to undertake these trials as well as others who prefer to stick to the old ways.
These pre-existing groups can be used to compare the symptom development of individuals who received the novel therapy with those who received the normal course of treatment, even though the groups weren’t chosen at random.
If any substantial variations between them can be well explained, you may be very assured that any differences are attributable to the treatment but not to other extraneous variables.
As we mentioned before, quasi-experimental research entails manipulating an independent variable by randomly assigning people to conditions or sequences of conditions. Non-equivalent group designs, pretest-posttest designs, and regression discontinuity designs are only a few of the essential types.
What are quasi-experimental research designs?
Quasi-experimental research designs are a type of research design that is similar to experimental designs but doesn’t give full control over the independent variable(s) like true experimental designs do.
In a quasi-experimental design, the researcher changes or watches an independent variable, but the participants are not put into groups at random. Instead, people are put into groups based on things they already have in common, like their age, gender, or how many times they have seen a certain stimulus.
Because the assignments are not random, it is harder to draw conclusions about cause and effect than in a real experiment. However, quasi-experimental designs are still useful when randomization is not possible or ethical.
The true experimental design may be impossible to accomplish or just too expensive, especially for researchers with few resources. Quasi-experimental designs enable you to investigate an issue by utilizing data that has already been paid for or gathered by others (often the government).
Because they allow better control for confounding variables than other forms of studies, they have higher external validity than most genuine experiments and higher internal validity (less than true experiments) than other non-experimental research.
Is quasi-experimental research quantitative or qualitative?
Quasi-experimental research is a quantitative research method. It involves numerical data collection and statistical analysis. Quasi-experimental research compares groups with different circumstances or treatments to find cause-and-effect links.
It draws statistical conclusions from quantitative data. Qualitative data can enhance quasi-experimental research by revealing participants’ experiences and opinions, but quantitative data is the method’s foundation.
Quasi-experimental research types
There are many different sorts of quasi-experimental designs. Three of the most popular varieties are described below: Design of non-equivalent groups, Discontinuity in regression, and Natural experiments.
Design of Non-equivalent Groups
Example: design of non-equivalent groups, discontinuity in regression, example: discontinuity in regression, natural experiments, example: natural experiments.
However, because they couldn’t afford to pay everyone who qualified for the program, they had to use a random lottery to distribute slots.
Experts were able to investigate the program’s impact by utilizing enrolled people as a treatment group and those who were qualified but did not play the jackpot as an experimental group.
How QuestionPro helps in quasi-experimental research?
QuestionPro can be a useful tool in quasi-experimental research because it includes features that can assist you in designing and analyzing your research study. Here are some ways in which QuestionPro can help in quasi-experimental research:
Design surveys
Randomize participants, collect data over time, analyze data, collaborate with your team.
With QuestionPro, you have access to the most mature market research platform and tool that helps you collect and analyze the insights that matter the most. By leveraging InsightsHub, the unified hub for data management, you can leverage the consolidated platform to organize, explore, search, and discover your research data in one organized data repository .
Optimize Your quasi-experimental research with QuestionPro. Get started now!
LEARN MORE FREE TRIAL
MORE LIKE THIS
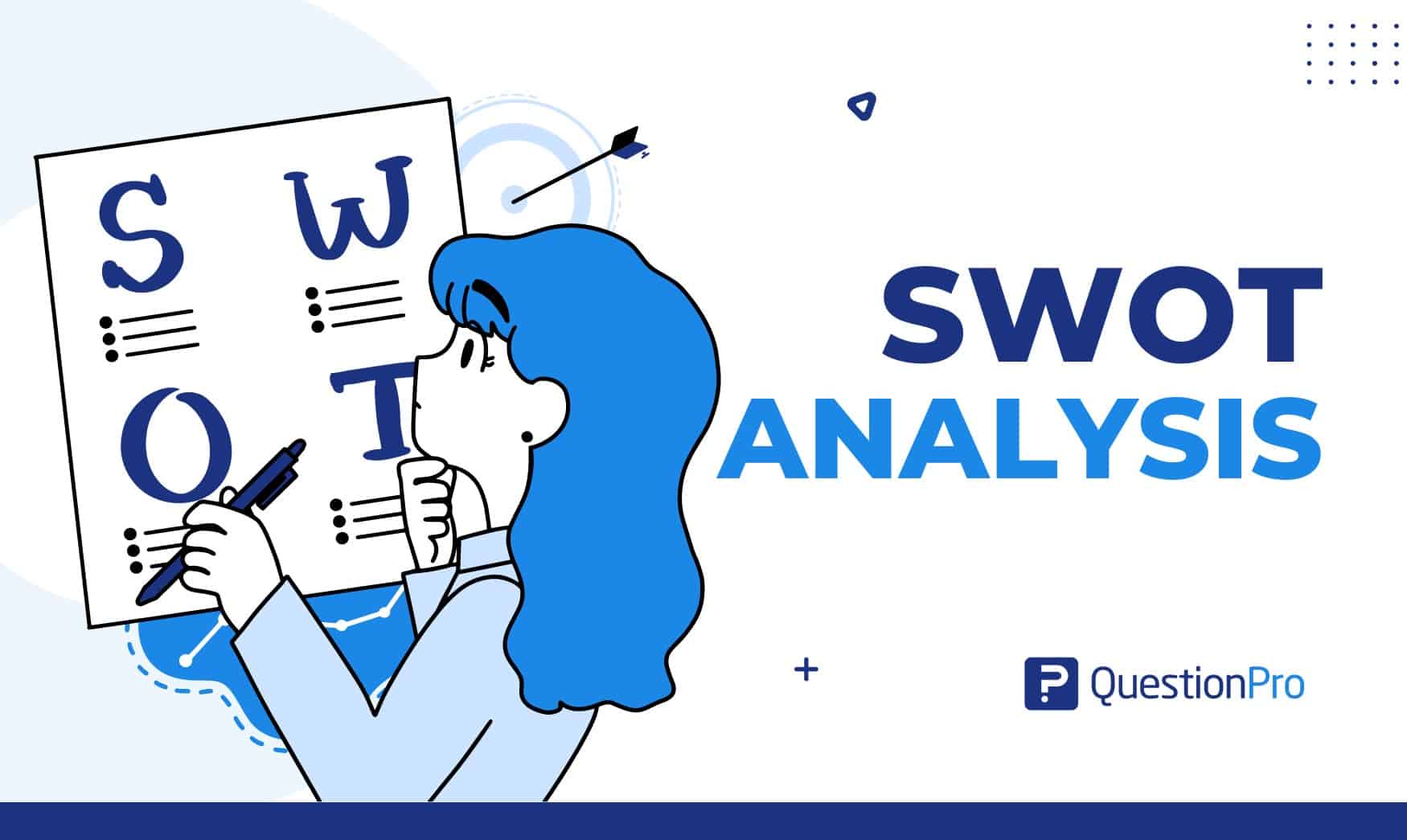
SWOT Analysis: What It Is And How To Do It?
Sep 27, 2024
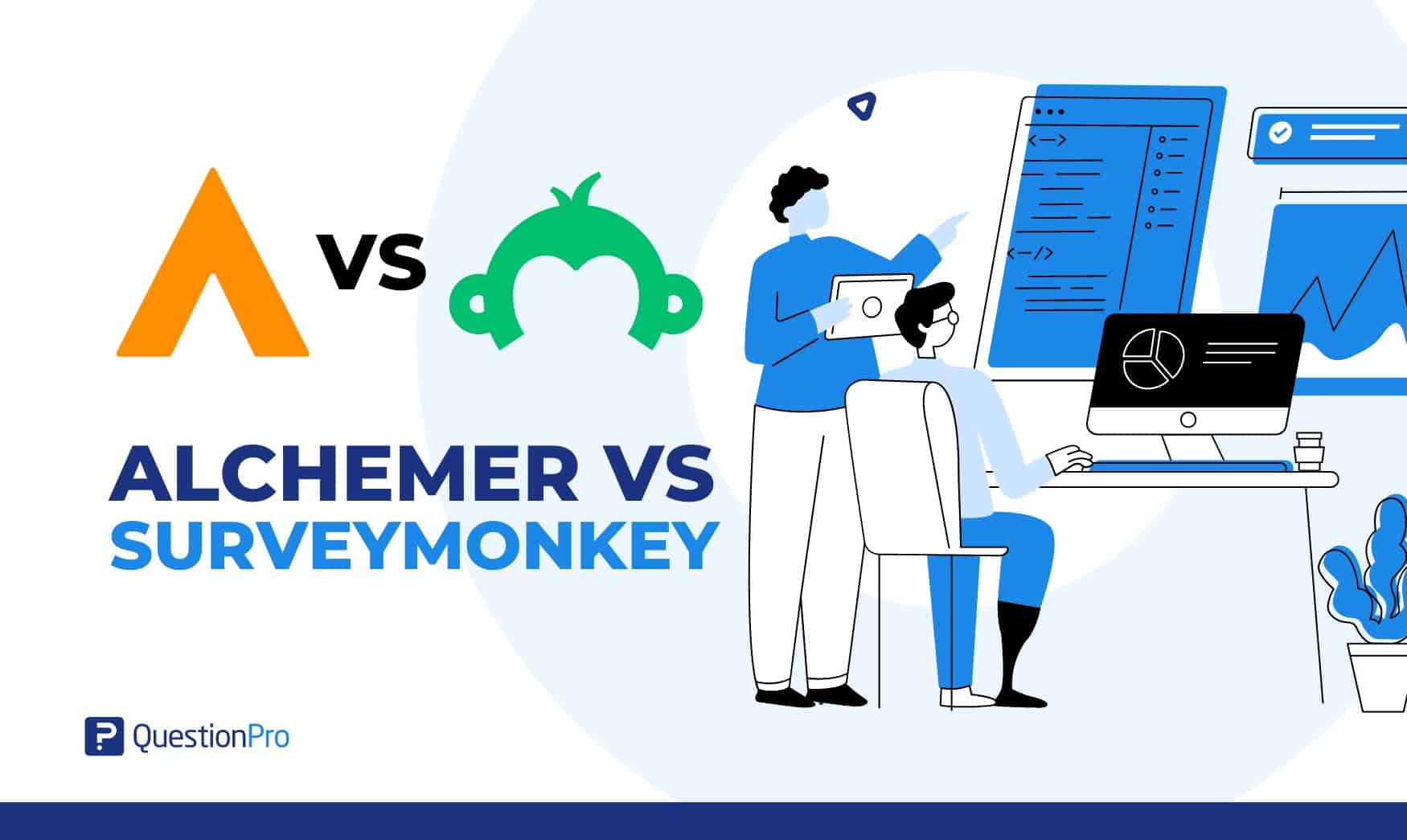
Alchemer vs SurveyMonkey: Which Survey Tool Is Best for You
Sep 26, 2024
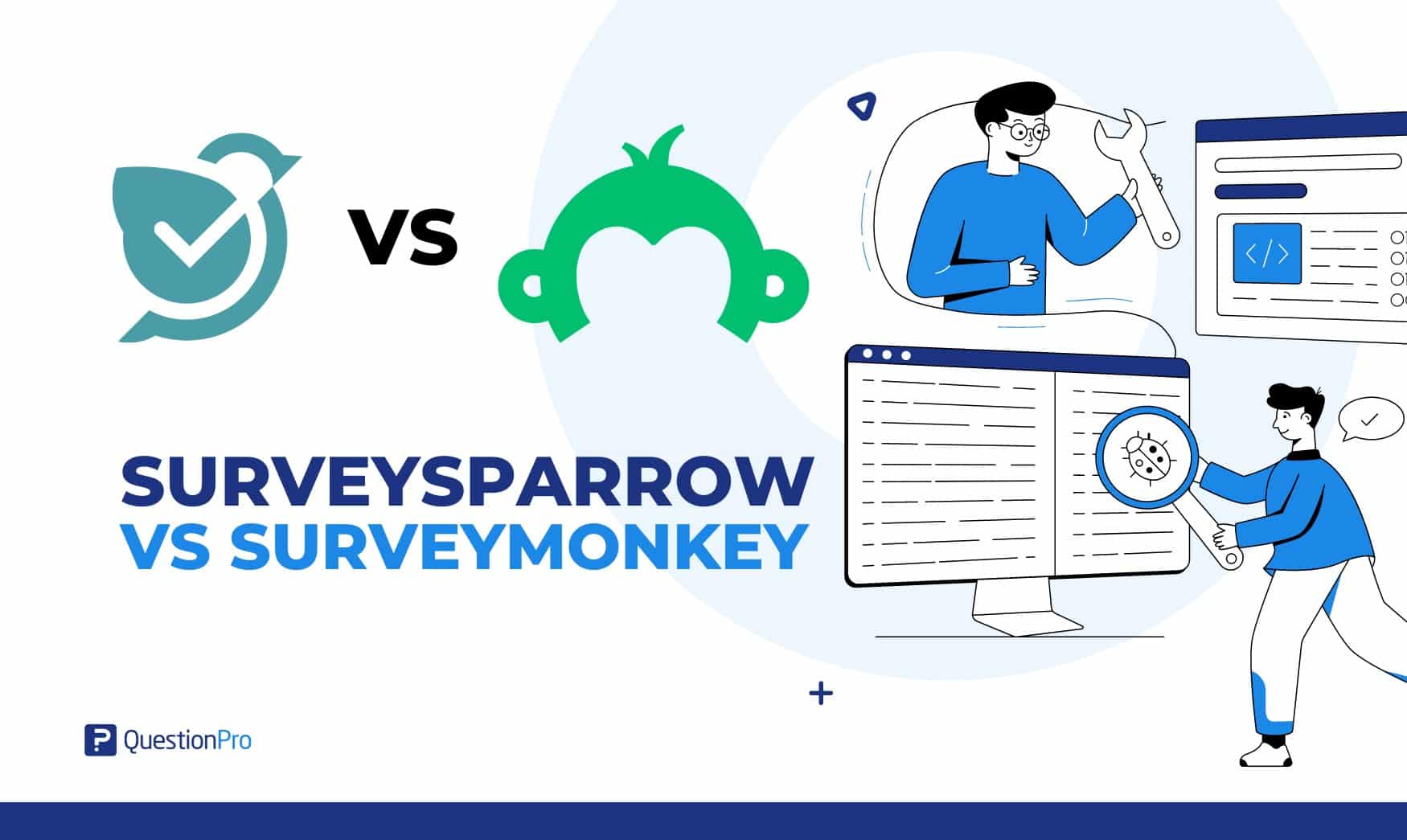
SurveySparrow vs SurveyMonkey: Choosing the Right Survey Tool
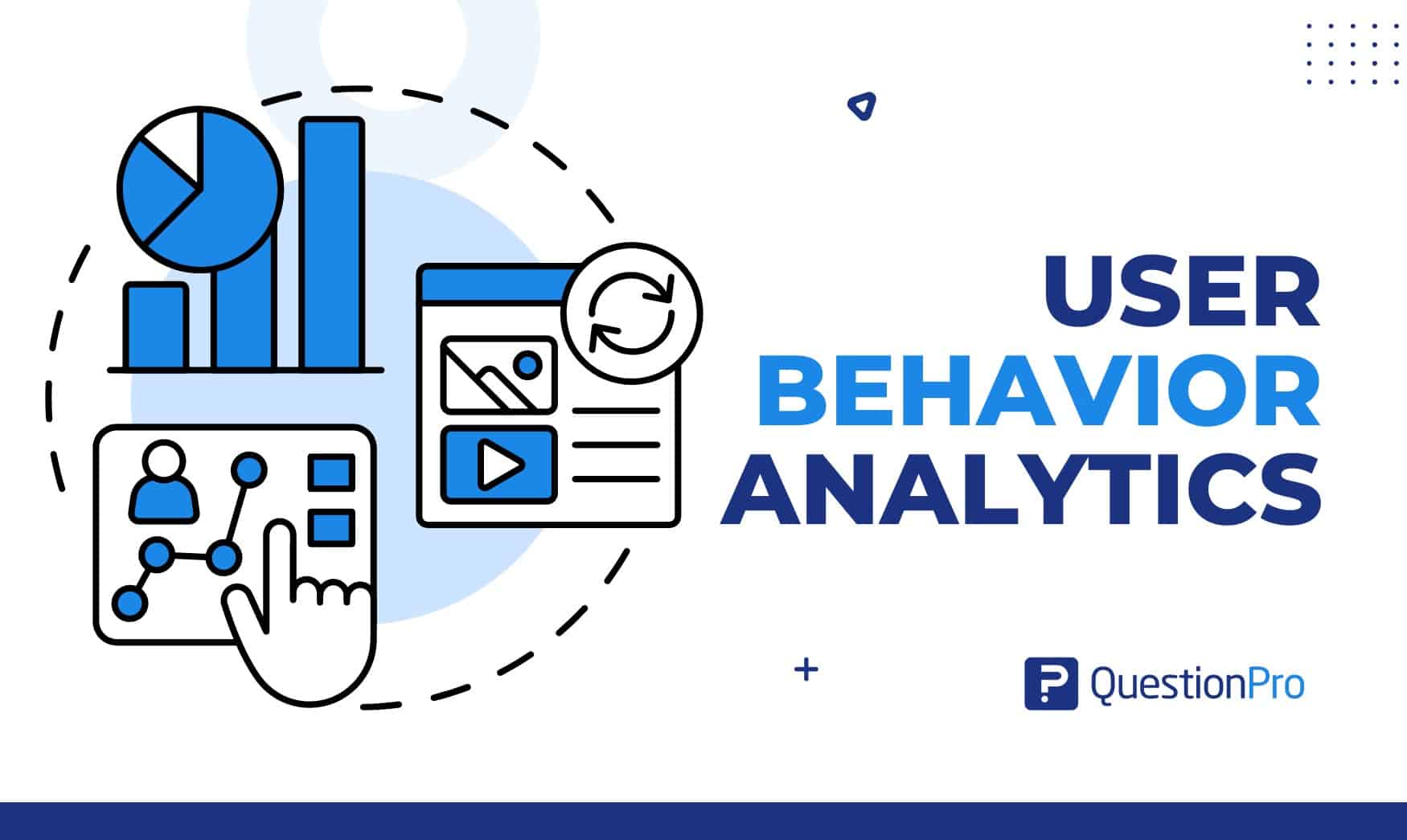
User Behavior Analytics: What it is, Importance, Uses & Tools
Other categories.
- Academic Research
- Artificial Intelligence
- Assessments
- Brand Awareness
- Case Studies
- Communities
- Consumer Insights
- Customer effort score
- Customer Engagement
- Customer Experience
- Customer Loyalty
- Customer Research
- Customer Satisfaction
- Employee Benefits
- Employee Engagement
- Employee Retention
- Friday Five
- General Data Protection Regulation
- Insights Hub
- Life@QuestionPro
- Market Research
- Mobile diaries
- Mobile Surveys
- New Features
- Online Communities
- Question Types
- Questionnaire
- QuestionPro Products
- Release Notes
- Research Tools and Apps
- Revenue at Risk
- Survey Templates
- Training Tips
- Tuesday CX Thoughts (TCXT)
- Uncategorized
- What’s Coming Up
- Workforce Intelligence
- Privacy Policy

Home » Quasi-Experimental Research Design – Types, Methods
Quasi-Experimental Research Design – Types, Methods
Table of Contents

Quasi-Experimental Design
Quasi-experimental design is a research method that seeks to evaluate the causal relationships between variables, but without the full control over the independent variable(s) that is available in a true experimental design.
In a quasi-experimental design, the researcher uses an existing group of participants that is not randomly assigned to the experimental and control groups. Instead, the groups are selected based on pre-existing characteristics or conditions, such as age, gender, or the presence of a certain medical condition.
Types of Quasi-Experimental Design
There are several types of quasi-experimental designs that researchers use to study causal relationships between variables. Here are some of the most common types:
Non-Equivalent Control Group Design
This design involves selecting two groups of participants that are similar in every way except for the independent variable(s) that the researcher is testing. One group receives the treatment or intervention being studied, while the other group does not. The two groups are then compared to see if there are any significant differences in the outcomes.
Interrupted Time-Series Design
This design involves collecting data on the dependent variable(s) over a period of time, both before and after an intervention or event. The researcher can then determine whether there was a significant change in the dependent variable(s) following the intervention or event.
Pretest-Posttest Design
This design involves measuring the dependent variable(s) before and after an intervention or event, but without a control group. This design can be useful for determining whether the intervention or event had an effect, but it does not allow for control over other factors that may have influenced the outcomes.
Regression Discontinuity Design
This design involves selecting participants based on a specific cutoff point on a continuous variable, such as a test score. Participants on either side of the cutoff point are then compared to determine whether the intervention or event had an effect.
Natural Experiments
This design involves studying the effects of an intervention or event that occurs naturally, without the researcher’s intervention. For example, a researcher might study the effects of a new law or policy that affects certain groups of people. This design is useful when true experiments are not feasible or ethical.
Data Analysis Methods
Here are some data analysis methods that are commonly used in quasi-experimental designs:
Descriptive Statistics
This method involves summarizing the data collected during a study using measures such as mean, median, mode, range, and standard deviation. Descriptive statistics can help researchers identify trends or patterns in the data, and can also be useful for identifying outliers or anomalies.
Inferential Statistics
This method involves using statistical tests to determine whether the results of a study are statistically significant. Inferential statistics can help researchers make generalizations about a population based on the sample data collected during the study. Common statistical tests used in quasi-experimental designs include t-tests, ANOVA, and regression analysis.
Propensity Score Matching
This method is used to reduce bias in quasi-experimental designs by matching participants in the intervention group with participants in the control group who have similar characteristics. This can help to reduce the impact of confounding variables that may affect the study’s results.
Difference-in-differences Analysis
This method is used to compare the difference in outcomes between two groups over time. Researchers can use this method to determine whether a particular intervention has had an impact on the target population over time.
Interrupted Time Series Analysis
This method is used to examine the impact of an intervention or treatment over time by comparing data collected before and after the intervention or treatment. This method can help researchers determine whether an intervention had a significant impact on the target population.
Regression Discontinuity Analysis
This method is used to compare the outcomes of participants who fall on either side of a predetermined cutoff point. This method can help researchers determine whether an intervention had a significant impact on the target population.
Steps in Quasi-Experimental Design
Here are the general steps involved in conducting a quasi-experimental design:
- Identify the research question: Determine the research question and the variables that will be investigated.
- Choose the design: Choose the appropriate quasi-experimental design to address the research question. Examples include the pretest-posttest design, non-equivalent control group design, regression discontinuity design, and interrupted time series design.
- Select the participants: Select the participants who will be included in the study. Participants should be selected based on specific criteria relevant to the research question.
- Measure the variables: Measure the variables that are relevant to the research question. This may involve using surveys, questionnaires, tests, or other measures.
- Implement the intervention or treatment: Implement the intervention or treatment to the participants in the intervention group. This may involve training, education, counseling, or other interventions.
- Collect data: Collect data on the dependent variable(s) before and after the intervention. Data collection may also include collecting data on other variables that may impact the dependent variable(s).
- Analyze the data: Analyze the data collected to determine whether the intervention had a significant impact on the dependent variable(s).
- Draw conclusions: Draw conclusions about the relationship between the independent and dependent variables. If the results suggest a causal relationship, then appropriate recommendations may be made based on the findings.
Quasi-Experimental Design Examples
Here are some examples of real-time quasi-experimental designs:
- Evaluating the impact of a new teaching method: In this study, a group of students are taught using a new teaching method, while another group is taught using the traditional method. The test scores of both groups are compared before and after the intervention to determine whether the new teaching method had a significant impact on student performance.
- Assessing the effectiveness of a public health campaign: In this study, a public health campaign is launched to promote healthy eating habits among a targeted population. The behavior of the population is compared before and after the campaign to determine whether the intervention had a significant impact on the target behavior.
- Examining the impact of a new medication: In this study, a group of patients is given a new medication, while another group is given a placebo. The outcomes of both groups are compared to determine whether the new medication had a significant impact on the targeted health condition.
- Evaluating the effectiveness of a job training program : In this study, a group of unemployed individuals is enrolled in a job training program, while another group is not enrolled in any program. The employment rates of both groups are compared before and after the intervention to determine whether the training program had a significant impact on the employment rates of the participants.
- Assessing the impact of a new policy : In this study, a new policy is implemented in a particular area, while another area does not have the new policy. The outcomes of both areas are compared before and after the intervention to determine whether the new policy had a significant impact on the targeted behavior or outcome.
Applications of Quasi-Experimental Design
Here are some applications of quasi-experimental design:
- Educational research: Quasi-experimental designs are used to evaluate the effectiveness of educational interventions, such as new teaching methods, technology-based learning, or educational policies.
- Health research: Quasi-experimental designs are used to evaluate the effectiveness of health interventions, such as new medications, public health campaigns, or health policies.
- Social science research: Quasi-experimental designs are used to investigate the impact of social interventions, such as job training programs, welfare policies, or criminal justice programs.
- Business research: Quasi-experimental designs are used to evaluate the impact of business interventions, such as marketing campaigns, new products, or pricing strategies.
- Environmental research: Quasi-experimental designs are used to evaluate the impact of environmental interventions, such as conservation programs, pollution control policies, or renewable energy initiatives.
When to use Quasi-Experimental Design
Here are some situations where quasi-experimental designs may be appropriate:
- When the research question involves investigating the effectiveness of an intervention, policy, or program : In situations where it is not feasible or ethical to randomly assign participants to intervention and control groups, quasi-experimental designs can be used to evaluate the impact of the intervention on the targeted outcome.
- When the sample size is small: In situations where the sample size is small, it may be difficult to randomly assign participants to intervention and control groups. Quasi-experimental designs can be used to investigate the impact of an intervention without requiring a large sample size.
- When the research question involves investigating a naturally occurring event : In some situations, researchers may be interested in investigating the impact of a naturally occurring event, such as a natural disaster or a major policy change. Quasi-experimental designs can be used to evaluate the impact of the event on the targeted outcome.
- When the research question involves investigating a long-term intervention: In situations where the intervention or program is long-term, it may be difficult to randomly assign participants to intervention and control groups for the entire duration of the intervention. Quasi-experimental designs can be used to evaluate the impact of the intervention over time.
- When the research question involves investigating the impact of a variable that cannot be manipulated : In some situations, it may not be possible or ethical to manipulate a variable of interest. Quasi-experimental designs can be used to investigate the relationship between the variable and the targeted outcome.
Purpose of Quasi-Experimental Design
The purpose of quasi-experimental design is to investigate the causal relationship between two or more variables when it is not feasible or ethical to conduct a randomized controlled trial (RCT). Quasi-experimental designs attempt to emulate the randomized control trial by mimicking the control group and the intervention group as much as possible.
The key purpose of quasi-experimental design is to evaluate the impact of an intervention, policy, or program on a targeted outcome while controlling for potential confounding factors that may affect the outcome. Quasi-experimental designs aim to answer questions such as: Did the intervention cause the change in the outcome? Would the outcome have changed without the intervention? And was the intervention effective in achieving its intended goals?
Quasi-experimental designs are useful in situations where randomized controlled trials are not feasible or ethical. They provide researchers with an alternative method to evaluate the effectiveness of interventions, policies, and programs in real-life settings. Quasi-experimental designs can also help inform policy and practice by providing valuable insights into the causal relationships between variables.
Overall, the purpose of quasi-experimental design is to provide a rigorous method for evaluating the impact of interventions, policies, and programs while controlling for potential confounding factors that may affect the outcome.
Advantages of Quasi-Experimental Design
Quasi-experimental designs have several advantages over other research designs, such as:
- Greater external validity : Quasi-experimental designs are more likely to have greater external validity than laboratory experiments because they are conducted in naturalistic settings. This means that the results are more likely to generalize to real-world situations.
- Ethical considerations: Quasi-experimental designs often involve naturally occurring events, such as natural disasters or policy changes. This means that researchers do not need to manipulate variables, which can raise ethical concerns.
- More practical: Quasi-experimental designs are often more practical than experimental designs because they are less expensive and easier to conduct. They can also be used to evaluate programs or policies that have already been implemented, which can save time and resources.
- No random assignment: Quasi-experimental designs do not require random assignment, which can be difficult or impossible in some cases, such as when studying the effects of a natural disaster. This means that researchers can still make causal inferences, although they must use statistical techniques to control for potential confounding variables.
- Greater generalizability : Quasi-experimental designs are often more generalizable than experimental designs because they include a wider range of participants and conditions. This can make the results more applicable to different populations and settings.
Limitations of Quasi-Experimental Design
There are several limitations associated with quasi-experimental designs, which include:
- Lack of Randomization: Quasi-experimental designs do not involve randomization of participants into groups, which means that the groups being studied may differ in important ways that could affect the outcome of the study. This can lead to problems with internal validity and limit the ability to make causal inferences.
- Selection Bias: Quasi-experimental designs may suffer from selection bias because participants are not randomly assigned to groups. Participants may self-select into groups or be assigned based on pre-existing characteristics, which may introduce bias into the study.
- History and Maturation: Quasi-experimental designs are susceptible to history and maturation effects, where the passage of time or other events may influence the outcome of the study.
- Lack of Control: Quasi-experimental designs may lack control over extraneous variables that could influence the outcome of the study. This can limit the ability to draw causal inferences from the study.
- Limited Generalizability: Quasi-experimental designs may have limited generalizability because the results may only apply to the specific population and context being studied.
About the author
Muhammad Hassan
Researcher, Academic Writer, Web developer
You may also like

Triangulation in Research – Types, Methods and...

Case Study – Methods, Examples and Guide

Correlational Research – Methods, Types and...

Experimental Design – Types, Methods, Guide

Descriptive Research Design – Types, Methods and...

Transformative Design – Methods, Types, Guide
- Skip to secondary menu
- Skip to main content
- Skip to primary sidebar
Statistics By Jim
Making statistics intuitive
Quasi Experimental Design Overview & Examples
By Jim Frost Leave a Comment
What is a Quasi Experimental Design?
A quasi experimental design is a method for identifying causal relationships that does not randomly assign participants to the experimental groups. Instead, researchers use a non-random process. For example, they might use an eligibility cutoff score or preexisting groups to determine who receives the treatment.

Quasi-experimental research is a design that closely resembles experimental research but is different. The term “quasi” means “resembling,” so you can think of it as a cousin to actual experiments. In these studies, researchers can manipulate an independent variable — that is, they change one factor to see what effect it has. However, unlike true experimental research, participants are not randomly assigned to different groups.
Learn more about Experimental Designs: Definition & Types .
When to Use Quasi-Experimental Design
Researchers typically use a quasi-experimental design because they can’t randomize due to practical or ethical concerns. For example:
- Practical Constraints : A school interested in testing a new teaching method can only implement it in preexisting classes and cannot randomly assign students.
- Ethical Concerns : A medical study might not be able to randomly assign participants to a treatment group for an experimental medication when they are already taking a proven drug.
Quasi-experimental designs also come in handy when researchers want to study the effects of naturally occurring events, like policy changes or environmental shifts, where they can’t control who is exposed to the treatment.
Quasi-experimental designs occupy a unique position in the spectrum of research methodologies, sitting between observational studies and true experiments. This middle ground offers a blend of both worlds, addressing some limitations of purely observational studies while navigating the constraints often accompanying true experiments.
A significant advantage of quasi-experimental research over purely observational studies and correlational research is that it addresses the issue of directionality, determining which variable is the cause and which is the effect. In quasi-experiments, an intervention typically occurs during the investigation, and the researchers record outcomes before and after it, increasing the confidence that it causes the observed changes.
However, it’s crucial to recognize its limitations as well. Controlling confounding variables is a larger concern for a quasi-experimental design than a true experiment because it lacks random assignment.
In sum, quasi-experimental designs offer a valuable research approach when random assignment is not feasible, providing a more structured and controlled framework than observational studies while acknowledging and attempting to address potential confounders.
Types of Quasi-Experimental Designs and Examples
Quasi-experimental studies use various methods, depending on the scenario.
Natural Experiments
This design uses naturally occurring events or changes to create the treatment and control groups. Researchers compare outcomes between those whom the event affected and those it did not affect. Analysts use statistical controls to account for confounders that the researchers must also measure.
Natural experiments are related to observational studies, but they allow for a clearer causality inference because the external event or policy change provides both a form of quasi-random group assignment and a definite start date for the intervention.
For example, in a natural experiment utilizing a quasi-experimental design, researchers study the impact of a significant economic policy change on small business growth. The policy is implemented in one state but not in neighboring states. This scenario creates an unplanned experimental setup, where the state with the new policy serves as the treatment group, and the neighboring states act as the control group.
Researchers are primarily interested in small business growth rates but need to record various confounders that can impact growth rates. Hence, they record state economic indicators, investment levels, and employment figures. By recording these metrics across the states, they can include them in the model as covariates and control them statistically. This method allows researchers to estimate differences in small business growth due to the policy itself, separate from the various confounders.
Nonequivalent Groups Design
This method involves matching existing groups that are similar but not identical. Researchers attempt to find groups that are as equivalent as possible, particularly for factors likely to affect the outcome.
For instance, researchers use a nonequivalent groups quasi-experimental design to evaluate the effectiveness of a new teaching method in improving students’ mathematics performance. A school district considering the teaching method is planning the study. Students are already divided into schools, preventing random assignment.
The researchers matched two schools with similar demographics, baseline academic performance, and resources. The school using the traditional methodology is the control, while the other uses the new approach. Researchers are evaluating differences in educational outcomes between the two methods.
They perform a pretest to identify differences between the schools that might affect the outcome and include them as covariates to control for confounding. They also record outcomes before and after the intervention to have a larger context for the changes they observe.
Regression Discontinuity
This process assigns subjects to a treatment or control group based on a predetermined cutoff point (e.g., a test score). The analysis primarily focuses on participants near the cutoff point, as they are likely similar except for the treatment received. By comparing participants just above and below the cutoff, the design controls for confounders that vary smoothly around the cutoff.
For example, in a regression discontinuity quasi-experimental design focusing on a new medical treatment for depression, researchers use depression scores as the cutoff point. Individuals with depression scores just above a certain threshold are assigned to receive the latest treatment, while those just below the threshold do not receive it. This method creates two closely matched groups: one that barely qualifies for treatment and one that barely misses out.
By comparing the mental health outcomes of these two groups over time, researchers can assess the effectiveness of the new treatment. The assumption is that the only significant difference between the groups is whether they received the treatment, thereby isolating its impact on depression outcomes.
Controlling Confounders in a Quasi-Experimental Design
Accounting for confounding variables is a challenging but essential task for a quasi-experimental design.
In a true experiment, the random assignment process equalizes confounders across the groups to nullify their overall effect. It’s the gold standard because it works on all confounders, known and unknown.
Unfortunately, the lack of random assignment can allow differences between the groups to exist before the intervention. These confounding factors might ultimately explain the results rather than the intervention.
Consequently, researchers must use other methods to equalize the groups roughly using matching and cutoff values or statistically adjust for preexisting differences they measure to reduce the impact of confounders.
A key strength of quasi-experiments is their frequent use of “pre-post testing.” This approach involves conducting initial tests before collecting data to check for preexisting differences between groups that could impact the study’s outcome. By identifying these variables early on and including them as covariates, researchers can more effectively control potential confounders in their statistical analysis.
Additionally, researchers frequently track outcomes before and after the intervention to better understand the context for changes they observe.
Statisticians consider these methods to be less effective than randomization. Hence, quasi-experiments fall somewhere in the middle when it comes to internal validity , or how well the study can identify causal relationships versus mere correlation . They’re more conclusive than correlational studies but not as solid as true experiments.
In conclusion, quasi-experimental designs offer researchers a versatile and practical approach when random assignment is not feasible. This methodology bridges the gap between controlled experiments and observational studies, providing a valuable tool for investigating cause-and-effect relationships in real-world settings. Researchers can address ethical and logistical constraints by understanding and leveraging the different types of quasi-experimental designs while still obtaining insightful and meaningful results.
Cook, T. D., & Campbell, D. T. (1979). Quasi-experimentation: Design & analysis issues in field settings . Boston, MA: Houghton Mifflin
Share this:

Reader Interactions
Comments and questions cancel reply.
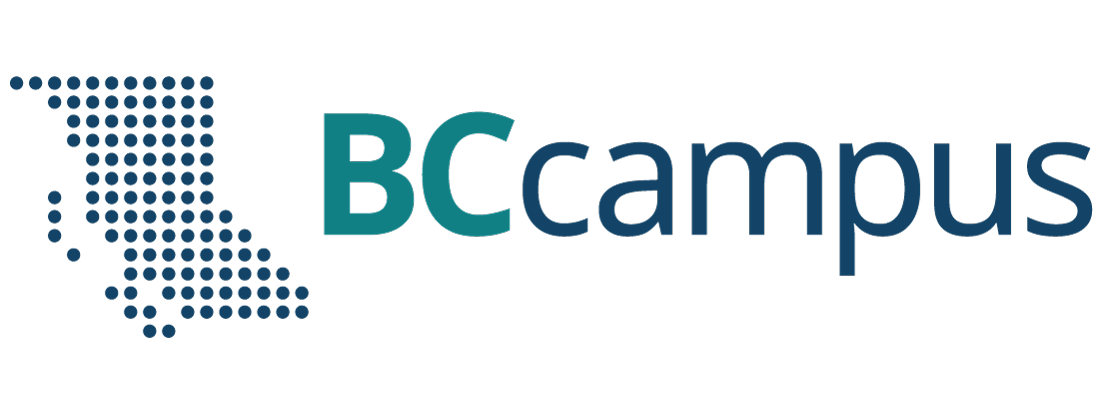
Want to create or adapt books like this? Learn more about how Pressbooks supports open publishing practices.
Chapter 7: Nonexperimental Research
Quasi-Experimental Research
Learning Objectives
- Explain what quasi-experimental research is and distinguish it clearly from both experimental and correlational research.
- Describe three different types of quasi-experimental research designs (nonequivalent groups, pretest-posttest, and interrupted time series) and identify examples of each one.
The prefix quasi means “resembling.” Thus quasi-experimental research is research that resembles experimental research but is not true experimental research. Although the independent variable is manipulated, participants are not randomly assigned to conditions or orders of conditions (Cook & Campbell, 1979). [1] Because the independent variable is manipulated before the dependent variable is measured, quasi-experimental research eliminates the directionality problem. But because participants are not randomly assigned—making it likely that there are other differences between conditions—quasi-experimental research does not eliminate the problem of confounding variables. In terms of internal validity, therefore, quasi-experiments are generally somewhere between correlational studies and true experiments.
Quasi-experiments are most likely to be conducted in field settings in which random assignment is difficult or impossible. They are often conducted to evaluate the effectiveness of a treatment—perhaps a type of psychotherapy or an educational intervention. There are many different kinds of quasi-experiments, but we will discuss just a few of the most common ones here.
Nonequivalent Groups Design
Recall that when participants in a between-subjects experiment are randomly assigned to conditions, the resulting groups are likely to be quite similar. In fact, researchers consider them to be equivalent. When participants are not randomly assigned to conditions, however, the resulting groups are likely to be dissimilar in some ways. For this reason, researchers consider them to be nonequivalent. A nonequivalent groups design , then, is a between-subjects design in which participants have not been randomly assigned to conditions.
Imagine, for example, a researcher who wants to evaluate a new method of teaching fractions to third graders. One way would be to conduct a study with a treatment group consisting of one class of third-grade students and a control group consisting of another class of third-grade students. This design would be a nonequivalent groups design because the students are not randomly assigned to classes by the researcher, which means there could be important differences between them. For example, the parents of higher achieving or more motivated students might have been more likely to request that their children be assigned to Ms. Williams’s class. Or the principal might have assigned the “troublemakers” to Mr. Jones’s class because he is a stronger disciplinarian. Of course, the teachers’ styles, and even the classroom environments, might be very different and might cause different levels of achievement or motivation among the students. If at the end of the study there was a difference in the two classes’ knowledge of fractions, it might have been caused by the difference between the teaching methods—but it might have been caused by any of these confounding variables.
Of course, researchers using a nonequivalent groups design can take steps to ensure that their groups are as similar as possible. In the present example, the researcher could try to select two classes at the same school, where the students in the two classes have similar scores on a standardized math test and the teachers are the same sex, are close in age, and have similar teaching styles. Taking such steps would increase the internal validity of the study because it would eliminate some of the most important confounding variables. But without true random assignment of the students to conditions, there remains the possibility of other important confounding variables that the researcher was not able to control.
Pretest-Posttest Design
In a pretest-posttest design , the dependent variable is measured once before the treatment is implemented and once after it is implemented. Imagine, for example, a researcher who is interested in the effectiveness of an antidrug education program on elementary school students’ attitudes toward illegal drugs. The researcher could measure the attitudes of students at a particular elementary school during one week, implement the antidrug program during the next week, and finally, measure their attitudes again the following week. The pretest-posttest design is much like a within-subjects experiment in which each participant is tested first under the control condition and then under the treatment condition. It is unlike a within-subjects experiment, however, in that the order of conditions is not counterbalanced because it typically is not possible for a participant to be tested in the treatment condition first and then in an “untreated” control condition.
If the average posttest score is better than the average pretest score, then it makes sense to conclude that the treatment might be responsible for the improvement. Unfortunately, one often cannot conclude this with a high degree of certainty because there may be other explanations for why the posttest scores are better. One category of alternative explanations goes under the name of history . Other things might have happened between the pretest and the posttest. Perhaps an antidrug program aired on television and many of the students watched it, or perhaps a celebrity died of a drug overdose and many of the students heard about it. Another category of alternative explanations goes under the name of maturation . Participants might have changed between the pretest and the posttest in ways that they were going to anyway because they are growing and learning. If it were a yearlong program, participants might become less impulsive or better reasoners and this might be responsible for the change.
Another alternative explanation for a change in the dependent variable in a pretest-posttest design is regression to the mean . This refers to the statistical fact that an individual who scores extremely on a variable on one occasion will tend to score less extremely on the next occasion. For example, a bowler with a long-term average of 150 who suddenly bowls a 220 will almost certainly score lower in the next game. Her score will “regress” toward her mean score of 150. Regression to the mean can be a problem when participants are selected for further study because of their extreme scores. Imagine, for example, that only students who scored especially low on a test of fractions are given a special training program and then retested. Regression to the mean all but guarantees that their scores will be higher even if the training program has no effect. A closely related concept—and an extremely important one in psychological research—is spontaneous remission . This is the tendency for many medical and psychological problems to improve over time without any form of treatment. The common cold is a good example. If one were to measure symptom severity in 100 common cold sufferers today, give them a bowl of chicken soup every day, and then measure their symptom severity again in a week, they would probably be much improved. This does not mean that the chicken soup was responsible for the improvement, however, because they would have been much improved without any treatment at all. The same is true of many psychological problems. A group of severely depressed people today is likely to be less depressed on average in 6 months. In reviewing the results of several studies of treatments for depression, researchers Michael Posternak and Ivan Miller found that participants in waitlist control conditions improved an average of 10 to 15% before they received any treatment at all (Posternak & Miller, 2001) [2] . Thus one must generally be very cautious about inferring causality from pretest-posttest designs.
Does Psychotherapy Work?
Early studies on the effectiveness of psychotherapy tended to use pretest-posttest designs. In a classic 1952 article, researcher Hans Eysenck summarized the results of 24 such studies showing that about two thirds of patients improved between the pretest and the posttest (Eysenck, 1952) [3] . But Eysenck also compared these results with archival data from state hospital and insurance company records showing that similar patients recovered at about the same rate without receiving psychotherapy. This parallel suggested to Eysenck that the improvement that patients showed in the pretest-posttest studies might be no more than spontaneous remission. Note that Eysenck did not conclude that psychotherapy was ineffective. He merely concluded that there was no evidence that it was, and he wrote of “the necessity of properly planned and executed experimental studies into this important field” (p. 323). You can read the entire article here: Classics in the History of Psychology .
Fortunately, many other researchers took up Eysenck’s challenge, and by 1980 hundreds of experiments had been conducted in which participants were randomly assigned to treatment and control conditions, and the results were summarized in a classic book by Mary Lee Smith, Gene Glass, and Thomas Miller (Smith, Glass, & Miller, 1980) [4] . They found that overall psychotherapy was quite effective, with about 80% of treatment participants improving more than the average control participant. Subsequent research has focused more on the conditions under which different types of psychotherapy are more or less effective.
Interrupted Time Series Design
A variant of the pretest-posttest design is the interrupted time-series design . A time series is a set of measurements taken at intervals over a period of time. For example, a manufacturing company might measure its workers’ productivity each week for a year. In an interrupted time series-design, a time series like this one is “interrupted” by a treatment. In one classic example, the treatment was the reduction of the work shifts in a factory from 10 hours to 8 hours (Cook & Campbell, 1979) [5] . Because productivity increased rather quickly after the shortening of the work shifts, and because it remained elevated for many months afterward, the researcher concluded that the shortening of the shifts caused the increase in productivity. Notice that the interrupted time-series design is like a pretest-posttest design in that it includes measurements of the dependent variable both before and after the treatment. It is unlike the pretest-posttest design, however, in that it includes multiple pretest and posttest measurements.
Figure 7.3 shows data from a hypothetical interrupted time-series study. The dependent variable is the number of student absences per week in a research methods course. The treatment is that the instructor begins publicly taking attendance each day so that students know that the instructor is aware of who is present and who is absent. The top panel of Figure 7.3 shows how the data might look if this treatment worked. There is a consistently high number of absences before the treatment, and there is an immediate and sustained drop in absences after the treatment. The bottom panel of Figure 7.3 shows how the data might look if this treatment did not work. On average, the number of absences after the treatment is about the same as the number before. This figure also illustrates an advantage of the interrupted time-series design over a simpler pretest-posttest design. If there had been only one measurement of absences before the treatment at Week 7 and one afterward at Week 8, then it would have looked as though the treatment were responsible for the reduction. The multiple measurements both before and after the treatment suggest that the reduction between Weeks 7 and 8 is nothing more than normal week-to-week variation.
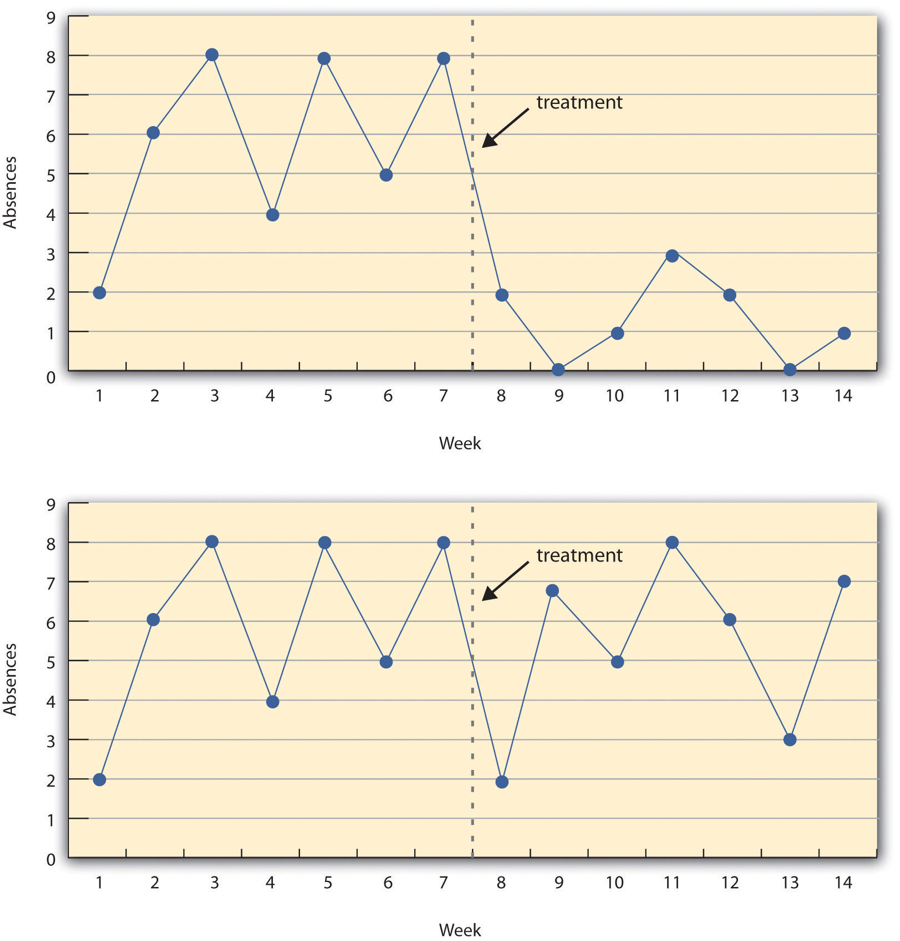
Combination Designs
A type of quasi-experimental design that is generally better than either the nonequivalent groups design or the pretest-posttest design is one that combines elements of both. There is a treatment group that is given a pretest, receives a treatment, and then is given a posttest. But at the same time there is a control group that is given a pretest, does not receive the treatment, and then is given a posttest. The question, then, is not simply whether participants who receive the treatment improve but whether they improve more than participants who do not receive the treatment.
Imagine, for example, that students in one school are given a pretest on their attitudes toward drugs, then are exposed to an antidrug program, and finally are given a posttest. Students in a similar school are given the pretest, not exposed to an antidrug program, and finally are given a posttest. Again, if students in the treatment condition become more negative toward drugs, this change in attitude could be an effect of the treatment, but it could also be a matter of history or maturation. If it really is an effect of the treatment, then students in the treatment condition should become more negative than students in the control condition. But if it is a matter of history (e.g., news of a celebrity drug overdose) or maturation (e.g., improved reasoning), then students in the two conditions would be likely to show similar amounts of change. This type of design does not completely eliminate the possibility of confounding variables, however. Something could occur at one of the schools but not the other (e.g., a student drug overdose), so students at the first school would be affected by it while students at the other school would not.
Finally, if participants in this kind of design are randomly assigned to conditions, it becomes a true experiment rather than a quasi experiment. In fact, it is the kind of experiment that Eysenck called for—and that has now been conducted many times—to demonstrate the effectiveness of psychotherapy.
Key Takeaways
- Quasi-experimental research involves the manipulation of an independent variable without the random assignment of participants to conditions or orders of conditions. Among the important types are nonequivalent groups designs, pretest-posttest, and interrupted time-series designs.
- Quasi-experimental research eliminates the directionality problem because it involves the manipulation of the independent variable. It does not eliminate the problem of confounding variables, however, because it does not involve random assignment to conditions. For these reasons, quasi-experimental research is generally higher in internal validity than correlational studies but lower than true experiments.
- Practice: Imagine that two professors decide to test the effect of giving daily quizzes on student performance in a statistics course. They decide that Professor A will give quizzes but Professor B will not. They will then compare the performance of students in their two sections on a common final exam. List five other variables that might differ between the two sections that could affect the results.
- regression to the mean
- spontaneous remission
Image Descriptions
Figure 7.3 image description: Two line graphs charting the number of absences per week over 14 weeks. The first 7 weeks are without treatment and the last 7 weeks are with treatment. In the first line graph, there are between 4 to 8 absences each week. After the treatment, the absences drop to 0 to 3 each week, which suggests the treatment worked. In the second line graph, there is no noticeable change in the number of absences per week after the treatment, which suggests the treatment did not work. [Return to Figure 7.3]
- Cook, T. D., & Campbell, D. T. (1979). Quasi-experimentation: Design & analysis issues in field settings . Boston, MA: Houghton Mifflin. ↵
- Posternak, M. A., & Miller, I. (2001). Untreated short-term course of major depression: A meta-analysis of studies using outcomes from studies using wait-list control groups. Journal of Affective Disorders, 66 , 139–146. ↵
- Eysenck, H. J. (1952). The effects of psychotherapy: An evaluation. Journal of Consulting Psychology, 16 , 319–324. ↵
- Smith, M. L., Glass, G. V., & Miller, T. I. (1980). The benefits of psychotherapy . Baltimore, MD: Johns Hopkins University Press. ↵
A between-subjects design in which participants have not been randomly assigned to conditions.
The dependent variable is measured once before the treatment is implemented and once after it is implemented.
A category of alternative explanations for differences between scores such as events that happened between the pretest and posttest, unrelated to the study.
An alternative explanation that refers to how the participants might have changed between the pretest and posttest in ways that they were going to anyway because they are growing and learning.
The statistical fact that an individual who scores extremely on a variable on one occasion will tend to score less extremely on the next occasion.
The tendency for many medical and psychological problems to improve over time without any form of treatment.
A set of measurements taken at intervals over a period of time that are interrupted by a treatment.
Research Methods in Psychology - 2nd Canadian Edition Copyright © 2015 by Paul C. Price, Rajiv Jhangiani, & I-Chant A. Chiang is licensed under a Creative Commons Attribution-NonCommercial-ShareAlike 4.0 International License , except where otherwise noted.
Share This Book
Instant insights, infinite possibilities
The use and interpretation of quasi-experimental design
Last updated
6 February 2023
Reviewed by
Miroslav Damyanov
Short on time? Get an AI generated summary of this article instead
- What is a quasi-experimental design?
Commonly used in medical informatics (a field that uses digital information to ensure better patient care), researchers generally use this design to evaluate the effectiveness of a treatment – perhaps a type of antibiotic or psychotherapy, or an educational or policy intervention.
Even though quasi-experimental design has been used for some time, relatively little is known about it. Read on to learn the ins and outs of this research design.
Make research less tedious
Dovetail streamlines research to help you uncover and share actionable insights
- When to use a quasi-experimental design
A quasi-experimental design is used when it's not logistically feasible or ethical to conduct randomized, controlled trials. As its name suggests, a quasi-experimental design is almost a true experiment. However, researchers don't randomly select elements or participants in this type of research.
Researchers prefer to apply quasi-experimental design when there are ethical or practical concerns. Let's look at these two reasons more closely.
Ethical reasons
In some situations, the use of randomly assigned elements can be unethical. For instance, providing public healthcare to one group and withholding it to another in research is unethical. A quasi-experimental design would examine the relationship between these two groups to avoid physical danger.
Practical reasons
Randomized controlled trials may not be the best approach in research. For instance, it's impractical to trawl through large sample sizes of participants without using a particular attribute to guide your data collection .
Recruiting participants and properly designing a data-collection attribute to make the research a true experiment requires a lot of time and effort, and can be expensive if you don’t have a large funding stream.
A quasi-experimental design allows researchers to take advantage of previously collected data and use it in their study.
- Examples of quasi-experimental designs
Quasi-experimental research design is common in medical research, but any researcher can use it for research that raises practical and ethical concerns. Here are a few examples of quasi-experimental designs used by different researchers:
Example 1: Determining the effectiveness of math apps in supplementing math classes
A school wanted to supplement its math classes with a math app. To select the best app, the school decided to conduct demo tests on two apps before selecting the one they will purchase.
Scope of the research
Since every grade had two math teachers, each teacher used one of the two apps for three months. They then gave the students the same math exams and compared the results to determine which app was most effective.
Reasons why this is a quasi-experimental study
This simple study is a quasi-experiment since the school didn't randomly assign its students to the applications. They used a pre-existing class structure to conduct the study since it was impractical to randomly assign the students to each app.
Example 2: Determining the effectiveness of teaching modern leadership techniques in start-up businesses
A hypothetical quasi-experimental study was conducted in an economically developing country in a mid-sized city.
Five start-ups in the textile industry and five in the tech industry participated in the study. The leaders attended a six-week workshop on leadership style, team management, and employee motivation.
After a year, the researchers assessed the performance of each start-up company to determine growth. The results indicated that the tech start-ups were further along in their growth than the textile companies.
The basis of quasi-experimental research is a non-randomized subject-selection process. This study didn't use specific aspects to determine which start-up companies should participate. Therefore, the results may seem straightforward, but several aspects may determine the growth of a specific company, apart from the variables used by the researchers.
Example 3: A study to determine the effects of policy reforms and of luring foreign investment on small businesses in two mid-size cities
In a study to determine the economic impact of government reforms in an economically developing country, the government decided to test whether creating reforms directed at small businesses or luring foreign investments would spur the most economic development.
The government selected two cities with similar population demographics and sizes. In one of the cities, they implemented specific policies that would directly impact small businesses, and in the other, they implemented policies to attract foreign investment.
After five years, they collected end-of-year economic growth data from both cities. They looked at elements like local GDP growth, unemployment rates, and housing sales.
The study used a non-randomized selection process to determine which city would participate in the research. Researchers left out certain variables that would play a crucial role in determining the growth of each city. They used pre-existing groups of people based on research conducted in each city, rather than random groups.
- Advantages of a quasi-experimental design
Some advantages of quasi-experimental designs are:
Researchers can manipulate variables to help them meet their study objectives.
It offers high external validity, making it suitable for real-world applications, specifically in social science experiments.
Integrating this methodology into other research designs is easier, especially in true experimental research. This cuts down on the time needed to determine your outcomes.
- Disadvantages of a quasi-experimental design
Despite the pros that come with a quasi-experimental design, there are several disadvantages associated with it, including the following:
It has a lower internal validity since researchers do not have full control over the comparison and intervention groups or between time periods because of differences in characteristics in people, places, or time involved. It may be challenging to determine whether all variables have been used or whether those used in the research impacted the results.
There is the risk of inaccurate data since the research design borrows information from other studies.
There is the possibility of bias since researchers select baseline elements and eligibility.
- What are the different quasi-experimental study designs?
There are three distinct types of quasi-experimental designs:

Nonequivalent
Regression discontinuity, natural experiment.
This is a hybrid of experimental and quasi-experimental methods and is used to leverage the best qualities of the two. Like the true experiment design, nonequivalent group design uses pre-existing groups believed to be comparable. However, it doesn't use randomization, the lack of which is a crucial element for quasi-experimental design.
Researchers usually ensure that no confounding variables impact them throughout the grouping process. This makes the groupings more comparable.
Example of a nonequivalent group design
A small study was conducted to determine whether after-school programs result in better grades. Researchers randomly selected two groups of students: one to implement the new program, the other not to. They then compared the results of the two groups.
This type of quasi-experimental research design calculates the impact of a specific treatment or intervention. It uses a criterion known as "cutoff" that assigns treatment according to eligibility.
Researchers often assign participants above the cutoff to the treatment group. This puts a negligible distinction between the two groups (treatment group and control group).
Example of regression discontinuity
Students must achieve a minimum score to be enrolled in specific US high schools. Since the cutoff score used to determine eligibility for enrollment is arbitrary, researchers can assume that the disparity between students who only just fail to achieve the cutoff point and those who barely pass is a small margin and is due to the difference in the schools that these students attend.
Researchers can then examine the long-term effects of these two groups of kids to determine the effect of attending certain schools. This information can be applied to increase the chances of students being enrolled in these high schools.
This research design is common in laboratory and field experiments where researchers control target subjects by assigning them to different groups. Researchers randomly assign subjects to a treatment group using nature or an external event or situation.
However, even with random assignment, this research design cannot be called a true experiment since nature aspects are observational. Researchers can also exploit these aspects despite having no control over the independent variables.
Example of the natural experiment approach
An example of a natural experiment is the 2008 Oregon Health Study.
Oregon intended to allow more low-income people to participate in Medicaid.
Since they couldn't afford to cover every person who qualified for the program, the state used a random lottery to allocate program slots.
Researchers assessed the program's effectiveness by assigning the selected subjects to a randomly assigned treatment group, while those that didn't win the lottery were considered the control group.
- Differences between quasi-experiments and true experiments
There are several differences between a quasi-experiment and a true experiment:
Participants in true experiments are randomly assigned to the treatment or control group, while participants in a quasi-experiment are not assigned randomly.
In a quasi-experimental design, the control and treatment groups differ in unknown or unknowable ways, apart from the experimental treatments that are carried out. Therefore, the researcher should try as much as possible to control these differences.
Quasi-experimental designs have several "competing hypotheses," which compete with experimental manipulation to explain the observed results.
Quasi-experiments tend to have lower internal validity (the degree of confidence in the research outcomes) than true experiments, but they may offer higher external validity (whether findings can be extended to other contexts) as they involve real-world interventions instead of controlled interventions in artificial laboratory settings.
Despite the distinct difference between true and quasi-experimental research designs, these two research methodologies share the following aspects:
Both study methods subject participants to some form of treatment or conditions.
Researchers have the freedom to measure some of the outcomes of interest.
Researchers can test whether the differences in the outcomes are associated with the treatment.
- An example comparing a true experiment and quasi-experiment
Imagine you wanted to study the effects of junk food on obese people. Here's how you would do this as a true experiment and a quasi-experiment:
How to carry out a true experiment
In a true experiment, some participants would eat junk foods, while the rest would be in the control group, adhering to a regular diet. At the end of the study, you would record the health and discomfort of each group.
This kind of experiment would raise ethical concerns since the participants assigned to the treatment group are required to eat junk food against their will throughout the experiment. This calls for a quasi-experimental design.
How to carry out a quasi-experiment
In quasi-experimental research, you would start by finding out which participants want to try junk food and which prefer to stick to a regular diet. This allows you to assign these two groups based on subject choice.
In this case, you didn't assign participants to a particular group, so you can confidently use the results from the study.
When is a quasi-experimental design used?
Quasi-experimental designs are used when researchers don’t want to use randomization when evaluating their intervention.
What are the characteristics of quasi-experimental designs?
Some of the characteristics of a quasi-experimental design are:
Researchers don't randomly assign participants into groups, but study their existing characteristics and assign them accordingly.
Researchers study the participants in pre- and post-testing to determine the progress of the groups.
Quasi-experimental design is ethical since it doesn’t involve offering or withholding treatment at random.
Quasi-experimental design encompasses a broad range of non-randomized intervention studies. This design is employed when it is not ethical or logistically feasible to conduct randomized controlled trials. Researchers typically employ it when evaluating policy or educational interventions, or in medical or therapy scenarios.
How do you analyze data in a quasi-experimental design?
You can use two-group tests, time-series analysis, and regression analysis to analyze data in a quasi-experiment design. Each option has specific assumptions, strengths, limitations, and data requirements.
Should you be using a customer insights hub?
Do you want to discover previous research faster?
Do you share your research findings with others?
Do you analyze research data?
Start for free today, add your research, and get to key insights faster
Editor’s picks
Last updated: 18 April 2023
Last updated: 27 February 2023
Last updated: 22 August 2024
Last updated: 5 February 2023
Last updated: 16 April 2023
Last updated: 9 March 2023
Last updated: 30 April 2024
Last updated: 12 December 2023
Last updated: 11 March 2024
Last updated: 4 July 2024
Last updated: 6 March 2024
Last updated: 5 March 2024
Last updated: 13 May 2024
Latest articles
Related topics, .css-je19u9{-webkit-align-items:flex-end;-webkit-box-align:flex-end;-ms-flex-align:flex-end;align-items:flex-end;display:-webkit-box;display:-webkit-flex;display:-ms-flexbox;display:flex;-webkit-flex-direction:row;-ms-flex-direction:row;flex-direction:row;-webkit-box-flex-wrap:wrap;-webkit-flex-wrap:wrap;-ms-flex-wrap:wrap;flex-wrap:wrap;-webkit-box-pack:center;-ms-flex-pack:center;-webkit-justify-content:center;justify-content:center;row-gap:0;text-align:center;max-width:671px;}@media (max-width: 1079px){.css-je19u9{max-width:400px;}.css-je19u9>span{white-space:pre;}}@media (max-width: 799px){.css-je19u9{max-width:400px;}.css-je19u9>span{white-space:pre;}} decide what to .css-1kiodld{max-height:56px;display:-webkit-box;display:-webkit-flex;display:-ms-flexbox;display:flex;-webkit-align-items:center;-webkit-box-align:center;-ms-flex-align:center;align-items:center;}@media (max-width: 1079px){.css-1kiodld{display:none;}} build next, decide what to build next, log in or sign up.
Get started for free
Our systems are now restored following recent technical disruption, and we’re working hard to catch up on publishing. We apologise for the inconvenience caused. Find out more: https://www.cambridge.org/universitypress/about-us/news-and-blogs/cambridge-university-press-publishing-update-following-technical-disruption
We use cookies to distinguish you from other users and to provide you with a better experience on our websites. Close this message to accept cookies or find out how to manage your cookie settings .
Login Alert
- > The Cambridge Handbook of Research Methods and Statistics for the Social and Behavioral Sciences
- > Quasi-Experimental Research
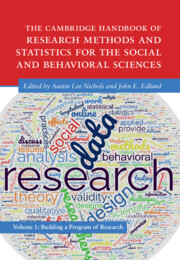
Book contents
- The Cambridge Handbook of Research Methods and Statistics for the Social and Behavioral Sciences
- Cambridge Handbooks in Psychology
- Copyright page
- Contributors
- Part I From Idea to Reality: The Basics of Research
- Part II The Building Blocks of a Study
- Part III Data Collection
- 13 Cross-Sectional Studies
- 14 Quasi-Experimental Research
- 15 Non-equivalent Control Group Pretest–Posttest Design in Social and Behavioral Research
- 16 Experimental Methods
- 17 Longitudinal Research: A World to Explore
- 18 Online Research Methods
- 19 Archival Data
- 20 Qualitative Research Design
- Part IV Statistical Approaches
- Part V Tips for a Successful Research Career
14 - Quasi-Experimental Research
from Part III - Data Collection
Published online by Cambridge University Press: 25 May 2023
In this chapter, we discuss the logic and practice of quasi-experimentation. Specifically, we describe four quasi-experimental designs – one-group pretest–posttest designs, non-equivalent group designs, regression discontinuity designs, and interrupted time-series designs – and their statistical analyses in detail. Both simple quasi-experimental designs and embellishments of these simple designs are presented. Potential threats to internal validity are illustrated along with means of addressing their potentially biasing effects so that these effects can be minimized. In contrast to quasi-experiments, randomized experiments are often thought to be the gold standard when estimating the effects of treatment interventions. However, circumstances frequently arise where quasi-experiments can usefully supplement randomized experiments or when quasi-experiments can fruitfully be used in place of randomized experiments. Researchers need to appreciate the relative strengths and weaknesses of the various quasi-experiments so they can choose among pre-specified designs or craft their own unique quasi-experiments.
Access options
Save book to kindle.
To save this book to your Kindle, first ensure [email protected] is added to your Approved Personal Document E-mail List under your Personal Document Settings on the Manage Your Content and Devices page of your Amazon account. Then enter the ‘name’ part of your Kindle email address below. Find out more about saving to your Kindle .
Note you can select to save to either the @free.kindle.com or @kindle.com variations. ‘@free.kindle.com’ emails are free but can only be saved to your device when it is connected to wi-fi. ‘@kindle.com’ emails can be delivered even when you are not connected to wi-fi, but note that service fees apply.
Find out more about the Kindle Personal Document Service .
- Quasi-Experimental Research
- By Charles S. Reichardt , Daniel Storage , Damon Abraham
- Edited by Austin Lee Nichols , Central European University, Vienna , John Edlund , Rochester Institute of Technology, New York
- Book: The Cambridge Handbook of Research Methods and Statistics for the Social and Behavioral Sciences
- Online publication: 25 May 2023
- Chapter DOI: https://doi.org/10.1017/9781009010054.015
Save book to Dropbox
To save content items to your account, please confirm that you agree to abide by our usage policies. If this is the first time you use this feature, you will be asked to authorise Cambridge Core to connect with your account. Find out more about saving content to Dropbox .
Save book to Google Drive
To save content items to your account, please confirm that you agree to abide by our usage policies. If this is the first time you use this feature, you will be asked to authorise Cambridge Core to connect with your account. Find out more about saving content to Google Drive .
Research Methodologies Guide
- Action Research
- Bibliometrics
- Case Studies
- Content Analysis
- Digital Scholarship This link opens in a new window
- Documentary
- Ethnography
- Focus Groups
- Grounded Theory
- Life Histories/Autobiographies
- Longitudinal
- Participant Observation
- Qualitative Research (General)
Quasi-Experimental Design
- Usability Studies
Quasi-Experimental Design is a unique research methodology because it is characterized by what is lacks. For example, Abraham & MacDonald (2011) state:
" Quasi-experimental research is similar to experimental research in that there is manipulation of an independent variable. It differs from experimental research because either there is no control group, no random selection, no random assignment, and/or no active manipulation. "
This type of research is often performed in cases where a control group cannot be created or random selection cannot be performed. This is often the case in certain medical and psychological studies.
For more information on quasi-experimental design, review the resources below:
Where to Start
Below are listed a few tools and online guides that can help you start your Quasi-experimental research. These include free online resources and resources available only through ISU Library.
- Quasi-Experimental Research Designs by Bruce A. Thyer This pocket guide describes the logic, design, and conduct of the range of quasi-experimental designs, encompassing pre-experiments, quasi-experiments making use of a control or comparison group, and time-series designs. An introductory chapter describes the valuable role these types of studies have played in social work, from the 1930s to the present. Subsequent chapters delve into each design type's major features, the kinds of questions it is capable of answering, and its strengths and limitations.
- Experimental and Quasi-Experimental Designs for Research by Donald T. Campbell; Julian C. Stanley. Call Number: Q175 C152e Written 1967 but still used heavily today, this book examines research designs for experimental and quasi-experimental research, with examples and judgments about each design's validity.
Online Resources
- Quasi-Experimental Design From the Web Center for Social Research Methods, this is a very good overview of quasi-experimental design.
- Experimental and Quasi-Experimental Research From Colorado State University.
- Quasi-experimental design--Wikipedia, the free encyclopedia Wikipedia can be a useful place to start your research- check the citations at the bottom of the article for more information.
- << Previous: Qualitative Research (General)
- Next: Sampling >>
- Last Updated: Sep 11, 2024 11:05 AM
- URL: https://instr.iastate.libguides.com/researchmethods

Want to create or adapt books like this? Learn more about how Pressbooks supports open publishing practices.
7.3 Quasi-Experimental Research
Learning objectives.
- Explain what quasi-experimental research is and distinguish it clearly from both experimental and correlational research.
- Describe three different types of quasi-experimental research designs (nonequivalent groups, pretest-posttest, and interrupted time series) and identify examples of each one.
The prefix quasi means “resembling.” Thus quasi-experimental research is research that resembles experimental research but is not true experimental research. Although the independent variable is manipulated, participants are not randomly assigned to conditions or orders of conditions (Cook & Campbell, 1979). Because the independent variable is manipulated before the dependent variable is measured, quasi-experimental research eliminates the directionality problem. But because participants are not randomly assigned—making it likely that there are other differences between conditions—quasi-experimental research does not eliminate the problem of confounding variables. In terms of internal validity, therefore, quasi-experiments are generally somewhere between correlational studies and true experiments.
Quasi-experiments are most likely to be conducted in field settings in which random assignment is difficult or impossible. They are often conducted to evaluate the effectiveness of a treatment—perhaps a type of psychotherapy or an educational intervention. There are many different kinds of quasi-experiments, but we will discuss just a few of the most common ones here.
Nonequivalent Groups Design
Recall that when participants in a between-subjects experiment are randomly assigned to conditions, the resulting groups are likely to be quite similar. In fact, researchers consider them to be equivalent. When participants are not randomly assigned to conditions, however, the resulting groups are likely to be dissimilar in some ways. For this reason, researchers consider them to be nonequivalent. A nonequivalent groups design , then, is a between-subjects design in which participants have not been randomly assigned to conditions.
Imagine, for example, a researcher who wants to evaluate a new method of teaching fractions to third graders. One way would be to conduct a study with a treatment group consisting of one class of third-grade students and a control group consisting of another class of third-grade students. This would be a nonequivalent groups design because the students are not randomly assigned to classes by the researcher, which means there could be important differences between them. For example, the parents of higher achieving or more motivated students might have been more likely to request that their children be assigned to Ms. Williams’s class. Or the principal might have assigned the “troublemakers” to Mr. Jones’s class because he is a stronger disciplinarian. Of course, the teachers’ styles, and even the classroom environments, might be very different and might cause different levels of achievement or motivation among the students. If at the end of the study there was a difference in the two classes’ knowledge of fractions, it might have been caused by the difference between the teaching methods—but it might have been caused by any of these confounding variables.
Of course, researchers using a nonequivalent groups design can take steps to ensure that their groups are as similar as possible. In the present example, the researcher could try to select two classes at the same school, where the students in the two classes have similar scores on a standardized math test and the teachers are the same sex, are close in age, and have similar teaching styles. Taking such steps would increase the internal validity of the study because it would eliminate some of the most important confounding variables. But without true random assignment of the students to conditions, there remains the possibility of other important confounding variables that the researcher was not able to control.
Pretest-Posttest Design
In a pretest-posttest design , the dependent variable is measured once before the treatment is implemented and once after it is implemented. Imagine, for example, a researcher who is interested in the effectiveness of an antidrug education program on elementary school students’ attitudes toward illegal drugs. The researcher could measure the attitudes of students at a particular elementary school during one week, implement the antidrug program during the next week, and finally, measure their attitudes again the following week. The pretest-posttest design is much like a within-subjects experiment in which each participant is tested first under the control condition and then under the treatment condition. It is unlike a within-subjects experiment, however, in that the order of conditions is not counterbalanced because it typically is not possible for a participant to be tested in the treatment condition first and then in an “untreated” control condition.
If the average posttest score is better than the average pretest score, then it makes sense to conclude that the treatment might be responsible for the improvement. Unfortunately, one often cannot conclude this with a high degree of certainty because there may be other explanations for why the posttest scores are better. One category of alternative explanations goes under the name of history . Other things might have happened between the pretest and the posttest. Perhaps an antidrug program aired on television and many of the students watched it, or perhaps a celebrity died of a drug overdose and many of the students heard about it. Another category of alternative explanations goes under the name of maturation . Participants might have changed between the pretest and the posttest in ways that they were going to anyway because they are growing and learning. If it were a yearlong program, participants might become less impulsive or better reasoners and this might be responsible for the change.
Another alternative explanation for a change in the dependent variable in a pretest-posttest design is regression to the mean . This refers to the statistical fact that an individual who scores extremely on a variable on one occasion will tend to score less extremely on the next occasion. For example, a bowler with a long-term average of 150 who suddenly bowls a 220 will almost certainly score lower in the next game. Her score will “regress” toward her mean score of 150. Regression to the mean can be a problem when participants are selected for further study because of their extreme scores. Imagine, for example, that only students who scored especially low on a test of fractions are given a special training program and then retested. Regression to the mean all but guarantees that their scores will be higher even if the training program has no effect. A closely related concept—and an extremely important one in psychological research—is spontaneous remission . This is the tendency for many medical and psychological problems to improve over time without any form of treatment. The common cold is a good example. If one were to measure symptom severity in 100 common cold sufferers today, give them a bowl of chicken soup every day, and then measure their symptom severity again in a week, they would probably be much improved. This does not mean that the chicken soup was responsible for the improvement, however, because they would have been much improved without any treatment at all. The same is true of many psychological problems. A group of severely depressed people today is likely to be less depressed on average in 6 months. In reviewing the results of several studies of treatments for depression, researchers Michael Posternak and Ivan Miller found that participants in waitlist control conditions improved an average of 10 to 15% before they received any treatment at all (Posternak & Miller, 2001). Thus one must generally be very cautious about inferring causality from pretest-posttest designs.
Does Psychotherapy Work?
Early studies on the effectiveness of psychotherapy tended to use pretest-posttest designs. In a classic 1952 article, researcher Hans Eysenck summarized the results of 24 such studies showing that about two thirds of patients improved between the pretest and the posttest (Eysenck, 1952). But Eysenck also compared these results with archival data from state hospital and insurance company records showing that similar patients recovered at about the same rate without receiving psychotherapy. This suggested to Eysenck that the improvement that patients showed in the pretest-posttest studies might be no more than spontaneous remission. Note that Eysenck did not conclude that psychotherapy was ineffective. He merely concluded that there was no evidence that it was, and he wrote of “the necessity of properly planned and executed experimental studies into this important field” (p. 323). You can read the entire article here:
http://psychclassics.yorku.ca/Eysenck/psychotherapy.htm
Fortunately, many other researchers took up Eysenck’s challenge, and by 1980 hundreds of experiments had been conducted in which participants were randomly assigned to treatment and control conditions, and the results were summarized in a classic book by Mary Lee Smith, Gene Glass, and Thomas Miller (Smith, Glass, & Miller, 1980). They found that overall psychotherapy was quite effective, with about 80% of treatment participants improving more than the average control participant. Subsequent research has focused more on the conditions under which different types of psychotherapy are more or less effective.

In a classic 1952 article, researcher Hans Eysenck pointed out the shortcomings of the simple pretest-posttest design for evaluating the effectiveness of psychotherapy.
Wikimedia Commons – CC BY-SA 3.0.
Interrupted Time Series Design
A variant of the pretest-posttest design is the interrupted time-series design . A time series is a set of measurements taken at intervals over a period of time. For example, a manufacturing company might measure its workers’ productivity each week for a year. In an interrupted time series-design, a time series like this is “interrupted” by a treatment. In one classic example, the treatment was the reduction of the work shifts in a factory from 10 hours to 8 hours (Cook & Campbell, 1979). Because productivity increased rather quickly after the shortening of the work shifts, and because it remained elevated for many months afterward, the researcher concluded that the shortening of the shifts caused the increase in productivity. Notice that the interrupted time-series design is like a pretest-posttest design in that it includes measurements of the dependent variable both before and after the treatment. It is unlike the pretest-posttest design, however, in that it includes multiple pretest and posttest measurements.
Figure 7.5 “A Hypothetical Interrupted Time-Series Design” shows data from a hypothetical interrupted time-series study. The dependent variable is the number of student absences per week in a research methods course. The treatment is that the instructor begins publicly taking attendance each day so that students know that the instructor is aware of who is present and who is absent. The top panel of Figure 7.5 “A Hypothetical Interrupted Time-Series Design” shows how the data might look if this treatment worked. There is a consistently high number of absences before the treatment, and there is an immediate and sustained drop in absences after the treatment. The bottom panel of Figure 7.5 “A Hypothetical Interrupted Time-Series Design” shows how the data might look if this treatment did not work. On average, the number of absences after the treatment is about the same as the number before. This figure also illustrates an advantage of the interrupted time-series design over a simpler pretest-posttest design. If there had been only one measurement of absences before the treatment at Week 7 and one afterward at Week 8, then it would have looked as though the treatment were responsible for the reduction. The multiple measurements both before and after the treatment suggest that the reduction between Weeks 7 and 8 is nothing more than normal week-to-week variation.
Figure 7.5 A Hypothetical Interrupted Time-Series Design

The top panel shows data that suggest that the treatment caused a reduction in absences. The bottom panel shows data that suggest that it did not.
Combination Designs
A type of quasi-experimental design that is generally better than either the nonequivalent groups design or the pretest-posttest design is one that combines elements of both. There is a treatment group that is given a pretest, receives a treatment, and then is given a posttest. But at the same time there is a control group that is given a pretest, does not receive the treatment, and then is given a posttest. The question, then, is not simply whether participants who receive the treatment improve but whether they improve more than participants who do not receive the treatment.
Imagine, for example, that students in one school are given a pretest on their attitudes toward drugs, then are exposed to an antidrug program, and finally are given a posttest. Students in a similar school are given the pretest, not exposed to an antidrug program, and finally are given a posttest. Again, if students in the treatment condition become more negative toward drugs, this could be an effect of the treatment, but it could also be a matter of history or maturation. If it really is an effect of the treatment, then students in the treatment condition should become more negative than students in the control condition. But if it is a matter of history (e.g., news of a celebrity drug overdose) or maturation (e.g., improved reasoning), then students in the two conditions would be likely to show similar amounts of change. This type of design does not completely eliminate the possibility of confounding variables, however. Something could occur at one of the schools but not the other (e.g., a student drug overdose), so students at the first school would be affected by it while students at the other school would not.
Finally, if participants in this kind of design are randomly assigned to conditions, it becomes a true experiment rather than a quasi experiment. In fact, it is the kind of experiment that Eysenck called for—and that has now been conducted many times—to demonstrate the effectiveness of psychotherapy.
Key Takeaways
- Quasi-experimental research involves the manipulation of an independent variable without the random assignment of participants to conditions or orders of conditions. Among the important types are nonequivalent groups designs, pretest-posttest, and interrupted time-series designs.
- Quasi-experimental research eliminates the directionality problem because it involves the manipulation of the independent variable. It does not eliminate the problem of confounding variables, however, because it does not involve random assignment to conditions. For these reasons, quasi-experimental research is generally higher in internal validity than correlational studies but lower than true experiments.
- Practice: Imagine that two college professors decide to test the effect of giving daily quizzes on student performance in a statistics course. They decide that Professor A will give quizzes but Professor B will not. They will then compare the performance of students in their two sections on a common final exam. List five other variables that might differ between the two sections that could affect the results.
Discussion: Imagine that a group of obese children is recruited for a study in which their weight is measured, then they participate for 3 months in a program that encourages them to be more active, and finally their weight is measured again. Explain how each of the following might affect the results:
- regression to the mean
- spontaneous remission
Cook, T. D., & Campbell, D. T. (1979). Quasi-experimentation: Design & analysis issues in field settings . Boston, MA: Houghton Mifflin.
Eysenck, H. J. (1952). The effects of psychotherapy: An evaluation. Journal of Consulting Psychology, 16 , 319–324.
Posternak, M. A., & Miller, I. (2001). Untreated short-term course of major depression: A meta-analysis of studies using outcomes from studies using wait-list control groups. Journal of Affective Disorders, 66 , 139–146.
Smith, M. L., Glass, G. V., & Miller, T. I. (1980). The benefits of psychotherapy . Baltimore, MD: Johns Hopkins University Press.
Research Methods in Psychology Copyright © 2016 by University of Minnesota is licensed under a Creative Commons Attribution-NonCommercial-ShareAlike 4.0 International License , except where otherwise noted.

An official website of the United States government
The .gov means it’s official. Federal government websites often end in .gov or .mil. Before sharing sensitive information, make sure you’re on a federal government site.
The site is secure. The https:// ensures that you are connecting to the official website and that any information you provide is encrypted and transmitted securely.
- Publications
- Account settings
The PMC website is updating on October 15, 2024. Learn More or Try it out now .
- Advanced Search
- Journal List
- J Am Med Inform Assoc
- v.13(1); Jan-Feb 2006
The Use and Interpretation of Quasi-Experimental Studies in Medical Informatics
Associated data.
Quasi-experimental study designs, often described as nonrandomized, pre-post intervention studies, are common in the medical informatics literature. Yet little has been written about the benefits and limitations of the quasi-experimental approach as applied to informatics studies. This paper outlines a relative hierarchy and nomenclature of quasi-experimental study designs that is applicable to medical informatics intervention studies. In addition, the authors performed a systematic review of two medical informatics journals, the Journal of the American Medical Informatics Association (JAMIA) and the International Journal of Medical Informatics (IJMI), to determine the number of quasi-experimental studies published and how the studies are classified on the above-mentioned relative hierarchy. They hope that future medical informatics studies will implement higher level quasi-experimental study designs that yield more convincing evidence for causal links between medical informatics interventions and outcomes.
Quasi-experimental studies encompass a broad range of nonrandomized intervention studies. These designs are frequently used when it is not logistically feasible or ethical to conduct a randomized controlled trial. Examples of quasi-experimental studies follow. As one example of a quasi-experimental study, a hospital introduces a new order-entry system and wishes to study the impact of this intervention on the number of medication-related adverse events before and after the intervention. As another example, an informatics technology group is introducing a pharmacy order-entry system aimed at decreasing pharmacy costs. The intervention is implemented and pharmacy costs before and after the intervention are measured.
In medical informatics, the quasi-experimental, sometimes called the pre-post intervention, design often is used to evaluate the benefits of specific interventions. The increasing capacity of health care institutions to collect routine clinical data has led to the growing use of quasi-experimental study designs in the field of medical informatics as well as in other medical disciplines. However, little is written about these study designs in the medical literature or in traditional epidemiology textbooks. 1 , 2 , 3 In contrast, the social sciences literature is replete with examples of ways to implement and improve quasi-experimental studies. 4 , 5 , 6
In this paper, we review the different pretest-posttest quasi-experimental study designs, their nomenclature, and the relative hierarchy of these designs with respect to their ability to establish causal associations between an intervention and an outcome. The example of a pharmacy order-entry system aimed at decreasing pharmacy costs will be used throughout this article to illustrate the different quasi-experimental designs. We discuss limitations of quasi-experimental designs and offer methods to improve them. We also perform a systematic review of four years of publications from two informatics journals to determine the number of quasi-experimental studies, classify these studies into their application domains, determine whether the potential limitations of quasi-experimental studies were acknowledged by the authors, and place these studies into the above-mentioned relative hierarchy.
The authors reviewed articles and book chapters on the design of quasi-experimental studies. 4 , 5 , 6 , 7 , 8 , 9 , 10 Most of the reviewed articles referenced two textbooks that were then reviewed in depth. 4 , 6
Key advantages and disadvantages of quasi-experimental studies, as they pertain to the study of medical informatics, were identified. The potential methodological flaws of quasi-experimental medical informatics studies, which have the potential to introduce bias, were also identified. In addition, a summary table outlining a relative hierarchy and nomenclature of quasi-experimental study designs is described. In general, the higher the design is in the hierarchy, the greater the internal validity that the study traditionally possesses because the evidence of the potential causation between the intervention and the outcome is strengthened. 4
We then performed a systematic review of four years of publications from two informatics journals. First, we determined the number of quasi-experimental studies. We then classified these studies on the above-mentioned hierarchy. We also classified the quasi-experimental studies according to their application domain. The categories of application domains employed were based on categorization used by Yearbooks of Medical Informatics 1992–2005 and were similar to the categories of application domains employed by Annual Symposiums of the American Medical Informatics Association. 11 The categories were (1) health and clinical management; (2) patient records; (3) health information systems; (4) medical signal processing and biomedical imaging; (5) decision support, knowledge representation, and management; (6) education and consumer informatics; and (7) bioinformatics. Because the quasi-experimental study design has recognized limitations, we sought to determine whether authors acknowledged the potential limitations of this design. Examples of acknowledgment included mention of lack of randomization, the potential for regression to the mean, the presence of temporal confounders and the mention of another design that would have more internal validity.
All original scientific manuscripts published between January 2000 and December 2003 in the Journal of the American Medical Informatics Association (JAMIA) and the International Journal of Medical Informatics (IJMI) were reviewed. One author (ADH) reviewed all the papers to identify the number of quasi-experimental studies. Other authors (ADH, JCM, JF) then independently reviewed all the studies identified as quasi-experimental. The three authors then convened as a group to resolve any disagreements in study classification, application domain, and acknowledgment of limitations.
Results and Discussion
What is a quasi-experiment.
Quasi-experiments are studies that aim to evaluate interventions but that do not use randomization. Similar to randomized trials, quasi-experiments aim to demonstrate causality between an intervention and an outcome. Quasi-experimental studies can use both preintervention and postintervention measurements as well as nonrandomly selected control groups.
Using this basic definition, it is evident that many published studies in medical informatics utilize the quasi-experimental design. Although the randomized controlled trial is generally considered to have the highest level of credibility with regard to assessing causality, in medical informatics, researchers often choose not to randomize the intervention for one or more reasons: (1) ethical considerations, (2) difficulty of randomizing subjects, (3) difficulty to randomize by locations (e.g., by wards), (4) small available sample size. Each of these reasons is discussed below.
Ethical considerations typically will not allow random withholding of an intervention with known efficacy. Thus, if the efficacy of an intervention has not been established, a randomized controlled trial is the design of choice to determine efficacy. But if the intervention under study incorporates an accepted, well-established therapeutic intervention, or if the intervention has either questionable efficacy or safety based on previously conducted studies, then the ethical issues of randomizing patients are sometimes raised. In the area of medical informatics, it is often believed prior to an implementation that an informatics intervention will likely be beneficial and thus medical informaticians and hospital administrators are often reluctant to randomize medical informatics interventions. In addition, there is often pressure to implement the intervention quickly because of its believed efficacy, thus not allowing researchers sufficient time to plan a randomized trial.
For medical informatics interventions, it is often difficult to randomize the intervention to individual patients or to individual informatics users. So while this randomization is technically possible, it is underused and thus compromises the eventual strength of concluding that an informatics intervention resulted in an outcome. For example, randomly allowing only half of medical residents to use pharmacy order-entry software at a tertiary care hospital is a scenario that hospital administrators and informatics users may not agree to for numerous reasons.
Similarly, informatics interventions often cannot be randomized to individual locations. Using the pharmacy order-entry system example, it may be difficult to randomize use of the system to only certain locations in a hospital or portions of certain locations. For example, if the pharmacy order-entry system involves an educational component, then people may apply the knowledge learned to nonintervention wards, thereby potentially masking the true effect of the intervention. When a design using randomized locations is employed successfully, the locations may be different in other respects (confounding variables), and this further complicates the analysis and interpretation.
In situations where it is known that only a small sample size will be available to test the efficacy of an intervention, randomization may not be a viable option. Randomization is beneficial because on average it tends to evenly distribute both known and unknown confounding variables between the intervention and control group. However, when the sample size is small, randomization may not adequately accomplish this balance. Thus, alternative design and analytical methods are often used in place of randomization when only small sample sizes are available.
What Are the Threats to Establishing Causality When Using Quasi-experimental Designs in Medical Informatics?
The lack of random assignment is the major weakness of the quasi-experimental study design. Associations identified in quasi-experiments meet one important requirement of causality since the intervention precedes the measurement of the outcome. Another requirement is that the outcome can be demonstrated to vary statistically with the intervention. Unfortunately, statistical association does not imply causality, especially if the study is poorly designed. Thus, in many quasi-experiments, one is most often left with the question: “Are there alternative explanations for the apparent causal association?” If these alternative explanations are credible, then the evidence of causation is less convincing. These rival hypotheses, or alternative explanations, arise from principles of epidemiologic study design.
Shadish et al. 4 outline nine threats to internal validity that are outlined in ▶ . Internal validity is defined as the degree to which observed changes in outcomes can be correctly inferred to be caused by an exposure or an intervention. In quasi-experimental studies of medical informatics, we believe that the methodological principles that most often result in alternative explanations for the apparent causal effect include (a) difficulty in measuring or controlling for important confounding variables, particularly unmeasured confounding variables, which can be viewed as a subset of the selection threat in ▶ ; (b) results being explained by the statistical principle of regression to the mean . Each of these latter two principles is discussed in turn.
Threats to Internal Validity
1. Ambiguous temporal precedence: Lack of clarity about whether intervention occurred before outcome |
2. Selection: Systematic differences over conditions in respondent characteristics that could also cause the observed effect |
3. History: Events occurring concurrently with intervention could cause the observed effect |
4. Maturation: Naturally occurring changes over time could be confused with a treatment effect |
5. Regression: When units are selected for their extreme scores, they will often have less extreme subsequent scores, an occurrence that can be confused with an intervention effect |
6. Attrition: Loss of respondents can produce artifactual effects if that loss is correlated with intervention |
7. Testing: Exposure to a test can affect scores on subsequent exposures to that test |
8. Instrumentation: The nature of a measurement may change over time or conditions |
9. Interactive effects: The impact of an intervention may depend on the level of another intervention |
Adapted from Shadish et al. 4
An inability to sufficiently control for important confounding variables arises from the lack of randomization. A variable is a confounding variable if it is associated with the exposure of interest and is also associated with the outcome of interest; the confounding variable leads to a situation where a causal association between a given exposure and an outcome is observed as a result of the influence of the confounding variable. For example, in a study aiming to demonstrate that the introduction of a pharmacy order-entry system led to lower pharmacy costs, there are a number of important potential confounding variables (e.g., severity of illness of the patients, knowledge and experience of the software users, other changes in hospital policy) that may have differed in the preintervention and postintervention time periods ( ▶ ). In a multivariable regression, the first confounding variable could be addressed with severity of illness measures, but the second confounding variable would be difficult if not nearly impossible to measure and control. In addition, potential confounding variables that are unmeasured or immeasurable cannot be controlled for in nonrandomized quasi-experimental study designs and can only be properly controlled by the randomization process in randomized controlled trials.

Example of confounding. To get the true effect of the intervention of interest, we need to control for the confounding variable.
Another important threat to establishing causality is regression to the mean. 12 , 13 , 14 This widespread statistical phenomenon can result in wrongly concluding that an effect is due to the intervention when in reality it is due to chance. The phenomenon was first described in 1886 by Francis Galton who measured the adult height of children and their parents. He noted that when the average height of the parents was greater than the mean of the population, the children tended to be shorter than their parents, and conversely, when the average height of the parents was shorter than the population mean, the children tended to be taller than their parents.
In medical informatics, what often triggers the development and implementation of an intervention is a rise in the rate above the mean or norm. For example, increasing pharmacy costs and adverse events may prompt hospital informatics personnel to design and implement pharmacy order-entry systems. If this rise in costs or adverse events is really just an extreme observation that is still within the normal range of the hospital's pharmaceutical costs (i.e., the mean pharmaceutical cost for the hospital has not shifted), then the statistical principle of regression to the mean predicts that these elevated rates will tend to decline even without intervention. However, often informatics personnel and hospital administrators cannot wait passively for this decline to occur. Therefore, hospital personnel often implement one or more interventions, and if a decline in the rate occurs, they may mistakenly conclude that the decline is causally related to the intervention. In fact, an alternative explanation for the finding could be regression to the mean.
What Are the Different Quasi-experimental Study Designs?
In the social sciences literature, quasi-experimental studies are divided into four study design groups 4 , 6 :
- Quasi-experimental designs without control groups
- Quasi-experimental designs that use control groups but no pretest
- Quasi-experimental designs that use control groups and pretests
- Interrupted time-series designs
There is a relative hierarchy within these categories of study designs, with category D studies being sounder than categories C, B, or A in terms of establishing causality. Thus, if feasible from a design and implementation point of view, investigators should aim to design studies that fall in to the higher rated categories. Shadish et al. 4 discuss 17 possible designs, with seven designs falling into category A, three designs in category B, and six designs in category C, and one major design in category D. In our review, we determined that most medical informatics quasi-experiments could be characterized by 11 of 17 designs, with six study designs in category A, one in category B, three designs in category C, and one design in category D because the other study designs were not used or feasible in the medical informatics literature. Thus, for simplicity, we have summarized the 11 study designs most relevant to medical informatics research in ▶ .
Relative Hierarchy of Quasi-experimental Designs
Quasi-experimental Study Designs | Design Notation |
---|---|
A. Quasi-experimental designs without control groups | |
1. The one-group posttest-only design | X O1 |
2. The one-group pretest-posttest design | O1 X O2 |
3. The one-group pretest-posttest design using a double pretest | O1 O2 X O3 |
4. The one-group pretest-posttest design using a nonequivalent dependent variable | (O1a, O1b) X (O2a, O2b) |
5. The removed-treatment design | O1 X O2 O3 removeX O4 |
6. The repeated-treatment design | O1 X O2 removeX O3 X O4 |
B. Quasi-experimental designs that use a control group but no pretest | |
1. Posttest-only design with nonequivalent groups | Intervention group: X O1 |
Control group: O2 | |
C. Quasi-experimental designs that use control groups and pretests | |
1. Untreated control group with dependent pretest and posttest samples | Intervention group: O1a X O2a |
Control group: O1b O2b | |
2. Untreated control group design with dependent pretest and posttest samples using a double pretest | Intervention group: O1a O2a X O3a |
Control group: O1b O2b O3b | |
3. Untreated control group design with dependent pretest and posttest samples using switching replications | Intervention group: O1a X O2a O3a |
Control group: O1b O2b X O3b | |
D. Interrupted time-series design | |
1. Multiple pretest and posttest observations spaced at equal intervals of time | O1 O2 O3 O4 O5 X O6 O7 O8 O9 O10 |
O = Observational Measurement; X = Intervention Under Study. Time moves from left to right.
The nomenclature and relative hierarchy were used in the systematic review of four years of JAMIA and the IJMI. Similar to the relative hierarchy that exists in the evidence-based literature that assigns a hierarchy to randomized controlled trials, cohort studies, case-control studies, and case series, the hierarchy in ▶ is not absolute in that in some cases, it may be infeasible to perform a higher level study. For example, there may be instances where an A6 design established stronger causality than a B1 design. 15 , 16 , 17
Quasi-experimental Designs without Control Groups
Here, X is the intervention and O is the outcome variable (this notation is continued throughout the article). In this study design, an intervention (X) is implemented and a posttest observation (O1) is taken. For example, X could be the introduction of a pharmacy order-entry intervention and O1 could be the pharmacy costs following the intervention. This design is the weakest of the quasi-experimental designs that are discussed in this article. Without any pretest observations or a control group, there are multiple threats to internal validity. Unfortunately, this study design is often used in medical informatics when new software is introduced since it may be difficult to have pretest measurements due to time, technical, or cost constraints.
This is a commonly used study design. A single pretest measurement is taken (O1), an intervention (X) is implemented, and a posttest measurement is taken (O2). In this instance, period O1 frequently serves as the “control” period. For example, O1 could be pharmacy costs prior to the intervention, X could be the introduction of a pharmacy order-entry system, and O2 could be the pharmacy costs following the intervention. Including a pretest provides some information about what the pharmacy costs would have been had the intervention not occurred.
The advantage of this study design over A2 is that adding a second pretest prior to the intervention helps provide evidence that can be used to refute the phenomenon of regression to the mean and confounding as alternative explanations for any observed association between the intervention and the posttest outcome. For example, in a study where a pharmacy order-entry system led to lower pharmacy costs (O3 < O2 and O1), if one had two preintervention measurements of pharmacy costs (O1 and O2) and they were both elevated, this would suggest that there was a decreased likelihood that O3 is lower due to confounding and regression to the mean. Similarly, extending this study design by increasing the number of measurements postintervention could also help to provide evidence against confounding and regression to the mean as alternate explanations for observed associations.
This design involves the inclusion of a nonequivalent dependent variable ( b ) in addition to the primary dependent variable ( a ). Variables a and b should assess similar constructs; that is, the two measures should be affected by similar factors and confounding variables except for the effect of the intervention. Variable a is expected to change because of the intervention X, whereas variable b is not. Taking our example, variable a could be pharmacy costs and variable b could be the length of stay of patients. If our informatics intervention is aimed at decreasing pharmacy costs, we would expect to observe a decrease in pharmacy costs but not in the average length of stay of patients. However, a number of important confounding variables, such as severity of illness and knowledge of software users, might affect both outcome measures. Thus, if the average length of stay did not change following the intervention but pharmacy costs did, then the data are more convincing than if just pharmacy costs were measured.
The Removed-Treatment Design
This design adds a third posttest measurement (O3) to the one-group pretest-posttest design and then removes the intervention before a final measure (O4) is made. The advantage of this design is that it allows one to test hypotheses about the outcome in the presence of the intervention and in the absence of the intervention. Thus, if one predicts a decrease in the outcome between O1 and O2 (after implementation of the intervention), then one would predict an increase in the outcome between O3 and O4 (after removal of the intervention). One caveat is that if the intervention is thought to have persistent effects, then O4 needs to be measured after these effects are likely to have disappeared. For example, a study would be more convincing if it demonstrated that pharmacy costs decreased after pharmacy order-entry system introduction (O2 and O3 less than O1) and that when the order-entry system was removed or disabled, the costs increased (O4 greater than O2 and O3 and closer to O1). In addition, there are often ethical issues in this design in terms of removing an intervention that may be providing benefit.
The Repeated-Treatment Design
The advantage of this design is that it demonstrates reproducibility of the association between the intervention and the outcome. For example, the association is more likely to be causal if one demonstrates that a pharmacy order-entry system results in decreased pharmacy costs when it is first introduced and again when it is reintroduced following an interruption of the intervention. As for design A5, the assumption must be made that the effect of the intervention is transient, which is most often applicable to medical informatics interventions. Because in this design, subjects may serve as their own controls, this may yield greater statistical efficiency with fewer numbers of subjects.
Quasi-experimental Designs That Use a Control Group but No Pretest
An intervention X is implemented for one group and compared to a second group. The use of a comparison group helps prevent certain threats to validity including the ability to statistically adjust for confounding variables. Because in this study design, the two groups may not be equivalent (assignment to the groups is not by randomization), confounding may exist. For example, suppose that a pharmacy order-entry intervention was instituted in the medical intensive care unit (MICU) and not the surgical intensive care unit (SICU). O1 would be pharmacy costs in the MICU after the intervention and O2 would be pharmacy costs in the SICU after the intervention. The absence of a pretest makes it difficult to know whether a change has occurred in the MICU. Also, the absence of pretest measurements comparing the SICU to the MICU makes it difficult to know whether differences in O1 and O2 are due to the intervention or due to other differences in the two units (confounding variables).
Quasi-experimental Designs That Use Control Groups and Pretests
The reader should note that with all the studies in this category, the intervention is not randomized. The control groups chosen are comparison groups. Obtaining pretest measurements on both the intervention and control groups allows one to assess the initial comparability of the groups. The assumption is that if the intervention and the control groups are similar at the pretest, the smaller the likelihood there is of important confounding variables differing between the two groups.
The use of both a pretest and a comparison group makes it easier to avoid certain threats to validity. However, because the two groups are nonequivalent (assignment to the groups is not by randomization), selection bias may exist. Selection bias exists when selection results in differences in unit characteristics between conditions that may be related to outcome differences. For example, suppose that a pharmacy order-entry intervention was instituted in the MICU and not the SICU. If preintervention pharmacy costs in the MICU (O1a) and SICU (O1b) are similar, it suggests that it is less likely that there are differences in the important confounding variables between the two units. If MICU postintervention costs (O2a) are less than preintervention MICU costs (O1a), but SICU costs (O1b) and (O2b) are similar, this suggests that the observed outcome may be causally related to the intervention.
In this design, the pretests are administered at two different times. The main advantage of this design is that it controls for potentially different time-varying confounding effects in the intervention group and the comparison group. In our example, measuring points O1 and O2 would allow for the assessment of time-dependent changes in pharmacy costs, e.g., due to differences in experience of residents, preintervention between the intervention and control group, and whether these changes were similar or different.
With this study design, the researcher administers an intervention at a later time to a group that initially served as a nonintervention control. The advantage of this design over design C2 is that it demonstrates reproducibility in two different settings. This study design is not limited to two groups; in fact, the study results have greater validity if the intervention effect is replicated in different groups at multiple times. In the example of a pharmacy order-entry system, one could implement or intervene in the MICU and then at a later time, intervene in the SICU. This latter design is often very applicable to medical informatics where new technology and new software is often introduced or made available gradually.
Interrupted Time-Series Designs
An interrupted time-series design is one in which a string of consecutive observations equally spaced in time is interrupted by the imposition of a treatment or intervention. The advantage of this design is that with multiple measurements both pre- and postintervention, it is easier to address and control for confounding and regression to the mean. In addition, statistically, there is a more robust analytic capability, and there is the ability to detect changes in the slope or intercept as a result of the intervention in addition to a change in the mean values. 18 A change in intercept could represent an immediate effect while a change in slope could represent a gradual effect of the intervention on the outcome. In the example of a pharmacy order-entry system, O1 through O5 could represent monthly pharmacy costs preintervention and O6 through O10 monthly pharmacy costs post the introduction of the pharmacy order-entry system. Interrupted time-series designs also can be further strengthened by incorporating many of the design features previously mentioned in other categories (such as removal of the treatment, inclusion of a nondependent outcome variable, or the addition of a control group).
Systematic Review Results
The results of the systematic review are in ▶ . In the four-year period of JAMIA publications that the authors reviewed, 25 quasi-experimental studies among 22 articles were published. Of these 25, 15 studies were of category A, five studies were of category B, two studies were of category C, and no studies were of category D. Although there were no studies of category D (interrupted time-series analyses), three of the studies classified as category A had data collected that could have been analyzed as an interrupted time-series analysis. Nine of the 25 studies (36%) mentioned at least one of the potential limitations of the quasi-experimental study design. In the four-year period of IJMI publications reviewed by the authors, nine quasi-experimental studies among eight manuscripts were published. Of these nine, five studies were of category A, one of category B, one of category C, and two of category D. Two of the nine studies (22%) mentioned at least one of the potential limitations of the quasi-experimental study design.
Systematic Review of Four Years of Quasi-designs in JAMIA
Study | Journal | Informatics Topic Category | Quasi-experimental Design | Limitation of Quasi-design Mentioned in Article |
---|---|---|---|---|
Staggers and Kobus | JAMIA | 1 | Counterbalanced study design | Yes |
Schriger et al. | JAMIA | 1 | A5 | Yes |
Patel et al. | JAMIA | 2 | A5 (study 1, phase 1) | No |
Patel et al. | JAMIA | 2 | A2 (study 1, phase 2) | No |
Borowitz | JAMIA | 1 | A2 | No |
Patterson and Harasym | JAMIA | 6 | C1 | Yes |
Rocha et al. | JAMIA | 5 | A2 | Yes |
Lovis et al. | JAMIA | 1 | Counterbalanced study design | No |
Hersh et al. | JAMIA | 6 | B1 | No |
Makoul et al. | JAMIA | 2 | B1 | Yes |
Ruland | JAMIA | 3 | B1 | No |
DeLusignan et al. | JAMIA | 1 | A1 | No |
Mekhjian et al. | JAMIA | 1 | A2 (study design 1) | Yes |
Mekhjian et al. | JAMIA | 1 | B1 (study design 2) | Yes |
Ammenwerth et al. | JAMIA | 1 | A2 | No |
Oniki et al. | JAMIA | 5 | C1 | Yes |
Liederman and Morefield | JAMIA | 1 | A1 (study 1) | No |
Liederman and Morefield | JAMIA | 1 | A2 (study 2) | No |
Rotich et al. | JAMIA | 2 | A2 | No |
Payne et al. | JAMIA | 1 | A1 | No |
Hoch et al. | JAMIA | 3 | A2 | No |
Laerum et al. | JAMIA | 1 | B1 | Yes |
Devine et al. | JAMIA | 1 | Counterbalanced study design | |
Dunbar et al. | JAMIA | 6 | A1 | |
Lenert et al. | JAMIA | 6 | A2 | |
Koide et al. | IJMI | 5 | D4 | No |
Gonzalez-Hendrich et al. | IJMI | 2 | A1 | No |
Anantharaman and Swee Han | IJMI | 3 | B1 | No |
Chae et al. | IJMI | 6 | A2 | No |
Lin et al. | IJMI | 3 | A1 | No |
Mikulich et al. | IJMI | 1 | A2 | Yes |
Hwang et al. | IJMI | 1 | A2 | Yes |
Park et al. | IJMI | 1 | C2 | No |
Park et al. | IJMI | 1 | D4 | No |
JAMIA = Journal of the American Medical Informatics Association; IJMI = International Journal of Medical Informatics.
In addition, three studies from JAMIA were based on a counterbalanced design. A counterbalanced design is a higher order study design than other studies in category A. The counterbalanced design is sometimes referred to as a Latin-square arrangement. In this design, all subjects receive all the different interventions but the order of intervention assignment is not random. 19 This design can only be used when the intervention is compared against some existing standard, for example, if a new PDA-based order entry system is to be compared to a computer terminal–based order entry system. In this design, all subjects receive the new PDA-based order entry system and the old computer terminal-based order entry system. The counterbalanced design is a within-participants design, where the order of the intervention is varied (e.g., one group is given software A followed by software B and another group is given software B followed by software A). The counterbalanced design is typically used when the available sample size is small, thus preventing the use of randomization. This design also allows investigators to study the potential effect of ordering of the informatics intervention.
Although quasi-experimental study designs are ubiquitous in the medical informatics literature, as evidenced by 34 studies in the past four years of the two informatics journals, little has been written about the benefits and limitations of the quasi-experimental approach. As we have outlined in this paper, a relative hierarchy and nomenclature of quasi-experimental study designs exist, with some designs being more likely than others to permit causal interpretations of observed associations. Strengths and limitations of a particular study design should be discussed when presenting data collected in the setting of a quasi-experimental study. Future medical informatics investigators should choose the strongest design that is feasible given the particular circumstances.
Supplementary Material
Dr. Harris was supported by NIH grants K23 AI01752-01A1 and R01 AI60859-01A1. Dr. Perencevich was supported by a VA Health Services Research and Development Service (HSR&D) Research Career Development Award (RCD-02026-1). Dr. Finkelstein was supported by NIH grant RO1 HL71690.

Critical Appraisal Resources for Evidence-Based Nursing Practice
- Levels of Evidence
- Systematic Reviews
- Randomized Controlled Trials
- Quasi-Experimental Studies
What is a Quasi-Experimental Study?
Pro tips: quasi-experimental checklist, articles on quasi-experimental design and methodology.
- Case-Control Studies
- Cohort Studies
- Analytical Cross-Sectional Studies
- Qualitative Research
E-Books for Terminology and Definitions
Quasi-experimental studies are a type of quantitative research used to investigate the effectiveness of interventions or treatments. These types of studies involve manipulation of the independent variable, yet they lack certain elements of a fully experimental design. Quasi-experimental studies have no random assignment of study subjects and lack a control group (Schmidt & Brown, 2019, p. 177). However, they may have a non-equivalent comparison group (Krishnan, 2019).
Krishnan P. (2019). A review of the non-equivalent control group post-test-only design . Nurse Researcher , 26 (2), 37–40. https://doi.org/10.7748/nr.2018.e1582
Schmidt N. A. & Brown J. M. (2019). Evidence-based practice for nurses: Appraisal and application of research (4th ed.). Jones & Bartlett Learning.
Each JBI Checklist provides tips and guidance on what to look for to answer each question. These tips begin on page 4.
Below are some additional Frequently Asked Questions about the Quasi-Experimental Checklist that have been asked students in previous semesters.
The 'cause' refers to the independent variable that is being manipulated to observe an 'effect.' The 'effect' is the dependent variable, or the outcome. You will often find this information in the beginning of the study in the objectives/purpose/aim/research question section. Is this information clearly stated? For example: "The purpose of this study is to identify whether mindfulness-based stress reduction ('the cause') reduces anxiety ('the effect') in cancer patients." | |
Check for information about the internal reliability or internal consistency of the research instruments (scales, questionnaires, surveys, tools, etc.) used in the study. Look for the Cronbach's alpha statistic which is used to indicate internal reliability of an instrument. |
Maciejewski, M. L. (2020). Quasi-experimental design . Biostatistics & Epidemiology , 4(1), 38-47. doi:10.1080/24709360.2018.1477468
Maciejewski, M. L., Curtis, L. H., & Dowd, B. (2013). Study design elements for rigorous quasi-experimental comparative effectiveness research . Journal of Comparative Effectiveness Research , 2 (2), 159–173. https://doi.org/10.2217/cer.13.7
Miller, C. J., Smith, S. N., & Pugatch, M. (2020). Experimental and quasi-experimental designs in implementation research . Psychiatry Research , 283 , 112452. https://doi.org/10.1016/j.psychres.2019.06.027
Siedlecki S. L. (2020). Quasi-experimental research designs . Clinical Nurse Specialist , 34 (5), 198–202. https://doi.org/10.1097/NUR.0000000000000540
- << Previous: Randomized Controlled Trials
- Next: Case-Control Studies >>
- Last Updated: Feb 22, 2024 11:26 AM
- URL: https://libguides.utoledo.edu/nursingappraisal
- Search Menu
Sign in through your institution
- Advance articles
- Featured articles
- Virtual Issues
- Browse content in B - History of Economic Thought, Methodology, and Heterodox Approaches
- Browse content in B4 - Economic Methodology
- B49 - Other
- Browse content in C - Mathematical and Quantitative Methods
- Browse content in C0 - General
- C01 - Econometrics
- Browse content in C1 - Econometric and Statistical Methods and Methodology: General
- C10 - General
- C11 - Bayesian Analysis: General
- C12 - Hypothesis Testing: General
- C13 - Estimation: General
- C14 - Semiparametric and Nonparametric Methods: General
- C15 - Statistical Simulation Methods: General
- Browse content in C2 - Single Equation Models; Single Variables
- C21 - Cross-Sectional Models; Spatial Models; Treatment Effect Models; Quantile Regressions
- C22 - Time-Series Models; Dynamic Quantile Regressions; Dynamic Treatment Effect Models; Diffusion Processes
- C23 - Panel Data Models; Spatio-temporal Models
- C26 - Instrumental Variables (IV) Estimation
- Browse content in C3 - Multiple or Simultaneous Equation Models; Multiple Variables
- C31 - Cross-Sectional Models; Spatial Models; Treatment Effect Models; Quantile Regressions; Social Interaction Models
- C33 - Panel Data Models; Spatio-temporal Models
- C34 - Truncated and Censored Models; Switching Regression Models
- C35 - Discrete Regression and Qualitative Choice Models; Discrete Regressors; Proportions
- C36 - Instrumental Variables (IV) Estimation
- Browse content in C4 - Econometric and Statistical Methods: Special Topics
- C41 - Duration Analysis; Optimal Timing Strategies
- C44 - Operations Research; Statistical Decision Theory
- Browse content in C5 - Econometric Modeling
- C50 - General
- C51 - Model Construction and Estimation
- C52 - Model Evaluation, Validation, and Selection
- C53 - Forecasting and Prediction Methods; Simulation Methods
- C54 - Quantitative Policy Modeling
- C55 - Large Data Sets: Modeling and Analysis
- C57 - Econometrics of Games and Auctions
- Browse content in C6 - Mathematical Methods; Programming Models; Mathematical and Simulation Modeling
- C61 - Optimization Techniques; Programming Models; Dynamic Analysis
- C62 - Existence and Stability Conditions of Equilibrium
- C63 - Computational Techniques; Simulation Modeling
- C67 - Input-Output Models
- C68 - Computable General Equilibrium Models
- Browse content in C7 - Game Theory and Bargaining Theory
- C70 - General
- C72 - Noncooperative Games
- C73 - Stochastic and Dynamic Games; Evolutionary Games; Repeated Games
- C78 - Bargaining Theory; Matching Theory
- C79 - Other
- Browse content in C8 - Data Collection and Data Estimation Methodology; Computer Programs
- C81 - Methodology for Collecting, Estimating, and Organizing Microeconomic Data; Data Access
- C83 - Survey Methods; Sampling Methods
- Browse content in C9 - Design of Experiments
- C90 - General
- C91 - Laboratory, Individual Behavior
- C92 - Laboratory, Group Behavior
- C93 - Field Experiments
- Browse content in D - Microeconomics
- Browse content in D0 - General
- D01 - Microeconomic Behavior: Underlying Principles
- D02 - Institutions: Design, Formation, Operations, and Impact
- D03 - Behavioral Microeconomics: Underlying Principles
- Browse content in D1 - Household Behavior and Family Economics
- D11 - Consumer Economics: Theory
- D12 - Consumer Economics: Empirical Analysis
- D13 - Household Production and Intrahousehold Allocation
- D14 - Household Saving; Personal Finance
- D15 - Intertemporal Household Choice: Life Cycle Models and Saving
- D18 - Consumer Protection
- Browse content in D2 - Production and Organizations
- D21 - Firm Behavior: Theory
- D22 - Firm Behavior: Empirical Analysis
- D23 - Organizational Behavior; Transaction Costs; Property Rights
- D24 - Production; Cost; Capital; Capital, Total Factor, and Multifactor Productivity; Capacity
- D25 - Intertemporal Firm Choice: Investment, Capacity, and Financing
- D29 - Other
- Browse content in D3 - Distribution
- D30 - General
- D31 - Personal Income, Wealth, and Their Distributions
- Browse content in D4 - Market Structure, Pricing, and Design
- D40 - General
- D41 - Perfect Competition
- D42 - Monopoly
- D43 - Oligopoly and Other Forms of Market Imperfection
- D44 - Auctions
- D47 - Market Design
- Browse content in D5 - General Equilibrium and Disequilibrium
- D50 - General
- D51 - Exchange and Production Economies
- D52 - Incomplete Markets
- D53 - Financial Markets
- D57 - Input-Output Tables and Analysis
- D58 - Computable and Other Applied General Equilibrium Models
- Browse content in D6 - Welfare Economics
- D60 - General
- D61 - Allocative Efficiency; Cost-Benefit Analysis
- D62 - Externalities
- D63 - Equity, Justice, Inequality, and Other Normative Criteria and Measurement
- D64 - Altruism; Philanthropy
- Browse content in D7 - Analysis of Collective Decision-Making
- D70 - General
- D71 - Social Choice; Clubs; Committees; Associations
- D72 - Political Processes: Rent-seeking, Lobbying, Elections, Legislatures, and Voting Behavior
- D73 - Bureaucracy; Administrative Processes in Public Organizations; Corruption
- D74 - Conflict; Conflict Resolution; Alliances; Revolutions
- D78 - Positive Analysis of Policy Formulation and Implementation
- Browse content in D8 - Information, Knowledge, and Uncertainty
- D80 - General
- D81 - Criteria for Decision-Making under Risk and Uncertainty
- D82 - Asymmetric and Private Information; Mechanism Design
- D83 - Search; Learning; Information and Knowledge; Communication; Belief; Unawareness
- D84 - Expectations; Speculations
- D85 - Network Formation and Analysis: Theory
- D86 - Economics of Contract: Theory
- Browse content in D9 - Micro-Based Behavioral Economics
- D90 - General
- D91 - Role and Effects of Psychological, Emotional, Social, and Cognitive Factors on Decision Making
- D92 - Intertemporal Firm Choice, Investment, Capacity, and Financing
- Browse content in E - Macroeconomics and Monetary Economics
- Browse content in E0 - General
- E00 - General
- E03 - Behavioral Macroeconomics
- Browse content in E1 - General Aggregative Models
- E10 - General
- E12 - Keynes; Keynesian; Post-Keynesian
- E13 - Neoclassical
- E17 - Forecasting and Simulation: Models and Applications
- Browse content in E2 - Consumption, Saving, Production, Investment, Labor Markets, and Informal Economy
- E20 - General
- E21 - Consumption; Saving; Wealth
- E22 - Investment; Capital; Intangible Capital; Capacity
- E23 - Production
- E24 - Employment; Unemployment; Wages; Intergenerational Income Distribution; Aggregate Human Capital; Aggregate Labor Productivity
- E25 - Aggregate Factor Income Distribution
- E26 - Informal Economy; Underground Economy
- E27 - Forecasting and Simulation: Models and Applications
- Browse content in E3 - Prices, Business Fluctuations, and Cycles
- E30 - General
- E31 - Price Level; Inflation; Deflation
- E32 - Business Fluctuations; Cycles
- E37 - Forecasting and Simulation: Models and Applications
- Browse content in E4 - Money and Interest Rates
- E40 - General
- E41 - Demand for Money
- E42 - Monetary Systems; Standards; Regimes; Government and the Monetary System; Payment Systems
- E43 - Interest Rates: Determination, Term Structure, and Effects
- E44 - Financial Markets and the Macroeconomy
- E49 - Other
- Browse content in E5 - Monetary Policy, Central Banking, and the Supply of Money and Credit
- E50 - General
- E51 - Money Supply; Credit; Money Multipliers
- E52 - Monetary Policy
- E58 - Central Banks and Their Policies
- Browse content in E6 - Macroeconomic Policy, Macroeconomic Aspects of Public Finance, and General Outlook
- E60 - General
- E61 - Policy Objectives; Policy Designs and Consistency; Policy Coordination
- E62 - Fiscal Policy
- E65 - Studies of Particular Policy Episodes
- Browse content in E7 - Macro-Based Behavioral Economics
- E70 - General
- E71 - Role and Effects of Psychological, Emotional, Social, and Cognitive Factors on the Macro Economy
- Browse content in F - International Economics
- Browse content in F1 - Trade
- F10 - General
- F11 - Neoclassical Models of Trade
- F12 - Models of Trade with Imperfect Competition and Scale Economies; Fragmentation
- F13 - Trade Policy; International Trade Organizations
- F14 - Empirical Studies of Trade
- F15 - Economic Integration
- F16 - Trade and Labor Market Interactions
- F17 - Trade Forecasting and Simulation
- F18 - Trade and Environment
- F19 - Other
- Browse content in F2 - International Factor Movements and International Business
- F20 - General
- F21 - International Investment; Long-Term Capital Movements
- F22 - International Migration
- F23 - Multinational Firms; International Business
- Browse content in F3 - International Finance
- F30 - General
- F31 - Foreign Exchange
- F32 - Current Account Adjustment; Short-Term Capital Movements
- F33 - International Monetary Arrangements and Institutions
- F34 - International Lending and Debt Problems
- F36 - Financial Aspects of Economic Integration
- Browse content in F4 - Macroeconomic Aspects of International Trade and Finance
- F40 - General
- F41 - Open Economy Macroeconomics
- F42 - International Policy Coordination and Transmission
- F43 - Economic Growth of Open Economies
- F44 - International Business Cycles
- Browse content in F5 - International Relations, National Security, and International Political Economy
- F50 - General
- F53 - International Agreements and Observance; International Organizations
- Browse content in F6 - Economic Impacts of Globalization
- F60 - General
- F62 - Macroeconomic Impacts
- F63 - Economic Development
- F64 - Environment
- F65 - Finance
- Browse content in G - Financial Economics
- Browse content in G0 - General
- G01 - Financial Crises
- G02 - Behavioral Finance: Underlying Principles
- Browse content in G1 - General Financial Markets
- G10 - General
- G11 - Portfolio Choice; Investment Decisions
- G12 - Asset Pricing; Trading volume; Bond Interest Rates
- G13 - Contingent Pricing; Futures Pricing
- G14 - Information and Market Efficiency; Event Studies; Insider Trading
- G15 - International Financial Markets
- G18 - Government Policy and Regulation
- Browse content in G2 - Financial Institutions and Services
- G20 - General
- G21 - Banks; Depository Institutions; Micro Finance Institutions; Mortgages
- G22 - Insurance; Insurance Companies; Actuarial Studies
- G23 - Non-bank Financial Institutions; Financial Instruments; Institutional Investors
- G24 - Investment Banking; Venture Capital; Brokerage; Ratings and Ratings Agencies
- G28 - Government Policy and Regulation
- Browse content in G3 - Corporate Finance and Governance
- G30 - General
- G31 - Capital Budgeting; Fixed Investment and Inventory Studies; Capacity
- G32 - Financing Policy; Financial Risk and Risk Management; Capital and Ownership Structure; Value of Firms; Goodwill
- G33 - Bankruptcy; Liquidation
- G34 - Mergers; Acquisitions; Restructuring; Corporate Governance
- G38 - Government Policy and Regulation
- Browse content in G4 - Behavioral Finance
- G40 - General
- G41 - Role and Effects of Psychological, Emotional, Social, and Cognitive Factors on Decision Making in Financial Markets
- Browse content in H - Public Economics
- Browse content in H0 - General
- H00 - General
- Browse content in H1 - Structure and Scope of Government
- H11 - Structure, Scope, and Performance of Government
- Browse content in H2 - Taxation, Subsidies, and Revenue
- H20 - General
- H21 - Efficiency; Optimal Taxation
- H23 - Externalities; Redistributive Effects; Environmental Taxes and Subsidies
- H24 - Personal Income and Other Nonbusiness Taxes and Subsidies; includes inheritance and gift taxes
- H25 - Business Taxes and Subsidies
- H26 - Tax Evasion and Avoidance
- Browse content in H3 - Fiscal Policies and Behavior of Economic Agents
- H30 - General
- H31 - Household
- Browse content in H4 - Publicly Provided Goods
- H41 - Public Goods
- H42 - Publicly Provided Private Goods
- Browse content in H5 - National Government Expenditures and Related Policies
- H50 - General
- H51 - Government Expenditures and Health
- H52 - Government Expenditures and Education
- H53 - Government Expenditures and Welfare Programs
- H55 - Social Security and Public Pensions
- H56 - National Security and War
- Browse content in H6 - National Budget, Deficit, and Debt
- H60 - General
- H63 - Debt; Debt Management; Sovereign Debt
- Browse content in H7 - State and Local Government; Intergovernmental Relations
- H71 - State and Local Taxation, Subsidies, and Revenue
- H75 - State and Local Government: Health; Education; Welfare; Public Pensions
- Browse content in H8 - Miscellaneous Issues
- H81 - Governmental Loans; Loan Guarantees; Credits; Grants; Bailouts
- Browse content in I - Health, Education, and Welfare
- Browse content in I0 - General
- I00 - General
- Browse content in I1 - Health
- I10 - General
- I11 - Analysis of Health Care Markets
- I12 - Health Behavior
- I13 - Health Insurance, Public and Private
- I14 - Health and Inequality
- I15 - Health and Economic Development
- I18 - Government Policy; Regulation; Public Health
- Browse content in I2 - Education and Research Institutions
- I20 - General
- I21 - Analysis of Education
- I22 - Educational Finance; Financial Aid
- I23 - Higher Education; Research Institutions
- I24 - Education and Inequality
- I25 - Education and Economic Development
- I26 - Returns to Education
- I28 - Government Policy
- Browse content in I3 - Welfare, Well-Being, and Poverty
- I30 - General
- I31 - General Welfare
- I32 - Measurement and Analysis of Poverty
- I38 - Government Policy; Provision and Effects of Welfare Programs
- I39 - Other
- Browse content in J - Labor and Demographic Economics
- Browse content in J0 - General
- J00 - General
- J01 - Labor Economics: General
- J08 - Labor Economics Policies
- Browse content in J1 - Demographic Economics
- J10 - General
- J11 - Demographic Trends, Macroeconomic Effects, and Forecasts
- J12 - Marriage; Marital Dissolution; Family Structure; Domestic Abuse
- J13 - Fertility; Family Planning; Child Care; Children; Youth
- J14 - Economics of the Elderly; Economics of the Handicapped; Non-Labor Market Discrimination
- J15 - Economics of Minorities, Races, Indigenous Peoples, and Immigrants; Non-labor Discrimination
- J16 - Economics of Gender; Non-labor Discrimination
- J17 - Value of Life; Forgone Income
- Browse content in J2 - Demand and Supply of Labor
- J20 - General
- J21 - Labor Force and Employment, Size, and Structure
- J22 - Time Allocation and Labor Supply
- J23 - Labor Demand
- J24 - Human Capital; Skills; Occupational Choice; Labor Productivity
- Browse content in J3 - Wages, Compensation, and Labor Costs
- J30 - General
- J31 - Wage Level and Structure; Wage Differentials
- J32 - Nonwage Labor Costs and Benefits; Retirement Plans; Private Pensions
- J33 - Compensation Packages; Payment Methods
- J38 - Public Policy
- Browse content in J4 - Particular Labor Markets
- J41 - Labor Contracts
- J42 - Monopsony; Segmented Labor Markets
- J44 - Professional Labor Markets; Occupational Licensing
- J47 - Coercive Labor Markets
- Browse content in J5 - Labor-Management Relations, Trade Unions, and Collective Bargaining
- J50 - General
- J52 - Dispute Resolution: Strikes, Arbitration, and Mediation; Collective Bargaining
- Browse content in J6 - Mobility, Unemployment, Vacancies, and Immigrant Workers
- J60 - General
- J61 - Geographic Labor Mobility; Immigrant Workers
- J62 - Job, Occupational, and Intergenerational Mobility
- J63 - Turnover; Vacancies; Layoffs
- J64 - Unemployment: Models, Duration, Incidence, and Job Search
- J65 - Unemployment Insurance; Severance Pay; Plant Closings
- J68 - Public Policy
- Browse content in J7 - Labor Discrimination
- J71 - Discrimination
- Browse content in J8 - Labor Standards: National and International
- J81 - Working Conditions
- J82 - Labor Force Composition
- J83 - Workers' Rights
- Browse content in K - Law and Economics
- Browse content in K0 - General
- K00 - General
- Browse content in K1 - Basic Areas of Law
- K14 - Criminal Law
- Browse content in K3 - Other Substantive Areas of Law
- K31 - Labor Law
- K33 - International Law
- K35 - Personal Bankruptcy Law
- Browse content in K4 - Legal Procedure, the Legal System, and Illegal Behavior
- K40 - General
- K41 - Litigation Process
- K42 - Illegal Behavior and the Enforcement of Law
- Browse content in L - Industrial Organization
- Browse content in L0 - General
- L00 - General
- Browse content in L1 - Market Structure, Firm Strategy, and Market Performance
- L10 - General
- L11 - Production, Pricing, and Market Structure; Size Distribution of Firms
- L12 - Monopoly; Monopolization Strategies
- L13 - Oligopoly and Other Imperfect Markets
- L14 - Transactional Relationships; Contracts and Reputation; Networks
- L15 - Information and Product Quality; Standardization and Compatibility
- Browse content in L2 - Firm Objectives, Organization, and Behavior
- L20 - General
- L22 - Firm Organization and Market Structure
- L23 - Organization of Production
- L25 - Firm Performance: Size, Diversification, and Scope
- Browse content in L3 - Nonprofit Organizations and Public Enterprise
- L31 - Nonprofit Institutions; NGOs; Social Entrepreneurship
- Browse content in L4 - Antitrust Issues and Policies
- L41 - Monopolization; Horizontal Anticompetitive Practices
- L42 - Vertical Restraints; Resale Price Maintenance; Quantity Discounts
- L43 - Legal Monopolies and Regulation or Deregulation
- Browse content in L5 - Regulation and Industrial Policy
- L50 - General
- L51 - Economics of Regulation
- Browse content in L6 - Industry Studies: Manufacturing
- L60 - General
- L62 - Automobiles; Other Transportation Equipment; Related Parts and Equipment
- L63 - Microelectronics; Computers; Communications Equipment
- L65 - Chemicals; Rubber; Drugs; Biotechnology
- Browse content in L7 - Industry Studies: Primary Products and Construction
- L71 - Mining, Extraction, and Refining: Hydrocarbon Fuels
- Browse content in L8 - Industry Studies: Services
- L81 - Retail and Wholesale Trade; e-Commerce
- L82 - Entertainment; Media
- L86 - Information and Internet Services; Computer Software
- Browse content in L9 - Industry Studies: Transportation and Utilities
- L93 - Air Transportation
- L94 - Electric Utilities
- L96 - Telecommunications
- Browse content in M - Business Administration and Business Economics; Marketing; Accounting; Personnel Economics
- Browse content in M0 - General
- M00 - General
- Browse content in M1 - Business Administration
- M11 - Production Management
- M14 - Corporate Culture; Social Responsibility
- Browse content in M2 - Business Economics
- M21 - Business Economics
- Browse content in M3 - Marketing and Advertising
- M31 - Marketing
- M37 - Advertising
- Browse content in M5 - Personnel Economics
- M50 - General
- M51 - Firm Employment Decisions; Promotions
- M52 - Compensation and Compensation Methods and Their Effects
- M54 - Labor Management
- M55 - Labor Contracting Devices
- Browse content in N - Economic History
- Browse content in N1 - Macroeconomics and Monetary Economics; Industrial Structure; Growth; Fluctuations
- N10 - General, International, or Comparative
- N13 - Europe: Pre-1913
- Browse content in N2 - Financial Markets and Institutions
- N20 - General, International, or Comparative
- Browse content in N3 - Labor and Consumers, Demography, Education, Health, Welfare, Income, Wealth, Religion, and Philanthropy
- N30 - General, International, or Comparative
- N31 - U.S.; Canada: Pre-1913
- N32 - U.S.; Canada: 1913-
- N33 - Europe: Pre-1913
- N34 - Europe: 1913-
- Browse content in N4 - Government, War, Law, International Relations, and Regulation
- N42 - U.S.; Canada: 1913-
- N43 - Europe: Pre-1913
- N44 - Europe: 1913-
- N45 - Asia including Middle East
- Browse content in N9 - Regional and Urban History
- N90 - General, International, or Comparative
- N92 - U.S.; Canada: 1913-
- N94 - Europe: 1913-
- Browse content in O - Economic Development, Innovation, Technological Change, and Growth
- Browse content in O1 - Economic Development
- O10 - General
- O11 - Macroeconomic Analyses of Economic Development
- O12 - Microeconomic Analyses of Economic Development
- O13 - Agriculture; Natural Resources; Energy; Environment; Other Primary Products
- O14 - Industrialization; Manufacturing and Service Industries; Choice of Technology
- O15 - Human Resources; Human Development; Income Distribution; Migration
- O16 - Financial Markets; Saving and Capital Investment; Corporate Finance and Governance
- O17 - Formal and Informal Sectors; Shadow Economy; Institutional Arrangements
- O18 - Urban, Rural, Regional, and Transportation Analysis; Housing; Infrastructure
- Browse content in O2 - Development Planning and Policy
- O23 - Fiscal and Monetary Policy in Development
- Browse content in O3 - Innovation; Research and Development; Technological Change; Intellectual Property Rights
- O30 - General
- O31 - Innovation and Invention: Processes and Incentives
- O32 - Management of Technological Innovation and R&D
- O33 - Technological Change: Choices and Consequences; Diffusion Processes
- O34 - Intellectual Property and Intellectual Capital
- O38 - Government Policy
- Browse content in O4 - Economic Growth and Aggregate Productivity
- O40 - General
- O41 - One, Two, and Multisector Growth Models
- O42 - Monetary Growth Models
- O43 - Institutions and Growth
- O44 - Environment and Growth
- O47 - Empirical Studies of Economic Growth; Aggregate Productivity; Cross-Country Output Convergence
- Browse content in O5 - Economywide Country Studies
- O51 - U.S.; Canada
- O55 - Africa
- Browse content in P - Economic Systems
- Browse content in P0 - General
- P00 - General
- Browse content in P1 - Capitalist Systems
- P16 - Political Economy
- Browse content in P2 - Socialist Systems and Transitional Economies
- P26 - Political Economy; Property Rights
- P4 - Other Economic Systems
- Browse content in Q - Agricultural and Natural Resource Economics; Environmental and Ecological Economics
- Browse content in Q1 - Agriculture
- Q15 - Land Ownership and Tenure; Land Reform; Land Use; Irrigation; Agriculture and Environment
- Q16 - R&D; Agricultural Technology; Biofuels; Agricultural Extension Services
- Browse content in Q3 - Nonrenewable Resources and Conservation
- Q33 - Resource Booms
- Browse content in Q4 - Energy
- Q41 - Demand and Supply; Prices
- Q43 - Energy and the Macroeconomy
- Browse content in Q5 - Environmental Economics
- Q51 - Valuation of Environmental Effects
- Q52 - Pollution Control Adoption Costs; Distributional Effects; Employment Effects
- Q53 - Air Pollution; Water Pollution; Noise; Hazardous Waste; Solid Waste; Recycling
- Q54 - Climate; Natural Disasters; Global Warming
- Q55 - Technological Innovation
- Q56 - Environment and Development; Environment and Trade; Sustainability; Environmental Accounts and Accounting; Environmental Equity; Population Growth
- Q58 - Government Policy
- Browse content in R - Urban, Rural, Regional, Real Estate, and Transportation Economics
- Browse content in R1 - General Regional Economics
- R10 - General
- R11 - Regional Economic Activity: Growth, Development, Environmental Issues, and Changes
- R12 - Size and Spatial Distributions of Regional Economic Activity
- R13 - General Equilibrium and Welfare Economic Analysis of Regional Economies
- R15 - Econometric and Input-Output Models; Other Models
- Browse content in R2 - Household Analysis
- R21 - Housing Demand
- R23 - Regional Migration; Regional Labor Markets; Population; Neighborhood Characteristics
- Browse content in R3 - Real Estate Markets, Spatial Production Analysis, and Firm Location
- R31 - Housing Supply and Markets
- R33 - Nonagricultural and Nonresidential Real Estate Markets
- Browse content in R4 - Transportation Economics
- R41 - Transportation: Demand, Supply, and Congestion; Travel Time; Safety and Accidents; Transportation Noise
- R48 - Government Pricing and Policy
- Browse content in R5 - Regional Government Analysis
- R51 - Finance in Urban and Rural Economies
- Browse content in Z - Other Special Topics
- Browse content in Z1 - Cultural Economics; Economic Sociology; Economic Anthropology
- Z10 - General
- Z12 - Religion
- Z13 - Economic Sociology; Economic Anthropology; Social and Economic Stratification
- Author Guidelines
- Submission Site
- Open Access
- About The Review of Economic Studies
- Editorial Board
- Advertising and Corporate Services
- Self-Archiving Policy
- Dispatch Dates
- Journals on Oxford Academic
- Books on Oxford Academic

- < Previous
Quasi-Experimental Shift-Share Research Designs
- Article contents
- Figures & tables
- Supplementary Data
Kirill Borusyak, Peter Hull, Xavier Jaravel, Quasi-Experimental Shift-Share Research Designs, The Review of Economic Studies , Volume 89, Issue 1, January 2022, Pages 181–213, https://doi.org/10.1093/restud/rdab030
- Permissions Icon Permissions
Many studies use shift-share (or “Bartik”) instruments, which average a set of shocks with exposure share weights. We provide a new econometric framework for shift-share instrumental variable (SSIV) regressions in which identification follows from the quasi-random assignment of shocks, while exposure shares are allowed to be endogenous. The framework is motivated by an equivalence result: the orthogonality between a shift-share instrument and an unobserved residual can be represented as the orthogonality between the underlying shocks and a shock-level unobservable. SSIV regression coefficients can similarly be obtained from an equivalent shock-level regression, motivating shock-level conditions for their consistency. We discuss and illustrate several practical insights of this framework in the setting of Autor et al. (2013) , estimating the effect of Chinese import competition on manufacturing employment across U.S. commuting zones.
Personal account
- Sign in with email/username & password
- Get email alerts
- Save searches
- Purchase content
- Activate your purchase/trial code
- Add your ORCID iD
Institutional access
Sign in with a library card.
- Sign in with username/password
- Recommend to your librarian
- Institutional account management
- Get help with access
Access to content on Oxford Academic is often provided through institutional subscriptions and purchases. If you are a member of an institution with an active account, you may be able to access content in one of the following ways:
IP based access
Typically, access is provided across an institutional network to a range of IP addresses. This authentication occurs automatically, and it is not possible to sign out of an IP authenticated account.
Choose this option to get remote access when outside your institution. Shibboleth/Open Athens technology is used to provide single sign-on between your institution’s website and Oxford Academic.
- Click Sign in through your institution.
- Select your institution from the list provided, which will take you to your institution's website to sign in.
- When on the institution site, please use the credentials provided by your institution. Do not use an Oxford Academic personal account.
- Following successful sign in, you will be returned to Oxford Academic.
If your institution is not listed or you cannot sign in to your institution’s website, please contact your librarian or administrator.
Enter your library card number to sign in. If you cannot sign in, please contact your librarian.
Society Members
Society member access to a journal is achieved in one of the following ways:
Sign in through society site
Many societies offer single sign-on between the society website and Oxford Academic. If you see ‘Sign in through society site’ in the sign in pane within a journal:
- Click Sign in through society site.
- When on the society site, please use the credentials provided by that society. Do not use an Oxford Academic personal account.
If you do not have a society account or have forgotten your username or password, please contact your society.
Sign in using a personal account
Some societies use Oxford Academic personal accounts to provide access to their members. See below.
A personal account can be used to get email alerts, save searches, purchase content, and activate subscriptions.
Some societies use Oxford Academic personal accounts to provide access to their members.
Viewing your signed in accounts
Click the account icon in the top right to:
- View your signed in personal account and access account management features.
- View the institutional accounts that are providing access.
Signed in but can't access content
Oxford Academic is home to a wide variety of products. The institutional subscription may not cover the content that you are trying to access. If you believe you should have access to that content, please contact your librarian.
For librarians and administrators, your personal account also provides access to institutional account management. Here you will find options to view and activate subscriptions, manage institutional settings and access options, access usage statistics, and more.
Short-term Access
To purchase short-term access, please sign in to your personal account above.
Don't already have a personal account? Register
Month: | Total Views: |
---|---|
June 2021 | 65 |
July 2021 | 36 |
August 2021 | 105 |
September 2021 | 128 |
October 2021 | 158 |
November 2021 | 153 |
December 2021 | 156 |
January 2022 | 580 |
February 2022 | 461 |
March 2022 | 465 |
April 2022 | 564 |
May 2022 | 480 |
June 2022 | 378 |
July 2022 | 345 |
August 2022 | 308 |
September 2022 | 389 |
October 2022 | 740 |
November 2022 | 484 |
December 2022 | 412 |
January 2023 | 423 |
February 2023 | 431 |
March 2023 | 633 |
April 2023 | 507 |
May 2023 | 465 |
June 2023 | 467 |
July 2023 | 361 |
August 2023 | 478 |
September 2023 | 493 |
October 2023 | 664 |
November 2023 | 559 |
December 2023 | 390 |
January 2024 | 490 |
February 2024 | 667 |
March 2024 | 600 |
April 2024 | 685 |
May 2024 | 608 |
June 2024 | 396 |
July 2024 | 413 |
August 2024 | 364 |
September 2024 | 454 |
Email alerts
Citing articles via.
- Recommend to your Library
- Journals Career Network
Affiliations
- Online ISSN 1467-937X
- Print ISSN 0034-6527
- Copyright © 2024 Review of Economic Studies Ltd
- About Oxford Academic
- Publish journals with us
- University press partners
- What we publish
- New features
- Open access
- Rights and permissions
- Accessibility
- Advertising
- Media enquiries
- Oxford University Press
- Oxford Languages
- University of Oxford
Oxford University Press is a department of the University of Oxford. It furthers the University's objective of excellence in research, scholarship, and education by publishing worldwide
- Copyright © 2024 Oxford University Press
- Cookie settings
- Cookie policy
- Privacy policy
- Legal notice
This Feature Is Available To Subscribers Only
Sign In or Create an Account
This PDF is available to Subscribers Only
For full access to this pdf, sign in to an existing account, or purchase an annual subscription.
Have a language expert improve your writing
Run a free plagiarism check in 10 minutes, generate accurate citations for free.
- Knowledge Base
Methodology
- Types of Research Designs Compared | Guide & Examples
Types of Research Designs Compared | Guide & Examples
Published on June 20, 2019 by Shona McCombes . Revised on June 22, 2023.
When you start planning a research project, developing research questions and creating a research design , you will have to make various decisions about the type of research you want to do.
There are many ways to categorize different types of research. The words you use to describe your research depend on your discipline and field. In general, though, the form your research design takes will be shaped by:
- The type of knowledge you aim to produce
- The type of data you will collect and analyze
- The sampling methods , timescale and location of the research
This article takes a look at some common distinctions made between different types of research and outlines the key differences between them.
Table of contents
Types of research aims, types of research data, types of sampling, timescale, and location, other interesting articles.
The first thing to consider is what kind of knowledge your research aims to contribute.
Type of research | What’s the difference? | What to consider |
---|---|---|
Basic vs. applied | Basic research aims to , while applied research aims to . | Do you want to expand scientific understanding or solve a practical problem? |
vs. | Exploratory research aims to , while explanatory research aims to . | How much is already known about your research problem? Are you conducting initial research on a newly-identified issue, or seeking precise conclusions about an established issue? |
aims to , while aims to . | Is there already some theory on your research problem that you can use to develop , or do you want to propose new theories based on your findings? |
Here's why students love Scribbr's proofreading services
Discover proofreading & editing
The next thing to consider is what type of data you will collect. Each kind of data is associated with a range of specific research methods and procedures.
Type of research | What’s the difference? | What to consider |
---|---|---|
Primary research vs secondary research | Primary data is (e.g., through or ), while secondary data (e.g., in government or scientific publications). | How much data is already available on your topic? Do you want to collect original data or analyze existing data (e.g., through a )? |
, while . | Is your research more concerned with measuring something or interpreting something? You can also create a research design that has elements of both. | |
vs | Descriptive research gathers data , while experimental research . | Do you want to identify characteristics, patterns and or test causal relationships between ? |
Finally, you have to consider three closely related questions: how will you select the subjects or participants of the research? When and how often will you collect data from your subjects? And where will the research take place?
Keep in mind that the methods that you choose bring with them different risk factors and types of research bias . Biases aren’t completely avoidable, but can heavily impact the validity and reliability of your findings if left unchecked.
Type of research | What’s the difference? | What to consider |
---|---|---|
allows you to , while allows you to draw conclusions . | Do you want to produce knowledge that applies to many contexts or detailed knowledge about a specific context (e.g. in a )? | |
vs | Cross-sectional studies , while longitudinal studies . | Is your research question focused on understanding the current situation or tracking changes over time? |
Field research vs laboratory research | Field research takes place in , while laboratory research takes place in . | Do you want to find out how something occurs in the real world or draw firm conclusions about cause and effect? Laboratory experiments have higher but lower . |
Fixed design vs flexible design | In a fixed research design the subjects, timescale and location are begins, while in a flexible design these aspects may . | Do you want to test hypotheses and establish generalizable facts, or explore concepts and develop understanding? For measuring, testing and making generalizations, a fixed research design has higher . |
Choosing between all these different research types is part of the process of creating your research design , which determines exactly how your research will be conducted. But the type of research is only the first step: next, you have to make more concrete decisions about your research methods and the details of the study.
Read more about creating a research design
If you want to know more about statistics , methodology , or research bias , make sure to check out some of our other articles with explanations and examples.
- Normal distribution
- Degrees of freedom
- Null hypothesis
- Discourse analysis
- Control groups
- Mixed methods research
- Non-probability sampling
- Quantitative research
- Ecological validity
Research bias
- Rosenthal effect
- Implicit bias
- Cognitive bias
- Selection bias
- Negativity bias
- Status quo bias
Cite this Scribbr article
If you want to cite this source, you can copy and paste the citation or click the “Cite this Scribbr article” button to automatically add the citation to our free Citation Generator.
McCombes, S. (2023, June 22). Types of Research Designs Compared | Guide & Examples. Scribbr. Retrieved September 27, 2024, from https://www.scribbr.com/methodology/types-of-research/
Is this article helpful?
Shona McCombes
Other students also liked, what is a research design | types, guide & examples, qualitative vs. quantitative research | differences, examples & methods, what is a research methodology | steps & tips, get unlimited documents corrected.
✔ Free APA citation check included ✔ Unlimited document corrections ✔ Specialized in correcting academic texts
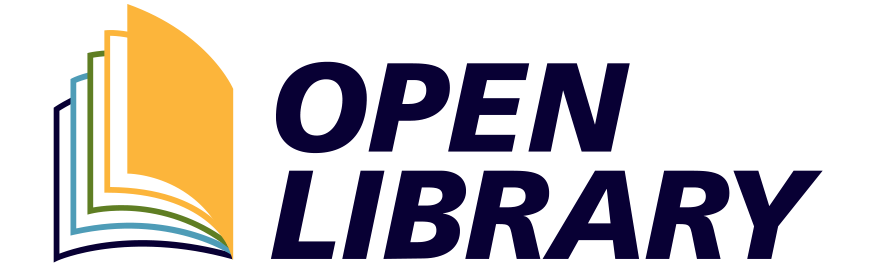
Want to create or adapt books like this? Learn more about how Pressbooks supports open publishing practices.
Quasi-Experimental Research
Learning objectives.
- Explain what quasi-experimental research is and distinguish it clearly from both experimental and correlational research.
- Describe three different types of quasi-experimental research designs (nonequivalent groups, pretest-posttest, and interrupted time series) and identify examples of each one.
The prefix quasi means “resembling.” Thus quasi-experimental research is research that resembles experimental research but is not true experimental research. Although the independent variable is manipulated, participants are not randomly assigned to conditions or orders of conditions (Cook & Campbell, 1979) [1] . Because the independent variable is manipulated before the dependent variable is measured, quasi-experimental research eliminates the directionality problem. But because participants are not randomly assigned—making it likely that there are other differences between conditions—quasi-experimental research does not eliminate the problem of confounding variables. In terms of internal validity, therefore, quasi-experiments are generally somewhere between correlational studies and true experiments.
Quasi-experiments are most likely to be conducted in field settings in which random assignment is difficult or impossible. They are often conducted to evaluate the effectiveness of a treatment—perhaps a type of psychotherapy or an educational intervention. There are many different kinds of quasi-experiments, but we will discuss just a few of the most common ones here.
Nonequivalent Groups Design
Recall that when participants in a between-subjects experiment are randomly assigned to conditions, the resulting groups are likely to be quite similar. In fact, researchers consider them to be equivalent. When participants are not randomly assigned to conditions, however, the resulting groups are likely to be dissimilar in some ways. For this reason, researchers consider them to be nonequivalent. A nonequivalent groups design , then, is a between-subjects design in which participants have not been randomly assigned to conditions.
Imagine, for example, a researcher who wants to evaluate a new method of teaching fractions to third graders. One way would be to conduct a study with a treatment group consisting of one class of third-grade students and a control group consisting of another class of third-grade students. This design would be a nonequivalent groups design because the students are not randomly assigned to classes by the researcher, which means there could be important differences between them. For example, the parents of higher achieving or more motivated students might have been more likely to request that their children be assigned to Ms. Williams’s class. Or the principal might have assigned the “troublemakers” to Mr. Jones’s class because he is a stronger disciplinarian. Of course, the teachers’ styles, and even the classroom environments, might be very different and might cause different levels of achievement or motivation among the students. If at the end of the study there was a difference in the two classes’ knowledge of fractions, it might have been caused by the difference between the teaching methods—but it might have been caused by any of these confounding variables.
Of course, researchers using a nonequivalent groups design can take steps to ensure that their groups are as similar as possible. In the present example, the researcher could try to select two classes at the same school, where the students in the two classes have similar scores on a standardized math test and the teachers are the same sex, are close in age, and have similar teaching styles. Taking such steps would increase the internal validity of the study because it would eliminate some of the most important confounding variables. But without true random assignment of the students to conditions, there remains the possibility of other important confounding variables that the researcher was not able to control.
Pretest-Posttest Design
In a pretest-posttest design , the dependent variable is measured once before the treatment is implemented and once after it is implemented. Imagine, for example, a researcher who is interested in the effectiveness of an antidrug education program on elementary school students’ attitudes toward illegal drugs. The researcher could measure the attitudes of students at a particular elementary school during one week, implement the antidrug program during the next week, and finally, measure their attitudes again the following week. The pretest-posttest design is much like a within-subjects experiment in which each participant is tested first under the control condition and then under the treatment condition. It is unlike a within-subjects experiment, however, in that the order of conditions is not counterbalanced because it typically is not possible for a participant to be tested in the treatment condition first and then in an “untreated” control condition.
If the average posttest score is better than the average pretest score, then it makes sense to conclude that the treatment might be responsible for the improvement. Unfortunately, one often cannot conclude this with a high degree of certainty because there may be other explanations for why the posttest scores are better. One category of alternative explanations goes under the name of history . Other things might have happened between the pretest and the posttest. Perhaps an antidrug program aired on television and many of the students watched it, or perhaps a celebrity died of a drug overdose and many of the students heard about it. Another category of alternative explanations goes under the name of maturation . Participants might have changed between the pretest and the posttest in ways that they were going to anyway because they are growing and learning. If it were a yearlong program, participants might become less impulsive or better reasoners and this might be responsible for the change.
Another alternative explanation for a change in the dependent variable in a pretest-posttest design is regression to the mean . This refers to the statistical fact that an individual who scores extremely on a variable on one occasion will tend to score less extremely on the next occasion. For example, a bowler with a long-term average of 150 who suddenly bowls a 220 will almost certainly score lower in the next game. Her score will “regress” toward her mean score of 150. Regression to the mean can be a problem when participants are selected for further study because of their extreme scores. Imagine, for example, that only students who scored especially low on a test of fractions are given a special training program and then retested. Regression to the mean all but guarantees that their scores will be higher even if the training program has no effect. A closely related concept—and an extremely important one in psychological research—is spontaneous remission . This is the tendency for many medical and psychological problems to improve over time without any form of treatment. The common cold is a good example. If one were to measure symptom severity in 100 common cold sufferers today, give them a bowl of chicken soup every day, and then measure their symptom severity again in a week, they would probably be much improved. This does not mean that the chicken soup was responsible for the improvement, however, because they would have been much improved without any treatment at all. The same is true of many psychological problems. A group of severely depressed people today is likely to be less depressed on average in 6 months. In reviewing the results of several studies of treatments for depression, researchers Michael Posternak and Ivan Miller found that participants in waitlist control conditions improved an average of 10 to 15% before they received any treatment at all (Posternak & Miller, 2001) [2] . Thus one must generally be very cautious about inferring causality from pretest-posttest designs.
Does Psychotherapy Work?
Early studies on the effectiveness of psychotherapy tended to use pretest-posttest designs. In a classic 1952 article, researcher Hans Eysenck summarized the results of 24 such studies showing that about two thirds of patients improved between the pretest and the posttest (Eysenck, 1952) [3] . But Eysenck also compared these results with archival data from state hospital and insurance company records showing that similar patients recovered at about the same rate without receiving psychotherapy. This parallel suggested to Eysenck that the improvement that patients showed in the pretest-posttest studies might be no more than spontaneous remission. Note that Eysenck did not conclude that psychotherapy was ineffective. He merely concluded that there was no evidence that it was, and he wrote of “the necessity of properly planned and executed experimental studies into this important field” (p. 323). You can read the entire article here:
The Effects of Psychotherapy: An Evaluation
Fortunately, many other researchers took up Eysenck’s challenge, and by 1980 hundreds of experiments had been conducted in which participants were randomly assigned to treatment and control conditions, and the results were summarized in a classic book by Mary Lee Smith, Gene Glass, and Thomas Miller (Smith, Glass, & Miller, 1980) [4] . They found that overall psychotherapy was quite effective, with about 80% of treatment participants improving more than the average control participant. Subsequent research has focused more on the conditions under which different types of psychotherapy are more or less effective.
Interrupted Time Series Design
A variant of the pretest-posttest design is the interrupted time-series design . A time series is a set of measurements taken at intervals over a period of time. For example, a manufacturing company might measure its workers’ productivity each week for a year. In an interrupted time series-design, a time series like this one is “interrupted” by a treatment. In one classic example, the treatment was the reduction of the work shifts in a factory from 10 hours to 8 hours (Cook & Campbell, 1979) [5] . Because productivity increased rather quickly after the shortening of the work shifts, and because it remained elevated for many months afterward, the researcher concluded that the shortening of the shifts caused the increase in productivity. Notice that the interrupted time-series design is like a pretest-posttest design in that it includes measurements of the dependent variable both before and after the treatment. It is unlike the pretest-posttest design, however, in that it includes multiple pretest and posttest measurements.
Figure 7.3 shows data from a hypothetical interrupted time-series study. The dependent variable is the number of student absences per week in a research methods course. The treatment is that the instructor begins publicly taking attendance each day so that students know that the instructor is aware of who is present and who is absent. The top panel of Figure 7.3 shows how the data might look if this treatment worked. There is a consistently high number of absences before the treatment, and there is an immediate and sustained drop in absences after the treatment. The bottom panel of Figure 7.3 shows how the data might look if this treatment did not work. On average, the number of absences after the treatment is about the same as the number before. This figure also illustrates an advantage of the interrupted time-series design over a simpler pretest-posttest design. If there had been only one measurement of absences before the treatment at Week 7 and one afterward at Week 8, then it would have looked as though the treatment were responsible for the reduction. The multiple measurements both before and after the treatment suggest that the reduction between Weeks 7 and 8 is nothing more than normal week-to-week variation.
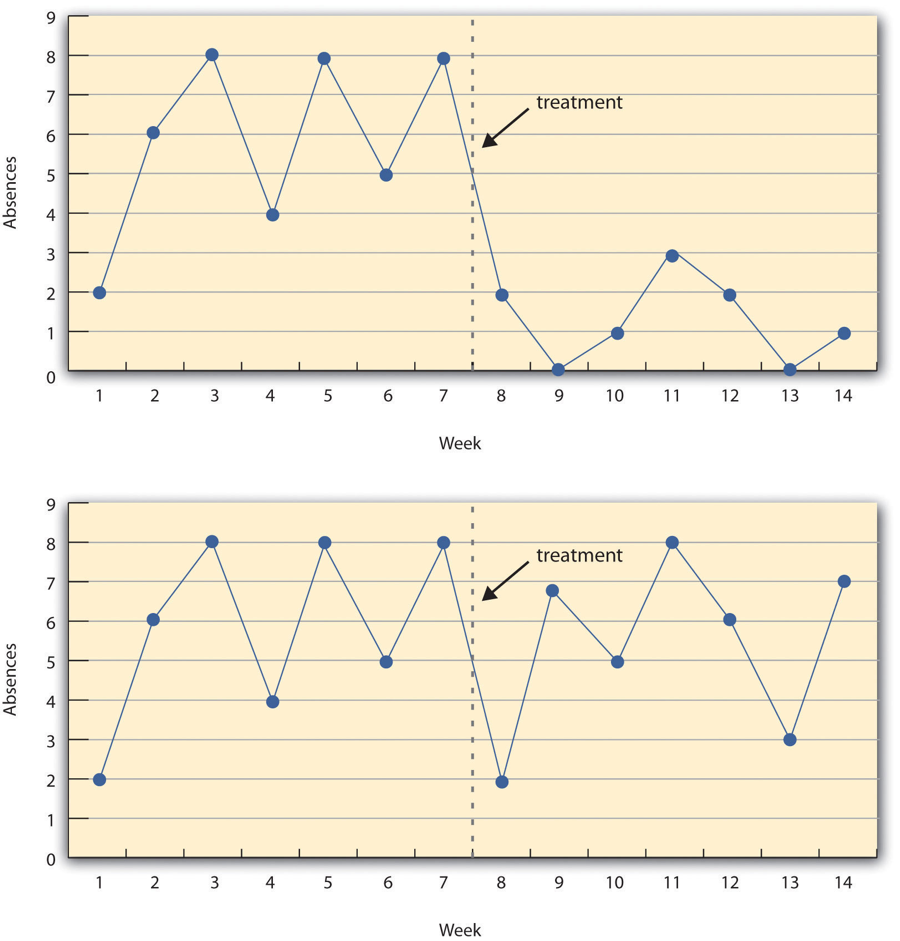
Combination Designs
A type of quasi-experimental design that is generally better than either the nonequivalent groups design or the pretest-posttest design is one that combines elements of both. There is a treatment group that is given a pretest, receives a treatment, and then is given a posttest. But at the same time there is a control group that is given a pretest, does not receive the treatment, and then is given a posttest. The question, then, is not simply whether participants who receive the treatment improve but whether they improve more than participants who do not receive the treatment.
Imagine, for example, that students in one school are given a pretest on their attitudes toward drugs, then are exposed to an antidrug program, and finally are given a posttest. Students in a similar school are given the pretest, not exposed to an antidrug program, and finally are given a posttest. Again, if students in the treatment condition become more negative toward drugs, this change in attitude could be an effect of the treatment, but it could also be a matter of history or maturation. If it really is an effect of the treatment, then students in the treatment condition should become more negative than students in the control condition. But if it is a matter of history (e.g., news of a celebrity drug overdose) or maturation (e.g., improved reasoning), then students in the two conditions would be likely to show similar amounts of change. This type of design does not completely eliminate the possibility of confounding variables, however. Something could occur at one of the schools but not the other (e.g., a student drug overdose), so students at the first school would be affected by it while students at the other school would not.
Finally, if participants in this kind of design are randomly assigned to conditions, it becomes a true experiment rather than a quasi experiment. In fact, it is the kind of experiment that Eysenck called for—and that has now been conducted many times—to demonstrate the effectiveness of psychotherapy.
Key Takeaways
- Quasi-experimental research involves the manipulation of an independent variable without the random assignment of participants to conditions or orders of conditions. Among the important types are nonequivalent groups designs, pretest-posttest, and interrupted time-series designs.
- Quasi-experimental research eliminates the directionality problem because it involves the manipulation of the independent variable. It does not eliminate the problem of confounding variables, however, because it does not involve random assignment to conditions. For these reasons, quasi-experimental research is generally higher in internal validity than correlational studies but lower than true experiments.
- Practice: Imagine that two professors decide to test the effect of giving daily quizzes on student performance in a statistics course. They decide that Professor A will give quizzes but Professor B will not. They will then compare the performance of students in their two sections on a common final exam. List five other variables that might differ between the two sections that could affect the results.
- regression to the mean
- spontaneous remission
- Cook, T. D., & Campbell, D. T. (1979). Quasi-experimentation: Design & analysis issues in field settings . Boston, MA: Houghton Mifflin. ↵
- Posternak, M. A., & Miller, I. (2001). Untreated short-term course of major depression: A meta-analysis of studies using outcomes from studies using wait-list control groups. Journal of Affective Disorders, 66 , 139–146. ↵
- Eysenck, H. J. (1952). The effects of psychotherapy: An evaluation. Journal of Consulting Psychology, 16 , 319–324. ↵
- Smith, M. L., Glass, G. V., & Miller, T. I. (1980). The benefits of psychotherapy . Baltimore, MD: Johns Hopkins University Press. ↵
Research Methods in Psychology Copyright © 2015 by Paul C. Price, Rajiv Jhangiani, & I-Chant A. Chiang is licensed under a Creative Commons Attribution-NonCommercial-ShareAlike 4.0 International License , except where otherwise noted.
Share This Book

COMMENTS
Revised on January 22, 2024. Like a true experiment, a quasi-experimental design aims to establish a cause-and-effect relationship between an independent and dependent variable. However, unlike a true experiment, a quasi-experiment does not rely on random assignment. Instead, subjects are assigned to groups based on non-random criteria.
Quasi-experimental research designs are a type of research design that is similar to experimental designs but doesn't give full control over the independent variable (s) like true experimental designs do. In a quasi-experimental design, the researcher changes or watches an independent variable, but the participants are not put into groups at ...
Quasi-experimental design is a research method that seeks to evaluate the causal relationships between variables, but without the full control over the independent variable (s) that is available in a true experimental design. In a quasi-experimental design, the researcher uses an existing group of participants that is not randomly assigned to ...
Quasi-experimental research is a design that closely resembles experimental research but is different. The term "quasi" means "resembling," so you can think of it as a cousin to actual experiments. In these studies, researchers can manipulate an independent variable — that is, they change one factor to see what effect it has.
The prefix quasi means "resembling." Thus quasi-experimental research is research that resembles experimental research but is not true experimental research. Although the independent variable is manipulated, participants are not randomly assigned to conditions or orders of conditions (Cook & Campbell, 1979). [1] Because the independent variable is manipulated before the dependent variable ...
A quasi-experimental study (also known as a non-randomized pre-post intervention) is a research design in which the independent variable is manipulated, but participants are not randomly assigned to conditions. Commonly used in medical informatics (a field that uses digital information to ensure better patient care), researchers generally use ...
Specifically, we describe four quasi-experimental designs - one-group pretest-posttest designs, non-equivalent group designs, regression discontinuity designs, and interrupted time-series designs - and their statistical analyses in detail. Both simple quasi-experimental designs and embellishments of these simple designs are presented.
Quasi-Experimental Research Designs by Bruce A. Thyer. This pocket guide describes the logic, design, and conduct of the range of quasi-experimental designs, encompassing pre-experiments, quasi-experiments making use of a control or comparison group, and time-series designs. An introductory chapter describes the valuable role these types of ...
Citation. Reichardt, C. S. (2019). Quasi-experimentation: A guide to design and analysis. The Guilford Press. Abstract. This volume explains the logic of both the design of quasi-experiments and the analysis of the data they produce to provide estimates of treatment effects that are as credible as can be obtained given the demanding constraints of research practice.
QEDs test causal hypotheses but, in lieu of fully randomized assignment of the intervention, seek to define a comparison group or time period that reflects the counter-factual (i.e., outcomes if the intervention had not been implemented) ().QEDs seek to identify a comparison group or time period that is as similar as possible to the treatment group or time period in terms of baseline (pre ...
This article discusses four of the strongest quasi-experimental designs for identifying causal effects: regression discontinuity design, instrumental variable design, matching and propensity score designs, and the comparative interrupted time series design. For each design we outline the strategy and assumptions for identifying a causal effect ...
A quasi-experiment is an empirical interventional study used to estimate the causal impact of an intervention on target population without random assignment. Quasi-experimental research shares similarities with the traditional experimental design or randomized controlled trial, but it specifically lacks the element of random assignment to ...
Describe three different types of quasi-experimental research designs (nonequivalent groups, pretest-posttest, and interrupted time series) and identify examples of each one. The prefix quasi means "resembling.". Thus quasi-experimental research is research that resembles experimental research but is not true experimental research.
See why leading organizations rely on MasterClass for learning & development. A quasi-experimental design can be a great option when ethical or practical concerns make true experiments impossible, but the research methodology does have its drawbacks. Learn all the ins and outs of a quasi-experimental design.
Pros of Quasi-Experimental Evaluation Designs. QEDs generally do not involve perceived denial of services, so ethical concerns are less than for RCTs . They have enhanced external validity compared with RCTs (i.e., their findings are likely to apply in many other contexts). QEDs can often rely on available data.
In medical informatics, the quasi-experimental, sometimes called the pre-post intervention, design often is used to evaluate the benefits of specific interventions. The increasing capacity of health care institutions to collect routine clinical data has led to the growing use of quasi-experimental study designs in the field of medical ...
In the past few decades, we have seen a rapid proliferation in the use of quasi-experimental research designs in education research. This trend, stemming in part from the "credibility revolution" in the social sciences, particularly economics, is notable along with the increasing use of randomized controlled trials in the strive toward rigorous causal inference.
Quasi-experimental studies are a type of quantitative research used to investigate the effectiveness of interventions or treatments. These types of studies involve manipulation of the independent variable, yet they lack certain elements of a fully experimental design. ... Check for information about the internal reliability or internal ...
The systematic review is essentially an analysis of the available literature (that is, evidence) and a. judgment of the effectiveness or otherwise of a practice, involving a series of complex steps. JBI takes a. particular view on what counts as evidence and the methods utilised to synthesise those different types of. evidence.
Abstract. Many studies use shift-share (or "Bartik") instruments, which average a set of shocks with exposure share weights. We provide a new econometric framework for shift-share instrumental variable (SSIV) regressions in which identification follows from the quasi-random assignment of shocks, while exposure shares are allowed to be ...
Researchers typically draw upon either experimental or quasi-experimental research designs to determine whether there is a causal relationship between the treatment and the outcome ...
Other interesting articles. If you want to know more about statistics, methodology, or research bias, make sure to check out some of our other articles with explanations and examples. Statistics. Normal distribution. Skewness. Kurtosis. Degrees of freedom. Variance. Null hypothesis.
The prefix quasi means "resembling." Thus quasi-experimental research is research that resembles experimental research but is not true experimental research. Although the independent variable is manipulated, participants are not randomly assigned to conditions or orders of conditions (Cook & Campbell, 1979) [1]. Because the independent variable is manipulated before the dependent variable ...