Have a language expert improve your writing
Run a free plagiarism check in 10 minutes, generate accurate citations for free.
- Knowledge Base
Methodology
- How to Write a Literature Review | Guide, Examples, & Templates

How to Write a Literature Review | Guide, Examples, & Templates
Published on January 2, 2023 by Shona McCombes . Revised on September 11, 2023.
What is a literature review? A literature review is a survey of scholarly sources on a specific topic. It provides an overview of current knowledge, allowing you to identify relevant theories, methods, and gaps in the existing research that you can later apply to your paper, thesis, or dissertation topic .
There are five key steps to writing a literature review:
- Search for relevant literature
- Evaluate sources
- Identify themes, debates, and gaps
- Outline the structure
- Write your literature review
A good literature review doesn’t just summarize sources—it analyzes, synthesizes , and critically evaluates to give a clear picture of the state of knowledge on the subject.
Instantly correct all language mistakes in your text
Upload your document to correct all your mistakes in minutes
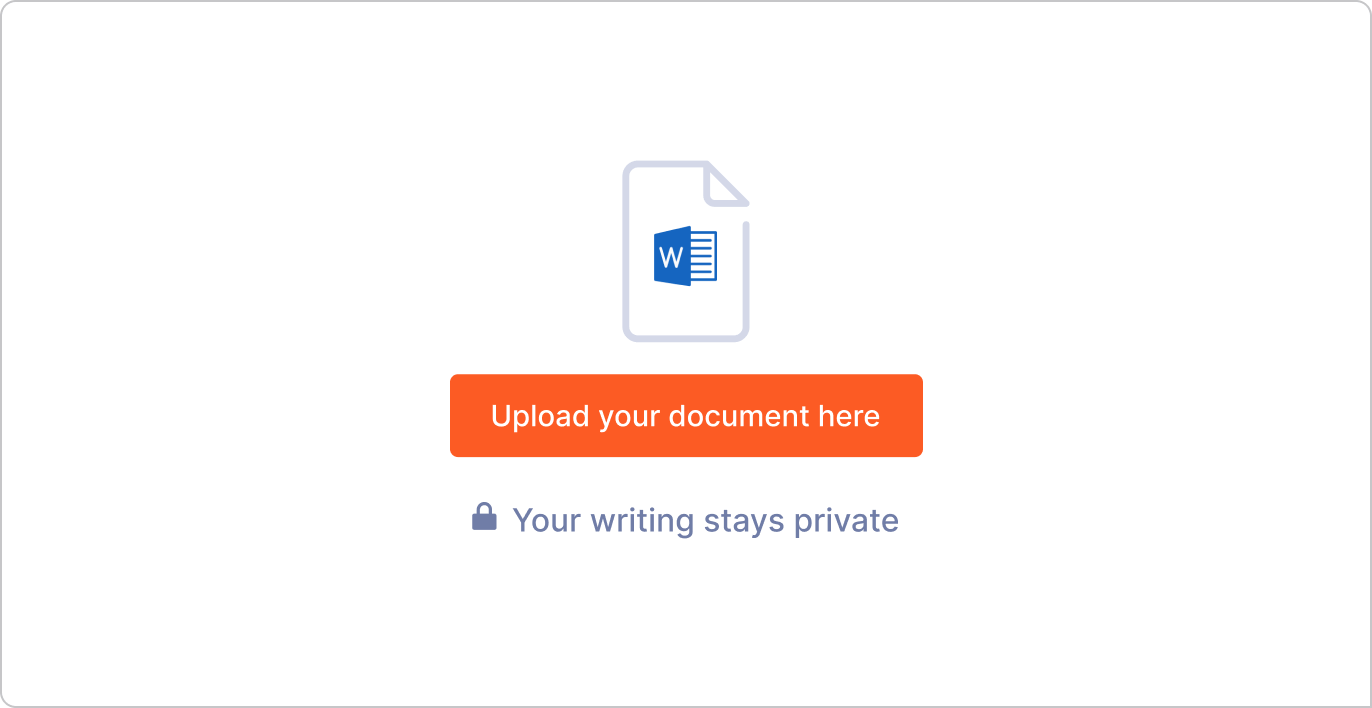
Table of contents
What is the purpose of a literature review, examples of literature reviews, step 1 – search for relevant literature, step 2 – evaluate and select sources, step 3 – identify themes, debates, and gaps, step 4 – outline your literature review’s structure, step 5 – write your literature review, free lecture slides, other interesting articles, frequently asked questions, introduction.
- Quick Run-through
- Step 1 & 2
When you write a thesis , dissertation , or research paper , you will likely have to conduct a literature review to situate your research within existing knowledge. The literature review gives you a chance to:
- Demonstrate your familiarity with the topic and its scholarly context
- Develop a theoretical framework and methodology for your research
- Position your work in relation to other researchers and theorists
- Show how your research addresses a gap or contributes to a debate
- Evaluate the current state of research and demonstrate your knowledge of the scholarly debates around your topic.
Writing literature reviews is a particularly important skill if you want to apply for graduate school or pursue a career in research. We’ve written a step-by-step guide that you can follow below.
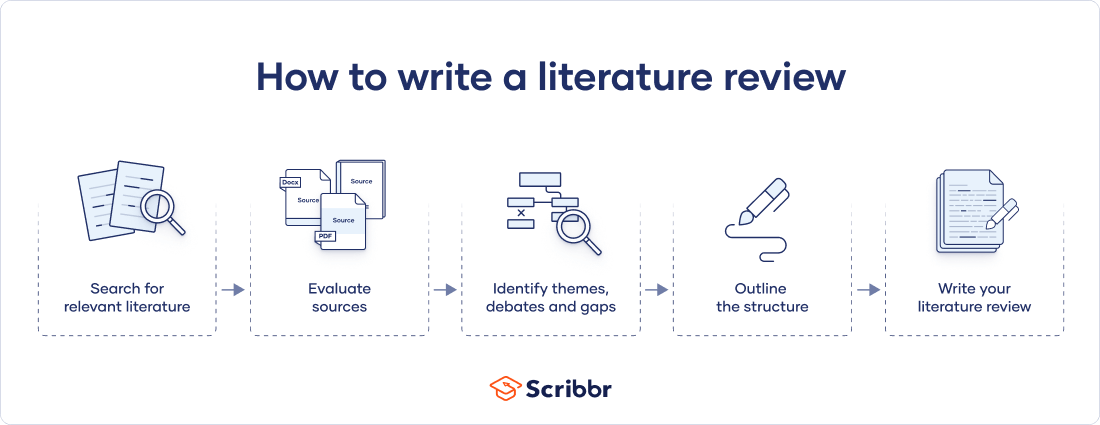
Prevent plagiarism. Run a free check.
Writing literature reviews can be quite challenging! A good starting point could be to look at some examples, depending on what kind of literature review you’d like to write.
- Example literature review #1: “Why Do People Migrate? A Review of the Theoretical Literature” ( Theoretical literature review about the development of economic migration theory from the 1950s to today.)
- Example literature review #2: “Literature review as a research methodology: An overview and guidelines” ( Methodological literature review about interdisciplinary knowledge acquisition and production.)
- Example literature review #3: “The Use of Technology in English Language Learning: A Literature Review” ( Thematic literature review about the effects of technology on language acquisition.)
- Example literature review #4: “Learners’ Listening Comprehension Difficulties in English Language Learning: A Literature Review” ( Chronological literature review about how the concept of listening skills has changed over time.)
You can also check out our templates with literature review examples and sample outlines at the links below.
Download Word doc Download Google doc
Before you begin searching for literature, you need a clearly defined topic .
If you are writing the literature review section of a dissertation or research paper, you will search for literature related to your research problem and questions .
Make a list of keywords
Start by creating a list of keywords related to your research question. Include each of the key concepts or variables you’re interested in, and list any synonyms and related terms. You can add to this list as you discover new keywords in the process of your literature search.
- Social media, Facebook, Instagram, Twitter, Snapchat, TikTok
- Body image, self-perception, self-esteem, mental health
- Generation Z, teenagers, adolescents, youth
Search for relevant sources
Use your keywords to begin searching for sources. Some useful databases to search for journals and articles include:
- Your university’s library catalogue
- Google Scholar
- Project Muse (humanities and social sciences)
- Medline (life sciences and biomedicine)
- EconLit (economics)
- Inspec (physics, engineering and computer science)
You can also use boolean operators to help narrow down your search.
Make sure to read the abstract to find out whether an article is relevant to your question. When you find a useful book or article, you can check the bibliography to find other relevant sources.
You likely won’t be able to read absolutely everything that has been written on your topic, so it will be necessary to evaluate which sources are most relevant to your research question.
For each publication, ask yourself:
- What question or problem is the author addressing?
- What are the key concepts and how are they defined?
- What are the key theories, models, and methods?
- Does the research use established frameworks or take an innovative approach?
- What are the results and conclusions of the study?
- How does the publication relate to other literature in the field? Does it confirm, add to, or challenge established knowledge?
- What are the strengths and weaknesses of the research?
Make sure the sources you use are credible , and make sure you read any landmark studies and major theories in your field of research.
You can use our template to summarize and evaluate sources you’re thinking about using. Click on either button below to download.
Take notes and cite your sources
As you read, you should also begin the writing process. Take notes that you can later incorporate into the text of your literature review.
It is important to keep track of your sources with citations to avoid plagiarism . It can be helpful to make an annotated bibliography , where you compile full citation information and write a paragraph of summary and analysis for each source. This helps you remember what you read and saves time later in the process.
Don't submit your assignments before you do this
The academic proofreading tool has been trained on 1000s of academic texts. Making it the most accurate and reliable proofreading tool for students. Free citation check included.
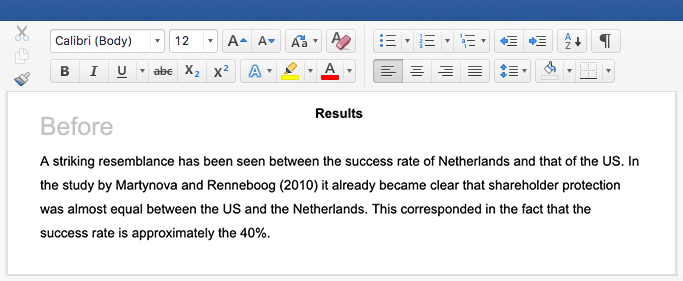
Try for free
To begin organizing your literature review’s argument and structure, be sure you understand the connections and relationships between the sources you’ve read. Based on your reading and notes, you can look for:
- Trends and patterns (in theory, method or results): do certain approaches become more or less popular over time?
- Themes: what questions or concepts recur across the literature?
- Debates, conflicts and contradictions: where do sources disagree?
- Pivotal publications: are there any influential theories or studies that changed the direction of the field?
- Gaps: what is missing from the literature? Are there weaknesses that need to be addressed?
This step will help you work out the structure of your literature review and (if applicable) show how your own research will contribute to existing knowledge.
- Most research has focused on young women.
- There is an increasing interest in the visual aspects of social media.
- But there is still a lack of robust research on highly visual platforms like Instagram and Snapchat—this is a gap that you could address in your own research.
There are various approaches to organizing the body of a literature review. Depending on the length of your literature review, you can combine several of these strategies (for example, your overall structure might be thematic, but each theme is discussed chronologically).
Chronological
The simplest approach is to trace the development of the topic over time. However, if you choose this strategy, be careful to avoid simply listing and summarizing sources in order.
Try to analyze patterns, turning points and key debates that have shaped the direction of the field. Give your interpretation of how and why certain developments occurred.
If you have found some recurring central themes, you can organize your literature review into subsections that address different aspects of the topic.
For example, if you are reviewing literature about inequalities in migrant health outcomes, key themes might include healthcare policy, language barriers, cultural attitudes, legal status, and economic access.
Methodological
If you draw your sources from different disciplines or fields that use a variety of research methods , you might want to compare the results and conclusions that emerge from different approaches. For example:
- Look at what results have emerged in qualitative versus quantitative research
- Discuss how the topic has been approached by empirical versus theoretical scholarship
- Divide the literature into sociological, historical, and cultural sources
Theoretical
A literature review is often the foundation for a theoretical framework . You can use it to discuss various theories, models, and definitions of key concepts.
You might argue for the relevance of a specific theoretical approach, or combine various theoretical concepts to create a framework for your research.
Like any other academic text , your literature review should have an introduction , a main body, and a conclusion . What you include in each depends on the objective of your literature review.
The introduction should clearly establish the focus and purpose of the literature review.
Depending on the length of your literature review, you might want to divide the body into subsections. You can use a subheading for each theme, time period, or methodological approach.
As you write, you can follow these tips:
- Summarize and synthesize: give an overview of the main points of each source and combine them into a coherent whole
- Analyze and interpret: don’t just paraphrase other researchers — add your own interpretations where possible, discussing the significance of findings in relation to the literature as a whole
- Critically evaluate: mention the strengths and weaknesses of your sources
- Write in well-structured paragraphs: use transition words and topic sentences to draw connections, comparisons and contrasts
In the conclusion, you should summarize the key findings you have taken from the literature and emphasize their significance.
When you’ve finished writing and revising your literature review, don’t forget to proofread thoroughly before submitting. Not a language expert? Check out Scribbr’s professional proofreading services !
This article has been adapted into lecture slides that you can use to teach your students about writing a literature review.
Scribbr slides are free to use, customize, and distribute for educational purposes.
Open Google Slides Download PowerPoint
If you want to know more about the research process , methodology , research bias , or statistics , make sure to check out some of our other articles with explanations and examples.
- Sampling methods
- Simple random sampling
- Stratified sampling
- Cluster sampling
- Likert scales
- Reproducibility
Statistics
- Null hypothesis
- Statistical power
- Probability distribution
- Effect size
- Poisson distribution
Research bias
- Optimism bias
- Cognitive bias
- Implicit bias
- Hawthorne effect
- Anchoring bias
- Explicit bias
A literature review is a survey of scholarly sources (such as books, journal articles, and theses) related to a specific topic or research question .
It is often written as part of a thesis, dissertation , or research paper , in order to situate your work in relation to existing knowledge.
There are several reasons to conduct a literature review at the beginning of a research project:
- To familiarize yourself with the current state of knowledge on your topic
- To ensure that you’re not just repeating what others have already done
- To identify gaps in knowledge and unresolved problems that your research can address
- To develop your theoretical framework and methodology
- To provide an overview of the key findings and debates on the topic
Writing the literature review shows your reader how your work relates to existing research and what new insights it will contribute.
The literature review usually comes near the beginning of your thesis or dissertation . After the introduction , it grounds your research in a scholarly field and leads directly to your theoretical framework or methodology .
A literature review is a survey of credible sources on a topic, often used in dissertations , theses, and research papers . Literature reviews give an overview of knowledge on a subject, helping you identify relevant theories and methods, as well as gaps in existing research. Literature reviews are set up similarly to other academic texts , with an introduction , a main body, and a conclusion .
An annotated bibliography is a list of source references that has a short description (called an annotation ) for each of the sources. It is often assigned as part of the research process for a paper .
Cite this Scribbr article
If you want to cite this source, you can copy and paste the citation or click the “Cite this Scribbr article” button to automatically add the citation to our free Citation Generator.
McCombes, S. (2023, September 11). How to Write a Literature Review | Guide, Examples, & Templates. Scribbr. Retrieved September 23, 2024, from https://www.scribbr.com/dissertation/literature-review/
Is this article helpful?
Shona McCombes
Other students also liked, what is a theoretical framework | guide to organizing, what is a research methodology | steps & tips, how to write a research proposal | examples & templates, what is your plagiarism score.
Purdue Online Writing Lab Purdue OWL® College of Liberal Arts
Writing a Literature Review
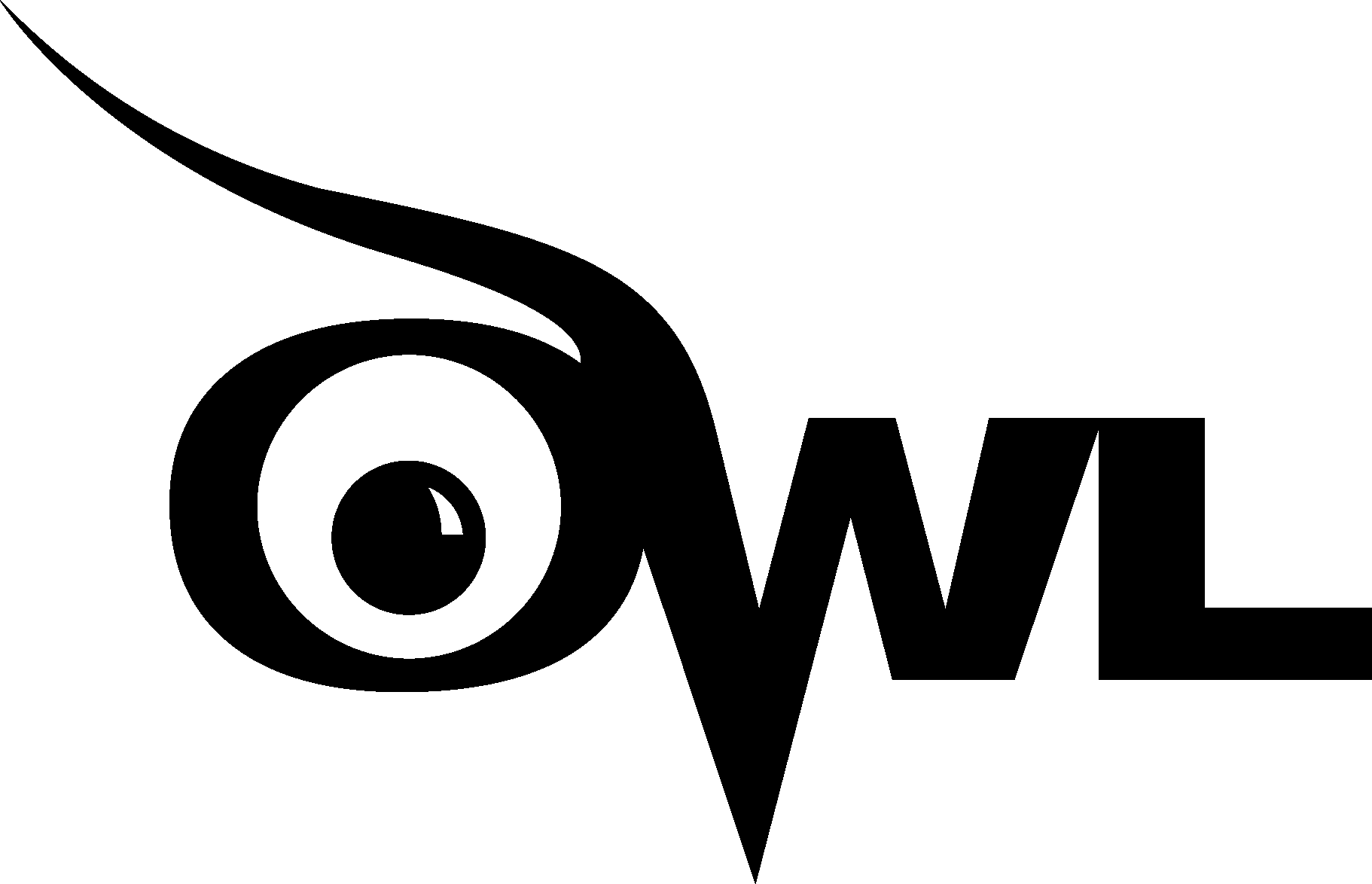
Welcome to the Purdue OWL
This page is brought to you by the OWL at Purdue University. When printing this page, you must include the entire legal notice.
Copyright ©1995-2018 by The Writing Lab & The OWL at Purdue and Purdue University. All rights reserved. This material may not be published, reproduced, broadcast, rewritten, or redistributed without permission. Use of this site constitutes acceptance of our terms and conditions of fair use.
A literature review is a document or section of a document that collects key sources on a topic and discusses those sources in conversation with each other (also called synthesis ). The lit review is an important genre in many disciplines, not just literature (i.e., the study of works of literature such as novels and plays). When we say “literature review” or refer to “the literature,” we are talking about the research ( scholarship ) in a given field. You will often see the terms “the research,” “the scholarship,” and “the literature” used mostly interchangeably.
Where, when, and why would I write a lit review?
There are a number of different situations where you might write a literature review, each with slightly different expectations; different disciplines, too, have field-specific expectations for what a literature review is and does. For instance, in the humanities, authors might include more overt argumentation and interpretation of source material in their literature reviews, whereas in the sciences, authors are more likely to report study designs and results in their literature reviews; these differences reflect these disciplines’ purposes and conventions in scholarship. You should always look at examples from your own discipline and talk to professors or mentors in your field to be sure you understand your discipline’s conventions, for literature reviews as well as for any other genre.
A literature review can be a part of a research paper or scholarly article, usually falling after the introduction and before the research methods sections. In these cases, the lit review just needs to cover scholarship that is important to the issue you are writing about; sometimes it will also cover key sources that informed your research methodology.
Lit reviews can also be standalone pieces, either as assignments in a class or as publications. In a class, a lit review may be assigned to help students familiarize themselves with a topic and with scholarship in their field, get an idea of the other researchers working on the topic they’re interested in, find gaps in existing research in order to propose new projects, and/or develop a theoretical framework and methodology for later research. As a publication, a lit review usually is meant to help make other scholars’ lives easier by collecting and summarizing, synthesizing, and analyzing existing research on a topic. This can be especially helpful for students or scholars getting into a new research area, or for directing an entire community of scholars toward questions that have not yet been answered.
What are the parts of a lit review?
Most lit reviews use a basic introduction-body-conclusion structure; if your lit review is part of a larger paper, the introduction and conclusion pieces may be just a few sentences while you focus most of your attention on the body. If your lit review is a standalone piece, the introduction and conclusion take up more space and give you a place to discuss your goals, research methods, and conclusions separately from where you discuss the literature itself.
Introduction:
- An introductory paragraph that explains what your working topic and thesis is
- A forecast of key topics or texts that will appear in the review
- Potentially, a description of how you found sources and how you analyzed them for inclusion and discussion in the review (more often found in published, standalone literature reviews than in lit review sections in an article or research paper)
- Summarize and synthesize: Give an overview of the main points of each source and combine them into a coherent whole
- Analyze and interpret: Don’t just paraphrase other researchers – add your own interpretations where possible, discussing the significance of findings in relation to the literature as a whole
- Critically Evaluate: Mention the strengths and weaknesses of your sources
- Write in well-structured paragraphs: Use transition words and topic sentence to draw connections, comparisons, and contrasts.
Conclusion:
- Summarize the key findings you have taken from the literature and emphasize their significance
- Connect it back to your primary research question
How should I organize my lit review?
Lit reviews can take many different organizational patterns depending on what you are trying to accomplish with the review. Here are some examples:
- Chronological : The simplest approach is to trace the development of the topic over time, which helps familiarize the audience with the topic (for instance if you are introducing something that is not commonly known in your field). If you choose this strategy, be careful to avoid simply listing and summarizing sources in order. Try to analyze the patterns, turning points, and key debates that have shaped the direction of the field. Give your interpretation of how and why certain developments occurred (as mentioned previously, this may not be appropriate in your discipline — check with a teacher or mentor if you’re unsure).
- Thematic : If you have found some recurring central themes that you will continue working with throughout your piece, you can organize your literature review into subsections that address different aspects of the topic. For example, if you are reviewing literature about women and religion, key themes can include the role of women in churches and the religious attitude towards women.
- Qualitative versus quantitative research
- Empirical versus theoretical scholarship
- Divide the research by sociological, historical, or cultural sources
- Theoretical : In many humanities articles, the literature review is the foundation for the theoretical framework. You can use it to discuss various theories, models, and definitions of key concepts. You can argue for the relevance of a specific theoretical approach or combine various theorical concepts to create a framework for your research.
What are some strategies or tips I can use while writing my lit review?
Any lit review is only as good as the research it discusses; make sure your sources are well-chosen and your research is thorough. Don’t be afraid to do more research if you discover a new thread as you’re writing. More info on the research process is available in our "Conducting Research" resources .
As you’re doing your research, create an annotated bibliography ( see our page on the this type of document ). Much of the information used in an annotated bibliography can be used also in a literature review, so you’ll be not only partially drafting your lit review as you research, but also developing your sense of the larger conversation going on among scholars, professionals, and any other stakeholders in your topic.
Usually you will need to synthesize research rather than just summarizing it. This means drawing connections between sources to create a picture of the scholarly conversation on a topic over time. Many student writers struggle to synthesize because they feel they don’t have anything to add to the scholars they are citing; here are some strategies to help you:
- It often helps to remember that the point of these kinds of syntheses is to show your readers how you understand your research, to help them read the rest of your paper.
- Writing teachers often say synthesis is like hosting a dinner party: imagine all your sources are together in a room, discussing your topic. What are they saying to each other?
- Look at the in-text citations in each paragraph. Are you citing just one source for each paragraph? This usually indicates summary only. When you have multiple sources cited in a paragraph, you are more likely to be synthesizing them (not always, but often
- Read more about synthesis here.
The most interesting literature reviews are often written as arguments (again, as mentioned at the beginning of the page, this is discipline-specific and doesn’t work for all situations). Often, the literature review is where you can establish your research as filling a particular gap or as relevant in a particular way. You have some chance to do this in your introduction in an article, but the literature review section gives a more extended opportunity to establish the conversation in the way you would like your readers to see it. You can choose the intellectual lineage you would like to be part of and whose definitions matter most to your thinking (mostly humanities-specific, but this goes for sciences as well). In addressing these points, you argue for your place in the conversation, which tends to make the lit review more compelling than a simple reporting of other sources.
State-of-the-art literature review methodology: A six-step approach for knowledge synthesis
- Original Article
- Open access
- Published: 05 September 2022
- Volume 11 , pages 281–288, ( 2022 )
Cite this article
You have full access to this open access article
- Erin S. Barry ORCID: orcid.org/0000-0003-0788-7153 1 , 2 ,
- Jerusalem Merkebu ORCID: orcid.org/0000-0003-3707-8920 3 &
- Lara Varpio ORCID: orcid.org/0000-0002-1412-4341 3
33k Accesses
26 Citations
18 Altmetric
Explore all metrics
Introduction
Researchers and practitioners rely on literature reviews to synthesize large bodies of knowledge. Many types of literature reviews have been developed, each targeting a specific purpose. However, these syntheses are hampered if the review type’s paradigmatic roots, methods, and markers of rigor are only vaguely understood. One literature review type whose methodology has yet to be elucidated is the state-of-the-art (SotA) review. If medical educators are to harness SotA reviews to generate knowledge syntheses, we must understand and articulate the paradigmatic roots of, and methods for, conducting SotA reviews.
We reviewed 940 articles published between 2014–2021 labeled as SotA reviews. We (a) identified all SotA methods-related resources, (b) examined the foundational principles and techniques underpinning the reviews, and (c) combined our findings to inductively analyze and articulate the philosophical foundations, process steps, and markers of rigor.
In the 940 articles reviewed, nearly all manuscripts (98%) lacked citations for how to conduct a SotA review. The term “state of the art” was used in 4 different ways. Analysis revealed that SotA articles are grounded in relativism and subjectivism.
This article provides a 6-step approach for conducting SotA reviews. SotA reviews offer an interpretive synthesis that describes: This is where we are now. This is how we got here. This is where we could be going. This chronologically rooted narrative synthesis provides a methodology for reviewing large bodies of literature to explore why and how our current knowledge has developed and to offer new research directions.
Similar content being viewed by others
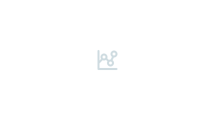
An analysis of current practices in undertaking literature reviews in nursing: findings from a focused mapping review and synthesis
Reviewing the literature, how systematic is systematic.
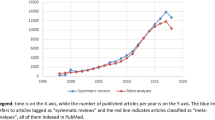
Reading and interpreting reviews for health professionals: a practical review
Explore related subjects.
- Artificial Intelligence
Avoid common mistakes on your manuscript.
Literature reviews play a foundational role in scientific research; they support knowledge advancement by collecting, describing, analyzing, and integrating large bodies of information and data [ 1 , 2 ]. Indeed, as Snyder [ 3 ] argues, all scientific disciplines require literature reviews grounded in a methodology that is accurate and clearly reported. Many types of literature reviews have been developed, each with a unique purpose, distinct methods, and distinguishing characteristics of quality and rigor [ 4 , 5 ].
Each review type offers valuable insights if rigorously conducted [ 3 , 6 ]. Problematically, this is not consistently the case, and the consequences can be dire. Medical education’s policy makers and institutional leaders rely on knowledge syntheses to inform decision making [ 7 ]. Medical education curricula are shaped by these syntheses. Our accreditation standards are informed by these integrations. Our patient care is guided by these knowledge consolidations [ 8 ]. Clearly, it is important for knowledge syntheses to be held to the highest standards of rigor. And yet, that standard is not always maintained. Sometimes scholars fail to meet the review’s specified standards of rigor; other times the markers of rigor have never been explicitly articulated. While we can do little about the former, we can address the latter. One popular literature review type whose methodology has yet to be fully described, vetted, and justified is the state-of-the-art (SotA) review.
While many types of literature reviews amalgamate bodies of literature, SotA reviews offer something unique. By looking across the historical development of a body of knowledge, SotA reviews delves into questions like: Why did our knowledge evolve in this way? What other directions might our investigations have taken? What turning points in our thinking should we revisit to gain new insights? A SotA review—a form of narrative knowledge synthesis [ 5 , 9 ]—acknowledges that history reflects a series of decisions and then asks what different decisions might have been made.
SotA reviews are frequently used in many fields including the biomedical sciences [ 10 , 11 ], medicine [ 12 , 13 , 14 ], and engineering [ 15 , 16 ]. However, SotA reviews are rarely seen in medical education; indeed, a bibliometrics analysis of literature reviews published in 14 core medical education journals between 1999 and 2019 reported only 5 SotA reviews out of the 963 knowledge syntheses identified [ 17 ]. This is not to say that SotA reviews are absent; we suggest that they are often unlabeled. For instance, Schuwirth and van der Vleuten’s article “A history of assessment in medical education” [ 14 ] offers a temporally organized overview of the field’s evolving thinking about assessment. Similarly, McGaghie et al. published a chronologically structured review of simulation-based medical education research that “reviews and critically evaluates historical and contemporary research on simulation-based medical education” [ 18 , p. 50]. SotA reviews certainly have a place in medical education, even if that place is not explicitly signaled.
This lack of labeling is problematic since it conceals the purpose of, and work involved in, the SotA review synthesis. In a SotA review, the author(s) collects and analyzes the historical development of a field’s knowledge about a phenomenon, deconstructs how that understanding evolved, questions why it unfolded in specific ways, and posits new directions for research. Senior medical education scholars use SotA reviews to share their insights based on decades of work on a topic [ 14 , 18 ]; their junior counterparts use them to critique that history and propose new directions [ 19 ]. And yet, SotA reviews are generally not explicitly signaled in medical education. We suggest that at least two factors contribute to this problem. First, it may be that medical education scholars have yet to fully grasp the unique contributions SotA reviews provide. Second, the methodology and methods of SotA reviews are poorly reported making this form of knowledge synthesis appear to lack rigor. Both factors are rooted in the same foundational problem: insufficient clarity about SotA reviews. In this study, we describe SotA review methodology so that medical educators can explicitly use this form of knowledge synthesis to further advance the field.
We developed a four-step research design to meet this goal, illustrated in Fig. 1 .
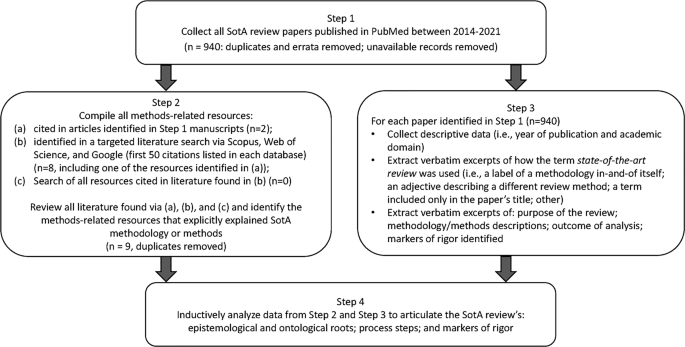
Four-step research design process used for developing a State-of-the-Art literature review methodology
Step 1: Collect SotA articles
To build our initial corpus of articles reporting SotA reviews, we searched PubMed using the strategy (″state of the art review″[ti] OR ″state of the art review*″) and limiting our search to English articles published between 2014 and 2021. We strategically focused on PubMed, which includes MEDLINE, and is considered the National Library of Medicine’s premier database of biomedical literature and indexes health professions education and practice literature [ 20 ]. We limited our search to 2014–2021 to capture modern use of SotA reviews. Of the 960 articles identified, nine were excluded because they were duplicates, erratum, or corrigendum records; full text copies were unavailable for 11 records. All articles identified ( n = 940) constituted the corpus for analysis.
Step 2: Compile all methods-related resources
EB, JM, or LV independently reviewed the 940 full-text articles to identify all references to resources that explained, informed, described, or otherwise supported the methods used for conducting the SotA review. Articles that met our criteria were obtained for analysis.
To ensure comprehensive retrieval, we also searched Scopus and Web of Science. Additionally, to find resources not indexed by these academic databases, we searched Google (see Electronic Supplementary Material [ESM] for the search strategies used for each database). EB also reviewed the first 50 items retrieved from each search looking for additional relevant resources. None were identified. Via these strategies, nine articles were identified and added to the collection of methods-related resources for analysis.
Step 3: Extract data for analysis
In Step 3, we extracted three kinds of information from the 940 articles papers identified in Step 1. First, descriptive data on each article were compiled (i.e., year of publication and the academic domain targeted by the journal). Second, each article was examined and excerpts collected about how the term state-of-the-art review was used (i.e., as a label for a methodology in-and-of itself; as an adjective qualifying another type of literature review; as a term included in the paper’s title only; or in some other way). Finally, we extracted excerpts describing: the purposes and/or aims of the SotA review; the methodology informing and methods processes used to carry out the SotA review; outcomes of analyses; and markers of rigor for the SotA review.
Two researchers (EB and JM) coded 69 articles and an interrater reliability of 94.2% was achieved. Any discrepancies were discussed. Given the high interrater reliability, the two authors split the remaining articles and coded independently.
Step 4: Construct the SotA review methodology
The methods-related resources identified in Step 2 and the data extractions from Step 3 were inductively analyzed by LV and EB to identify statements and research processes that revealed the ontology (i.e., the nature of reality that was reflected) and the epistemology (i.e., the nature of knowledge) underpinning the descriptions of the reviews. These authors studied these data to determine if the synthesis adhered to an objectivist or a subjectivist orientation, and to synthesize the purposes realized in these papers.
To confirm these interpretations, LV and EB compared their ontology, epistemology, and purpose determinations against two expectations commonly required of objectivist synthesis methods (e.g., systematic reviews): an exhaustive search strategy and an appraisal of the quality of the research data. These expectations were considered indicators of a realist ontology and objectivist epistemology [ 21 ] (i.e., that a single correct understanding of the topic can be sought through objective data collection {e.g., systematic reviews [ 22 ]}). Conversely, the inverse of these expectations were considered indicators of a relativist ontology and subjectivist epistemology [ 21 ] (i.e., that no single correct understanding of the topic is available; there are multiple valid understandings that can be generated and so a subjective interpretation of the literature is sought {e.g., narrative reviews [ 9 ]}).
Once these interpretations were confirmed, LV and EB reviewed and consolidated the methods steps described in these data. Markers of rigor were then developed that aligned with the ontology, epistemology, and methods of SotA reviews.
Of the 940 articles identified in Step 1, 98% ( n = 923) lacked citations or other references to resources that explained, informed, or otherwise supported the SotA review process. Of the 17 articles that included supporting information, 16 cited Grant and Booth’s description [ 4 ] consisting of five sentences describing the overall purpose of SotA reviews, three sentences noting perceived strengths, and four sentences articulating perceived weaknesses. This resource provides no guidance on how to conduct a SotA review methodology nor markers of rigor. The one article not referencing Grant and Booth used “an adapted comparative effectiveness research search strategy that was adapted by a health sciences librarian” [ 23 , p. 381]. One website citation was listed in support of this strategy; however, the page was no longer available in summer 2021. We determined that the corpus was uninformed by a cardinal resource or a publicly available methodology description.
In Step 2 we identified nine resources [ 4 , 5 , 24 , 25 , 26 , 27 , 28 ]; none described the methodology and/or processes of carrying out SotA reviews. Nor did they offer explicit descriptions of the ontology or epistemology underpinning SotA reviews. Instead, these resources provided short overview statements (none longer than one paragraph) about the review type [ 4 , 5 , 24 , 25 , 26 , 27 , 28 ]. Thus, we determined that, to date, there are no available methodology papers describing how to conduct a SotA review.
Step 3 revealed that “state of the art” was used in 4 different ways across the 940 articles (see Fig. 2 for the frequency with which each was used). In 71% ( n = 665 articles), the phrase was used only in the title, abstract, and/or purpose statement of the article; the phrase did not appear elsewhere in the paper and no SotA methodology was discussed. Nine percent ( n = 84) used the phrase as an adjective to qualify another literature review type and so relied entirely on the methodology of a different knowledge synthesis approach (e.g., “a state of the art systematic review [ 29 ]”). In 5% ( n = 52) of the articles, the phrase was not used anywhere within the article; instead, “state of the art” was the type of article within a journal. In the remaining 15% ( n = 139), the phrase denoted a specific methodology (see ESM for all methodology articles). Via Step 4’s inductive analysis, the following foundational principles of SotA reviews were developed: (1) the ontology, (2) epistemology, and (3) purpose of SotA reviews.
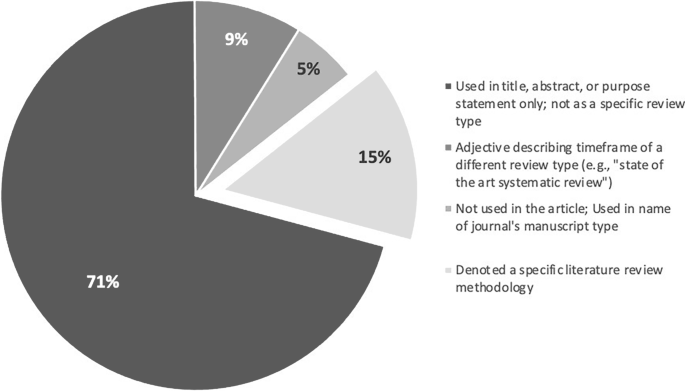
Four ways the term “state of the art” is used in the corpus and how frequently each is used
Ontology of SotA reviews: Relativism
SotA reviews rest on four propositions:
The literature addressing a phenomenon offers multiple perspectives on that topic (i.e., different groups of researchers may hold differing opinions and/or interpretations of data about a phenomenon).
The reality of the phenomenon itself cannot be completely perceived or understood (i.e., due to limitations [e.g., the capabilities of current technologies, a research team’s disciplinary orientation] we can only perceive a limited part of the phenomenon).
The reality of the phenomenon is a subjective and inter-subjective construction (i.e., what we understand about a phenomenon is built by individuals and so their individual subjectivities shape that understanding).
The context in which the review was conducted informs the review (e.g., a SotA review of literature about gender identity and sexual function will be synthesized differently by researchers in the domain of gender studies than by scholars working in sex reassignment surgery).
As these propositions suggest, SotA scholars bring their experiences, expectations, research purposes, and social (including academic) orientations to bear on the synthesis work. In other words, a SotA review synthesizes the literature based on a specific orientation to the topic being addressed. For instance, a SotA review written by senior scholars who are experts in the field of medical education may reflect on the turning points that have shaped the way our field has evolved the modern practices of learner assessment, noting how the nature of the problem of assessment has moved: it was first a measurement problem, then a problem that embraced human judgment but needed assessment expertise, and now a whole system problem that is to be addressed from an integrated—not a reductionist—perspective [ 12 ]. However, if other scholars were to examine this same history from a technological orientation, learner assessment could be framed as historically constricted by the media available through which to conduct assessment, pointing to how artificial intelligence is laying the foundation for the next wave of assessment in medical education [ 30 ].
Given these foundational propositions, SotA reviews are steeped in a relativist ontology—i.e., reality is socially and experientially informed and constructed, and so no single objective truth exists. Researchers’ interpretations reflect their conceptualization of the literature—a conceptualization that could change over time and that could conflict with the understandings of others.
Epistemology of SotA reviews: Subjectivism
SotA reviews embrace subjectivism. The knowledge generated through the review is value-dependent, growing out of the subjective interpretations of the researcher(s) who conducted the synthesis. The SotA review generates an interpretation of the data that is informed by the expertise, experiences, and social contexts of the researcher(s). Furthermore, the knowledge developed through SotA reviews is shaped by the historical point in time when the review was conducted. SotA reviews are thus steeped in the perspective that knowledge is shaped by individuals and their community, and is a synthesis that will change over time.
Purpose of SotA reviews
SotA reviews create a subjectively informed summary of modern thinking about a topic. As a chronologically ordered synthesis, SotA reviews describe the history of turning points in researchers’ understanding of a phenomenon to contextualize a description of modern scientific thinking on the topic. The review presents an argument about how the literature could be interpreted; it is not a definitive statement about how the literature should or must be interpreted. A SotA review explores: the pivotal points shaping the historical development of a topic, the factors that informed those changes in understanding, and the ways of thinking about and studying the topic that could inform the generation of further insights. In other words, the purpose of SotA reviews is to create a three-part argument: This is where we are now in our understanding of this topic. This is how we got here. This is where we could go next.
The SotA methodology
Based on study findings and analyses, we constructed a six-stage SotA review methodology. This six-stage approach is summarized and guiding questions are offered in Tab. 1 .
Stage 1: Determine initial research question and field of inquiry
In Stage 1, the researcher(s) creates an initial description of the topic to be summarized and so must determine what field of knowledge (and/or practice) the search will address. Knowledge developed through the SotA review process is shaped by the context informing it; thus, knowing the domain in which the review will be conducted is part of the review’s foundational work.
Stage 2: Determine timeframe
This stage involves determining the period of time that will be defined as SotA for the topic being summarized. The researcher(s) should engage in a broad-scope overview of the literature, reading across the range of literature available to develop insights into the historical development of knowledge on the topic, including the turning points that shape the current ways of thinking about a topic. Understanding the full body of literature is required to decide the dates or events that demarcate the timeframe of now in the first of the SotA’s three-part argument: where we are now . Stage 2 is complete when the researcher(s) can explicitly justify why a specific year or event is the right moment to mark the beginning of state-of-the-art thinking about the topic being summarized.
Stage 3: Finalize research question(s) to reflect timeframe
Based on the insights developed in Stage 2, the researcher(s) will likely need to revise their initial description of the topic to be summarized. The formal research question(s) framing the SotA review are finalized in Stage 3. The revised description of the topic, the research question(s), and the justification for the timeline start year must be reported in the review article. These are markers of rigor and prerequisites for moving to Stage 4.
Stage 4: Develop search strategy to find relevant articles
In Stage 4, the researcher(s) develops a search strategy to identify the literature that will be included in the SotA review. The researcher(s) needs to determine which literature databases contain articles from the domain of interest. Because the review describes how we got here , the review must include literature that predates the state-of-the-art timeframe, determined in Stage 2, to offer this historical perspective.
Developing the search strategy will be an iterative process of testing and revising the search strategy to enable the researcher(s) to capture the breadth of literature required to meet the SotA review purposes. A librarian should be consulted since their expertise can expedite the search processes and ensure that relevant resources are identified. The search strategy must be reported (e.g., in the manuscript itself or in a supplemental file) so that others may replicate the process if they so choose (e.g., to construct a different SotA review [and possible different interpretations] of the same literature). This too is a marker of rigor for SotA reviews: the search strategies informing the identification of literature must be reported.
Stage 5: Analyses
The literature analysis undertaken will reflect the subjective insights of the researcher(s); however, the foundational premises of inductive research should inform the analysis process. Therefore, the researcher(s) should begin by reading the articles in the corpus to become familiar with the literature. This familiarization work includes: noting similarities across articles, observing ways-of-thinking that have shaped current understandings of the topic, remarking on assumptions underpinning changes in understandings, identifying important decision points in the evolution of understanding, and taking notice of gaps and assumptions in current knowledge.
The researcher(s) can then generate premises for the state-of-the-art understanding of the history that gave rise to modern thinking, of the current body of knowledge, and of potential future directions for research. In this stage of the analysis, the researcher(s) should document the articles that support or contradict their premises, noting any collections of authors or schools of thinking that have dominated the literature, searching for marginalized points of view, and studying the factors that contributed to the dominance of particular ways of thinking. The researcher(s) should also observe historical decision points that could be revisited. Theory can be incorporated at this stage to help shape insights and understandings. It should be highlighted that not all corpus articles will be used in the SotA review; instead, the researcher(s) will sample across the corpus to construct a timeline that represents the seminal moments of the historical development of knowledge.
Next, the researcher(s) should verify the thoroughness and strength of their interpretations. To do this, the researcher(s) can select different articles included in the corpus and examine if those articles reflect the premises the researcher(s) set out. The researcher(s) may also seek out contradictory interpretations in the literature to be sure their summary refutes these positions. The goal of this verification work is not to engage in a triangulation process to ensure objectivity; instead, this process helps the researcher(s) ensure the interpretations made in the SotA review represent the articles being synthesized and respond to the interpretations offered by others. This is another marker of rigor for SotA reviews: the authors should engage in and report how they considered and accounted for differing interpretations of the literature, and how they verified the thoroughness of their interpretations.
Stage 6: Reflexivity
Given the relativist subjectivism of a SotA review, it is important that the manuscript offer insights into the subjectivity of the researcher(s). This reflexivity description should articulate how the subjectivity of the researcher(s) informed interpretations of the data. These reflections will also influence the suggested directions offered in the last part of the SotA three-part argument: where we could go next. This is the last marker of rigor for SotA reviews: researcher reflexivity must be considered and reported.
SotA reviews have much to offer our field since they provide information on the historical progression of medical education’s understanding of a topic, the turning points that guided that understanding, and the potential next directions for future research. Those future directions may question the soundness of turning points and prior decisions, and thereby offer new paths of investigation. Since we were unable to find a description of the SotA review methodology, we inductively developed a description of the methodology—including its paradigmatic roots, the processes to be followed, and the markers of rigor—so that scholars can harness the unique affordances of this type of knowledge synthesis.
Given their chronology- and turning point-based orientation, SotA reviews are inherently different from other types of knowledge synthesis. For example, systematic reviews focus on specific research questions that are narrow in scope [ 32 , 33 ]; in contrast, SotA reviews present a broader historical overview of knowledge development and the decisions that gave rise to our modern understandings. Scoping reviews focus on mapping the present state of knowledge about a phenomenon including, for example, the data that are currently available, the nature of that data, and the gaps in knowledge [ 34 , 35 ]; conversely, SotA reviews offer interpretations of the historical progression of knowledge relating to a phenomenon centered on significant shifts that occurred during that history. SotA reviews focus on the turning points in the history of knowledge development to suggest how different decisions could give rise to new insights. Critical reviews draw on literature outside of the domain of focus to see if external literature can offer new ways of thinking about the phenomenon of interest (e.g., drawing on insights from insects’ swarm intelligence to better understand healthcare team adaptation [ 36 ]). SotA reviews focus on one domain’s body of literature to construct a timeline of knowledge development, demarcating where we are now, demonstrating how this understanding came to be via different turning points, and offering new research directions. Certainly, SotA reviews offer a unique kind of knowledge synthesis.
Our six-stage process for conducting these reviews reflects the subjectivist relativism that underpins the methodology. It aligns with the requirements proposed by others [ 24 , 25 , 26 , 27 ], what has been written about SotA reviews [ 4 , 5 ], and the current body of published SotA reviews. In contrast to existing guidance [ 4 , 5 , 20 , 21 , 22 , 23 ], our description offers a detailed reporting of the ontology, epistemology, and methodology processes for conducting the SotA review.
This explicit methodology description is essential since many academic journals list SotA reviews as an accepted type of literature review. For instance, Educational Research Review [ 24 ], the American Academy of Pediatrics [ 25 ], and Thorax all lists SotA reviews as one of the types of knowledge syntheses they accept [ 27 ]. However, while SotA reviews are valued by academia, guidelines or specific methodology descriptions for researchers to follow when conducting this type of knowledge synthesis are conspicuously absent. If academics in general, and medical education more specifically, are to take advantage of the insights that SotA reviews can offer, we need to rigorously engage in this synthesis work; to do that, we need clear descriptions of the methodology underpinning this review. This article offers such a description. We hope that more medical educators will conduct SotA reviews to generate insights that will contribute to further advancing our field’s research and scholarship.
Cooper HM. Organizing knowledge syntheses: a taxonomy of literature reviews. Knowl Soc. 1988;1:104.
Google Scholar
Badger D, Nursten J, Williams P, Woodward M. Should all literature reviews be systematic? Eval Res Educ. 2000;14:220–30.
Article Google Scholar
Snyder H. Literature review as a research methodology: an overview and guidelines. J Bus Res. 2019;104:333–9.
Grant MJ, Booth A. A typology of reviews: an analysis of 14 review types and associated methodologies. Health Info Libr J. 2009;26:91–108.
Sutton A, Clowes M, Preston L, Booth A. Meeting the review family: exploring review types and associated information retrieval requirements. Health Info Libr J. 2019;36:202–22.
Moher D, Liberati A, Tetzlaff J, Altman DG, Prisma Group. Preferred reporting items for systematic reviews and meta-analyses: the PRISMA statement. PLoS Med. 2009;6:e1000097.
Tricco AC, Langlois E, Straus SE, World Health Organization, Alliance for Health Policy and Systems Research. Rapid reviews to strengthen health policy and systems: a practical guide. Geneva: World Health Organization; 2017.
Jackson R, Feder G. Guidelines for clinical guidelines: a simple, pragmatic strategy for guideline development. Br Med J. 1998;317:427–8.
Greenhalgh T, Thorne S, Malterud K. Time to challenge the spurious hierarchy of systematic over narrative reviews? Eur J Clin Invest. 2018;48:e12931.
Bach QV, Chen WH. Pyrolysis characteristics and kinetics of microalgae via thermogravimetric analysis (TGA): a state-of-the-art review. Bioresour Technol. 2017;246:88–100.
Garofalo C, Milanović V, Cardinali F, Aquilanti L, Clementi F, Osimani A. Current knowledge on the microbiota of edible insects intended for human consumption: a state-of-the-art review. Food Res Int. 2019;125:108527.
Carbone S, Dixon DL, Buckley LF, Abbate A. Glucose-lowering therapies for cardiovascular risk reduction in type 2 diabetes mellitus: state-of-the-art review. Mayo Clin Proc. 2018;93:1629–47.
Hofkens PJ, Verrijcken A, Merveille K, et al. Common pitfalls and tips and tricks to get the most out of your transpulmonary thermodilution device: results of a survey and state-of-the-art review. Anaesthesiol Intensive Ther. 2015;47:89–116.
Schuwirth LW, van der Vleuten CP. A history of assessment in medical education. Adv Health Sci Educ Theory Pract. 2020;25:1045–56.
Arena A, Prete F, Rambaldi E, et al. Nanostructured zirconia-based ceramics and composites in dentistry: a state-of-the-art review. Nanomaterials. 2019;9:1393.
Bahraminasab M, Farahmand F. State of the art review on design and manufacture of hybrid biomedical materials: hip and knee prostheses. Proc Inst Mech Eng H. 2017;231:785–813.
Maggio LA, Costello JA, Norton C, Driessen EW, Artino AR Jr. Knowledge syntheses in medical education: a bibliometric analysis. Perspect Med Educ. 2021;10:79–87.
McGaghie WC, Issenberg SB, Petrusa ER, Scalese RJ. A critical review of simulation-based medical education research: 2003–2009. Med Educ. 2010;44:50–63.
Krishnan DG, Keloth AV, Ubedulla S. Pros and cons of simulation in medical education: a review. Education. 2017;3:84–7.
National Library of Medicine. MEDLINE: overview. 2021. https://www.nlm.nih.gov/medline/medline_overview.html . Accessed 17 Dec 2021.
Bergman E, de Feijter J, Frambach J, et al. AM last page: a guide to research paradigms relevant to medical education. Acad Med. 2012;87:545.
Maggio LA, Samuel A, Stellrecht E. Systematic reviews in medical education. J Grad Med Educ. 2022;14:171–5.
Bandari J, Wessel CB, Jacobs BL. Comparative effectiveness in urology: a state of the art review utilizing a systematic approach. Curr Opin Urol. 2017;27:380–94.
Elsevier. A guide for writing scholarly articles or reviews for the educational research review. 2010. https://www.elsevier.com/__data/promis_misc/edurevReviewPaperWriting.pdf . Accessed 3 Mar 2020.
American Academy of Pediatrics. Pediatrics author guidelines. 2020. https://pediatrics.aappublications.org/page/author-guidelines . Accessed 3 Mar 2020.
Journal of the American College of Cardiology. JACC instructions for authors. 2020. https://www.jacc.org/pb-assets/documents/author-instructions-jacc-1598995793940.pdf . Accessed 3 Mar 2020.
Thorax. Authors. 2020. https://thorax.bmj.com/pages/authors/ . Accessed 3 Mar 2020.
Berven S, Carl A. State of the art review. Spine Deform. 2019;7:381.
Ilardi CR, Chieffi S, Iachini T, Iavarone A. Neuropsychology of posteromedial parietal cortex and conversion factors from mild cognitive impairment to Alzheimer’s disease: systematic search and state-of-the-art review. Aging Clin Exp Res. 2022;34:289–307.
Chan KS, Zary N. Applications and challenges of implementing artificial intelligence in medical education: integrative review. JMIR Med Educ. 2019;5:e13930.
World Health Organization. Framework for action on interprofessional education and collaborative practice. 2010. https://www.who.int/publications/i/item/framework-for-action-on-interprofessional-education-collaborative-practice . Accessed July 1 2021.
Hammersley M. On ‘systematic’ reviews of research literatures: a ‘narrative’ response to Evans & Benefield. Br Educ Res J. 2001;27:543–54.
Chen F, Lui AM, Martinelli SM. A systematic review of the effectiveness of flipped classrooms in medical education. Med Educ. 2017;51:585–97.
Arksey H, O’Malley L. Scoping studies: towards a methodological framework. Int J Soc Res Methodol. 2005;8:19–32.
Matsas B, Goralnick E, Bass M, Barnett E, Nagle B, Sullivan E. Leadership development in US undergraduate medical education: a scoping review of curricular content and competency frameworks. Acad Med. 2022;97:899–908.
Cristancho SM. On collective self-healing and traces: How can swarm intelligence help us think differently about team adaptation? Med Educ. 2021;55:441–7.
Download references
Acknowledgements
We thank Rhonda Allard for her help with the literature review and compiling all available articles. We also want to thank the PME editors who offered excellent development and refinement suggestions that greatly improved this manuscript.
Author information
Authors and affiliations.
Department of Anesthesiology, F. Edward Hébert School of Medicine, Uniformed Services University, Bethesda, MD, USA
Erin S. Barry
School of Health Professions Education (SHE), Maastricht University, Maastricht, The Netherlands
Department of Medicine, F. Edward Hébert School of Medicine, Uniformed Services University, Bethesda, MD, USA
Jerusalem Merkebu & Lara Varpio
You can also search for this author in PubMed Google Scholar
Corresponding author
Correspondence to Erin S. Barry .
Ethics declarations
Conflict of interest.
E.S. Barry, J. Merkebu and L. Varpio declare that they have no competing interests.
Additional information
The opinions and assertions contained in this article are solely those of the authors and are not to be construed as reflecting the views of the Uniformed Services University of the Health Sciences, the Department of Defense, or the Henry M. Jackson Foundation for the Advancement of Military Medicine.
Supplementary Information
40037_2022_725_moesm1_esm.docx.
For information regarding the search strategy to develop the corpus and search strategy for confirming capture of any available State of the Art review methodology descriptions. Additionally, a list of the methodology articles found through the search strategy/corpus is included
Rights and permissions
Open Access This article is licensed under a Creative Commons Attribution 4.0 International License, which permits use, sharing, adaptation, distribution and reproduction in any medium or format, as long as you give appropriate credit to the original author(s) and the source, provide a link to the Creative Commons licence, and indicate if changes were made. The images or other third party material in this article are included in the article’s Creative Commons licence, unless indicated otherwise in a credit line to the material. If material is not included in the article’s Creative Commons licence and your intended use is not permitted by statutory regulation or exceeds the permitted use, you will need to obtain permission directly from the copyright holder. To view a copy of this licence, visit http://creativecommons.org/licenses/by/4.0/ .
Reprints and permissions
About this article
Barry, E.S., Merkebu, J. & Varpio, L. State-of-the-art literature review methodology: A six-step approach for knowledge synthesis. Perspect Med Educ 11 , 281–288 (2022). https://doi.org/10.1007/s40037-022-00725-9
Download citation
Received : 03 December 2021
Revised : 25 July 2022
Accepted : 27 July 2022
Published : 05 September 2022
Issue Date : October 2022
DOI : https://doi.org/10.1007/s40037-022-00725-9
Share this article
Anyone you share the following link with will be able to read this content:
Sorry, a shareable link is not currently available for this article.
Provided by the Springer Nature SharedIt content-sharing initiative
- State-of-the-art literature review
- Literature review
- Literature review methodology
- Find a journal
- Publish with us
- Track your research
Harvey Cushing/John Hay Whitney Medical Library
- Collections
- Research Help
YSN Doctoral Programs: Steps in Conducting a Literature Review
- Biomedical Databases
- Global (Public Health) Databases
- Soc. Sci., History, and Law Databases
- Grey Literature
- Trials Registers
- Data and Statistics
- Public Policy
- Google Tips
- Recommended Books
- Steps in Conducting a Literature Review
What is a literature review?
A literature review is an integrated analysis -- not just a summary-- of scholarly writings and other relevant evidence related directly to your research question. That is, it represents a synthesis of the evidence that provides background information on your topic and shows a association between the evidence and your research question.
A literature review may be a stand alone work or the introduction to a larger research paper, depending on the assignment. Rely heavily on the guidelines your instructor has given you.
Why is it important?
A literature review is important because it:
- Explains the background of research on a topic.
- Demonstrates why a topic is significant to a subject area.
- Discovers relationships between research studies/ideas.
- Identifies major themes, concepts, and researchers on a topic.
- Identifies critical gaps and points of disagreement.
- Discusses further research questions that logically come out of the previous studies.
APA7 Style resources
APA Style Blog - for those harder to find answers
1. Choose a topic. Define your research question.
Your literature review should be guided by your central research question. The literature represents background and research developments related to a specific research question, interpreted and analyzed by you in a synthesized way.
- Make sure your research question is not too broad or too narrow. Is it manageable?
- Begin writing down terms that are related to your question. These will be useful for searches later.
- If you have the opportunity, discuss your topic with your professor and your class mates.
2. Decide on the scope of your review
How many studies do you need to look at? How comprehensive should it be? How many years should it cover?
- This may depend on your assignment. How many sources does the assignment require?
3. Select the databases you will use to conduct your searches.
Make a list of the databases you will search.
Where to find databases:
- use the tabs on this guide
- Find other databases in the Nursing Information Resources web page
- More on the Medical Library web page
- ... and more on the Yale University Library web page
4. Conduct your searches to find the evidence. Keep track of your searches.
- Use the key words in your question, as well as synonyms for those words, as terms in your search. Use the database tutorials for help.
- Save the searches in the databases. This saves time when you want to redo, or modify, the searches. It is also helpful to use as a guide is the searches are not finding any useful results.
- Review the abstracts of research studies carefully. This will save you time.
- Use the bibliographies and references of research studies you find to locate others.
- Check with your professor, or a subject expert in the field, if you are missing any key works in the field.
- Ask your librarian for help at any time.
- Use a citation manager, such as EndNote as the repository for your citations. See the EndNote tutorials for help.
Review the literature
Some questions to help you analyze the research:
- What was the research question of the study you are reviewing? What were the authors trying to discover?
- Was the research funded by a source that could influence the findings?
- What were the research methodologies? Analyze its literature review, the samples and variables used, the results, and the conclusions.
- Does the research seem to be complete? Could it have been conducted more soundly? What further questions does it raise?
- If there are conflicting studies, why do you think that is?
- How are the authors viewed in the field? Has this study been cited? If so, how has it been analyzed?
Tips:
- Review the abstracts carefully.
- Keep careful notes so that you may track your thought processes during the research process.
- Create a matrix of the studies for easy analysis, and synthesis, across all of the studies.
- << Previous: Recommended Books
- Last Updated: Jun 20, 2024 9:08 AM
- URL: https://guides.library.yale.edu/YSNDoctoral

Research Methods and Design
- Action Research
- Case Study Design
Literature Review
- Quantitative Research Methods
- Qualitative Research Methods
- Mixed Methods Study
- Indigenous Research and Ethics This link opens in a new window
- Identifying Empirical Research Articles This link opens in a new window
- Research Ethics and Quality
- Data Literacy
- Get Help with Writing Assignments
A literature review is a discussion of the literature (aka. the "research" or "scholarship") surrounding a certain topic. A good literature review doesn't simply summarize the existing material, but provides thoughtful synthesis and analysis. The purpose of a literature review is to orient your own work within an existing body of knowledge. A literature review may be written as a standalone piece or be included in a larger body of work.
You can read more about literature reviews, what they entail, and how to write one, using the resources below.
Am I the only one struggling to write a literature review?
Dr. Zina O'Leary explains the misconceptions and struggles students often have with writing a literature review. She also provides step-by-step guidance on writing a persuasive literature review.
An Introduction to Literature Reviews
Dr. Eric Jensen, Professor of Sociology at the University of Warwick, and Dr. Charles Laurie, Director of Research at Verisk Maplecroft, explain how to write a literature review, and why researchers need to do so. Literature reviews can be stand-alone research or part of a larger project. They communicate the state of academic knowledge on a given topic, specifically detailing what is still unknown.
This is the first video in a whole series about literature reviews. You can find the rest of the series in our SAGE database, Research Methods:

Videos covering research methods and statistics
Identify Themes and Gaps in Literature (with real examples) | Scribbr
Finding connections between sources is key to organizing the arguments and structure of a good literature review. In this video, you'll learn how to identify themes, debates, and gaps between sources, using examples from real papers.
4 Tips for Writing a Literature Review's Intro, Body, and Conclusion | Scribbr
While each review will be unique in its structure--based on both the existing body of both literature and the overall goals of your own paper, dissertation, or research--this video from Scribbr does a good job simplifying the goals of writing a literature review for those who are new to the process. In this video, you’ll learn what to include in each section, as well as 4 tips for the main body illustrated with an example.
- Literature Review This chapter in SAGE's Encyclopedia of Research Design describes the types of literature reviews and scientific standards for conducting literature reviews.
- UNC Writing Center: Literature Reviews This handout from the Writing Center at UNC will explain what literature reviews are and offer insights into the form and construction of literature reviews in the humanities, social sciences, and sciences.
- Purdue OWL: Writing a Literature Review The overview of literature reviews comes from Purdue's Online Writing Lab. It explains the basic why, what, and how of writing a literature review.
Organizational Tools for Literature Reviews
One of the most daunting aspects of writing a literature review is organizing your research. There are a variety of strategies that you can use to help you in this task. We've highlighted just a few ways writers keep track of all that information! You can use a combination of these tools or come up with your own organizational process. The key is choosing something that works with your own learning style.
Citation Managers
Citation managers are great tools, in general, for organizing research, but can be especially helpful when writing a literature review. You can keep all of your research in one place, take notes, and organize your materials into different folders or categories. Read more about citations managers here:
- Manage Citations & Sources
Concept Mapping
Some writers use concept mapping (sometimes called flow or bubble charts or "mind maps") to help them visualize the ways in which the research they found connects.

There is no right or wrong way to make a concept map. There are a variety of online tools that can help you create a concept map or you can simply put pen to paper. To read more about concept mapping, take a look at the following help guides:
- Using Concept Maps From Williams College's guide, Literature Review: A Self-guided Tutorial
Synthesis Matrix
A synthesis matrix is is a chart you can use to help you organize your research into thematic categories. By organizing your research into a matrix, like the examples below, can help you visualize the ways in which your sources connect.
- Walden University Writing Center: Literature Review Matrix Find a variety of literature review matrix examples and templates from Walden University.
- Writing A Literature Review and Using a Synthesis Matrix An example synthesis matrix created by NC State University Writing and Speaking Tutorial Service Tutors. If you would like a copy of this synthesis matrix in a different format, like a Word document, please ask a librarian. CC-BY-SA 3.0
- << Previous: Case Study Design
- Next: Quantitative Research Methods >>
- Last Updated: May 7, 2024 9:51 AM
CityU Home - CityU Catalog

- USC Libraries
- Research Guides
Organizing Your Social Sciences Research Paper
- 5. The Literature Review
- Purpose of Guide
- Design Flaws to Avoid
- Independent and Dependent Variables
- Glossary of Research Terms
- Reading Research Effectively
- Narrowing a Topic Idea
- Broadening a Topic Idea
- Extending the Timeliness of a Topic Idea
- Academic Writing Style
- Applying Critical Thinking
- Choosing a Title
- Making an Outline
- Paragraph Development
- Research Process Video Series
- Executive Summary
- The C.A.R.S. Model
- Background Information
- The Research Problem/Question
- Theoretical Framework
- Citation Tracking
- Content Alert Services
- Evaluating Sources
- Primary Sources
- Secondary Sources
- Tiertiary Sources
- Scholarly vs. Popular Publications
- Qualitative Methods
- Quantitative Methods
- Insiderness
- Using Non-Textual Elements
- Limitations of the Study
- Common Grammar Mistakes
- Writing Concisely
- Avoiding Plagiarism
- Footnotes or Endnotes?
- Further Readings
- Generative AI and Writing
- USC Libraries Tutorials and Other Guides
- Bibliography
A literature review surveys prior research published in books, scholarly articles, and any other sources relevant to a particular issue, area of research, or theory, and by so doing, provides a description, summary, and critical evaluation of these works in relation to the research problem being investigated. Literature reviews are designed to provide an overview of sources you have used in researching a particular topic and to demonstrate to your readers how your research fits within existing scholarship about the topic.
Fink, Arlene. Conducting Research Literature Reviews: From the Internet to Paper . Fourth edition. Thousand Oaks, CA: SAGE, 2014.
Importance of a Good Literature Review
A literature review may consist of simply a summary of key sources, but in the social sciences, a literature review usually has an organizational pattern and combines both summary and synthesis, often within specific conceptual categories . A summary is a recap of the important information of the source, but a synthesis is a re-organization, or a reshuffling, of that information in a way that informs how you are planning to investigate a research problem. The analytical features of a literature review might:
- Give a new interpretation of old material or combine new with old interpretations,
- Trace the intellectual progression of the field, including major debates,
- Depending on the situation, evaluate the sources and advise the reader on the most pertinent or relevant research, or
- Usually in the conclusion of a literature review, identify where gaps exist in how a problem has been researched to date.
Given this, the purpose of a literature review is to:
- Place each work in the context of its contribution to understanding the research problem being studied.
- Describe the relationship of each work to the others under consideration.
- Identify new ways to interpret prior research.
- Reveal any gaps that exist in the literature.
- Resolve conflicts amongst seemingly contradictory previous studies.
- Identify areas of prior scholarship to prevent duplication of effort.
- Point the way in fulfilling a need for additional research.
- Locate your own research within the context of existing literature [very important].
Fink, Arlene. Conducting Research Literature Reviews: From the Internet to Paper. 2nd ed. Thousand Oaks, CA: Sage, 2005; Hart, Chris. Doing a Literature Review: Releasing the Social Science Research Imagination . Thousand Oaks, CA: Sage Publications, 1998; Jesson, Jill. Doing Your Literature Review: Traditional and Systematic Techniques . Los Angeles, CA: SAGE, 2011; Knopf, Jeffrey W. "Doing a Literature Review." PS: Political Science and Politics 39 (January 2006): 127-132; Ridley, Diana. The Literature Review: A Step-by-Step Guide for Students . 2nd ed. Los Angeles, CA: SAGE, 2012.
Types of Literature Reviews
It is important to think of knowledge in a given field as consisting of three layers. First, there are the primary studies that researchers conduct and publish. Second are the reviews of those studies that summarize and offer new interpretations built from and often extending beyond the primary studies. Third, there are the perceptions, conclusions, opinion, and interpretations that are shared informally among scholars that become part of the body of epistemological traditions within the field.
In composing a literature review, it is important to note that it is often this third layer of knowledge that is cited as "true" even though it often has only a loose relationship to the primary studies and secondary literature reviews. Given this, while literature reviews are designed to provide an overview and synthesis of pertinent sources you have explored, there are a number of approaches you could adopt depending upon the type of analysis underpinning your study.
Argumentative Review This form examines literature selectively in order to support or refute an argument, deeply embedded assumption, or philosophical problem already established in the literature. The purpose is to develop a body of literature that establishes a contrarian viewpoint. Given the value-laden nature of some social science research [e.g., educational reform; immigration control], argumentative approaches to analyzing the literature can be a legitimate and important form of discourse. However, note that they can also introduce problems of bias when they are used to make summary claims of the sort found in systematic reviews [see below].
Integrative Review Considered a form of research that reviews, critiques, and synthesizes representative literature on a topic in an integrated way such that new frameworks and perspectives on the topic are generated. The body of literature includes all studies that address related or identical hypotheses or research problems. A well-done integrative review meets the same standards as primary research in regard to clarity, rigor, and replication. This is the most common form of review in the social sciences.
Historical Review Few things rest in isolation from historical precedent. Historical literature reviews focus on examining research throughout a period of time, often starting with the first time an issue, concept, theory, phenomena emerged in the literature, then tracing its evolution within the scholarship of a discipline. The purpose is to place research in a historical context to show familiarity with state-of-the-art developments and to identify the likely directions for future research.
Methodological Review A review does not always focus on what someone said [findings], but how they came about saying what they say [method of analysis]. Reviewing methods of analysis provides a framework of understanding at different levels [i.e. those of theory, substantive fields, research approaches, and data collection and analysis techniques], how researchers draw upon a wide variety of knowledge ranging from the conceptual level to practical documents for use in fieldwork in the areas of ontological and epistemological consideration, quantitative and qualitative integration, sampling, interviewing, data collection, and data analysis. This approach helps highlight ethical issues which you should be aware of and consider as you go through your own study.
Systematic Review This form consists of an overview of existing evidence pertinent to a clearly formulated research question, which uses pre-specified and standardized methods to identify and critically appraise relevant research, and to collect, report, and analyze data from the studies that are included in the review. The goal is to deliberately document, critically evaluate, and summarize scientifically all of the research about a clearly defined research problem . Typically it focuses on a very specific empirical question, often posed in a cause-and-effect form, such as "To what extent does A contribute to B?" This type of literature review is primarily applied to examining prior research studies in clinical medicine and allied health fields, but it is increasingly being used in the social sciences.
Theoretical Review The purpose of this form is to examine the corpus of theory that has accumulated in regard to an issue, concept, theory, phenomena. The theoretical literature review helps to establish what theories already exist, the relationships between them, to what degree the existing theories have been investigated, and to develop new hypotheses to be tested. Often this form is used to help establish a lack of appropriate theories or reveal that current theories are inadequate for explaining new or emerging research problems. The unit of analysis can focus on a theoretical concept or a whole theory or framework.
NOTE: Most often the literature review will incorporate some combination of types. For example, a review that examines literature supporting or refuting an argument, assumption, or philosophical problem related to the research problem will also need to include writing supported by sources that establish the history of these arguments in the literature.
Baumeister, Roy F. and Mark R. Leary. "Writing Narrative Literature Reviews." Review of General Psychology 1 (September 1997): 311-320; Mark R. Fink, Arlene. Conducting Research Literature Reviews: From the Internet to Paper . 2nd ed. Thousand Oaks, CA: Sage, 2005; Hart, Chris. Doing a Literature Review: Releasing the Social Science Research Imagination . Thousand Oaks, CA: Sage Publications, 1998; Kennedy, Mary M. "Defining a Literature." Educational Researcher 36 (April 2007): 139-147; Petticrew, Mark and Helen Roberts. Systematic Reviews in the Social Sciences: A Practical Guide . Malden, MA: Blackwell Publishers, 2006; Torracro, Richard. "Writing Integrative Literature Reviews: Guidelines and Examples." Human Resource Development Review 4 (September 2005): 356-367; Rocco, Tonette S. and Maria S. Plakhotnik. "Literature Reviews, Conceptual Frameworks, and Theoretical Frameworks: Terms, Functions, and Distinctions." Human Ressource Development Review 8 (March 2008): 120-130; Sutton, Anthea. Systematic Approaches to a Successful Literature Review . Los Angeles, CA: Sage Publications, 2016.
Structure and Writing Style
I. Thinking About Your Literature Review
The structure of a literature review should include the following in support of understanding the research problem :
- An overview of the subject, issue, or theory under consideration, along with the objectives of the literature review,
- Division of works under review into themes or categories [e.g. works that support a particular position, those against, and those offering alternative approaches entirely],
- An explanation of how each work is similar to and how it varies from the others,
- Conclusions as to which pieces are best considered in their argument, are most convincing of their opinions, and make the greatest contribution to the understanding and development of their area of research.
The critical evaluation of each work should consider :
- Provenance -- what are the author's credentials? Are the author's arguments supported by evidence [e.g. primary historical material, case studies, narratives, statistics, recent scientific findings]?
- Methodology -- were the techniques used to identify, gather, and analyze the data appropriate to addressing the research problem? Was the sample size appropriate? Were the results effectively interpreted and reported?
- Objectivity -- is the author's perspective even-handed or prejudicial? Is contrary data considered or is certain pertinent information ignored to prove the author's point?
- Persuasiveness -- which of the author's theses are most convincing or least convincing?
- Validity -- are the author's arguments and conclusions convincing? Does the work ultimately contribute in any significant way to an understanding of the subject?
II. Development of the Literature Review
Four Basic Stages of Writing 1. Problem formulation -- which topic or field is being examined and what are its component issues? 2. Literature search -- finding materials relevant to the subject being explored. 3. Data evaluation -- determining which literature makes a significant contribution to the understanding of the topic. 4. Analysis and interpretation -- discussing the findings and conclusions of pertinent literature.
Consider the following issues before writing the literature review: Clarify If your assignment is not specific about what form your literature review should take, seek clarification from your professor by asking these questions: 1. Roughly how many sources would be appropriate to include? 2. What types of sources should I review (books, journal articles, websites; scholarly versus popular sources)? 3. Should I summarize, synthesize, or critique sources by discussing a common theme or issue? 4. Should I evaluate the sources in any way beyond evaluating how they relate to understanding the research problem? 5. Should I provide subheadings and other background information, such as definitions and/or a history? Find Models Use the exercise of reviewing the literature to examine how authors in your discipline or area of interest have composed their literature review sections. Read them to get a sense of the types of themes you might want to look for in your own research or to identify ways to organize your final review. The bibliography or reference section of sources you've already read, such as required readings in the course syllabus, are also excellent entry points into your own research. Narrow the Topic The narrower your topic, the easier it will be to limit the number of sources you need to read in order to obtain a good survey of relevant resources. Your professor will probably not expect you to read everything that's available about the topic, but you'll make the act of reviewing easier if you first limit scope of the research problem. A good strategy is to begin by searching the USC Libraries Catalog for recent books about the topic and review the table of contents for chapters that focuses on specific issues. You can also review the indexes of books to find references to specific issues that can serve as the focus of your research. For example, a book surveying the history of the Israeli-Palestinian conflict may include a chapter on the role Egypt has played in mediating the conflict, or look in the index for the pages where Egypt is mentioned in the text. Consider Whether Your Sources are Current Some disciplines require that you use information that is as current as possible. This is particularly true in disciplines in medicine and the sciences where research conducted becomes obsolete very quickly as new discoveries are made. However, when writing a review in the social sciences, a survey of the history of the literature may be required. In other words, a complete understanding the research problem requires you to deliberately examine how knowledge and perspectives have changed over time. Sort through other current bibliographies or literature reviews in the field to get a sense of what your discipline expects. You can also use this method to explore what is considered by scholars to be a "hot topic" and what is not.
III. Ways to Organize Your Literature Review
Chronology of Events If your review follows the chronological method, you could write about the materials according to when they were published. This approach should only be followed if a clear path of research building on previous research can be identified and that these trends follow a clear chronological order of development. For example, a literature review that focuses on continuing research about the emergence of German economic power after the fall of the Soviet Union. By Publication Order your sources by publication chronology, then, only if the order demonstrates a more important trend. For instance, you could order a review of literature on environmental studies of brown fields if the progression revealed, for example, a change in the soil collection practices of the researchers who wrote and/or conducted the studies. Thematic [“conceptual categories”] A thematic literature review is the most common approach to summarizing prior research in the social and behavioral sciences. Thematic reviews are organized around a topic or issue, rather than the progression of time, although the progression of time may still be incorporated into a thematic review. For example, a review of the Internet’s impact on American presidential politics could focus on the development of online political satire. While the study focuses on one topic, the Internet’s impact on American presidential politics, it would still be organized chronologically reflecting technological developments in media. The difference in this example between a "chronological" and a "thematic" approach is what is emphasized the most: themes related to the role of the Internet in presidential politics. Note that more authentic thematic reviews tend to break away from chronological order. A review organized in this manner would shift between time periods within each section according to the point being made. Methodological A methodological approach focuses on the methods utilized by the researcher. For the Internet in American presidential politics project, one methodological approach would be to look at cultural differences between the portrayal of American presidents on American, British, and French websites. Or the review might focus on the fundraising impact of the Internet on a particular political party. A methodological scope will influence either the types of documents in the review or the way in which these documents are discussed.
Other Sections of Your Literature Review Once you've decided on the organizational method for your literature review, the sections you need to include in the paper should be easy to figure out because they arise from your organizational strategy. In other words, a chronological review would have subsections for each vital time period; a thematic review would have subtopics based upon factors that relate to the theme or issue. However, sometimes you may need to add additional sections that are necessary for your study, but do not fit in the organizational strategy of the body. What other sections you include in the body is up to you. However, only include what is necessary for the reader to locate your study within the larger scholarship about the research problem.
Here are examples of other sections, usually in the form of a single paragraph, you may need to include depending on the type of review you write:
- Current Situation : Information necessary to understand the current topic or focus of the literature review.
- Sources Used : Describes the methods and resources [e.g., databases] you used to identify the literature you reviewed.
- History : The chronological progression of the field, the research literature, or an idea that is necessary to understand the literature review, if the body of the literature review is not already a chronology.
- Selection Methods : Criteria you used to select (and perhaps exclude) sources in your literature review. For instance, you might explain that your review includes only peer-reviewed [i.e., scholarly] sources.
- Standards : Description of the way in which you present your information.
- Questions for Further Research : What questions about the field has the review sparked? How will you further your research as a result of the review?
IV. Writing Your Literature Review
Once you've settled on how to organize your literature review, you're ready to write each section. When writing your review, keep in mind these issues.
Use Evidence A literature review section is, in this sense, just like any other academic research paper. Your interpretation of the available sources must be backed up with evidence [citations] that demonstrates that what you are saying is valid. Be Selective Select only the most important points in each source to highlight in the review. The type of information you choose to mention should relate directly to the research problem, whether it is thematic, methodological, or chronological. Related items that provide additional information, but that are not key to understanding the research problem, can be included in a list of further readings . Use Quotes Sparingly Some short quotes are appropriate if you want to emphasize a point, or if what an author stated cannot be easily paraphrased. Sometimes you may need to quote certain terminology that was coined by the author, is not common knowledge, or taken directly from the study. Do not use extensive quotes as a substitute for using your own words in reviewing the literature. Summarize and Synthesize Remember to summarize and synthesize your sources within each thematic paragraph as well as throughout the review. Recapitulate important features of a research study, but then synthesize it by rephrasing the study's significance and relating it to your own work and the work of others. Keep Your Own Voice While the literature review presents others' ideas, your voice [the writer's] should remain front and center. For example, weave references to other sources into what you are writing but maintain your own voice by starting and ending the paragraph with your own ideas and wording. Use Caution When Paraphrasing When paraphrasing a source that is not your own, be sure to represent the author's information or opinions accurately and in your own words. Even when paraphrasing an author’s work, you still must provide a citation to that work.
V. Common Mistakes to Avoid
These are the most common mistakes made in reviewing social science research literature.
- Sources in your literature review do not clearly relate to the research problem;
- You do not take sufficient time to define and identify the most relevant sources to use in the literature review related to the research problem;
- Relies exclusively on secondary analytical sources rather than including relevant primary research studies or data;
- Uncritically accepts another researcher's findings and interpretations as valid, rather than examining critically all aspects of the research design and analysis;
- Does not describe the search procedures that were used in identifying the literature to review;
- Reports isolated statistical results rather than synthesizing them in chi-squared or meta-analytic methods; and,
- Only includes research that validates assumptions and does not consider contrary findings and alternative interpretations found in the literature.
Cook, Kathleen E. and Elise Murowchick. “Do Literature Review Skills Transfer from One Course to Another?” Psychology Learning and Teaching 13 (March 2014): 3-11; Fink, Arlene. Conducting Research Literature Reviews: From the Internet to Paper . 2nd ed. Thousand Oaks, CA: Sage, 2005; Hart, Chris. Doing a Literature Review: Releasing the Social Science Research Imagination . Thousand Oaks, CA: Sage Publications, 1998; Jesson, Jill. Doing Your Literature Review: Traditional and Systematic Techniques . London: SAGE, 2011; Literature Review Handout. Online Writing Center. Liberty University; Literature Reviews. The Writing Center. University of North Carolina; Onwuegbuzie, Anthony J. and Rebecca Frels. Seven Steps to a Comprehensive Literature Review: A Multimodal and Cultural Approach . Los Angeles, CA: SAGE, 2016; Ridley, Diana. The Literature Review: A Step-by-Step Guide for Students . 2nd ed. Los Angeles, CA: SAGE, 2012; Randolph, Justus J. “A Guide to Writing the Dissertation Literature Review." Practical Assessment, Research, and Evaluation. vol. 14, June 2009; Sutton, Anthea. Systematic Approaches to a Successful Literature Review . Los Angeles, CA: Sage Publications, 2016; Taylor, Dena. The Literature Review: A Few Tips On Conducting It. University College Writing Centre. University of Toronto; Writing a Literature Review. Academic Skills Centre. University of Canberra.
Writing Tip
Break Out of Your Disciplinary Box!
Thinking interdisciplinarily about a research problem can be a rewarding exercise in applying new ideas, theories, or concepts to an old problem. For example, what might cultural anthropologists say about the continuing conflict in the Middle East? In what ways might geographers view the need for better distribution of social service agencies in large cities than how social workers might study the issue? You don’t want to substitute a thorough review of core research literature in your discipline for studies conducted in other fields of study. However, particularly in the social sciences, thinking about research problems from multiple vectors is a key strategy for finding new solutions to a problem or gaining a new perspective. Consult with a librarian about identifying research databases in other disciplines; almost every field of study has at least one comprehensive database devoted to indexing its research literature.
Frodeman, Robert. The Oxford Handbook of Interdisciplinarity . New York: Oxford University Press, 2010.
Another Writing Tip
Don't Just Review for Content!
While conducting a review of the literature, maximize the time you devote to writing this part of your paper by thinking broadly about what you should be looking for and evaluating. Review not just what scholars are saying, but how are they saying it. Some questions to ask:
- How are they organizing their ideas?
- What methods have they used to study the problem?
- What theories have been used to explain, predict, or understand their research problem?
- What sources have they cited to support their conclusions?
- How have they used non-textual elements [e.g., charts, graphs, figures, etc.] to illustrate key points?
When you begin to write your literature review section, you'll be glad you dug deeper into how the research was designed and constructed because it establishes a means for developing more substantial analysis and interpretation of the research problem.
Hart, Chris. Doing a Literature Review: Releasing the Social Science Research Imagination . Thousand Oaks, CA: Sage Publications, 1 998.
Yet Another Writing Tip
When Do I Know I Can Stop Looking and Move On?
Here are several strategies you can utilize to assess whether you've thoroughly reviewed the literature:
- Look for repeating patterns in the research findings . If the same thing is being said, just by different people, then this likely demonstrates that the research problem has hit a conceptual dead end. At this point consider: Does your study extend current research? Does it forge a new path? Or, does is merely add more of the same thing being said?
- Look at sources the authors cite to in their work . If you begin to see the same researchers cited again and again, then this is often an indication that no new ideas have been generated to address the research problem.
- Search Google Scholar to identify who has subsequently cited leading scholars already identified in your literature review [see next sub-tab]. This is called citation tracking and there are a number of sources that can help you identify who has cited whom, particularly scholars from outside of your discipline. Here again, if the same authors are being cited again and again, this may indicate no new literature has been written on the topic.
Onwuegbuzie, Anthony J. and Rebecca Frels. Seven Steps to a Comprehensive Literature Review: A Multimodal and Cultural Approach . Los Angeles, CA: Sage, 2016; Sutton, Anthea. Systematic Approaches to a Successful Literature Review . Los Angeles, CA: Sage Publications, 2016.
- << Previous: Theoretical Framework
- Next: Citation Tracking >>
- Last Updated: Sep 27, 2024 1:09 PM
- URL: https://libguides.usc.edu/writingguide
- UConn Library
- Literature Review: The What, Why and How-to Guide
- Introduction
Literature Review: The What, Why and How-to Guide — Introduction
- Getting Started
- How to Pick a Topic
- Strategies to Find Sources
- Evaluating Sources & Lit. Reviews
- Tips for Writing Literature Reviews
- Writing Literature Review: Useful Sites
- Citation Resources
- Other Academic Writings
What are Literature Reviews?
So, what is a literature review? "A literature review is an account of what has been published on a topic by accredited scholars and researchers. In writing the literature review, your purpose is to convey to your reader what knowledge and ideas have been established on a topic, and what their strengths and weaknesses are. As a piece of writing, the literature review must be defined by a guiding concept (e.g., your research objective, the problem or issue you are discussing, or your argumentative thesis). It is not just a descriptive list of the material available, or a set of summaries." Taylor, D. The literature review: A few tips on conducting it . University of Toronto Health Sciences Writing Centre.
Goals of Literature Reviews
What are the goals of creating a Literature Review? A literature could be written to accomplish different aims:
- To develop a theory or evaluate an existing theory
- To summarize the historical or existing state of a research topic
- Identify a problem in a field of research
Baumeister, R. F., & Leary, M. R. (1997). Writing narrative literature reviews . Review of General Psychology , 1 (3), 311-320.
What kinds of sources require a Literature Review?
- A research paper assigned in a course
- A thesis or dissertation
- A grant proposal
- An article intended for publication in a journal
All these instances require you to collect what has been written about your research topic so that you can demonstrate how your own research sheds new light on the topic.
Types of Literature Reviews
What kinds of literature reviews are written?
Narrative review: The purpose of this type of review is to describe the current state of the research on a specific topic/research and to offer a critical analysis of the literature reviewed. Studies are grouped by research/theoretical categories, and themes and trends, strengths and weakness, and gaps are identified. The review ends with a conclusion section which summarizes the findings regarding the state of the research of the specific study, the gaps identify and if applicable, explains how the author's research will address gaps identify in the review and expand the knowledge on the topic reviewed.
- Example : Predictors and Outcomes of U.S. Quality Maternity Leave: A Review and Conceptual Framework: 10.1177/08948453211037398
Systematic review : "The authors of a systematic review use a specific procedure to search the research literature, select the studies to include in their review, and critically evaluate the studies they find." (p. 139). Nelson, L. K. (2013). Research in Communication Sciences and Disorders . Plural Publishing.
- Example : The effect of leave policies on increasing fertility: a systematic review: 10.1057/s41599-022-01270-w
Meta-analysis : "Meta-analysis is a method of reviewing research findings in a quantitative fashion by transforming the data from individual studies into what is called an effect size and then pooling and analyzing this information. The basic goal in meta-analysis is to explain why different outcomes have occurred in different studies." (p. 197). Roberts, M. C., & Ilardi, S. S. (2003). Handbook of Research Methods in Clinical Psychology . Blackwell Publishing.
- Example : Employment Instability and Fertility in Europe: A Meta-Analysis: 10.1215/00703370-9164737
Meta-synthesis : "Qualitative meta-synthesis is a type of qualitative study that uses as data the findings from other qualitative studies linked by the same or related topic." (p.312). Zimmer, L. (2006). Qualitative meta-synthesis: A question of dialoguing with texts . Journal of Advanced Nursing , 53 (3), 311-318.
- Example : Women’s perspectives on career successes and barriers: A qualitative meta-synthesis: 10.1177/05390184221113735
Literature Reviews in the Health Sciences
- UConn Health subject guide on systematic reviews Explanation of the different review types used in health sciences literature as well as tools to help you find the right review type
- << Previous: Getting Started
- Next: How to Pick a Topic >>
- Last Updated: Sep 21, 2022 2:16 PM
- URL: https://guides.lib.uconn.edu/literaturereview

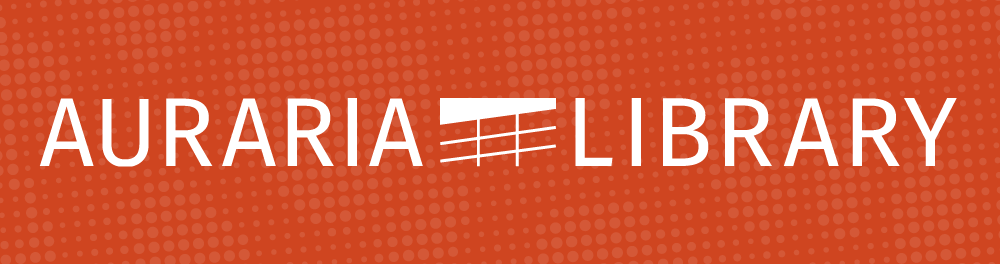
Research Methods: Literature Reviews
- Annotated Bibliographies
- Literature Reviews
- Scoping Reviews
- Systematic Reviews
- Scholarship of Teaching and Learning
- Persuasive Arguments
- Subject Specific Methodology
A literature review involves researching, reading, analyzing, evaluating, and summarizing scholarly literature (typically journals and articles) about a specific topic. The results of a literature review may be an entire report or article OR may be part of a article, thesis, dissertation, or grant proposal. A literature review helps the author learn about the history and nature of their topic, and identify research gaps and problems.
Steps & Elements
Problem formulation
- Determine your topic and its components by asking a question
- Research: locate literature related to your topic to identify the gap(s) that can be addressed
- Read: read the articles or other sources of information
- Analyze: assess the findings for relevancy
- Evaluating: determine how the article are relevant to your research and what are the key findings
- Synthesis: write about the key findings and how it is relevant to your research
Elements of a Literature Review
- Summarize subject, issue or theory under consideration, along with objectives of the review
- Divide works under review into categories (e.g. those in support of a particular position, those against, those offering alternative theories entirely)
- Explain how each work is similar to and how it varies from the others
- Conclude which pieces are best considered in their argument, are most convincing of their opinions, and make the greatest contribution to the understanding and development of an area of research
Writing a Literature Review Resources
- How to Write a Literature Review From the Wesleyan University Library
- Write a Literature Review From the University of California Santa Cruz Library. A Brief overview of a literature review, includes a list of stages for writing a lit review.
- Literature Reviews From the University of North Carolina Writing Center. Detailed information about writing a literature review.
- Undertaking a literature review: a step-by-step approach Cronin, P., Ryan, F., & Coughan, M. (2008). Undertaking a literature review: A step-by-step approach. British Journal of Nursing, 17(1), p.38-43
Literature Review Tutorial
- << Previous: Annotated Bibliographies
- Next: Scoping Reviews >>
- Last Updated: Jul 8, 2024 3:13 PM
- URL: https://guides.auraria.edu/researchmethods
1100 Lawrence Street Denver, CO 80204 303-315-7700 Ask Us Directions
Research Methods
- Getting Started
- Literature Review Research
- Research Design
- Research Design By Discipline
- SAGE Research Methods
- Teaching with SAGE Research Methods
Literature Review
- What is a Literature Review?
- What is NOT a Literature Review?
- Purposes of a Literature Review
- Types of Literature Reviews
- Literature Reviews vs. Systematic Reviews
- Systematic vs. Meta-Analysis
Literature Review is a comprehensive survey of the works published in a particular field of study or line of research, usually over a specific period of time, in the form of an in-depth, critical bibliographic essay or annotated list in which attention is drawn to the most significant works.
Also, we can define a literature review as the collected body of scholarly works related to a topic:
- Summarizes and analyzes previous research relevant to a topic
- Includes scholarly books and articles published in academic journals
- Can be an specific scholarly paper or a section in a research paper
The objective of a Literature Review is to find previous published scholarly works relevant to an specific topic
- Help gather ideas or information
- Keep up to date in current trends and findings
- Help develop new questions
A literature review is important because it:
- Explains the background of research on a topic.
- Demonstrates why a topic is significant to a subject area.
- Helps focus your own research questions or problems
- Discovers relationships between research studies/ideas.
- Suggests unexplored ideas or populations
- Identifies major themes, concepts, and researchers on a topic.
- Tests assumptions; may help counter preconceived ideas and remove unconscious bias.
- Identifies critical gaps, points of disagreement, or potentially flawed methodology or theoretical approaches.
- Indicates potential directions for future research.
All content in this section is from Literature Review Research from Old Dominion University
Keep in mind the following, a literature review is NOT:
Not an essay
Not an annotated bibliography in which you summarize each article that you have reviewed. A literature review goes beyond basic summarizing to focus on the critical analysis of the reviewed works and their relationship to your research question.
Not a research paper where you select resources to support one side of an issue versus another. A lit review should explain and consider all sides of an argument in order to avoid bias, and areas of agreement and disagreement should be highlighted.
A literature review serves several purposes. For example, it
- provides thorough knowledge of previous studies; introduces seminal works.
- helps focus one’s own research topic.
- identifies a conceptual framework for one’s own research questions or problems; indicates potential directions for future research.
- suggests previously unused or underused methodologies, designs, quantitative and qualitative strategies.
- identifies gaps in previous studies; identifies flawed methodologies and/or theoretical approaches; avoids replication of mistakes.
- helps the researcher avoid repetition of earlier research.
- suggests unexplored populations.
- determines whether past studies agree or disagree; identifies controversy in the literature.
- tests assumptions; may help counter preconceived ideas and remove unconscious bias.
As Kennedy (2007) notes*, it is important to think of knowledge in a given field as consisting of three layers. First, there are the primary studies that researchers conduct and publish. Second are the reviews of those studies that summarize and offer new interpretations built from and often extending beyond the original studies. Third, there are the perceptions, conclusions, opinion, and interpretations that are shared informally that become part of the lore of field. In composing a literature review, it is important to note that it is often this third layer of knowledge that is cited as "true" even though it often has only a loose relationship to the primary studies and secondary literature reviews.
Given this, while literature reviews are designed to provide an overview and synthesis of pertinent sources you have explored, there are several approaches to how they can be done, depending upon the type of analysis underpinning your study. Listed below are definitions of types of literature reviews:
Argumentative Review This form examines literature selectively in order to support or refute an argument, deeply imbedded assumption, or philosophical problem already established in the literature. The purpose is to develop a body of literature that establishes a contrarian viewpoint. Given the value-laden nature of some social science research [e.g., educational reform; immigration control], argumentative approaches to analyzing the literature can be a legitimate and important form of discourse. However, note that they can also introduce problems of bias when they are used to to make summary claims of the sort found in systematic reviews.
Integrative Review Considered a form of research that reviews, critiques, and synthesizes representative literature on a topic in an integrated way such that new frameworks and perspectives on the topic are generated. The body of literature includes all studies that address related or identical hypotheses. A well-done integrative review meets the same standards as primary research in regard to clarity, rigor, and replication.
Historical Review Few things rest in isolation from historical precedent. Historical reviews are focused on examining research throughout a period of time, often starting with the first time an issue, concept, theory, phenomena emerged in the literature, then tracing its evolution within the scholarship of a discipline. The purpose is to place research in a historical context to show familiarity with state-of-the-art developments and to identify the likely directions for future research.
Methodological Review A review does not always focus on what someone said [content], but how they said it [method of analysis]. This approach provides a framework of understanding at different levels (i.e. those of theory, substantive fields, research approaches and data collection and analysis techniques), enables researchers to draw on a wide variety of knowledge ranging from the conceptual level to practical documents for use in fieldwork in the areas of ontological and epistemological consideration, quantitative and qualitative integration, sampling, interviewing, data collection and data analysis, and helps highlight many ethical issues which we should be aware of and consider as we go through our study.
Systematic Review This form consists of an overview of existing evidence pertinent to a clearly formulated research question, which uses pre-specified and standardized methods to identify and critically appraise relevant research, and to collect, report, and analyse data from the studies that are included in the review. Typically it focuses on a very specific empirical question, often posed in a cause-and-effect form, such as "To what extent does A contribute to B?"
Theoretical Review The purpose of this form is to concretely examine the corpus of theory that has accumulated in regard to an issue, concept, theory, phenomena. The theoretical literature review help establish what theories already exist, the relationships between them, to what degree the existing theories have been investigated, and to develop new hypotheses to be tested. Often this form is used to help establish a lack of appropriate theories or reveal that current theories are inadequate for explaining new or emerging research problems. The unit of analysis can focus on a theoretical concept or a whole theory or framework.
* Kennedy, Mary M. "Defining a Literature." Educational Researcher 36 (April 2007): 139-147.
All content in this section is from The Literature Review created by Dr. Robert Larabee USC
Robinson, P. and Lowe, J. (2015), Literature reviews vs systematic reviews. Australian and New Zealand Journal of Public Health, 39: 103-103. doi: 10.1111/1753-6405.12393
What's in the name? The difference between a Systematic Review and a Literature Review, and why it matters . By Lynn Kysh from University of Southern California
Systematic review or meta-analysis?
A systematic review answers a defined research question by collecting and summarizing all empirical evidence that fits pre-specified eligibility criteria.
A meta-analysis is the use of statistical methods to summarize the results of these studies.
Systematic reviews, just like other research articles, can be of varying quality. They are a significant piece of work (the Centre for Reviews and Dissemination at York estimates that a team will take 9-24 months), and to be useful to other researchers and practitioners they should have:
- clearly stated objectives with pre-defined eligibility criteria for studies
- explicit, reproducible methodology
- a systematic search that attempts to identify all studies
- assessment of the validity of the findings of the included studies (e.g. risk of bias)
- systematic presentation, and synthesis, of the characteristics and findings of the included studies
Not all systematic reviews contain meta-analysis.
Meta-analysis is the use of statistical methods to summarize the results of independent studies. By combining information from all relevant studies, meta-analysis can provide more precise estimates of the effects of health care than those derived from the individual studies included within a review. More information on meta-analyses can be found in Cochrane Handbook, Chapter 9 .
A meta-analysis goes beyond critique and integration and conducts secondary statistical analysis on the outcomes of similar studies. It is a systematic review that uses quantitative methods to synthesize and summarize the results.
An advantage of a meta-analysis is the ability to be completely objective in evaluating research findings. Not all topics, however, have sufficient research evidence to allow a meta-analysis to be conducted. In that case, an integrative review is an appropriate strategy.
Some of the content in this section is from Systematic reviews and meta-analyses: step by step guide created by Kate McAllister.
- << Previous: Getting Started
- Next: Research Design >>
- Last Updated: Jul 15, 2024 10:34 AM
- URL: https://guides.lib.udel.edu/researchmethods

Teaching and Research guides
Literature reviews.
- Introduction
- Plan your search
- Where to search
- Refine and update your search
- Finding grey literature
- Writing the review
- Referencing
Research methods overview
Finding literature on research methodologies, sage research methods online.
- Get material not at RMIT
- Further help
What are research methods?
Research methodology is the specific strategies, processes, or techniques utilised in the collection of information that is created and analysed.
The methodology section of a research paper, or thesis, enables the reader to critically evaluate the study’s validity and reliability by addressing how the data was collected or generated, and how it was analysed.
Types of research methods
There are three main types of research methods which use different designs for data collection.
(1) Qualitative research
Qualitative research gathers data about lived experiences, emotions or behaviours, and the meanings individuals attach to them. It assists in enabling researchers to gain a better understanding of complex concepts, social interactions or cultural phenomena. This type of research is useful in the exploration of how or why things have occurred, interpreting events and describing actions.
Examples of qualitative research designs include:
- focus groups
- observations
- document analysis
- oral history or life stories
(2) Quantitative research
Quantitative research gathers numerical data which can be ranked, measured or categorised through statistical analysis. It assists with uncovering patterns or relationships, and for making generalisations. This type of research is useful for finding out how many, how much, how often, or to what extent.
Examples of quantitative research designs include:
- surveys or questionnaires
- observation
- document screening
- experiments
(3) Mixed method research
Mixed Methods research integrates both Qualitative research and Quantitative research. It provides a holistic approach combining and analysing the statistical data with deeper contextualised insights. Using Mixed Methods also enables triangulation, or verification, of the data from two or more sources.
Sometimes in your literature review, you might need to discuss and evaluate relevant research methodologies in order to justify your own choice of research methodology.
When searching for literature on research methodologies it is important to search across a range of sources. No single information source will supply all that you need. Selecting appropriate sources will depend upon your research topic.
Developing a robust search strategy will help reduce irrelevant results. It is good practice to plan a strategy before you start to search.
Search tips
(1) free text keywords.
Free text searching is the use of natural language words to conduct your search. Use selective free text keywords such as: phenomenological, "lived experience", "grounded theory", "life experiences", "focus groups", interview, quantitative, survey, validity, variance, correlation and statistical.
To locate books on your desired methodology, try LibrarySearch . Remember to use refine options such as books, ebooks, subject, and publication date.
(2) Subject headings in Databases
Databases categorise their records using subject terms, or a controlled vocabulary (thesaurus). These subject headings may be useful to use, in addition to utilising free text keywords in a database search.
Subject headings will differ across databases, for example, the PubMed database uses 'Qualitative Research' whilst the CINHAL database uses 'Qualitative Studies.'
(3) Limiting search results
Databases enable sets of results to be limited or filtered by specific fields, look for options such as Publication Type, Article Type, etc. and apply them to your search.
(4) Browse the Library shelves
To find books on research methods browse the Library shelves at call number 001.42
- SAGE Research Methods Online SAGE Research Methods Online (SRMO) is a research tool supported by a newly devised taxonomy that links content and methods terms. It provides the most comprehensive picture available today of research methods (quantitative, qualitative and mixed methods) across the social and behavioural sciences.
SAGE Research Methods Overview (2:07 min) by SAGE Publishing ( YouTube )
- << Previous: Referencing
- Next: Get material not at RMIT >>

- Last Updated: Sep 14, 2024 4:19 PM
- URL: https://rmit.libguides.com/literature-review

Education: Lit Review + Methods
- Getting Started
- News and Data Sources
- Children's Literature
- Lit Review + Methods
- Praxis Prep
- Need help? Off campus?

What is a Literature Review?
A literature review is a comprehensive and up-to-date overview of the principal research about the topic being studied. Your literature review should contain the following information:
- The most pertinent studies and important past and current research and practices in the field
- An overview of sources you have explored while researching a particular topic
- An explanation to your readers as to how your research fits within a larger field of study.
The review helps form the intellectual framework for the study.
17 - what is a literature review from Joshua Vossler on Vimeo .
Why do a Literature Review?
At its core, a literature provides a summary of existing knowledge on a subject or topic and identifies areas where research is lacking: missing information, incomplete studies or studies that draw conflicting conclusions, or perhaps even outdated methods of research.
This can be especially helpful if you intend to conduct research of your own on this topic; by explaining where the previous studies have fallen short or leave openings for further examination, you provide a strong foundation and justification for the research project you intend to embark on.
Literature reviews can stand on their own as an article or assignment for a class, or they can serve as an introduction to a larger work, such as an article describing a study or even a book. They can also vary in granularity: a literature review in the beginning of an article might only summarize the largest or most influential studies, while an academic literature review will not only describe the research so far but look for common themes, analyze the quality of the research, and explain gaps where further research is needed.
Elements of a Successful Literature Review
When preparing your literature review, keep these questions in mind:
- What is your literature review about?
- Why are you studying this topic?
- How will you organize your sources? (You could group them by themes or subtopics, or perhaps keep them in chronological order. The way you present your sources is important, so make sure you think hard about this!)
- What are the major themes/subtopics that you discovered when reading your sources?
- Where could more research be done to increase our understanding of this topic?
For each individual source, be prepared to analyze:
- Who were the key researchers and what are their qualifications?
- How was the research conducted?
- The similarities and differences between this source and the others in your literature review
- How this source contributes to greater understanding of the topic as a whole
- Any questions you have about the research done, which could identify opportunities for further study
When preparing your literature review, examine these elements and determine which ones would be best for your paper. (Tip: If you're not sure which parts of the literature review to include, ask your professor!)
Electronic Resources Librarian

- << Previous: Children's Literature
- Next: Praxis Prep >>
- Last Updated: Sep 25, 2024 11:33 AM
- URL: https://library.usca.edu/Ed
- UWF Libraries
Literature Review: Conducting & Writing
- Sample Literature Reviews
- Steps for Conducting a Lit Review
- Finding "The Literature"
- Organizing/Writing
- APA Style This link opens in a new window
- Chicago: Notes Bibliography This link opens in a new window
- MLA Style This link opens in a new window
Sample Lit Reviews from Communication Arts
Have an exemplary literature review.
Note: These are sample literature reviews from a class that were given to us by an instructor when APA 6th edition was still in effect. These were excellent papers from her class, but it does not mean they are perfect or contain no errors. Thanks to the students who let us post!
- Literature Review Sample 1
- Literature Review Sample 2
- Literature Review Sample 3
Have you written a stellar literature review you care to share for teaching purposes?
Are you an instructor who has received an exemplary literature review and have permission from the student to post?
Please contact Britt McGowan at [email protected] for inclusion in this guide. All disciplines welcome and encouraged.
- << Previous: MLA Style
- Next: Get Help! >>
- Last Updated: Sep 11, 2024 1:37 PM
- URL: https://libguides.uwf.edu/litreview

SOC 200 - Sims: How to Write a Lit Review
- What are Literature Reviews?
- How to Write a Lit Review
- How to Choose a Topic
- Finding the Literature
How to write a literature review
Below are the steps you should follow when crafting a lit review for your class assignment.
- It's preferable if you can select a topic that you find interesting, because this will make the work seem less like work.
- It's also important to select a topic that many researchers have already explored. This way, you'll actually have "literature" to "review."
- Sometimes, doing a very general search and reading other literature reviews can reveal a topic or avenue of research to you.
- It's important to gain an understanding of your topic's research history, in order to properly comprehend how and why the current (emerging) research exists.
- One trick is to look at the References (aka Bibliographies aka Works Cited pages) of any especially relevant articles, in order to expand your search for those same sources. This is because there is often overlap between works, and if you're paying attention, one source can point you to several others.
- One method is to start with the most recently-published research and then use their citations to identify older research, allowing you to piece together a timeline and work backwards.
- Chronologically : discuss the literature in order of its writing/publication. This will demonstrate a change in trends over time, and/or detail a history of controversy in the field, and/or illustrate developments in the field.
- Thematically : group your sources by subject or theme. This will show the variety of angels from which your topic has been studied. This method works well if you are trying to identify a sub-topic that has so far been overlooked by other researchers.
- Methodologically : group your sources by methodology. For example, divide the literature into categories like qualitative versus quantitative, or by population or geographical region, etc.
- Theoretically : group your sources by theoretical lens. Your textbook should have a section(s) dedicated to the various theories in your field. If you're unsure, you should ask your professor.
- Are there disagreements on some issues, and consensus on others?
- How does this impact the path of research and discovery?
- Many articles will have a Limitations section, or a Discussion section, wherein suggestions are provided for next steps to further the research.
- These are goldmines for helping you see a possible outlook of the situation.
- Identifying any gaps in the literature that are of a particular interest to your research goals will help you justify why your own research should be performed.
- Be selective about which points from the source you use. The information should be the most important and the most relevant.
- Use direct quotes sparingly, and don't rely too heavily on summaries and paraphrasing. You should be drawing conclusions about how the literature relates to your own analysis or the other literature.
- Synthesize your sources. The goal is not to make a list of summaries, but to show how the sources relate to one another and to your own analysis.
- At the end, make suggestions for future research. What subjects, populations, methodologies, or theoretical lenses warrant further exploration? What common flaws or biases did you identify that could be corrected in future studies?
- Common citation styles for sociology classes include APA and ASA.
Understanding how a literature review is structured will help you as you craft your own.
Below is information and example articles that you should review, in order to comprehend why they are written a certain way.
Below are some very good examples of Literature Reviews:
Cyberbullying: How Physical Intimidation Influences the Way People are Bullied
Use of Propofol and Emergence Agitation in Children
Eternity and Immortality in Spinoza's 'Ethics'
As you read these, take note of the sections that comprise the main structure of each one:
- Introduction
- Summarize sources
- Synthesize sources

Below are some articles that provide very good examples of an "Introduction" section, which includes a "Review of the Literature."
- Sometimes, there is both an Introduction section, and a separate Review of the Literature section (oftentimes, it simply depends on the publication)
Krimm, H., & Lund, E. (2021). Efficacy of online learning modules for teaching dialogic reading strategies and phonemic awareness. Language, Speech & Hearing Services in Schools, 52 (4), 1020-1030. https://doi.org/10.1044/2021_LSHSS-21-00011

Melfsen, S., Jans, T., Romanos, M., & Walitza, S. (2022). Emotion regulation in selective mutism: A comparison group study in children and adolescents with selective mutism. Journal of Psychiatric Research, 151 , 710-715. https://doi.org/10.1016/j.jpsychires.2022.05.040
Citation Resources
- MU Library's Citing Sources page
- Purdue OWL's APA Guide
- APA Citation Style - Quick Guide
- Purdue OWL's ASA Guide
- ASA Citation Style - Quick Tips
Suggested Reading
- How to: Conduct a Lit Review (from Central Michigan University)
- Purdue OWL Writing Lab's Advice for Writing a Lit Review
How to Read a Scholarly Article
read:.
- Things to consider when reading a scholarly article This helpful guide, from Meriam Library at California State University in Chico, explains what a scholarly article is and provides tips for reading them.
Watch:
- How to read a scholarly article (YouTube) This tutorial, from Western University, quickly and efficiently describes how to read a scholarly article.
- << Previous: What are Literature Reviews?
- Next: How to Choose a Topic >>
- Last Updated: Sep 27, 2024 3:57 PM
- URL: https://libguides.marshall.edu/soc200-sims
Information
- Author Services
Initiatives
You are accessing a machine-readable page. In order to be human-readable, please install an RSS reader.
All articles published by MDPI are made immediately available worldwide under an open access license. No special permission is required to reuse all or part of the article published by MDPI, including figures and tables. For articles published under an open access Creative Common CC BY license, any part of the article may be reused without permission provided that the original article is clearly cited. For more information, please refer to https://www.mdpi.com/openaccess .
Feature papers represent the most advanced research with significant potential for high impact in the field. A Feature Paper should be a substantial original Article that involves several techniques or approaches, provides an outlook for future research directions and describes possible research applications.
Feature papers are submitted upon individual invitation or recommendation by the scientific editors and must receive positive feedback from the reviewers.
Editor’s Choice articles are based on recommendations by the scientific editors of MDPI journals from around the world. Editors select a small number of articles recently published in the journal that they believe will be particularly interesting to readers, or important in the respective research area. The aim is to provide a snapshot of some of the most exciting work published in the various research areas of the journal.
Original Submission Date Received: .
- Active Journals
- Find a Journal
- Journal Proposal
- Proceedings Series
- For Authors
- For Reviewers
- For Editors
- For Librarians
- For Publishers
- For Societies
- For Conference Organizers
- Open Access Policy
- Institutional Open Access Program
- Special Issues Guidelines
- Editorial Process
- Research and Publication Ethics
- Article Processing Charges
- Testimonials
- Preprints.org
- SciProfiles
- Encyclopedia
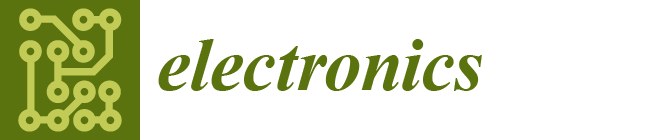
Article Menu

- Subscribe SciFeed
- Recommended Articles
- Google Scholar
- on Google Scholar
- Table of Contents
Find support for a specific problem in the support section of our website.
Please let us know what you think of our products and services.
Visit our dedicated information section to learn more about MDPI.
JSmol Viewer
Literature review of explainable tabular data analysis.
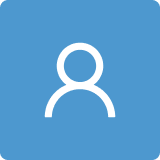
1. Introduction
The objectives of this survey.
- To analyze the various techniques, inputs, and methods used to build XAI models since 2021, aiming to identify any superior models for tabular data that have been created since Sahakyan et al.’s, paper.
- To identify and expand upon Sahakyan et al.’s description of need, challenges, gaps, and opportunities in XAI for tabular data.
- To explore evaluation methods and metrics used to assess the effectiveness of XAI models specifically concerning tabular data and to see if any new metrics have been developed.
2. Background
Aspects of Transparency | Definitions | Reference |
---|---|---|
Transparency | Transparency does not ensure that a user will fully understand the system, but it does provide access to all relevant information regarding the training data, data preprocessing, system performance, and more. | [ ] |
Algorithmic transparency | Refers to the user’s capacity to comprehend the process the model uses to generate a specific output based on its input data. The main limitation for algorithmically transparent models is that they must be entirely accessible for exploration through mathematical analysis and techniques. | [ ] |
Decomposability | Decomposability is the capacity to explain each component of a model, including its inputs, parameters, and calculations. This enhances the understanding and interpretation of the model’s behavior. However, similar to algorithmic transparency, not all models can achieve this. For a model to be decomposable, each input must be easily interpretable, meaning complex features may hinder this ability. Additionally, for an algorithmically transparent model to be decomposable, all its parts must be comprehensible to a human without needing external tools. | [ ] |
Simulatability | This is a model’s capacity to be understood and conceptualized by a human, with complexity being a main factor. Simple models like single perceptron neural networks fit this criterion, more complex rule-based systems with excessive rules do not. An interpretable model should be easily explained through text and visualizations. The model must be sufficiently self-contained for a person to consider and reason on it as a whole. | [ ] |
Interaction transparency | Is the clarity and openness in the interactions between users and AI systems? It involves giving users feedback they understand about the system’s actions, decisions, and processes, allowing them to understand how their inputs influence outcomes. This transparency fosters trust and enables users to engage more effectively with technology, as they can see and understand the rationale behind the AI’s behavior. | [ ] |
Social transparency | This is the openness and clarity of an AI system’s impact on social dynamics and user interactions. It involves making the system’s intentions, decision-making processes, and effects on individuals and communities clear to users and stakeholders. Social transparency helps users understand how AI influences relationships, societal norms, and behaviors, fostering trust and the responsible use of technology. | [ ] |
3. Existing Techniques for Explainable Tabular Data Analysis
4. challenges and gaps in explainable tabular data analysis, 4.1. challenges of tabular data, 4.2. bias, incomplete and inaccurate data, 4.3. explanation quality, 4.4. scalability of techniques, 4.5. neural networks, 4.6. xai methods, 4.7. benchmark datasets, 4.8. scalability, 4.9. data structure, 4.10. model evaluation and benchmarks, 4.11. review, 5. applications of explainable tabular data analysis, 5.1. financial sector, 5.2. healthcare sector, 5.4. retail sector, 5.5. manufacturing sector, 5.6. utility sector, 5.7. education, 5.8. summary, 6. future directions and emerging trends, 7. conclusions, author contributions, data availability statement, conflicts of interest.
- Ali, S.; Abuhmed, T.; El-Sappagh, S.; Muhammad, K.; Alonso-Moral, J.M.; Confalonieri, R.; Guidotti, R.; Del Ser, J.; Díaz-Rodríguez, N.; Herrera, F. Explainable Artificial Intelligence (XAI): What we know and what is left to attain Trustworthy Artificial Intelligence. Inf. Fusion 2023 , 99 , 101805. [ Google Scholar ] [ CrossRef ]
- Burkart, N.; Huber, M.F. A Survey on the Explainability of Supervised Machine Learning. J. Artif. Intell. Res. 2021 , 70 , 245–317. [ Google Scholar ] [ CrossRef ]
- Weber, L.; Lapuschkin, S.; Binder, A.; Samek, W. Beyond explaining: Opportunities and challenges of XAI-based model improvement. Inf. Fusion 2023 , 92 , 154–176. [ Google Scholar ] [ CrossRef ]
- Marcinkevičs, R.; Vogt, J.E. Interpretable and explainable machine learning: A methods-centric overview with concrete examples. WIREs Data Min. Knowl. Discov. 2023 , 13 , e1493. [ Google Scholar ] [ CrossRef ]
- Sahakyan, M.; Aung, Z.; Rahwan, T. Explainable Artificial Intelligence for Tabular Data: A Survey. IEEE Access 2021 , 9 , 135392–135422. [ Google Scholar ] [ CrossRef ]
- Alicioglu, G.; Sun, B. A survey of visual analytics for Explainable Artificial Intelligence methods. Comput. Graph. 2021 , 102 , 502–520. [ Google Scholar ] [ CrossRef ]
- Cambria, E.; Malandri, L.; Mercorio, F.; Mezzanzanica, M.; Nobani, N. A survey on XAI and natural language explanations. Inf. Process. Manag. 2023 , 60 , 103111. [ Google Scholar ] [ CrossRef ]
- Chinu, U.; Bansal, U. Explainable AI: To Reveal the Logic of Black-Box Models. New Gener. Comput. 2023 , 42 , 53–87. [ Google Scholar ] [ CrossRef ]
- Schwalbe, G.; Finzel, B. A comprehensive taxonomy for explainable artificial intelligence: A systematic survey of surveys on methods and concepts. Data Min. Knowl. Discov. 2023 , 38 , 3043–3101. [ Google Scholar ] [ CrossRef ]
- Yang, W.; Wei, Y.; Wei, H.; Chen, Y.; Huang, G.; Li, X.; Li, R.; Yao, N.; Wang, X.; Gu, X.; et al. Survey on Explainable AI: From Approaches, Limitations and Applications Aspects. Hum.-Centric Intell. Syst. 2023 , 3 , 161–188. [ Google Scholar ] [ CrossRef ]
- Hamm, P.; Klesel, M.; Coberger, P.; Wittmann, H.F. Explanation matters: An experimental study on explainable AI. Electron. Mark. 2023 , 33 , 17. [ Google Scholar ] [ CrossRef ]
- Lance, E. Ways That the GDPR Encompasses Stipulations for Explainable AI or XAI ; SSRN, Stanford Center for Legal Informatics: Stanford, CA, USA, 2022; pp. 1–7. Available online: https://ssrn.com/abstract=4085089 (accessed on 30 August 2023).
- Gunning, D.; Vorm, E.; Wang, J.Y.; Turek, M. DARPA’s explainable AI (XAI) program: A retrospective. Appl. AI Lett. 2021 , 2 , e61. [ Google Scholar ] [ CrossRef ]
- Allgaier, J.; Mulansky, L.; Draelos, R.L.; Pryss, R. How does the model make predictions? A systematic literature review on the explainability power of machine learning in healthcare. Artif. Intell. Med. 2023 , 143 , 102616. [ Google Scholar ] [ CrossRef ] [ PubMed ]
- Graziani, M.; Dutkiewicz, L.; Calvaresi, D.; Amorim, J.P.; Yordanova, K.; Vered, M.; Nair, R.; Abreu, P.H.; Blanke, T.; Pulignano, V.; et al. A global taxonomy of interpretable AI: Unifying the terminology for the technical and social sciences. Artif. Intell. Rev. 2022 , 56 , 3473–3504. [ Google Scholar ] [ CrossRef ]
- Bellucci, M.; Delestre, N.; Malandain, N.; Zanni-Merk, C. Towards a terminology for a fully contextualized XAI. Procedia Comput. Sci. 2021 , 192 , 241–250. [ Google Scholar ] [ CrossRef ]
- Barbiero, P.; Fioravanti, S.; Giannini, F.; Tonda, A.; Lio, P.; Di Lavore, E. Categorical Foundations of Explainable AI: A Unifying Formalism of Structures and Semantics. In Explainable Artificial Intelligence. xAI, Proceedings of the Communications in Computer and Information Science, Delhi, India, 21–24 May 2024 ; Springer: Cham, Switzerland, 2024; Volume 2155, pp. 185–206. [ Google Scholar ] [ CrossRef ]
- Vilone, G.; Longo, L. Notions of explainability and evaluation approaches for explainable artificial intelligence. Inf. Fusion 2021 , 76 , 89–106. [ Google Scholar ] [ CrossRef ]
- Rudin, C. Stop explaining black box machine learning models for high stakes decisions and use interpretable models instead. Nat. Mach. Intell. 2019 , 1 , 206–215. [ Google Scholar ] [ CrossRef ]
- Arrieta, A.B.; Díaz-Rodríguez, N.; Del Ser, J.; Bennetot, A.; Tabik, S.; Barbado, A.; Garcia, S.; Gil-Lopez, S.; Molina, D.; Benjamins, R.; et al. Explainable Artificial Intelligence (XAI): Concepts, taxonomies, opportunities and challenges toward responsible AI. Inf. Fusion 2020 , 58 , 82–115. [ Google Scholar ] [ CrossRef ]
- Haresamudram, K.; Larsson, S.; Heintz, F. Three Levels of AI Transparency. Computer 2023 , 56 , 93–100. [ Google Scholar ] [ CrossRef ]
- Wadden, J.J. Defining the undefinable: The black box problem in healthcare artificial intelligence. J. Med Ethic 2021 , 48 , 764–768. [ Google Scholar ] [ CrossRef ]
- Burrell, J. How the machine ‘thinks’: Understanding opacity in machine learning algorithms. Big Data Soc. 2016 , 3 , 1–12. [ Google Scholar ] [ CrossRef ]
- Markus, A.F.; Kors, J.A.; Rijnbeek, P.R. The role of explainability in creating trustworthy artificial intelligence for health care: A comprehensive survey of the terminology, design choices, and evaluation strategies. J. Biomed. Inform. 2021 , 113 , 103655. [ Google Scholar ] [ CrossRef ] [ PubMed ]
- Brożek, B.; Furman, M.; Jakubiec, M.; Kucharzyk, B. The black box problem revisited. Real and imaginary challenges for automated legal decision making. Artif. Intell. Law 2023 , 32 , 427–440. [ Google Scholar ] [ CrossRef ]
- Li, D.; Liu, Y.; Huang, J.; Wang, Z. A Trustworthy View on Explainable Artificial Intelligence Method Evaluation. Computer 2023 , 56 , 50–60. [ Google Scholar ] [ CrossRef ]
- Nauta, M.; Trienes, J.; Pathak, S.; Nguyen, E.; Peters, M.; Schmitt, Y.; Schlötterer, J.; van Keulen, M.; Seifert, C. From Anecdotal Evidence to Quantitative Evaluation Methods: A Systematic Review on Evaluating Explainable AI. ACM Comput. Surv. 2023 , 55 , 295. [ Google Scholar ] [ CrossRef ]
- Lopes, P.; Silva, E.; Braga, C.; Oliveira, T.; Rosado, L. XAI Systems Evaluation: A Review of Human and Computer-Centred Methods. Appl. Sci. 2022 , 12 , 9423. [ Google Scholar ] [ CrossRef ]
- Baptista, M.L.; Goebel, K.; Henriques, E.M. Relation between prognostics predictor evaluation metrics and local interpretability SHAP values. Artif. Intell. 2022 , 306 , 103667. [ Google Scholar ] [ CrossRef ]
- Fouladgar, N.; Alirezaie, M.; Framling, K. Metrics and Evaluations of Time Series Explanations: An Application in Affect Computing. IEEE Access 2022 , 10 , 23995–24009. [ Google Scholar ] [ CrossRef ]
- Oblizanov, A.; Shevskaya, N.; Kazak, A.; Rudenko, M.; Dorofeeva, A. Evaluation Metrics Research for Explainable Artificial Intelligence Global Methods Using Synthetic Data. Appl. Syst. Innov. 2023 , 6 , 26. [ Google Scholar ] [ CrossRef ]
- Speith, T. A Review of Taxonomies of Explainable Artificial Intelligence (XAI) Methods. In Proceedings of the FAccT ‘22: 2022 ACM Conference on Fairness, Accountability, and Transparency, Seoul, Republic of Korea, 21–24 June 2022; pp. 2239–2250. [ Google Scholar ]
- Kurdziolek, M. Explaining the Unexplainable: Explainable AI (XAI) for UX. User Experience Magazine . 2022. Available online: https://uxpamagazine.org/explaining-the-unexplainable-explainable-ai-xai-for-ux/ (accessed on 20 August 2023).
- Kim, B.; Wattenberg, M.; Gilmer, J.; Cai, C.; Wexler, J.; Viegas, F.; Sayres, R. Interpretability beyond feature attribution: Quantitative Testing with Concept Activation Vectors (TCAV). In Proceedings of the 35th International Conference on Machine Learning, ICML, Stockholm, Sweden, 10–15 July 2018; Volume 6, pp. 4186–4195. Available online: https://proceedings.mlr.press/v80/kim18d/kim18d.pdf (accessed on 30 July 2024).
- Kenny, E.M.; Keane, M.T. Explaining Deep Learning using examples: Optimal feature weighting methods for twin systems using post-hoc, explanation-by-example in XAI. Knowl. Based Syst. 2021 , 233 , 107530. [ Google Scholar ] [ CrossRef ]
- Alfeo, A.L.; Zippo, A.G.; Catrambone, V.; Cimino, M.G.; Toschi, N.; Valenza, G. From local counterfactuals to global feature importance: Efficient, robust, and model-agnostic explanations for brain connectivity networks. Comput. Methods Programs Biomed. 2023 , 236 , 107550. [ Google Scholar ] [ CrossRef ] [ PubMed ]
- An, J.; Zhang, Y.; Joe, I. Specific-Input LIME Explanations for Tabular Data Based on Deep Learning Models. Appl. Sci. 2023 , 13 , 8782. [ Google Scholar ] [ CrossRef ]
- Bharati, S.; Mondal, M.R.H.; Podder, P. A Review on Explainable Artificial Intelligence for Healthcare: Why, How, and When? IEEE Trans. Artif. Intell. 2023 , 5 , 1429–1442. [ Google Scholar ] [ CrossRef ]
- Chaddad, A.; Peng, J.; Xu, J.; Bouridane, A. Survey of Explainable AI Techniques in Healthcare. Sensors 2023 , 23 , 634. [ Google Scholar ] [ CrossRef ]
- Chamola, V.; Hassija, V.; Sulthana, A.R.; Ghosh, D.; Dhingra, D.; Sikdar, B. A Review of Trustworthy and Explainable Artificial Intelligence (XAI). IEEE Access 2023 , 11 , 78994–79015. [ Google Scholar ] [ CrossRef ]
- Chen, X.-Q.; Ma, C.-Q.; Ren, Y.-S.; Lei, Y.-T.; Huynh, N.Q.A.; Narayan, S. Explainable artificial intelligence in finance: A bibliometric review. Financ. Res. Lett. 2023 , 56 , 104145. [ Google Scholar ] [ CrossRef ]
- Di Martino, F.; Delmastro, F. Explainable AI for clinical and remote health applications: A survey on tabular and time series data. Artif. Intell. Rev. 2022 , 56 , 5261–5315. [ Google Scholar ] [ CrossRef ]
- Kök, I.; Okay, F.Y.; Muyanlı, O.; Özdemir, S. Explainable Artificial Intelligence (XAI) for Internet of Things: A Survey. IEEE Internet Things J. 2023 , 10 , 14764–14779. [ Google Scholar ] [ CrossRef ]
- Haque, A.B.; Islam, A.N.; Mikalef, P. Explainable Artificial Intelligence (XAI) from a user perspective: A synthesis of prior literature and problematizing avenues for future research. Technol. Forecast. Soc. Chang. 2023 , 186 , 122120. [ Google Scholar ] [ CrossRef ]
- Sahoh, B.; Choksuriwong, A. The role of explainable Artificial Intelligence in high-stakes decision-making systems: A systematic review. J. Ambient. Intell. Humaniz. Comput. 2023 , 14 , 7827–7843. [ Google Scholar ] [ CrossRef ]
- Saranya, A.; Subhashini, R. A systematic review of Explainable Artificial Intelligence models and applications: Recent developments and future trends. Decis. Anal. J. 2023 , 7 , 100230. [ Google Scholar ] [ CrossRef ]
- Sosa-Espadas, C.E.; Orozco-Del-Castillo, M.G.; Cuevas-Cuevas, N.; Recio-Garcia, J.A. IREX: Iterative Refinement and Explanation of classification models for tabular datasets. SoftwareX 2023 , 23 , 101420. [ Google Scholar ] [ CrossRef ]
- Meding, K.; Hagendorff, T. Fairness Hacking: The Malicious Practice of Shrouding Unfairness in Algorithms. Philos. Technol. 2024 , 37 , 4. [ Google Scholar ] [ CrossRef ]
- Batko, K.; Ślęzak, A. The use of Big Data Analytics in healthcare. J. Big Data 2022 , 9 , 3. [ Google Scholar ] [ CrossRef ]
- Borisov, V.; Leemann, T.; Seßler, K.; Haug, J.; Pawelczyk, M.; Kasneci, G. Deep Neural Networks and Tabular Data: A Survey. IEEE Trans. Neural Netw. Learn. Syst. 2022 , 35 , 7499–7519. [ Google Scholar ] [ CrossRef ]
- Mbanaso, M.U.; Abrahams, L.; Okafor, K.C. Data Collection, Presentation and Analysis. In Research Techniques for Computer Science, Information Systems and Cybersecurity ; Springer: Cham, Switzerland, 2023; pp. 115–138. [ Google Scholar ] [ CrossRef ]
- Tjoa, E.; Guan, C. A Survey on Explainable Artificial Intelligence (XAI): Toward Medical XAI. IEEE Trans. Neural Networks Learn. Syst. 2021 , 32 , 4793–4813. [ Google Scholar ] [ CrossRef ]
- Gajcin, J.; Dusparic, I. Redefining Counterfactual Explanations for Reinforcement Learning: Overview, Challenges and Opportunities. ACM Comput. Surv. 2024 , 56 , 219. [ Google Scholar ] [ CrossRef ]
- Hassija, V.; Chamola, V.; Mahapatra, A.; Singal, A.; Goel, D.; Huang, K.; Scardapane, S.; Spinelli, I.; Mahmud, M.; Hussain, A. Interpreting Black-Box Models: A Review on Explainable Artificial Intelligence. Cogn. Comput. 2023 , 16 , 45–74. [ Google Scholar ] [ CrossRef ]
- Lötsch, J.; Kringel, D.; Ultsch, A. Explainable Artificial Intelligence (XAI) in Biomedicine: Making AI Decisions Trustworthy for Physicians and Patients. BioMedInformatics 2021 , 2 , 1–17. [ Google Scholar ] [ CrossRef ]
- Hossain, I.; Zamzmi, G.; Mouton, P.R.; Salekin, S.; Sun, Y.; Goldgof, D. Explainable AI for Medical Data: Current Methods, Limitations, and Future Directions. ACM Comput. Surv. 2023 . [ Google Scholar ] [ CrossRef ]
- Rudin, C.; Chen, C.; Chen, Z.; Huang, H.; Semenova, L.; Zhong, C. Interpretable machine learning: Fundamental principles and 10 grand challenges. Stat. Surv. 2022 , 16 , 1–85. [ Google Scholar ] [ CrossRef ]
- Zhong, X.; Gallagher, B.; Liu, S.; Kailkhura, B.; Hiszpanski, A.; Han, T.Y.-J. Explainable machine learning in materials science. NPJ Comput. Mater. 2022 , 8 , 204. [ Google Scholar ] [ CrossRef ]
- Ekanayake, I.; Meddage, D.; Rathnayake, U. A novel approach to explain the black-box nature of machine learning in compressive strength predictions of concrete using Shapley additive explanations (SHAP). Case Stud. Constr. Mater. 2022 , 16 , e01059. [ Google Scholar ] [ CrossRef ]
- Černevičienė, J.; Kabašinskas, A. Explainable artificial intelligence (XAI) in finance: A systematic literature review. Artif. Intell. Rev. 2024 , 57 , 216. [ Google Scholar ] [ CrossRef ]
- Weber, P.; Carl, K.V.; Hinz, O. Applications of Explainable Artificial Intelligence in Finance—A systematic review of Finance, Information Systems, and Computer Science literature. Manag. Rev. Q. 2024 , 74 , 867–907. [ Google Scholar ] [ CrossRef ]
- Leijnen, S.; Kuiper, O.; van der Berg, M. Impact Your Future Xai in the Financial Sector a Conceptual Framework for Explainable Ai (Xai). Hogeschool Utrecht, Lectoraat Artificial Intelligence, Whitepaper, Version 1, 1–24. 2020. Available online: https://www.hu.nl/onderzoek/projecten/uitlegbare-ai-in-de-financiele-sector (accessed on 2 August 2024).
- Dastile, X.; Celik, T. Counterfactual Explanations with Multiple Properties in Credit Scoring. IEEE Access 2024 , 12 , 110713–110728. [ Google Scholar ] [ CrossRef ]
- Martins, T.; de Almeida, A.M.; Cardoso, E.; Nunes, L. Explainable Artificial Intelligence (XAI): A Systematic Literature Review on Taxonomies and Applications in Finance. IEEE Access 2023 , 12 , 618–629. [ Google Scholar ] [ CrossRef ]
- Kalra, A.; Mittal, R. Explainable AI for Improved Financial Decision Support in Trading. In Proceedings of the 2024 11th International Conference on Reliability, Infocom Technologies and Optimization (Trends and Future Directions) (ICRITO), Noida, India, 14–15 March 2024; pp. 1–6. [ Google Scholar ]
- Wani, N.A.; Kumar, R.; Mamta; Bedi, J.; Rida, I. Explainable AI-driven IoMT fusion: Unravelling techniques, opportunities, and challenges with Explainable AI in healthcare. Inf. Fusion 2024 , 110 , 102472. [ Google Scholar ] [ CrossRef ]
- Li, Y.; Song, X.; Wei, T.; Zhu, B. Counterfactual learning in customer churn prediction under class imbalance. In Proceedings of the 2023 6th International Conference on Big Data Technologies (ICBDT ‘23), Qingdao, China, 22–24 September 2023; pp. 96–102. [ Google Scholar ]
- Zhang, L.; Zhu, Y.; Ni, Q.; Zheng, X.; Gao, Z.; Zhao, Q. Local/Global explainability empowered expert-involved frameworks for essential tremor action recognition. Biomed. Signal Process. Control 2024 , 95 , 106457. [ Google Scholar ] [ CrossRef ]
- Sadeghi, Z.; Alizadehsani, R.; Cifci, M.A.; Kausar, S.; Rehman, R.; Mahanta, P.; Bora, P.K.; Almasri, A.; Alkhawaldeh, R.S.; Hussain, S.; et al. A review of Explainable Artificial Intelligence in healthcare. Comput. Electr. Eng. 2024 , 118 , 109370. [ Google Scholar ] [ CrossRef ]
- Alizadehsani, R.; Oyelere, S.S.; Hussain, S.; Jagatheesaperumal, S.K.; Calixto, R.R.; Rahouti, M.; Roshanzamir, M.; De Albuquerque, V.H.C. Explainable Artificial Intelligence for Drug Discovery and Development: A Comprehensive Survey. IEEE Access 2024 , 12 , 35796–35812. [ Google Scholar ] [ CrossRef ]
- Murindanyi, S.; Mugalu, B.W.; Nakatumba-Nabende, J.; Marvin, G. Interpretable Machine Learning for Predicting Customer Churn in Retail Banking. In Proceedings of the 2023 7th International Conference on Trends in Electronics and Informatics (ICOEI)., Tirunelveli, India, 11–13 April 2023; pp. 967–974. [ Google Scholar ]
- Mill, E.; Garn, W.; Ryman-Tubb, N.; Turner, C. Opportunities in Real Time Fraud Detection: An Explainable Artificial Intelligence (XAI) Research Agenda. Int. J. Adv. Comput. Sci. Appl. 2023 , 14 , 1172–1186. [ Google Scholar ] [ CrossRef ]
- Dutta, J.; Puthal, D.; Yeun, C.Y. Next Generation Healthcare with Explainable AI: IoMT-Edge-Cloud Based Advanced eHealth. In Proceedings of the IEEE Global Communications Conference, GLOBECOM, Kuala Lumpur, Malaysia, 4–8 December 2023; pp. 7327–7332. [ Google Scholar ]
- Njoku, J.N.; Nwakanma, C.I.; Lee, J.-M.; Kim, D.-S. Evaluating regression techniques for service advisor performance analysis in automotive dealerships. J. Retail. Consum. Serv. 2024 , 80 , 103933. [ Google Scholar ] [ CrossRef ]
- Agostinho, C.; Dikopoulou, Z.; Lavasa, E.; Perakis, K.; Pitsios, S.; Branco, R.; Reji, S.; Hetterich, J.; Biliri, E.; Lampathaki, F.; et al. Explainability as the key ingredient for AI adoption in Industry 5.0 settings. Front. Artif. Intell. 2023 , 6 , 1264372. [ Google Scholar ] [ CrossRef ]
- Finzel, B.; Tafler, D.E.; Thaler, A.M.; Schmid, U. Multimodal Explanations for User-centric Medical Decision Support Systems. CEUR Workshop Proc. 2021 , 3068 , 1–6. [ Google Scholar ]
- Brochado, F.; Rocha, E.M.; Addo, E.; Silva, S. Performance Evaluation and Explainability of Last-Mile Delivery. Procedia Comput. Sci. 2024 , 232 , 2478–2487. [ Google Scholar ] [ CrossRef ]
- Kostopoulos, G.; Davrazos, G.; Kotsiantis, S. Explainable Artificial Intelligence-Based Decision Support Systems: A Recent Review. Electronics 2024 , 13 , 2842. [ Google Scholar ] [ CrossRef ]
- Nyrup, R.; Robinson, D. Explanatory pragmatism: A context-sensitive framework for explainable medical AI. Ethics Inf. Technol. 2022 , 24 , 13. [ Google Scholar ] [ CrossRef ]
- Talaat, F.M.; Aljadani, A.; Alharthi, B.; Farsi, M.A.; Badawy, M.; Elhosseini, M. A Mathematical Model for Customer Segmentation Leveraging Deep Learning, Explainable AI, and RFM Analysis in Targeted Marketing. Mathematics 2023 , 11 , 3930. [ Google Scholar ] [ CrossRef ]
- Kulkarni, S.; Rodd, S.F. Context Aware Recommendation Systems: A review of the state of the art techniques. Comput. Sci. Rev. 2020 , 37 , 100255. [ Google Scholar ] [ CrossRef ]
- Sarker, A.A.; Shanmugam, B.; Azam, S.; Thennadil, S. Enhancing smart grid load forecasting: An attention-based deep learning model integrated with federated learning and XAI for security and interpretability. Intell. Syst. Appl. 2024 , 23 , 200422. [ Google Scholar ] [ CrossRef ]
- Nnadi, L.C.; Watanobe, Y.; Rahman, M.; John-Otumu, A.M. Prediction of Students’ Adaptability Using Explainable AI in Educational Machine Learning Models. Appl. Sci. 2024 , 14 , 5141. [ Google Scholar ] [ CrossRef ]
- Vellido, A.; Martín-Guerrero, J.D.; Lisboa, P.J.G. Making machine learning models interpretable. In Proceedings of the 20th European Symposium on Artificial Neural Networks, Computational Intelligence and Machine Learning, Bruges, Belgium, 25–27 April 2012; pp. 163–172. Available online: https://www.esann.org/sites/default/files/proceedings/legacy/es2012-7.pdf (accessed on 16 August 2024).
- Alkhatib, A.; Ennadir, S.; Boström, H.; Vazirgiannis, M. Interpretable Graph Neural Networks for Tabular Data. In Proceedings of the ICLR 2024 Data-Centric Machine Learning Research (DMLR) Workshop, Vienna, Austria, 26–27 July 2024; pp. 1–35. Available online: https://openreview.net/pdf/60ce21fd5bcf7b6442b1c9138d40e45251d03791.pdf (accessed on 23 August 2024).
- Saeed, W.; Omlin, C. Explainable AI (XAI): A systematic meta-survey of current challenges and future opportunities. Knowl. Based Syst. 2023 , 263 , 110273. [ Google Scholar ] [ CrossRef ]
- de Oliveira, R.M.B.; Martens, D. A Framework and Benchmarking Study for Counterfactual Generating Methods on Tabular Data. Appl. Sci. 2021 , 11 , 7274. [ Google Scholar ] [ CrossRef ]
- Bienefeld, N.; Boss, J.M.; Lüthy, R.; Brodbeck, D.; Azzati, J.; Blaser, M.; Willms, J.; Keller, E. Solving the explainable AI conundrum by bridging clinicians’ needs and developers’ goals. NPJ Digit. Med. 2023 , 6 , 94. [ Google Scholar ] [ CrossRef ]
- Molnar, C.; Casalicchio, G.; Bischl, B. Interpretable Machine Learning—A Brief History, State-of-the-Art and Challenges. In ECML PKDD 2020 Workshops, Proceedings of the ECML PKDD 2020, Ghent, Belgium, 14–18 September 2020 ; Koprinska, I., Kamp, M., Appice, A., Loglisci, C., Antonie, L., Zimmermann, A., Guidotti, R., Özgöbek, Ö., Ribeiro, R.P., Gavaldà, R., et al., Eds.; Springer: Cham, Switzerland, 2021; Volume 1323, pp. 417–431. [ Google Scholar ] [ CrossRef ]
- Pawlicki, M.; Pawlicka, A.; Kozik, R.; Choraś, M. Advanced insights through systematic analysis: Mapping future research directions and opportunities for xAI in deep learning and artificial intelligence used in cybersecurity. Neurocomputing 2024 , 590 , 127759. [ Google Scholar ] [ CrossRef ]
- Hartog, P.B.R.; Krüger, F.; Genheden, S.; Tetko, I.V. Using test-time augmentation to investigate explainable AI: Inconsistencies between method, model and human intuition. J. Cheminform. 2024 , 16 , 39. [ Google Scholar ] [ CrossRef ] [ PubMed ]
- Srinivasu, P.N.; Sandhya, N.; Jhaveri, R.H.; Raut, R. From Blackbox to Explainable AI in Healthcare: Existing Tools and Case Studies. Mob. Inf. Syst. 2022 , 2022 , 167821. [ Google Scholar ] [ CrossRef ]
- Rong, Y.; Leemann, T.; Nguyen, T.-T.; Fiedler, L.; Qian, P.; Unhelkar, V.; Seidel, T.; Kasneci, G.; Kasneci, E. Towards Human-Centered Explainable AI: A Survey of User Studies for Model Explanations. IEEE Trans. Pattern Anal. Mach. Intell. 2024 , 46 , 2104–2122. [ Google Scholar ] [ CrossRef ]
- Baniecki, H.; Biecek, P. Adversarial attacks and defenses in explainable artificial intelligence: A survey. Inf. Fusion 2024 , 107 , 102303. [ Google Scholar ] [ CrossRef ]
- Panigutti, C.; Hamon, R.; Hupont, I.; Llorca, D.F.; Yela, D.F.; Junklewitz, H.; Scalzo, S.; Mazzini, G.; Sanchez, I.; Garrido, J.S.; et al. The role of explainable AI in the context of the AI Act. In Proceedings of the FAccT ‘23: Proceedings of the 2023 ACM Conference on Fairness, Accountability, and Transparency, Chicago, IL, USA, 12–15 June 2023; pp. 1139–1150. [ Google Scholar ]
- Madiega, T.; Chahri, S. EU Legislation in Progress: Artificial Intelligence Act, 1–12. 2024. Available online: https://www.europarl.europa.eu/RegData/etudes/BRIE/2021/698792/EPRS_BRI(2021)698792_EN.pdf (accessed on 16 August 2024).
Click here to enlarge figure
Domains | Examples of Applications of Explainable Tabular Data |
---|---|
Financial Sector | Identity verification in client onboarding |
Transaction data analysis | |
Fraud detection in claims management | |
Anti-money laundering monitoring | |
Financial trading | |
Risk management | |
Processing of loan applications | |
Bankruptcy prediction | |
Insurance industry | Insurance premium calculation |
Healthcare Sector | Patient diagnosis |
Drug efficacy | |
Personalized healthcare | |
Fraud | Identification of fraudulent transactions |
Retail Sector | Customer churn prediction |
Improve product suggestions to a customer | |
Customer segmentation | |
Human resources | Employee churn prediction |
Evaluate employee performance | |
Manufacturing Sector | Logistics and supply chain management |
Order fulfilment | |
Quality control | |
Process control | |
Planning and scheduling | |
Predictive maintenance | |
Utility Sector | Smart grid load balancing |
Forecast energy consumption for customers | |
Education | Predict student adaptability |
Predict student exam grades | |
Course recommendations |
Database | Reasons |
---|---|
Google Scholar | Comprehensive Coverage: Accesses a wide range of disciplines and sources, including articles, theses, books, and conference papers, providing a broad view of available literature. User-Friendly Interface: Easy to use, making it accessible Citation Tracking: Shows how often articles have been cited and helps to gauge their influence and relevance. |
IEEE Xplore | Specialised Focus: On electrical engineering, computer science, and electronics. High-Quality Publications: Includes peer-reviewed journals and conference proceedings from reputable organizations. Cutting-Edge Research: Provides access to the latest research published in technology and engineering. |
ACM Digital Library | Focus on Computing and Information Technology: Resources specifically related to computing, software engineering, and information systems. Peer-Reviewed Content: High academic quality through rigorous peer review. Conference Proceedings: Important conferences in computing, giving the latest research developments. |
PubMed | Biomedical Focus: A vast collection of literature in medicine, life sciences, and health, often innovative computing solutions. Free Access: Many articles are available for free. High-Quality Research: Peer-reviewed journals and is a trusted source for medical and clinical research. |
Scopus | Extensive Database: A wide range of disciplines Citation Analysis Tools: Provides metrics for authors and journals. Quality Control: Peer-reviewed literature, reliability of the sources. |
ScienceDirect | Multidisciplinary Coverage: A vast collection of scientific and technical research. Quality Journals: High-impact journals. Full-Text Access: Access to a large number of full-text articles, facilitating in-depth research. |
Search Terms | Number of Papers |
---|---|
XAI AND explainable artificial intelligence | 128 |
XAI AND explainable artificial intelligence AND 2021 | 28 |
XAI AND explainable artificial intelligence AND 2022 | 43 |
XAI AND explainable artificial intelligence AND 2023 | 57 |
2021 AND tabular | 2 |
2022 AND tabular | 5 |
2023 AND tabular | 5 |
2021 AND survey (in title) | 5 |
2022 AND survey (in title) | 1 |
2023 AND survey (in title) | 8 |
2021 AND survey AND tabular | 1 |
2022 AND survey AND tabular | 6 |
2023 AND survey AND tabular | 11 |
2021 AND survey AND tabular AND Sahakyan (Sahakyan’s article) | 1 |
2022 AND survey AND tabular AND Sahakyan | 0 |
2023 AND survey AND tabular AND Sahakyan | 2 |
Comprehensibility | Definitions | Reference |
---|---|---|
Comprehensibility | The clarity of the language employed by a method for providing explanations. | [ ] |
Comprehensible systems | Understandable systems produce symbols, allowing users to generate explanations for how a conclusion is derived. | [ ] |
Degree of comprehensibility | This is a subjective evaluation, as the potential for understanding relies on the viewer’s background knowledge. The more specialized the AI application, the greater the reliance on domain knowledge for the comprehensibility of the XAI system. | [ ] |
Comprehensibility of individual explanations | The length of explanations and how readable they are. | [ ] |
Summary of XAI Types | |||||
---|---|---|---|---|---|
Type of XAI | Description | Examples | Pros | Cons | Evaluation |
Counterfactual explanations | Counterfactual explanations illustrate how minimal changes in input features can change the model’s prediction, e.g., “If income increases by £5000, the loan is approved”. | DiCE | Causal insight—understand the causal relationship between input features and predictions. | Complexity—generating counterfactuals is computationally intensive, particularly for complex models and high-dimensional data. | Alignment with predicted outcome—ensuring the generated counterfactual instances closely reflect the intended predicted outcome. |
WatcherCF | Personalized explanations—tailors individualized insights for better insights. | Complexity—generating counterfactuals is computationally intensive, particularly for complex models and high-dimensional data. | Alignment with predicted outcome—ensuring the generated counterfactual instances closely reflect the intended predicted outcome. | ||
GrowingSpheresCF | Decision support—aids decision making with actionable outcome-focused changes | Model specificity—effectiveness is influenced by the underlying model’s characteristics. | Proximity to original instance—maintaining similarity to the original instance whilst altering the fewest features possible. | ||
Interpretation—conveying implications can necessitate domain expertise. | Diverse outputs—capable of producing multiple diverse counterfactual explanations. | ||||
Feasible feature values—the counterfactual features should be practical and adhere to the data distribution. | |||||
Feature importance | Feature importance methods assess how much each feature contributes to the model’s predictions. | Permutation Importance | Helps in feature selection and model interpretability. | May not capture complex feature interactions. | Relative importance—rank features based on their contribution to the model’s prediction. |
Gain Importance. | Provides insight into the most influential features driving the model’s decisions. | Can be sensitive to data noise and model assumptions. | Stability—ensure consistency of feature importance over different subsets of the data or re-trainings of the model. | ||
SHAP | Model impact—Assessing the influence of individual features on the model’s predictive performance | ||||
LIME | |||||
Feature interactions | Feature interaction analysis looks at how the combined effect of multiple input features influences the model’s predictions. | Partial Dependence plots | Reveals intricate and synergistic connections among features. | Visualizing and interpreting features can be difficult, especially when dealing with high-dimensional data. | Non-linear relationships—uncovers and visualizes complex, non-linear interactions among the features. |
Accumulated Local Effects plots. | Enhances insight into the model’s decision-making mechanism. | The computational complexity grows as the number of interacting features increases. | Holistic insight—provides a comprehensive understanding of how features collectively impact the model’s predictions. | ||
Individual Conditional Expectation Plots | Predictive power—evaluates the combined effects of interacting features on the model ‘s performance. | ||||
Interaction Values | |||||
Decision rules | Decision rules provide clear, human-readable guidelines derived from the model, such as “If age > 30 and income > 50k, then approve loan”. | Decision Trees | Provides clear and intuitive insights into the model’s predictions. | Might struggle to capture complex relationships in the data, leading to oversimplification. | Transparency—offers clear and interpretable explanations of the conditions and criteria used for decision making. |
Rule-Based Models | Easily understood by non-technical stakeholders. | Can be prone to overfitting, reducing generalization performance | Understandability—ensures ease of understanding by non-technical stakeholders and experts alike. | ||
Anchors | Model adherence—check that decision rules capture accurately the model’s decision logic without oversimplification. | ||||
Simplified models | Simplified models are interpretable machine learning models that approximate the behavior of a more complex black-box model | Generalized Additive Models. | Gives a balance between model interpretability and model complexity. | Might not capture the total complexity of the underlying data generating process. | Balance of complexity—achieves an optimal compromise between model simplicity and predictive performance. |
Interpretable Tree Ensembles. | Offers global insights into the model’s decision-making process | Needs careful model choice and tunning to maintain a good trade-off between interpretability and accuracy. | Interpretable representation—ensures that the offers transparent and intuitive insights into the original complex model’s behavior. | ||
Fidelity to original model—Assesses the extent to which the simplified model captures the key characteristics and patterns of the original complex model. |
Possible Research Areas | Suggestions |
---|---|
Hybrid Explanations [ ] | Combining multiple XAI techniques to provide more comprehensive and robust explanations for tabular data models [ ]. Integrating global and local interpretability methods to offer both high-level and instance-specific insights. |
Counterfactual Explanations | Generating counterfactual examples that show how the model’s predictions would change if certain feature values were altered [ ]. Helping users understand the sensitivity of the model to different feature inputs and how to achieve desired outcomes. |
Causal Inference [ ] | Incorporating causal reasoning into XAI methods to better understand the underlying relationships and dependencies in tabular data [ ]. Identifying causal features that drive the model’s predictions, beyond just correlational relationships. |
Interactive Visualizations | Developing interactive visualization tools that allow users to explore and interpret the model’s behavior on tabular data [ ]. Enabling users to interactively adjust feature values and observe the corresponding changes in model outputs [ ]. |
Scalable XAI Techniques [ ] | Designing XAI methods that can handle the growing volume and complexity of tabular datasets across various domains [ ]. Improving the computational efficiency and scalability of XAI techniques to support real-world applications. |
Domain-specific XAI | Tailoring XAI approaches to the specific needs and requirements of different industries and applications that rely on tabular data, such as finance, healthcare, and manufacturing. Incorporating domain knowledge and constraints to enhance the relevance and interpretability of explanations [ ]. |
Automated Explanation Generation [ ] | Developing AI-powered systems that can automatically generate natural language explanations for the model’s decisions on tabular data [ ]. Bridging the gap between the technical aspects of the model and the end-user’s understanding [ ]. |
The statements, opinions and data contained in all publications are solely those of the individual author(s) and contributor(s) and not of MDPI and/or the editor(s). MDPI and/or the editor(s) disclaim responsibility for any injury to people or property resulting from any ideas, methods, instructions or products referred to in the content. |
Share and Cite
O’Brien Quinn, H.; Sedky, M.; Francis, J.; Streeton, M. Literature Review of Explainable Tabular Data Analysis. Electronics 2024 , 13 , 3806. https://doi.org/10.3390/electronics13193806
O’Brien Quinn H, Sedky M, Francis J, Streeton M. Literature Review of Explainable Tabular Data Analysis. Electronics . 2024; 13(19):3806. https://doi.org/10.3390/electronics13193806
O’Brien Quinn, Helen, Mohamed Sedky, Janet Francis, and Michael Streeton. 2024. "Literature Review of Explainable Tabular Data Analysis" Electronics 13, no. 19: 3806. https://doi.org/10.3390/electronics13193806
Article Metrics
Article access statistics, further information, mdpi initiatives, follow mdpi.
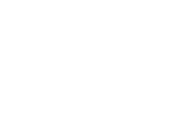
Subscribe to receive issue release notifications and newsletters from MDPI journals

An official website of the United States government
The .gov means it’s official. Federal government websites often end in .gov or .mil. Before sharing sensitive information, make sure you’re on a federal government site.
The site is secure. The https:// ensures that you are connecting to the official website and that any information you provide is encrypted and transmitted securely.
- Publications
- Account settings
The PMC website is updating on October 15, 2024. Learn More or Try it out now .
- Advanced Search
- Journal List
- Perspect Med Educ
- v.7(1); 2018 Feb

Writing an effective literature review
Lorelei lingard.
Schulich School of Medicine & Dentistry, Health Sciences Addition, Western University, London, Ontario Canada
In the Writer’s Craft section we offer simple tips to improve your writing in one of three areas: Energy, Clarity and Persuasiveness. Each entry focuses on a key writing feature or strategy, illustrates how it commonly goes wrong, teaches the grammatical underpinnings necessary to understand it and offers suggestions to wield it effectively. We encourage readers to share comments on or suggestions for this section on Twitter, using the hashtag: #how’syourwriting?
This Writer’s Craft instalment is the first in a two-part series that offers strategies for effectively presenting the literature review section of a research manuscript. This piece alerts writers to the importance of not only summarizing what is known but also identifying precisely what is not, in order to explicitly signal the relevance of their research. In this instalment, I will introduce readers to the mapping the gap metaphor, the knowledge claims heuristic, and the need to characterize the gap.
Mapping the gap
The purpose of the literature review section of a manuscript is not to report what is known about your topic. The purpose is to identify what remains unknown— what academic writing scholar Janet Giltrow has called the ‘knowledge deficit’ — thus establishing the need for your research study [ 1 ]. In an earlier Writer’s Craft instalment, the Problem-Gap-Hook heuristic was introduced as a way of opening your paper with a clear statement of the problem that your work grapples with, the gap in our current knowledge about that problem, and the reason the gap matters [ 2 ]. This article explains how to use the literature review section of your paper to build and characterize the Gap claim in your Problem-Gap-Hook. The metaphor of ‘mapping the gap’ is a way of thinking about how to select and arrange your review of the existing literature so that readers can recognize why your research needed to be done, and why its results constitute a meaningful advance on what was already known about the topic.
Many writers have learned that the literature review should describe what is known. The trouble with this approach is that it can produce a laundry list of facts-in-the-world that does not persuade the reader that the current study is a necessary next step. Instead, think of your literature review as painting in a map of your research domain: as you review existing knowledge, you are painting in sections of the map, but your goal is not to end with the whole map fully painted. That would mean there is nothing more we need to know about the topic, and that leaves no room for your research. What you want to end up with is a map in which painted sections surround and emphasize a white space, a gap in what is known that matters. Conceptualizing your literature review this way helps to ensure that it achieves its dual goal: of presenting what is known and pointing out what is not—the latter of these goals is necessary for your literature review to establish the necessity and importance of the research you are about to describe in the methods section which will immediately follow the literature review.
To a novice researcher or graduate student, this may seem counterintuitive. Hopefully you have invested significant time in reading the existing literature, and you are understandably keen to demonstrate that you’ve read everything ever published about your topic! Be careful, though, not to use the literature review section to regurgitate all of your reading in manuscript form. For one thing, it creates a laundry list of facts that makes for horrible reading. But there are three other reasons for avoiding this approach. First, you don’t have the space. In published medical education research papers, the literature review is quite short, ranging from a few paragraphs to a few pages, so you can’t summarize everything you’ve read. Second, you’re preaching to the converted. If you approach your paper as a contribution to an ongoing scholarly conversation,[ 2 ] then your literature review should summarize just the aspects of that conversation that are required to situate your conversational turn as informed and relevant. Third, the key to relevance is to point to a gap in what is known. To do so, you summarize what is known for the express purpose of identifying what is not known . Seen this way, the literature review should exert a gravitational pull on the reader, leading them inexorably to the white space on the map of knowledge you’ve painted for them. That white space is the space that your research fills.
Knowledge claims
To help writers move beyond the laundry list, the notion of ‘knowledge claims’ can be useful. A knowledge claim is a way of presenting the growing understanding of the community of researchers who have been exploring your topic. These are not disembodied facts, but rather incremental insights that some in the field may agree with and some may not, depending on their different methodological and disciplinary approaches to the topic. Treating the literature review as a story of the knowledge claims being made by researchers in the field can help writers with one of the most sophisticated aspects of a literature review—locating the knowledge being reviewed. Where does it come from? What is debated? How do different methodologies influence the knowledge being accumulated? And so on.
Consider this example of the knowledge claims (KC), Gap and Hook for the literature review section of a research paper on distributed healthcare teamwork:
KC: We know that poor team communication can cause errors. KC: And we know that team training can be effective in improving team communication. KC: This knowledge has prompted a push to incorporate teamwork training principles into health professions education curricula. KC: However, most of what we know about team training research has come from research with co-located teams—i. e., teams whose members work together in time and space. Gap: Little is known about how teamwork training principles would apply in distributed teams, whose members work asynchronously and are spread across different locations. Hook: Given that much healthcare teamwork is distributed rather than co-located, our curricula will be severely lacking until we create refined teamwork training principles that reflect distributed as well as co-located work contexts.
The ‘We know that …’ structure illustrated in this example is a template for helping you draft and organize. In your final version, your knowledge claims will be expressed with more sophistication. For instance, ‘We know that poor team communication can cause errors’ will become something like ‘Over a decade of patient safety research has demonstrated that poor team communication is the dominant cause of medical errors.’ This simple template of knowledge claims, though, provides an outline for the paragraphs in your literature review, each of which will provide detailed evidence to illustrate a knowledge claim. Using this approach, the order of the paragraphs in the literature review is strategic and persuasive, leading the reader to the gap claim that positions the relevance of the current study. To expand your vocabulary for creating such knowledge claims, linking them logically and positioning yourself amid them, I highly recommend Graff and Birkenstein’s little handbook of ‘templates’ [ 3 ].
As you organize your knowledge claims, you will also want to consider whether you are trying to map the gap in a well-studied field, or a relatively understudied one. The rhetorical challenge is different in each case. In a well-studied field, like professionalism in medical education, you must make a strong, explicit case for the existence of a gap. Readers may come to your paper tired of hearing about this topic and tempted to think we can’t possibly need more knowledge about it. Listing the knowledge claims can help you organize them most effectively and determine which pieces of knowledge may be unnecessary to map the white space your research attempts to fill. This does not mean that you leave out relevant information: your literature review must still be accurate. But, since you will not be able to include everything, selecting carefully among the possible knowledge claims is essential to producing a coherent, well-argued literature review.
Characterizing the gap
Once you’ve identified the gap, your literature review must characterize it. What kind of gap have you found? There are many ways to characterize a gap, but some of the more common include:
- a pure knowledge deficit—‘no one has looked at the relationship between longitudinal integrated clerkships and medical student abuse’
- a shortcoming in the scholarship, often due to philosophical or methodological tendencies and oversights—‘scholars have interpreted x from a cognitivist perspective, but ignored the humanist perspective’ or ‘to date, we have surveyed the frequency of medical errors committed by residents, but we have not explored their subjective experience of such errors’
- a controversy—‘scholars disagree on the definition of professionalism in medicine …’
- a pervasive and unproven assumption—‘the theme of technological heroism—technology will solve what ails teamwork—is ubiquitous in the literature, but what is that belief based on?’
To characterize the kind of gap, you need to know the literature thoroughly. That means more than understanding each paper individually; you also need to be placing each paper in relation to others. This may require changing your note-taking technique while you’re reading; take notes on what each paper contributes to knowledge, but also on how it relates to other papers you’ve read, and what it suggests about the kind of gap that is emerging.
In summary, think of your literature review as mapping the gap rather than simply summarizing the known. And pay attention to characterizing the kind of gap you’ve mapped. This strategy can help to make your literature review into a compelling argument rather than a list of facts. It can remind you of the danger of describing so fully what is known that the reader is left with the sense that there is no pressing need to know more. And it can help you to establish a coherence between the kind of gap you’ve identified and the study methodology you will use to fill it.
Acknowledgements
Thanks to Mark Goldszmidt for his feedback on an early version of this manuscript.
PhD, is director of the Centre for Education Research & Innovation at Schulich School of Medicine & Dentistry, and professor for the Department of Medicine at Western University in London, Ontario, Canada.
Thank you for visiting nature.com. You are using a browser version with limited support for CSS. To obtain the best experience, we recommend you use a more up to date browser (or turn off compatibility mode in Internet Explorer). In the meantime, to ensure continued support, we are displaying the site without styles and JavaScript.
- View all journals
- Explore content
- About the journal
- Publish with us
- Sign up for alerts
- Review Article
- Open access
- Published: 27 September 2024
Mapping the main research themes in digital human resources
- Laura García-Fernández 1 ,
- Marta Ortiz-de-Urbina-Criado ORCID: orcid.org/0000-0001-7527-6798 1 &
- María-José García-López 1
Humanities and Social Sciences Communications volume 11 , Article number: 1267 ( 2024 ) Cite this article
Metrics details
- Business and management
- Information systems and information technology
The COVID-19 pandemic sped up the digitalization process and revolutionized the world of the digital employee. And today, advances in artificial intelligence are having a major impact on the field of Digital HR. In that context, further literature review work is needed on the term Digital HR to complement previous studies and lay the foundation for more pioneering literature on this topic. Then, the aim of this paper is to provide a framework for organizing the main themes discussed in the pioneering literature on digital HR by answering the following research question: What is the knowledge structure of the research in the field of digital human resources? An adaptation of the PRISMA model is used to structure the research design. Applying a mixed methodology, this paper uses a bibliometric technique to identify the main topics studied in Digital HR. Subsequently, in-depth analysis and logical reasoning are applied and a model is proposed based on four questions (how, what, where, who) in order to understand and develop research on digital HR. The RQ4 Digital-HR model constitutes a useful tool in academic, practical, professional, and social contexts. It is worth highlighting the importance of the inclusion of artificial intelligence in the daily processes of a company, and therefore in the progress of the proposed research topic.
Introduction
The world is witnessing constant change due to digitalization and its effects on companies and their staff. The pandemic accelerated the adoption of digital technologies, which had an immense impact on all business sectors and brought about permanent changes in the workplace (Gkinko and Elbanna, 2023 ). Many organizations started working in hybrid mode, combining digital ways of working with the traditional ways of working prior to the pandemic. Moreover, the use of digital technologies and the acceptance of more agile and flexible procedures and rules have changed the way in which work is being done (Mićić and Mastilo, 2022 ).
In general, our daily lives have been altered by technological advances, one of the most innovative being the advances in artificial intelligence, which is transforming the way people carry out their daily activities of work, communication, and decision making (Duke, 2022 ). The concept of artificial intelligence seems to be relatively recent, and in many cases, its true meaning or significance is unclear. However, it was not until the 2010s that the AI paradigm was reconfigured to be based on the classification and storage of massive data (Cetindamar et al. 2024 ).
Human resource management has evolved over time. Twenty-five years ago, its main focus was on implementing practices that promoted the development of organizations. However, the need for organizations to adapt to more competitive environments has forced businesses to adjust the traditional business management model, moving from strategic management to a more sustainable management approach (Villajos et al. 2019 ). One of the main factors influencing the adaptation of human resources management to the new sustainable management model has been digitalization (Le et al. 2024 ). Through digitalization, all employees, professionals, managers and business leaders, who are key to making the necessary changes to increase workplace productivity, can see their tasks facilitated through this phenomenon. In this context, human resources management develops practices that promote the welfare of the employee and the company (Le et al. 2024 ). Thus, in recent years, and especially during the COVID-19 pandemic, Digital Human Resources (Digital HR) has received a great deal of attention, particularly regarding Digital Employees and Digital Leaders. Advances in artificial intelligence are having a major impact on the field of Digital HR (Gkinko and Elbanna, 2023 ). Although there are still few publications on the acceptance of the effects of AI on workers and how the current increase in the use of digital technologies affects the skills and expectations of the digital workforce (Alan, 2023 ; Cetindamar et al. 2024 ).
In the academic context, there has been a surge in the literature on Digital HR. However, literature review studies on this topic are lacking, with most of them focusing on analyzing the digital workplace phenomenon (De Moraes et al. 2024 ; Marsh et al. 2022 ; Mićić and Mastilo, 2022 ), digital employee experience (Moganadas and Goh, 2022 ), and workforce training in digital workplaces (Patino and Naffi, 2023 ). Only two reviews address the issue of Digital HR more generally. Theres and Strohmeier ( 2023 ) conducted a meta-analysis to analyze theories applied in research on Digital HRM adoption and proposed a unified theory. Alan ( 2023 ) performed a co-word analysis, considering Electronic Human Resources Management (e-HRM) as the main term, and analyzed previous literature found in the Web of Science (WoS) for the period of 2012–2022.
Thus, further literature review work is needed on the term Digital HR that analyzes the literature published before and during the pandemic to complement previous studies and lay the foundation for more pioneering literature on this topic. The interest in analyzing changes during the pandemic is motivated by the fact that adapting to the new context requires new human resources actions that are closely related to the phenomenon of digitalization. Digital HR is a constantly evolving topic, and pioneering studies are fundamental to understanding this new phenomenon. Today, there is also the challenge of appropriately and ethically adopting artificial intelligence in the context of human resources (Cetindamar et al. 2024 ; Gkinko and Elbanna, 2023 ). In the face of a novel topic, it is important to gain an overview of the aspects studied, to understand the changes that have occurred around the pandemic, and to provide a logical framework of analysis by which to explore such phenomena.
This paper aims to provide an overview of the pioneering research landscape in the field of digital HR, filling in some of the existing research gaps. As a complement to Alan’s ( 2023 ) work, this research will focus on the topic of ‘digital HR’ and conduct a co-word analysis to identify the main themes studied. Moreover, a second step, which is not usually included in previous literature reviews on this topic, will be carried out to detect the applications of digital human resources. To this end, a model based on questions (how, what, where, who) is proposed to facilitate the understanding and development of digital HR research.
Thus, the aim of this study is to provide a framework for the organization of the main themes that are discussed in the pioneering literature on Digital HR. The research question addressed is What is the knowledge structure of the research in the field of digital human resources? To answer this question, the Background section is developed and a mixed methodology is applied, adapting the PRISMA process. A bibliometric technique is used to identify the main topics studied in Digital HR. Subsequently, in-depth analysis and logical reasoning are applied to propose a model and some lines of future research. Finally, the Conclusion section contains theoretical and practical implications, the study limitations, and future lines of research.
This paper is an original contribution. Literature reviews, and more on rapidly developing novel topics, play an important role in advancing research as they help to synthesize and organize existing knowledge and identify areas or topics for future research. This article proposes an integrative review (Patriotta, 2020 ) that offers another voice to guide and write new articles on digital human resources. Authors such as Post et al. ( 2020 ) have also highlighted the importance of literature reviews as they can serve several purposes such as helping researchers understand the research topic, discerning important and under-examined areas and connecting research findings from disparate sources to create new perspectives and phenomena. Moreover, the topic “Digital HR” calls for looking for models that help connect academic research with the business world. As Markman ( 2022 ) proclaims, academia is challenged to develop research that addresses current problems affecting people, business and society to make the world a better place. In that line, the RQ4 Digital-HR model constitutes a useful tool for academic, practical, professional, and social contexts.
The global pandemic has accelerated digital transformation in every sense, and the rise of digital technology in the workplace is unstoppable (Kalischko and Riedl, 2021 ). Technology plays a vital role in our day-to-day lives. Digitization has arrived, yet what that means or entails at a work and/personal level remains unclear. According to Kraus et al. ( 2022 ), it is necessary to have a fundamental understanding of literature reviews as independent studies. Therefore, the key texts must be identified that lay the foundations of Digital Human Resources Management (Digital HRM) before undertaking a bibliometric study.
Main concepts
Few papers over the last decades have provided a clear, agreed-upon definition of the term “Digital Human Resources Management” that is shared by the scientific community. Most papers have only superficially addressed the whole social and economic context that affects the new confection of digital employee models. Moreover, papers have tended to narrow their focus to a specific aspect of human resources management (Alan, 2023 ; Costa et al. 2022 ), digital employee experience (Moganadas and Goh, 2022 ), and job performance (Kalischko and Riedl, 2021 ; Marsh et al. 2022 ), analyzing the situation individually and rather than as a whole. Therefore, the starting point for this study is to introduce some of the terms or concepts commonly used in previous literature on digital HR. Two widely used terms are “digital worker” and “digital employee”. A key resource in any company is the employee, the one who can contribute to superior and solid performance over time (Moganadas and Goh, 2022 ). For example, Fuchs ( 2014 ) defines digital employees as the workforce required for the existence, use, and application of digital media. Other studies define digital employees as those employees whose work is performed primarily using digital resources (Nelson, 2018 ). IBM ( 2024 ) states that “in the past, the term ‘digital worker’ described a human employee with digital skills, but more recently, the market has defined it as a category of software robots, which are trained to perform specific tasks or processes in partnership with their human colleagues.”
Another concept used is “digital workplace.” As management has adapted to new technologies, the workplace has also had to adapt. This new leadership style brings with it concepts such as flexibility, which in this context refers to the non-limitation of the workspace to a specific physical location. This new digital workplace refers to the set of technologies that employees use to perform their functions (Marsh, 2018 ) and includes, among others, the intranet, communication tools, e-mail, CRM, etc. It also refers to a set of procedures and rules that maximize productivity and improve collaboration, communication, and knowledge management (Mićić et al. 2022 ). Some researchers use the term “digital labor”, which initially referred to the unpaid work performed by consumers online during leisure time. However, this term is now used to describe all work in which digital technology plays a role (Jarrett, 2022 ). The term has also been used to describe employees who work independently, receiving low wages and no social security, in business models supported by digital platforms, such as Uber (Fumagalli et al. 2018 ), or to describe the workforce that uses other business models that are also based on digital platforms, such as Facebook or Google, and that capture information to transform it into big data (Fuchs and Sevignani, 2013 ).
In that context, another important concept is digital platform. Digital platforms are transforming almost every industry today (Reuver et al. 2018 ). They are continuously evolving and becoming increasingly complex. These digital platforms are the ones that facilitate online communities of consumers (Reuver et al. 2018 ). While there are several definitions of digital platforms that refer to the codes, software, and hardware of which they are composed, for this study the most suitable definition of digital platform would be the environment in which companies combine all the information available from their stakeholders to generate or co-create value (Karhu et al. 2018 ). According to Murati ( 2021 ), a digital platform is an open infrastructure that exercises a facilitator role or a high level of control and influence over providers and users.
The meeting point of each one of these concepts is the term Human Resource Management, which is understood as the processes that involve activities from recruitment to salary management and that are carried out simultaneously (Alan, 2023 ). All of these processes have been equipped with more technology and innovative methods over time. Thus, the concept has evolved to Digital Human Resource Management (HRM), understood as the set of software, hardware, and digital resources designed to automate the HR function (Jani et al. 2021 ; Marler and Parry, 2016 ), or in other words, to develop consistent, efficient and high-quality HR practices through the use of digital transformation and new technologies (Bondarouk and Brewster, 2016 ).
Previous literature reviews
Previous literature reviews established a set of definitions that, despite using common concepts, have left nuances that have yet to be fully addressed in subsequent works. Most of the work that reviews previous literature has focused on studying digital workplaces. Mićić and Mastilo ( 2022 ) conducted a systematic literature review on the digital transformation of the workplace and employees’ workplace preferences. The search terms used were “digital workplace”, “COVID-19”, and “innovation”, and the search was limited to English language papers published after 2010. The benefits of digital workplace transformation are analyzed and the critical success factors and significant challenges are identified.
Marsh et al. ( 2022 ) studied the application of digital technologies in the workplace with a particular focus on their dark side. They conducted an integrative literature review and limited the search to papers published between January 2007 and June 2020 that were written in English and carried out in Western countries only (in the United States, Europe, Canada, Australia, Latin America, and New Zealand). De Moraes et al. ( 2024 ) conducted a systematic review of the literature on the design of digital workplaces. Their main results include a definition of digital workplace and a four-phase model with guidelines for designing digital workplaces. Patino and Naffi ( 2023 ) conducted a systematic review of training approaches and resources for workforce development in digital workplaces. Using the PRISMA model, they analyzed articles published between 2020 and 2022. Their paper offers research-based perspectives and recommendations for employee training in highly digitalized workplaces.
Another aspect that has been studied is the experience of the digital worker. Moganadas and Goh ( 2022 ) discuss the concept of digital employee experience (DEX). They conducted a comprehensive literature review on DEX by analyzing the content of academic publications and professional reports. They used the Scopus and Google Scholar databases to identify “DEX” or “digital employee experience” in their title, abstract, and keywords and found 17 articles between 2016 and 2022. To complement these papers, they included grey literature to identify studies that addressed a similar topic, such as digital transformation, digital workplace, and employee experience.
Finally, a few papers have reviewed the literature on human resource management in a digital environment. Theres and Strohmeier ( 2023 ) analyzed the phenomenon of digital HRM. In their paper, they present an overview of the theories applied in digital HRM adoption research and propose a unified theory. To test their theory, they performed a combination of meta-analysis and structural equation modelling. Alan ( 2023 ) presented a systematic bibliometric analysis of electronic human resource management (e-HRM) by conducting a literature search in the Web of Science (WoS) for the period of 2012–2022.
Methodology
Figure 1 presents the methodological process used in this study. The methodological design used includes two parts. In the first, a multi-step process has been followed to perform the bibliometric analysis: sample selection, filtering of documents and keywords, and co-word analysis. In the second, a reflexive analysis was carried out. To facilitate the understanding of the process followed, the PRISMA 2020 statement has been adapted, which has been designed primarily for systematic reviews of studies (Moher et al. 2010 ; Page et al. 2021 ). The adaptation of the PRISMA process provides a more transparent view of the methodology used and the analyses carried out.
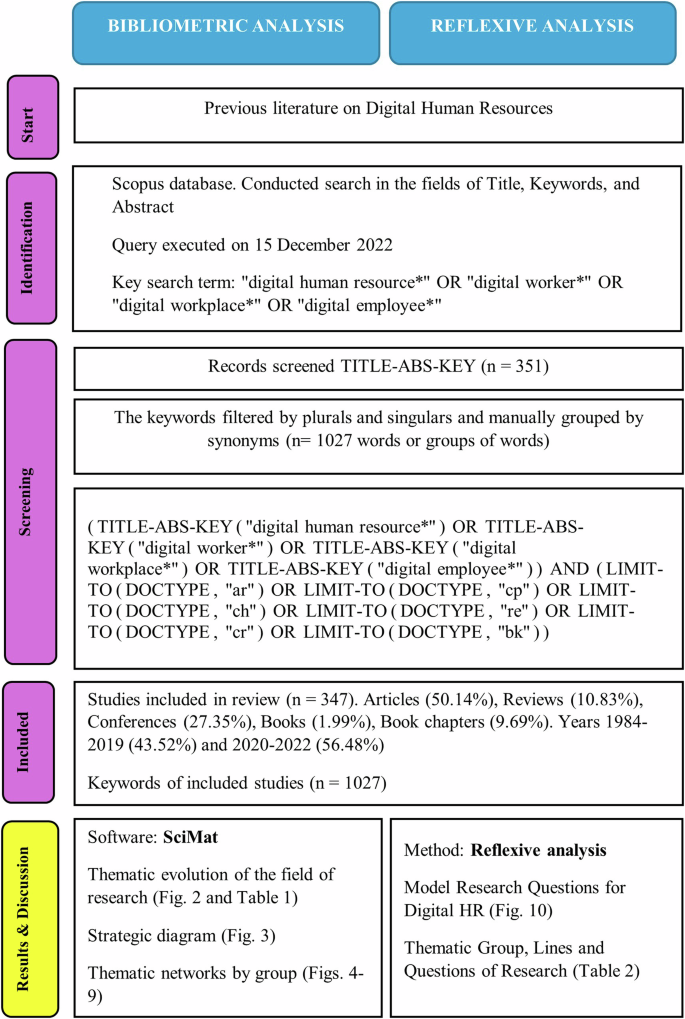
Own elaboration based on the PRISMA model.
The Scopus database was used. There is an open debate regarding whether Scopus or WoS is superior. Both have advantages and disadvantages (Stahlschmidt and Stephen, 2020 ). The Scopus database was chosen for this paper because it offered a larger sample of documents than did WoS. Although the research on Digital HR began over 35 years ago, most of the articles have been published in the last three years, demonstrating the impact of the COVID-19 pandemic on this topic. Until the year 2016, contributions were sporadic, and it is not until a year later, in 2017, that the research begins to approach 25 articles per year. Of the total of articles (347), 56% (196) were published between 2020 and 2022, with 2021 being the most important year, when a total of 82 articles (25%) were published.
A co-word analysis in conjunction with the SciMat program was used to identify the various themes covered in the literature on Digital HR (Cobo et al. 2012 ). Of the many tools that enable co-word analysis, SciMat was chosen for its ability to carry out the analysis with simplicity and rigor. Moral-Muñoz et al. ( 2019 ; 2020 ) describe the various tools that are available for bibliometric analysis and comment on SciMat as being a valid tool for co-word analysis. SciMat was suitable for achieving the objective of this paper because it analyzes the keywords of selected articles and calculates the strategic diagrams and networks for each thematic group. Moreover, SciMat incorporates all the necessary elements (methods, algorithms, and measurements) for performing a co-word analysis and obtaining its visualizations (Cobo et al. 2012 ).
Regarding the strategic diagram, centrality and density are calculated for each thematic group (Cobo et al. 2018 ). Centrality is a measure of the importance of a theme in the development of a field of knowledge. Density reflects the strength of a network’s internal relationships, thus identifying the level of development of that theme. The strategic diagram classifies the themes into four groups (Cobo et al. 2018 ). In the upper-right quadrant are the motor themes, which comprise themes that have strong centrality and high density. In the upper-left quadrant are the well-developed and/or isolated themes. The themes in the lower-left quadrant are presented as emerging or disappearing themes, while in the lower-right quadrant are themes that are considered basic and transversal themes.
This section presents the results of the co-word analysis. The bibliometric technique is suitable for identifying the knowledge structure of a research topic. Given the volume of articles published between 2020 and 2022, two periods of analysis were carried out to compare the networks that emerged prior to and after the COVID-19 pandemic. Figure 2 shows the evolution of all the topics mentioned, their typology, and how, depending on the period, they transform into a new topic.
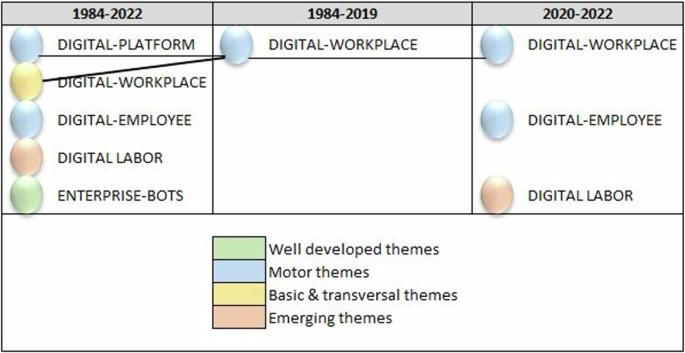
Results from SciMat, diagram composed of themes by number of documents for all the periods.
Table 1 presents the evolution that Digital HR research has experienced during these years.
Main themes studied in Digital HR
Regarding the total period (1984–2022), previous research focused on “digital workplace” and “digital platform” and “digital employee” as the motor themes. “Digital labor” appears as an emerging topic and as something remarkable. Despite not being connected to the human resources area, this entire digitization process is linked to the topic “enterprise bots”, a concept that had previously been highly developed in scientific fields. During the pre-COVID period (1984–2019), the motor theme was the “digital workplace”. During the COVID period (2020–2022), the motor themes were “digital employee” and “digital workplace”. Lastly, the emerging theme for all the periods is “digital labor”.
During the first period (1984–2019), only one theme, “digital workplace”, is positioned as a motor theme. It makes sense that after the 4 th industrial revolution, developed between 1950 and 1970, a study period would begin regarding how this digitization has affected the workspaces as well as how to continue innovating and improving them. Companies have needed workplaces to be transformed from a traditional perspective to a digital one (Colbert et al. 2016 ; Kaarst-Brown et al. 2018 ), since this change is key to organizational success (Colbert et al. 2016 , Köffer, 2015 ).
In the 2020–2022 period, two additional topics to those appearing in the previous period emerge. These are “digital employee” and “digital labor”, positioned as a motor and an emerging theme, respectively. These topics correlate with what occurred during the pandemic, which forced the digitalization of all types of situations. As a result, the research on this area has focused on the employee and, above all, the digitization of work that, as mentioned, appears as an emerging topic.
Based on these results and for the completion of the analyses, a manually and logical regrouping of themes was conducted in the SciMat program, and another strategic diagram was identified. Figure 3 , which presents the strategic diagram obtained from this new analysis, shows that the motor themes are “digital platform” and “workplace”. “Manufacturing”, “digital employee”, and “social media” are well-developed themes. “Digital”, “learning”, and “labor” are positioned as basic themes. Finally, the emerging theme is “artificial intelligence”. A logical knowledge structure of the study topics can be observed. On the one hand, what emerges are the themes related to the more digital aspect of work, namely “digital”, “digital platform”, and “digital employee”. On the other hand, there are items that refer to the more physical aspect, “labor” and “workplace”. Tangential to the most digital aspect linked to work is the communication channel or media used at work, “social media”. In turn, in the main sector where the research is applied or where the literature has further explored these issues, the theme of “manufacturing” is also well defined.
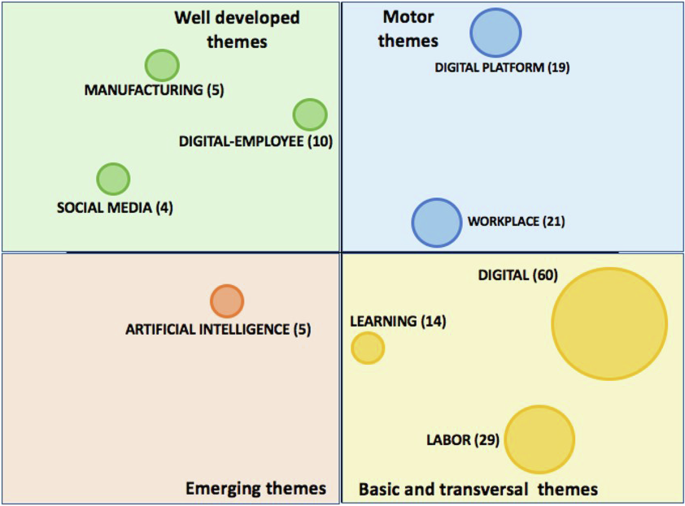
Results from SciMat, diagram composed of themes by a number of documents for all the periods.
Also evident in the diagram is the channel through which employees can progress in the work environment, through “learning”, a Human Resources practice that has been developed for some time but has become more crucial in recent years. Learning is the key to employees acquiring digital competencies and feeling comfortable in digital work environments. Finally, as an emerging topic, is everything related to “artificial intelligence”. This topic, of relatively recent creation, has all the works published in the year 2022 or later, and is creating a highly critical space in the business environment, and in this particular case, in everything related to Human Resources and how to implement it in departmental processes.
Thematic networks in Digital HR
It is interesting to know the thematic networks in which the most significant keyword (the one with the highest centrality) is placed at the center. The size of each node represents the number of documents containing that word, and the thickness of the line indicates the strength of the association between those topics.
Digital platform
Analysis of the “Digital Platform” subnetwork (Fig. 4 ) for the period 1984–2022, reveals four documents and a wide network of terms that correlate with each other. The most notable relationships are those related to health care. However, within our scope, there are several studies that focus on the use of digital platforms as a means of offering work in the “gig economy.” Taylor et al. ( 2017 ) define the concept of Gig Economy as the use of applications or platforms for work.

Results from SciMat cluster network for the digital platform.
The analysis also revealed the importance of collaborative work for the improvement of digital platforms, as shown through the connections between the terms “collaborative designs” and “co-creation”. The research also showed two important advances in what has been studied in recent years: the flexibility that this type of work facilitates (Soriano and Cabanes, 2020 ) and how these new jobs can change the lifestyle of digital employees (Graham et al. 2017 ).
Digital workplace
The “Digital workplace” network (Fig. 5 ) for the period 1984–2022 includes six documents and shows that the most important keywords in the cluster are “digital transformation” and “artificial intelligence”. Again, it is crucial for this network to talk about “collaboration”, as well as “cross-functional teams” and their “dynamic capabilities” that play a special role in developing the digital workplace. As Selimović et al. ( 2021 ) posit, the inclusion of the employee in the decisions on digital transformation is a key to its success. Moreover, the use of artificial intelligence, through the “chatbots” makes improvement in the workplace possible (Cetindamar et al. 2024 ). In both cases, the focus is placed on the inclusion of the employee as a key part of these processes. This demonstrates a strong relationship between digital and emotions in the cluster, since understanding how the use of technology affects employees’ emotions (Gkinko and Elbanna, 2022 ) is one of the most relevant topics in the current research.
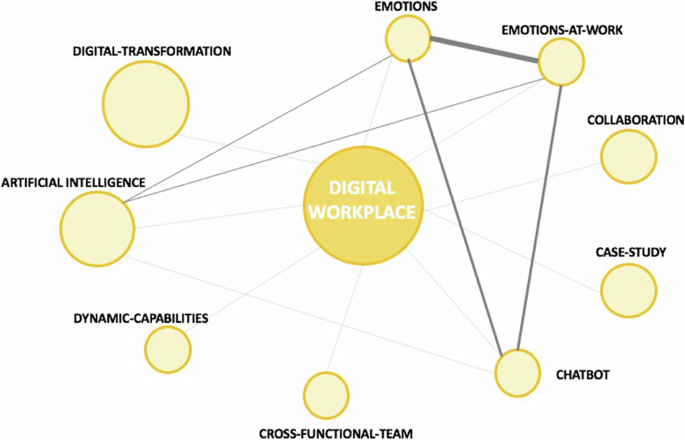
Results from SciMat cluster network for the digital workplace.
The analysis of the “Digital workplace” network (Fig. 6 ) for the period 1984–2019, which contains 15 documents, reveals the strong presence of terms related to a collaborative work environment, such as “collaboration”, “cloud”, “cloud computing”; or even advancing further in the collaboration itself, it becomes necessary to talk about “digital platforms” or “crowdsourcing”, as means for it, being the key tools for developing the digital workplace (White, 2012 ; Attaran et al. 2019 ). Indeed, the most remarkable aspect of the network is the strong connection between “cloud computing” and “mobile working”. It must be considered that during these years, prior to the COVID-19 pandemic, the now-standardized option of mobile working was merely a practice applied by a few companies. Thus, it makes sense that during these years of strong digitization, research focuses on it. There is also one term, “artificial intelligence” (here also mentioned as “social software”), that researchers start to investigate during these years, since its use in the digital workplace is continuing to increase (Martensen et al. 2016 ) and, as could be seen throughout the paper, it will also become of vast importance for other networks.
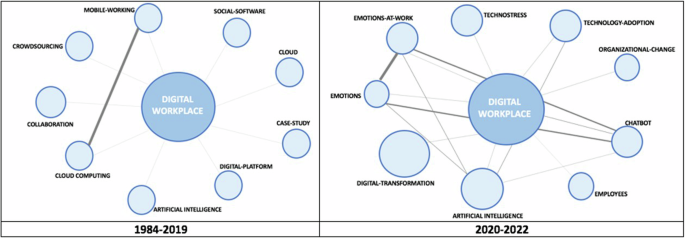
For the last years (2020–2022), “Digital workplace” network (Fig. 6 ) contains the highest number of documents (16) and shows two remarkable themes “digital transformation” and “artificial intelligence”. In the figure can be seen a triangle formed by “emotions”, “emotions at work”, and “chatbot”, as employee users experience a connection emotion when using artificial intelligence (Gkinko and Elbanna, 2022 ), and there is an effort to understand how employees will accept these new systems in the enterprise context (Brachten et al. 2021 ). Moreover, in these recent years, the changes that companies must make to achieve the digitalization of the workplaces takes on a special relevance. Thus, it is not surprising that the investigation is linked to “organizational change” and “technology-adoption”. It should not be overlooked that none of these changes would be possible without including the “employees” in said decisions (Cetindamar et al. 2024 ).
There is a topic that remains throughout the analysis: “artificial intelligence” (Fig. 6 ). However, there is a positive evolution between the topics analyzed prior to the pandemic and those analyzed after it. In the first period (1984–2022), the topics were focused on how the workplace should be or what it should contain and how it should be digitized, as well as on platforms and software and everything related to the cloud. However, during the pandemic period, some changes were perceived, with the introduction of themes arising from having been forced to implement the digital work modality. These topics include technostress, emotions, and employees, as well as everything related to organizational change.
Digital employee
The “Digital employee” network (Fig. 7 ) for the period 1984–2022 contains 10 documents and shows, as previously mentioned, the relevance of the pandemic in this research. In this sense, it is crucial to understand how this situation affected the employee, the work itself, and the life experience of employees (Muszyński et al. 2021 ). Above all, it shows a strong connection between the concepts related to “Robotic Process Automation” (RPA), “digital automation process”, and “software robot”, as a means to increasing productivity in a company, leaving the routine tasks to RPA and assigning employees to perform more difficult tasks (Choi et al. 2021 ). Clearly, it is crucial to talk about the “digital competencies” that employees have or need to acquire to be included in the “new work” that globalization is forcing us to implement.

Results from SciMat cluster network for digital employees.
The “Digital employee” network, for the last years (2020–2022) (Fig. 7 ), with eight documents, shows that there have been no changes in recent years compared to what was already being studied. The only difference is that the research in these years does not focus on the new types of work, implemented post-pandemic, but studies how to improve the implementation of RPA (Costa et al. 2022 ) to achieve better economic results and an improved digital employee experience.
Digital labor
The “Digital labor” network (Fig. 8 ) for the years 1984–2022, contains nine documents and shows a star-shaped network characterized by the presence of keywords that only correlate with the cluster topic. The main theme of the cluster is the work itself with its main versions, with research on the best type of work being very common (Babapour Chafi et al. 2022 ): office work or digital work (commonly called digital nomadism). There is also an important connection with the information society.
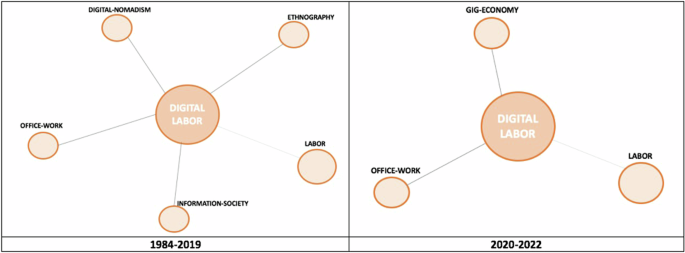
Results from SciMat cluster network for digital labor.
“Digital Labor”, for the last years (2020–2022), provides six documents (Fig. 8 ). Studies related to the “gig economy” and the types of jobs related to digital platforms proliferate during this period. In addition, once the pandemic period was over, it was expected that employees would return to office work. Thus, there arises a need to understand which work model (remote, face-to-face, or hybrid) is more productive and which is more valued by the employee (Babapour Chafi et al. 2022 ).
A comparison of studies prior to the pandemic with those of recent years reveals that initially there were several issues related to digital work, whereas in recent years these have been reduced to two issues: office work or work through digital platforms.
Enterprise bots
The “Enterprise bots” network for the period 1984–2022 (Fig. 9 ) contains two documents that co-relate two concepts, virtual assistants and virtual agents, as being crucial to understanding the differences between them, and above all, to understanding the differences in use between the individual and the business context (Stieglitz et al. 2018 ). The focus was therefore on teaching an employer how to effectively introduce these systems in the company (Brachten et al. 2021 ).
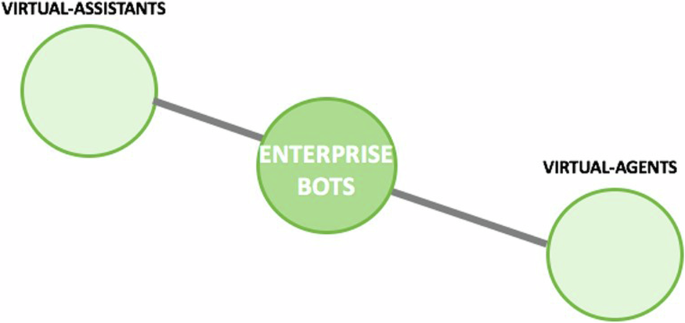
Results from SciMat cluster network for enterprise bots.
The results of this study complement those of previous literature review studies. Alan ( 2023 ) focused on the term Electronic Human Resources Management (e-HRM) and conducted a review of the literature included in WoS from 2012 to 2022. Our study focused on the term “digital human resources” and used the Scopus database. Our study also included pioneering literature up to 2022 and an analysis of the differences between the pre-pandemic period (1984 to 2019) and the peak years of the pandemic (2020–2022).
Alan ( 2023 ) categorizes the research on this topic into three groups: the theoretical studies and theories used in the studies reviewed, empirical qualitative studies, and empirical quantitative studies. Alan ( 2023 ) presents summary tables for each category that include following information: related theoretical framework, related terms, studies, typology of study, aim of the study, and the main findings and propositions. In a complementary way, this current paper presents the studied themes and classifies them into four groups according to the strategic diagram and analyses the networks for each thematic group. Additionally, based on the results obtained in the previous section, a process of analysis and reflection was carried out to establish the roadmap of topics studied and to define the emerging and future topics. Four main research questions (how, what, where, who) are considered to propose a model (Fig. 10 ).
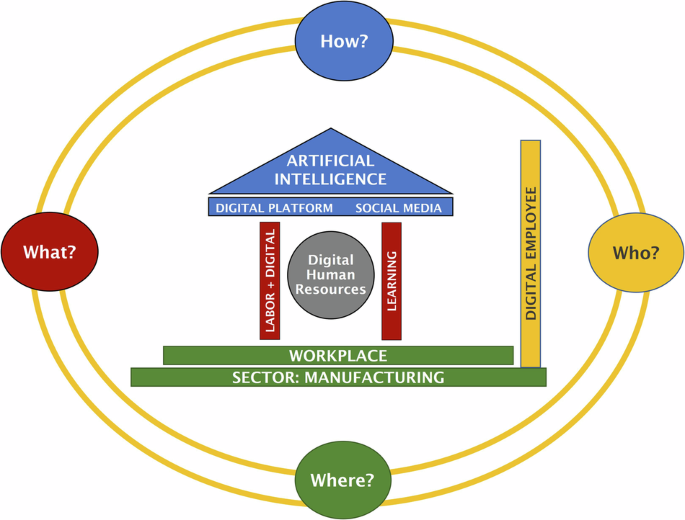
Own elaboration.
The RQ4 Digital-HR model presents four fundamental questions for understanding and developing research on digital HR -Research Questions for Digital HR.
The basis of the proposed model (Fig. 10 ) refers to “where” to apply it. The results of the analysis show that there is a sector where deep research on the subject has already been carried out, the manufacturing sector. However, the research should not stop there. Future research should take this model to other sectors of much greater complexity and scope, such as the service sector.
The pillars that support the model, “the what,” are on two levels: the advances in the digitalization of work on the one hand and, on the other hand, all the learning that a company can guarantee to its employees and that employees are able to assimilate. Clearly, the cross-cutting issue, “the who,” is the digital employee, the workforce member that drives the change, the one who is able to implement any Human Resources practice, and therefore the one who is able to assimilate business-driven change.
The roof of the model is “the how.” The implementation of all the changes we are forced to make is only possible through the implementation and improvement of three items in our daily processes: firstly, the digital platforms that we use every day at work, secondly, the management of information through the channels provided by the company, and thirdly—and most importantly—the inclusion of artificial intelligence in all our processes, as a means of improving productivity. This technology is transforming, and revolutionizing, the future of workspaces to make them more productive (Gkinko and Elbanna 2022 ), but again studies on the subject do not address how to accomplish this. Most of the texts reviewed on the subject focus on investigating some aspect of the implementation of artificial intelligence systems and their errors (Costa et al. 2022 ) or on employees’ acceptance of or trust in such technology (Gkinko and Elbanna, 2023 ). However, the work of Gkinko and Elbanna ( 2022 ) offers a starting point for how to incorporate this technology into a company, since the emotions of employees need to be taken into account when such tools are being created in order to facilitate their inclusion in day-to-day activities.
This makes it vital to focus the research on Digital HR, which requires researchers to collaborate to determine the what, where, how, and who. Based on the questions in the model, a further step has been taken to identify the aspects that would be interesting to analyze in future work to answer each of these fundamental questions.
WHAT: In reference to this, it is important to discover what digital processes should be introduced in our daily lives, what learning tools will help us to channel digitization, and what new labor trends can be found in the post-COVID stage.
WHERE: Future research should focus on analyzing what kind of workspaces exist in the labor market. This first line of research will undoubtedly lead towards the different sectors of activity, so it is also important to see what we know about the different sectors and their relationship with Digital HR, especially in the service sector, since globally it is the most present sector of activity.
HOW: Research should continue to determine how to do this and, as broken down in the table, it is important to address how to adopt digital platforms in the work environment, how to adapt existing social networks to the business context, and how to apply new artificial intelligence models.
WHO: As mentioned in this paper, digital HR is a transversal entity in all these lines of research. However, it is not left out of the future lines of research since it is essential to understand who this digital HR includes.
Finally, based on the results of this study (thematic groups) and the proposed model (questions), Table 2 presents a proposal for future research lines and questions.
The proposed model revolves around four fundamental research questions (Fig. 10 ). The importance of researchers developing the ability to formulate questions has an epistemological background expressed by Bachelard ( 1982 , p. 16) as ‘for a scientific spirit all knowledge is an answer to a question. If there was no question, there can be no scientific knowledge’. It should be noted that the quality of the questions asked is closely related to the prior knowledge they have about a given topic (Neber and Anton, 2008 ). Systematic questioning about different phenomena fosters meaningful learning by drawing on prior knowledge in a non-arbitrary and non-literal way (Moreira, 2000 ). Furthermore, knowing the background of a subject facilitates scientific modelling, an activity inherent to science, which can be understood as a process of constructing models for the purpose of apprehending reality (Giere, 1988 ) and providing answers to questions formulated about real facts or assumptions (Halloun, 1996 ). The model presented in Fig. 10 and developed in Table 2 , helps to logically order the themes studied in the previous literature and to propose emerging and current themes of great interest for the development of the literature on digital HR.
In addition, the model helps to sort out what company should focus on meeting the needs of its employees without leaving its own needs behind, first the basis, then the pillars and finally the roof. In this regard, it is important to start managing the workers’ workplaces to adapt them to the environment. Subsequently, the training needs of employees must be addressed, along with the necessary adaptations to enable them to function digitally. Thirdly, companies must develop internal and external communication systems that allow them to be in contact with all their stakeholders. Only having developed these points will be able to focus on meeting current social demands and introducing artificial intelligence in their daily work.
Therefore, if companies’ human resources departments understand this model and its order, will be able to act effectively and thus be more ethical and sustainable. On the other hand, acting in an inverted order will leave some of the pillars of the Triple Bottom Line uncovered, with the risks that this entails. The Triple Bottom Line (triple P´s) model is a model that calls for corporate commitment to measure its social (Person), environmental (Planet), and financial (Profit) impact. This is why it becomes necessary to have a human resources management model adapted to the current and changing context of the organization (Kramar, 2014 ).
In turn, for the employee, the implementation of a model will help them to prioritize their needs to be covered by the company so that, once managed, they can lead to a higher and better performance and thus achieve a high level of well-being at work. Ruiz-Palomino et al. ( 2019 ) explain that a good way to improve a company productivity is to promote corporate wellness and entrepreneurship.
Conclusions
This paper has answered the question What is the knowledge structure of pioneering research in the field of digital human resources? A mixed methodology was used to identify the main topics studied in Digital HR and to propose a model and some future research lines and questions.
This article presents an integrative review to generate ordered knowledge spaces, which as Patriotta ( 2020 ) explains serves to ‘put boundaries around an existing area of research in order to provide an organized sample of what is available and build a platform for future research’ (p. 1274).
Implications
Theoretical implications.
In terms of theoretical implications, this paper highlights the interest of extending current research to concretely define what the digitalization of work means as well as its implications and requirements. This will enable the discovery or even the proposal of new digital work models, incorporating those positions redefined as a result of the incorporation of artificial intelligence and thus make it possible to delimit digital HR. On the other hand, it is noted that the incorporation of new trends in the market must be reflected in the teaching/learning methods to achieve greater professionalization. In turn, a company will become the protagonist in designing these new training processes that are linked to its specific professional activity and the profile of its employees. On the other hand, emphasis can be placed on studying how to increase productivity through the application of artificial intelligence in routine tasks.
In addition, based on the proposed model, an expansion of research on Digital HR human resources is proposed, incorporating new lines and research questions, which will lead to a new categorization of workplaces according to their capacity to adopt digital models. This will lay the foundation for a new labor framework and the development of innovative capabilities in this regard. This broadening of research can be related to the development of thematic lines and professional sectors, especially in the service sector, thus accommodating the most important sector for the European economy. Future research can consider the development of new TAM (Technology Acceptance Model) models to measure the adoption of digital technologies in digital work environments. Social networks used in the work environment can also be investigated to detect those that best help to channel the processes of labor digitalization.
Practical implications
In terms of practical implications, the results obtained and the proposed model can be used to encourage the application of new technologies in the work environment; guarantee employees digital learning processes to increase productivity, facilitating this new learning process; and create policies and standards that include artificial intelligence and social networks in the business environment, thus standardizing their proper use to generate greater productivity and economic results. New and innovative workspaces can also be developed in order to integrate the improvements derived from digitalization and artificial intelligence. Information channels can also be developed to connect the new processes with stakeholders and adapt the work activity to the new demand of employees and the market, thus including, from its conception, digital natives in the entire process.
Social Implications
The results also have social implications. In this sense, the study of the digitization of human resources helps to adapt the usual performance of employees to the inclusion of new technologies in the business environment and to involve employees in training processes to promote professional and labor development. In turn, it can be used to involve employees in the development of new workspaces to maximize productivity, for the implementation of new work models designed by the company and the use of social networks as a means of labor communication. As a corollary, artificial intelligence can be considered as a tool to improve productivity, reducing the volume of monotonous or routine tasks and reinforcing those in which only the employee can provide real value.
Limitations and future research lines
The authors acknowledge the limitations of the methodology used in this study and call for further research to expand our understanding of the topic. Future studies could complement the co-word analysis with other bibliometric techniques such as co-citation analysis and develop theoretical and empirical models on the applications of digital human resources.
Data availability
Documents that support the findings of this study can be consulted in the Scopus database by following the search procedure indicated in the methodology section.
Alan H (2023) A systematic bibliometric analysis on the current digital human resources management studies and directions for future research. J Chin Hum Resour Manag 14:38–59. https://doi.org/10.47297/wspchrmWSP2040-800502.20231401
Article Google Scholar
Attaran M, Attaran S, Kirkland D (2019) The need for digital workplace: increasing workforce productivity in the information age. Int J Enterp Inf Syst 15:1–23. https://doi.org/10.4018/IJEIS.2019010101
Babapour Chafi M, Hultberg A, Bozic Yams N (2022) Post-Pandemic office work: perceived challenges and opportunities for a sustainable work environment. Sustainability 14(1):294. https://doi.org/10.3390/su14010294
Bachelard G (1982) La formación del espíritu científico. Siglo XXI Editores, México
Google Scholar
Bondarouk T, Brewster C (2016) Conceptualising the future of HRM and technology research. Int J Hum Resour Man 27(21):2652–2671. https://doi.org/10.1080/09585192.2016.1232296
Brachten F, Kissmer T, Stieglitz S (2021) The acceptance of chatbots in an enterprise context–A survey study. Int J Inf Manage 60. https://doi.org/10.1016/j.ijinfomgt.2021.102375
Cetindamar D, Kitto K, Wu M, Zhang Y, Abedin B, Knight S (2024) Explaining AI literacy of employees at digital workplaces. IEEE Trans Eng Manag 71:810–823. https://doi.org/10.1109/TEM.2021.3138503
Choi D, R’bigui H, Cho C (2021) Robotic process automation implementation challenges. In: Pattnaik PK, Sain M, Al-Absi AA, Kumar P (eds) Proceedings of International Conference on Smart Computing and Cyber Security. SMARTCYBER 2020. Lecture Notes in Networks and Systems vol 149. Springer, Singapore. https://doi.org/10.1007/978-981-15-7990-5_29
Cobo MJ, López-Herrera AG, Herrera-Viedma E, Herrera F (2012) SciMAT: a new science mapping analysis software tool. J Am Soc Inf Sci Tec 63(8):1609–1630. https://doi.org/10.1002/asi.22688
Cobo MJ, Jürgens B, Herrero-Solana V, Martínez MA, Herrera-Viedma E (2018) Industry 4.0: a perspective based on bibliometric analysis. Procedia Comput Sci 139:364–371. https://doi.org/10.1016/j.procs.2018.10.278
Colbert A, Yee N, George G (2016) The digital workforce and the workplace of the future. Acad Manag J 59(3):731–739. https://doi.org/10.5465/amj.2016.4003
Costa D, Mamede H, Mira da Silva M (2022) Robotic process automation (RPA) adoption: a systematic literature review. Eng Manag Prod Serv 14:1–12. https://doi.org/10.2478/emj-2022-0012
De Moraes CR, da Cunha PR, Ramos I (2024) Designing digital workplaces: a four-phase iterative approach with guidelines concerning virtuality and enterprise integration obituaries. Pac Asia J Assoc Inf 16(1):74–98. https://aisel.aisnet.org/pajais/vol16/iss1/4 . Available at Accessed 03 June 20
Duke B (2022) 24/7 Digital work-based spy: the effects of technological panopticism on workers in the digital age. J Labor Soc 25(4):520–558. https://doi.org/10.1163/24714607-bja10068
Fuchs C, Sevignani S (2013) What is digital labour? what is digital work? what’s their difference? and why do these questions matter for understanding social media? TripleC-Commun Capit 11 (2). https://doi.org/10.31269/triplec.v11i2.461
Fuchs C (2014) Digital labour and Karl Marx. Routledge, New York
Book Google Scholar
Fumagalli A, Lucarelli S, Musolino E, Rocchi G (2018) Digital labour in the platform economy: the case of Facebook. Sustainability 10(6):1757. https://doi.org/10.3390/su10061757
Giere R (1988) Explaining science: a cognitive approach. Chicago: University of Chicago
Gkinko L, Elbanna A (2022) Hope, tolerance and empathy: employees’ emotions when using an AI-enabled chatbot in a digitized workplace. Inf Technol Peopl 35(6):1714–1743. https://doi.org/10.1108/ITP-04-2021-0328
Gkinko L, Elbanna A (2023) Designing trust: the formation of employees’ trust in conversational AI in the digital workplace. J Bus Res 158. https://doi.org/10.1016/j.jbusres.2023.113707
Graham M, Hjorth I, Lehdonvirta V (2017) Digital labour and development: impacts of global digital labour platforms and the gig economy on worker livelihoods. Transf -Lond 23(2):135–162. https://doi.org/10.1177/1024258916687250
Halloun I (1996) Schematic modeling for meaningful learning of physics. J Res Sci Teach 33(9):1019–1041
IBM (2024). The IBM register: https://www.ibm.com/topics/digital-worker Accesed on January 2024
Jani A, Muduli A, Kishore K (2021) Human resource transformation in India: examining the role digital human resource technology and human resource role. Int J Organ Anal 31(4):959–972. https://doi.org/10.1108/IJOA-08-2021-2886
Jarrett K (2022) Digital Labor. Cambridge, UK
Kaarst-Brown M, Quesenberry J, Niederman F, Weitzel T (2018) Special issue editorial: new approaches to optimizing the digital workplace. Mis Q Exec 18(1):9–14. https://aisel.aisnet.org/misqe/vol18/iss1/3
Kalischko T, Riedl R (2021) Electronic performance monitoring in the digital workplace: conceptualization, review of effects and moderators, and future research opportunities. Front Psychol 12:633031. https://doi.org/10.3389/fpsyg.2021.633031
Article PubMed PubMed Central Google Scholar
Karhu K, Gustafsson R, Lyytinen K (2018) Exploiting and defending open digital platforms with boundary resources: android’s five platform forks. Inf Syst Res 29(2):479–497. https://doi.org/10.1287/isre.2018.0786
Köffer S (2015) Designing the digital workplace of the future–what scholars recommend to practitioners. ICIS 2015 Proceedings. 4. https://aisel.aisnet.org/icis2015/proceedings/PracticeResearch/4
Kramar R (2014) Beyond strategic human resource management: is sustainable human resource management the next approach? Int J Hum Resour Man 25(8):1069–1089. https://doi.org/10.1080/09585192.2013.816863
Kraus S, Breier M, Lim WM (2022) Literature reviews as independent studies: guidelines for academic practice. Rev Manag Sci 16:2577–2595. https://doi.org/10.1007/s11846-022-00588-8
Le KBQ, Sajtos L, Kunz WH, Fernandez KV (2024) The Future of Work: Understanding the Effectiveness of Collaboration Between Human and Digital Employees in Service. J Serv Res 0(0). https://doi.org/10.1177/10946705241229419
Markman GD (2022) Will your study make the world a better place? J Manag Stud 59(6):1597–1603. https://doi.org/10.1111/joms.12843
Marler J, Parry E (2016) Human resource management, strategic involvement and e-HRM technology. Int J Hum Resour Man 27(19):2233–2253. https://doi.org/10.1080/09585192.2015.1091980
Marsh E (2018) Understanding the effect of digital literacy on employees’ digital workplace continuance intentions and individual performance. Int J Digital Lit Digital Competence 9(2):15–33. https://doi.org/10.4018/IJDLDC.2018040102
Marsh E, Pérez-Vallejos E, Spence E (2022) The digital workplace and its dark side: An integrative review. Comput Hum Behav 128. https://doi.org/10.1016/j.chb.2021.107118
Martensen M, Ryschka S, Blesik T, Bick M (2016) Collaboration in the consulting industry: Analyzing differences in the professional use of social software. Bus Process Manag J 22(4):693–711. https://doi.org/10.1108/BPMJ-06-2015-0093
Mićić L, Khamooshi H, Rakovic L, Matkovic P (2022) Defining the digital workplace: a systematic literature review. Strateg Manag 27(2):29–43. https://doi.org/10.5937/straman2200010m
Mićić L, Mastilo Z (2022) Digital workplace transformation: innovative approach after Covid-19 pandemic. Economics 10(2):63–76. https://doi.org/10.2478/eoik-2022-0014
Moganadas SR, Goh GGG (2022) Digital employee experience constructs and measurement framework: a review and synthesis. Int J Technol 13(5):999–1012. https://doi.org/10.14716/ijtech.v13i5.5830
Moher D, Liberati A, Tetzlaff J, Altman DG, Prisma Group (2010) Preferred reporting items for systematic reviews and meta-analyses: The PRISMA statement. Int J Surg 8(5):336–341. https://doi.org/10.1136/bmj.b2535
Article PubMed Google Scholar
Moral-Muñoz JA, López-Herrera AG, Herrera-Viedma E, Cobo MJ (2019) Science mapping analysis software tools: a review. In: Glänzel W, Moed HF, Schmoch U, Thelwall M (eds) Springer Handbook of Science and Technology Indicators. Springer Handbooks. Springer, Cham. https://doi.org/10.1007/978-3-030-02511-3_7
Moral-Muñoz JA, Herrera-Viedma E, Santisteban-Espejo A, Cobo MJ (2020) Software tools for conducting bibliometric analysis in science: An up-to-date review. Prof Info 29(1). https://doi.org/10.3145/epi.2020.ene.03
Moreira MA (2000) Aprendizaje significativo: teoría y práctica. Visor, Madrid
Muszyński K, Pulignano V, Domecka M, Mrozowicki A (2021) Coping with precarity during COVID-19: A study of platform work in Poland. Int Labour Rev 161(3):463–485. https://doi.org/10.1111/ilr.12224
Murati E (2021) What are digital platforms? An overview of definitions, typologies, economics, and legal challenges arising from the platform economy in EU. Eur J Priv Law Technol 2021(1):19–55. https://universitypress.unisob.na.it/ojs/index.php/ejplt/article/view/1264/662
Neber H, Anton M (2008) Promoting pre-experimental activities in high-school chemistry: focusing on the role of students’ epistemic questions. Int J Sci Educ 30(13):1801–1821
Nelson T (2018) Unions in digital labour studies: a review of information society and Marxist autonomist approaches. TripleC-Commun Capit 16(2). https://doi.org/10.31269/triplec.v16i2.1065
Page MJ, McKenzie J, Bossuyt PM et al. (2021) The PRISMA 2020 statement: an updated guideline for reporting systematic reviews. Syst Rev 10(89):2–11. https://doi.org/10.1186/s13643-021-01626-4
Patino A, Naffi N (2023) Lifelong training approaches for the post-pandemic workforces: a systematic review. Int J Lifelong Educ 42(3):249–269. https://doi.org/10.1080/02601370.2023.2214333
Patriotta G (2020) Writing impactful review articles. J Manag Stud 57(6):1272–1276. https://doi.org/10.1111/joms.12608
Post C, Sarala R, Gatrell C, Prescott E (2020) Advancing theory with review articles. J Manag Stud 57(2):351–376. https://doi.org/10.1111/joms.12549
Reuver M, Sørensen C, Basole (2018) The digital platform: a research agenda. J Inf Technol -UK 33(2):124–135. https://doi.org/10.1057/s41265-016-0033-3
Ruiz-Palomino P, Linuesa-Langreo J, Kelly L (2019) Hacia nuevos modelos empresariales más sociales y humanos: El papel de las mujeres en procesos de emprendimiento social y economía de comunión. Empresa y Humanismo 22(2):87–122. https://doi.org/10.15581/015.XXII.2.87-122
Selimović J, Pilav-Velić A, Krndžija L (2021) Digital workplace transformation in the financial service sector: investigating the relationship between employees’ expectations and intentions. Technol Soc 66:101640. https://doi.org/10.1016/j.techsoc.2021.101640
Soriano C, Cabanes JV (2020) Entrepreneurial solidarities: social media collectives and Filipino digital platform workers. Soc Media Soc 6(2):1–11. https://doi.org/10.1177/2056305120926484
Stahlschmidt S, Stephen D (2020) Comparison of Web of Science, Scopus and dimensions databases. KB Forschungspoolprojekt 2020. Available at: https://bibliometrie.info/downloads/DZHW-Comparison-DIM-SCP-WOS.PDF
Stieglitz S, Brachten F, Kissmer T (2018) Defining bots in an enterprise context. International Conference on Interaction Sciences. CIS 2018 Proceedings. 5. https://aisel.aisnet.org/icis2018/impact/Presentations/5
Taylor M, Marsh G, Nicole D, Broadbent P (2017) Good work: the Taylor review of modern working practices. Available via https://www.gov.uk/government/publications/good-work-the-taylor-review-of-modern-working-practices Accessed 03 June 2024
Theres C, Strohmeier S (2023) Consolidating the theoretical foundations of digital human resource management acceptance and use research: a meta-analytic validation of UTAUT. Manag Rev Q https://doi.org/10.1007/s11301-023-00367-z
Villajos E, Tordera N, Peiró JM, van Veldhoven M (2019) Refinement and validation of a comprehensive scale for measuring HR practices aimed at performance-enhancement and employee-support. Eur Manag J 37(3):387–397. https://doi.org/10.1016/j.emj.2018.10.003
White M (2012) Digital workplaces: vision and reality. Bus Inf Rev 29(4):205–214. https://doi.org/10.1177/0266382112470412
Download references
Acknowledgements
This paper has been supported by Project PID2021-124641NB-I00 of the Ministry of Science and Innovation (Spain) and by research group in Open Innovation, Universidad Rey Juan Carlos (Spain). Open Access funding enabled and organized by Project V1313 “Sustainability Support”, signed under Article 60 of the LOSU between the Universidad Rey Juan Carlos (Spain) and the company Triple Sustainability SLU to carry out scientific-technical work and training activities.
Author information
Authors and affiliations.
Universidad Rey Juan Carlos, Madrid, Spain
Laura García-Fernández, Marta Ortiz-de-Urbina-Criado & María-José García-López
You can also search for this author in PubMed Google Scholar
Contributions
Authors contributed equally to this work, and they jointly supervised this work. Contributors to the concept or design of the article: LGF, MOUC, MJGL. Contributed to analysis and interpretation of data: LGF, MOUC, MJGL. Drafting work or critically revising it for important intellectual content: LGF, MOUC, MJGL. Final approval of the version: LGF, MOUC, MJGL. Agreement to be responsible for all aspects of work: LGF, MOUC, MJGL.
Corresponding author
Correspondence to Laura García-Fernández .
Ethics declarations
Competing interests.
The authors declare no competing interests.
Ethical approval
Ethical approval was not required as the study did not involve human participants.
Informed consent
This article does not contain any studies with human participants performed by any of the authors.
Additional information
Publisher’s note Springer Nature remains neutral with regard to jurisdictional claims in published maps and institutional affiliations.
Rights and permissions
Open Access This article is licensed under a Creative Commons Attribution-NonCommercial-NoDerivatives 4.0 International License, which permits any non-commercial use, sharing, distribution and reproduction in any medium or format, as long as you give appropriate credit to the original author(s) and the source, provide a link to the Creative Commons licence, and indicate if you modified the licensed material. You do not have permission under this licence to share adapted material derived from this article or parts of it. The images or other third party material in this article are included in the article’s Creative Commons licence, unless indicated otherwise in a credit line to the material. If material is not included in the article’s Creative Commons licence and your intended use is not permitted by statutory regulation or exceeds the permitted use, you will need to obtain permission directly from the copyright holder. To view a copy of this licence, visit http://creativecommons.org/licenses/by-nc-nd/4.0/ .
Reprints and permissions
About this article
Cite this article.
García-Fernández, L., Ortiz-de-Urbina-Criado, M. & García-López, MJ. Mapping the main research themes in digital human resources. Humanit Soc Sci Commun 11 , 1267 (2024). https://doi.org/10.1057/s41599-024-03795-8
Download citation
Received : 29 May 2023
Accepted : 16 September 2024
Published : 27 September 2024
DOI : https://doi.org/10.1057/s41599-024-03795-8
Share this article
Anyone you share the following link with will be able to read this content:
Sorry, a shareable link is not currently available for this article.
Provided by the Springer Nature SharedIt content-sharing initiative
Quick links
- Explore articles by subject
- Guide to authors
- Editorial policies

- Open access
- Published: 27 September 2024
Narrative Medicine: theory, clinical practice and education - a scoping review
- Ilaria Palla 1 ,
- Giuseppe Turchetti 1 &
- Stefania Polvani 2 , 3
BMC Health Services Research volume 24 , Article number: 1116 ( 2024 ) Cite this article
Metrics details
The origin of Narrative Medicine dates back to more than 20 years ago at an international level. Narrative Medicine is not an alternative to evidence-based medicine, however these two approaches are integrated. Narrative Medicine is a methodology based on specific communication skills where storytelling is a fundamental tool to acquire, understand and integrate several points of view related to persons involving in the disease and in the healthcare process. Narrative Medicine, henceforth NM, represents a union between disease and illness between the doctor’s clinical knowledge and the patient’s experience. According to Byron Good, “we cannot have direct access to the experience of others’ illness , not even through in-depth investigations: one of the ways in which we can learn more from the experience of others is to listen to the stories of what has happened to other people.” Several studies have been published on NM; however, to the best of our knowledge, no scoping review of the literature has been performed.
This paper aims to map and synthetize studies on NM according to theory, clinical practice and education/training.
The scoping review was carried out in accordance with the Preferred Reporting Items for Systematic Reviews and Meta-Analyses extension for scoping reviews (PRISMA-ScR) checklist. A search was conducted in PubMed, APA PsycNet and Jstor. Two authors independently assessed the eligibility and methodological quality of the studies and extracted the data. This review refers to the period from 1998 to 2022.
A total of 843 abstracts were identified of which 274 papers were selected based on the title/abstract. A total of 152 papers in full text were evaluated and 76 were included in the review. Papers were classified according to three issues:
✘ Nineteen studies focused on the definition and concept of NM (Theoretical).
✘ Thirty-eight papers focused on the collection of stories, projects and case reports (Clinical practice).
✘ Nineteen papers focused on the implementation of the Narrative Medicine approach in the education and training of medical doctors (Education and training).
Conclusions
This scoping review presents an overview of the state of the art of the Narrative Medicine. It collect studies performed mainly in Italy and in the United States as these are the countries developing the Narrative Medicine approach in three identified areas, theoretical, clinical practice and education and training. This scoping review will help to promote the power of Narrative Medicine in all three areas supporting the development of methods to evaluate and to measure the Narrative Medicine approach using key performance indicators.
Peer Review reports
Introduction
The role and involvement of patients in healthcare have changed, as has their relationship with healthcare professionals. The patient is no longer a passive subject but part of the healthcare process. Over the years, many approaches to patients’ involvement in healthcare have been developed in the literature, with significant differences in terms of concept and significance.
NM represents a focus on the patient’s needs and the empowerment of their active participation in the healthcare process.
Narrative Medicine enables patients to share their stories with healthcare professionals so that the latter can gain the necessary skills to recognize, interpret and relate to patients [ 1 ]. Stories of illness have an important impact on patients and their caregivers, healthcare professionals and organisational systems [ 2 ].
Trisha Greenhalgh, an academic in primary healthcare who trained as a General Practitioner, and Brian Hurwitz, an Emeritus Professor of Medicine and The Arts at King’s College (London) [ 3 , 4 ], affirmed that the core clinical skills in terms of listening, questioning, outlining, collecting, explaining and interpreting can provide a way of navigating among the very different worlds of patients and health professionals. These tasks need to be performed well because they can affect disease outcomes from the patient’s perspective and the scientific aspects of diagnosis and treatment.
In 2013, Rita Charon, a general internist and professor at Columbia University (New York), and Brian Hurwitz promoted “a narrative future for healthcare” , the first global conference on Narrative Based Medicine (NBM). The global conference took place in London in June 2013, where experts in humanities, social sciences and professionals interested in shaping a narrative future for healthcare discussed several topics, such as increasing the visibility of narrative-based concepts and methods; developing strategies that can influence traditional clinical institutions; spreading appreciation for the role of creativity in caring for the sick; articulating the risks of narrative practices in health care; providing a space for Narrative Medicine in the context of other fields, including personalized medicine; and sharing goals for training, research, and clinical care. The conference was the first important opportunity to share different points of view and perspectives at the global level involving several stakeholders with different backgrounds [ 5 ].
In the early 2000s, the first Italian experience of Narrative Medicine occurred in Florence with NaMe, a project endorsed by the Local Health Authority aimed at diffusing the culture of patient-centered medicine and integrating strategies to improve doctor‒patient communication in clinical practice [ 6 ]. This project was inspired by the articles of Hurwitz and Greenhalgh [ 3 , 4 ]. In addition, significant input was derived from Arthur Kleinman [ 7 ] and Byron Good [ 8 ], psychiatrists and anthropologists who studied medicine as a cultural system, as a set of symbolic meanings involving the story of the sick person. Health and illness represent the subjective experience of the person.
Kleinmann [ 7 ] defines three dimensions to explain the illness using three different significances:
✘ Disease: “only as an alteration in biological structure or functioning” .
✘ Illness: the subjective experience of suffering and discomfort.
✘ Sickness: the social representation.
Narrative Medicine can be used in several areas such as prevention, diagnosis, treatment, and rehabilitation; adherence to treatment; organization of the care team; awareness of the professional role and the emotional world by health and social workers; prevention of the burnout of professionals and caregivers; promotion and implementation of Patient Care Pathways (PCPs); and prevention of legal disputes and defensive medicine.
The Italian guidelines established by the National Institute of Health in 2015 [ 9 ] represent a fundamental step in the process of diffusion and implementation of Narrative Medicine in Italy and currently represents the only document. The guidelines define Narrative Medicine as an intervention methodology based on specific communication skills. Storytelling is a fundamental instrument for acquiring, understanding and integrating the different perspectives of those involved in the disease and in the healthcare process. Storytelling represents a moment of contact between a healthcare professional and the patient’s world. The story told involves people, those who narrate and those who listen. Telling stories is a way of transferring knowledge and experience, connecting, reflecting and feeling emotions.
In the last few years, several studies have been carried out with different objectives and perspectives, but no literature review on Medicine Narrative has been performed. We founded the study of Rui et al. [ 10 ] performing a bibliometric analysis of the literature on medical narratives published from 2011 to 2021 showing that the field of narrative medicine is dominated by a few countries. Respect to 736 studies included in the review, 48% (369) are performed in US and 98 papers in Italy.
The objective of scoping review was to map and synthetize studies on NM according to theory, clinical practice and education/training, three settings where NM was developed.
The research questions formulated: (1) What is Narrative Medicine?; (2) How is Narrative Medicine implemented in clinical practice?; (3) What is the role of Narrative Medicine in education and training for medical doctors?
The study protocol follows the PRISMA-ScR checklist (PRISMA extension for Scoping Reviews) but it is not registered (Additional file 1).
We included peer-reviewed papers published from 1998 to December 2022 written in Italian or in English. We excluded papers written in other languages. We included articles according to one of these issues: studies on theory of Narrative Medicine, on clinical practice or education/training of Narrative Medicine. We excluded books, case reports, reviews. To identify potentially relevant studies, the following databases were searched from 1998 to December 2022: PubMed, APA PsycNet and Jstor. The search strategy can be founded in Additional file 2. A data charting form was developed by two reviewers to define which variables can be extracted. The reviewers independently charted the data and discussed the results. We grouped the studies by type of application related to the Narrative Medicine and summarized objective, methods and reflections/conclusions. The scoping review maps the evidence on Narrative Medicine according one of the three fields of diffusion and implementation (Fig. 1 ). Furthermore, the studies classified in “theoretical field “are grouped in subcategories to explain in best way the concepts and permit a clearer and more streamlined reading.
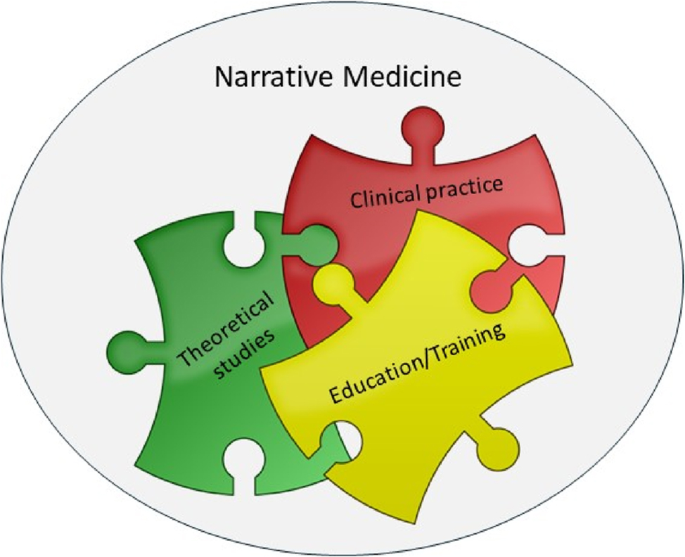
Categories of Narrative Medicine
Review process
After removing duplicates, 843 abstracts from PubMed, Jstor and APA PsycNet were screened. A total of 274 papers were screened based on the abstracts, of which 122 were excluded. A total of 152 full texts were evaluated, and 76 were included in the review (Fig. 2 ).
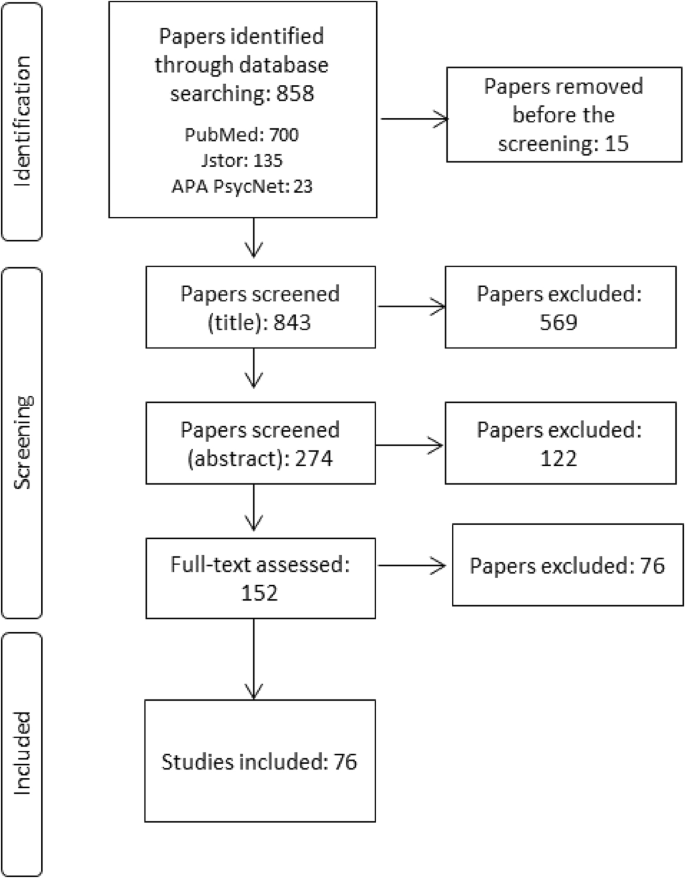
PRISMA Flow-chart
The studies included were classified into the three fields where the Narrative Medicine is implemented:
✘ Theoretical studies: 19.
✘ Clinical Practice: 38.
✘ Education and training: 19.
The scoping review did not present the results of papers included but the main objectives and the methods used as the aim of the scoping review was to map the studies performed in terms of theory, clinical practice and education/training. We have tried to organize the studies published so far, making it increasingly clear how Narrative Medicine has developed.
Theoretical studies
This section presents the 19 selected theoretical studies grouped into subcategories (Additional file 3).
Narrative Medicine: advantages
In this section, we present seven papers that highlight the benefits of narrative medicine.
Of the seven papers considered, four were performed by Rita Charon emphasizing the value of Narrative Medicine in four different contexts. In the first [ 11 ], the study by Goupy et al. evaluated a Narrative Medicine elective course at the Paris-Descartes School of Medicine. In the second [ 12 ], Charon rewrote a patient’s family illness to demonstrate how medicine that respects the narrative dimension of illness and care can improve the care of individual patients, their colleagues and effective medical practice. The third paper [ 13 ] describes a visit to the Rothko Room at the Tate Modern in London as a pretext to emphasize how for narrative medicine, creativity is at the heart of health care and that the care of the sick is a work of art.
In the fourth [ 14 ], Charon provides the elements of narrative theory through a careful reading of the form and content of an excerpt from a medical record. This is part of an audio-recorded interview with a medical student and a reflection on a short section of a modernist novel to show how to determine the significance of patients’ situations.
According to Abettan [ 15 ], Narrative Medicine can play a key role in the reform of current medical practice, although to date, there has been little focus on how and why it can deliver results and be cost-effective.
Cenci [ 16 ] underlines that the existential objective of the patient is fundamental to know the person’s life project and how they would like to live their future years.
Zaharias [ 17 ], whose main sources are Charon and Launer, has published three articles on NM as a valid approach that, if practiced more widely by general practitioners, could significantly benefit both patients and doctors. If the patient’s condition is central, the NM shifts the doctor’s focus from the need to solve the problem to the need to understand. Consequently, the patient‒physician relationship is strengthened, and patients’ needs and concerns are addressed more effectively and with better results.
Narrative Medicine: the role of digital technologies
This section includes 3 papers on the role of digital technologies in Narrative Medicine. Digital narrative medicine is diffusing in care relationship as presents an opportunity for the patient and the clinician. The patient has more time to reflect on his/her needs and communicate in best way with the healthcare professionals. The clinician can access to more information as quantitative and qualitative information and data provided by the patient. These information represent an instrument for the clinician to personalize the care and respond to patient’s unmet needs.
The use of digital technologies, particularly the digital health storymap tool described by Cenci [ 16 ], for obtaining a multidisciplinary understanding of the patient’s medical history facilitates communication between the patient and caregiver. According to Charon [ 18 ], the relentless specialization and technologization of medicine damages the therapeutic importance of recognizing the context of patients’ lives and witnessing their suffering.
Rosti [ 19 ] affirms that e-health technologies will build new bridges and permit professionals to have more time to use narrative techniques with patients.
The increased use of digital technologies could reduce the opportunity for narrative contact but provide a starting point for discussion through the use of electronically transmitted patient pain diaries.
Narrative Medicine: integration with evidence-based medicine
Greenhalgh’s [ 20 ] and Rosti’s [ 19 ] studies address one of the most significant issues, the integration of Narrative Medicine with Evidence Based Medicine. Narrative Medicine is not an alternative to Evidence Based Medicine, they coexist and can complement each other in clinical practice.
Greenhalgh’s work [ 20 ] clearly shows how NM and EBM can be integrated. EBM requires an interpretative paradigm in which the patient experiences the disease in a unique and contextual way and the clinician can draw on all aspects of the evidence and thus arrive at an integrated clinical judgement.
Rosti [ 19 ] believes that even “evidence-based” physicians sustain the importance of competence and clinical judgement. Clinicians also need to rely on patients’ narratives to integrate more objective clinical results. Clinical methods are not without their limitations, which Narrative Medicine can help to overcome. Lederman [ 21 ] enphatises the importance of social sciences to analyze the stories and to improve the care.
Narrative-based Medicine: insidious
Three papers in this section focus on the possible risks of the Narrative Medicine approach. It is needing a more awareness on role of Narrative Medicine as a robust methodology.
The study by Kalitzus [ 22 ] shows how a narrative approach in medicine will be successful only if it has a positive effect on daily clinical practice instead of merely increasing existing problems.
Complex narratives on diseases published in biographies or collected by social scientists are useful only for training and research purposes. NM requires time and effort and cannot be considered the only important issue in medicine. According to Abettan [ 15 ], Narrative Medicine can make the treatment more personalised for each patient, but it is not the only way.
Zaharias [ 17 ] affirms that Narrative Medicine is often described simplistically as listening to the patient’s story, whereas it is much more common and requires special communication skills. Perhaps for these reasons, and despite its advantages, NM is not as widely practiced as it could be. Narrative skills are an integral part of practice and learning them takes time. As the author also states, “the healing power of storytelling is repeatedly attested to while evidence of effectiveness is scarce”. Lanphier [ 23 ] underlines the need to explain the term "narrative medicine" to avoid misunderstandings and to analyze the use of narrative as a tool.
Narrative Medicine: training
Liao et al. [ 24 ] presented a study aimed at helping students improve their relationships with patients by listening to them. These results, similar to those described by Charon [ 25 ], suggest that Narrative Medicine is worth recommending in academic training. The essay by O’Mahony [ 26 ] aims to provoke a debate on how and what the medical humanities should teach. Narratology and narrative medicine are linked to empathy.
Narrative Medicine: clinician-patient communication
Papers included within this category focus on the relationship between the clinician and patient, which is important in the healthcare context.
American healthcare institutions recognize the use of the Narrative Medicine approach to develop quality patient care. As a gastroenterologist at a health centre in Minnesota (US), Rian [ 27 ] concluded that the practice of Narrative Medicine should not be kept on the fringes of medicine as a hobby or ancillary treatment for the benefit of the patients but should be considered key to the healthcare process. Improving doctor‒patient communication merits more attention.
According to Rosti [ 19 ], NM can be seen as a tool to promote better communication. Although time constraints are often mentioned as an obstacle, the time needed to listen to patients is not excessive, and all healthcare professionals should consider giving patients more freedom from time constraints during consultations by encouraging them to talk about their experiences. The use of NM may also be associated with better diagnosis and treatment of pain.
Zaharias [ 28 ] underlines that communication skills are crucial. General practitioners can further develop the strong communication skills they already possess by practicing NM through neutrality, circular questions and hypotheses, and reflective skills.
Narrative Medicine: bioethics in qualitative research
The use of qualitative research in bioethics and narrative approaches to conducting and analysing qualitative interviews are becoming increasingly widespread. As Roest [ 29 ] states, this approach enables more “diagnostic thinking”. It is about promoting listening skills and the careful reading of people and healthcare practices, as well as quality criteria for the ethical evaluation of research and training.
- Clinical practice
In this classification, we included case studies performed in clinical care. We focused on methods used to guide the patients’ stories or narratives written by healthcare professionals. We analysed how Narrative Medicine has been implemented in clinical healthcare practice.
The studies included (38) were performed in the following countries: Italy (28), USA (4), Australia (1), Canada (1), China (1), Colombia (1), Norway (1), and several European countries (1) (Table 1 ). The main methods used were semi-structured interviews that guided the patient’s and physician’s narration [ 30 , 31 , 32 , 33 ], narrative diaries written by patients [ 34 ], and paper parallel charts (an instrument to integrate the patients’ stories in clinical practice) written by clinicians [ 34 , 35 , 36 ].
The studies underlined the usefulness of narrative medicine not only in qualitative research but also in integration with quantitative analysis. Gargiulo et al. [ 45 ] highlighted the importance of integrating narrative medicine and evidence-based approaches to improve therapeutic effectiveness and organizational pathways. Cappuccio et al. [ 36 ] affirmed that narrative medicine can be effective in supporting clinicians in their relationships with patients and caregivers.
Narrative Medicine is an important instrument for patients, caregivers and healthcare professionals [ 63 ]. Suter et al. [ 60 ] affirmed that patients’ stories can help other patients with similar experiences. The studies performed by Cercato [ 39 , 40 ] and Zocher [ 67 ] highlighted the role of digital diaries in the care process from the perspective of healthcare professionals and patients. Sansone et al. [ 55 ] highlighted that the use of diaries in the intensive care unit is helpful in facilitating communication between healthcare professionals and the family.
Education and training
This section includes studies on the role of Narrative Medicine in the education and training of medical students and healthcare professionals. The studies discuss the experiences, roles and programmes of the Narrative Medicine programme in education and training. Nineteen studies were carried out, 10 of which were in the USA (Table 2 ). Only two studies were carried out in Europe, 4 in Taiwan, 1 in Canada, 1 in Iran and 1 in Israel. Seven studies focused on the role of narrative medicine for healthcare professionals [ 68 , 69 , 70 , 71 , 72 , 73 , 74 ], and 11 were aimed at medical students from different disciplines. All studies underlined the positive role of Narrative Medicine in training. Chou et al. [ 75 ] affirmed that the new model of narrative medicine training, “community-based participatory narrative medicine”, which focuses on shared narrative work between healthcare trainees and patients, facilitates the formation of therapeutic patient-clinician relationships but also creates new opportunities to evaluate those relationships. Darayazadeh et al. [ 70 ] underlined the effectiveness of Narrative Medicine in improving students’ reflections and empathy with patients. Additionally, Lam et al. [ 76 ] highlighted that Narrative Medicine could be a useful tool for improving clinical empathy skills. The studies used different approaches to implement the Narrative Medicine method. Arntfield et al. [ 77 ] proposed three tools at different steps of the study (survey, focus group and open-ended questions). Chou et al. [ 75 ] asked participants to write a personal narrative. DasGupta and Charon [ 78 ] used a reflective writing exercise to analyse personal experiences of illness.
In this scoping review we identified 76 studies addressing dissemination and implementation of Narrative Medicine across three settings between 1998 and 2022. The studies performed by Hurwitz [ 3 ] and Greenhalgh [ 4 ] provide a path towards the Narrative Medicine affirm that sickness episodes are important milestones in patient life stories. Not only we live through storytelling, but often, with our doctor or nurse as a witness, we get sick, we improve, we get worse, we are stable and finally we also die through the story. affirms that the stories are often evocative and memorable. They are image rich, action packed and laden with emotions. Most people recall them better than they recall lists, graphs or numbers. Stories can convey important elements of nuance, including mood, tone and urgency. We learn through stories because the story form allows our existing schemas to be modified in the light of emerging experiential knowledge. The stories can capture tacit knowledge: in healthcare organizations they can bridge the gap between explicit, codified and formal knowledge (job descriptions, guidelines and protocols) and informal, not codified knowledge (knowing how to get things done in a particular organization or team, sometimes referred to as knowing the ropes). The “story” is the focal point in the studies related to the clinical practice as these discuss about the patient’s experience, illness story thought tools as questionnaires, narrative diary, chart parallels. The patient is an expert patient able to interact with the healthcare professionals, he/she had not a passive role; the patient is part of the process with the other involved stakeholders. Also, the Italian guidelines on Narrative Medicine [ 9 ] considers the storytelling as a fundamental instrument to acquire, understand and integrate several points of view related to persons involving in the disease and in the healthcare process. Storytelling represents the interaction between a healthcare professional and the patient’s world. According to this perspective, it is useful to educate in Narrative Medicine the healthcare professionals from the University to provide instruments to communicate and interact with their patients. Charon [ 11 ] emphasizes the role of training in narrative skills as an important tool permitting to physicians and medical students to improve their care. Charon [ 24 ] underlines that narrative training permits to explore the clinician’s attention to patients and to establish a relationship with patients, colleagues, and the self. The study of Liao [ 22 ] underlines that Narrative Medicine is worth recommending for healthcare education as resource for interdisciplinary collaboration among students from different discipline.
John Launer in The Art of Medicine. Narrative medicine , narrative practice , and the creation of meaning (2023) [ 87 ] affirm that Narrative Medicine could be complemented by the skills and pedagogy of narrative practice. In addition to the creation and study of words on the page, learners could bring their spoken accounts of their experiences at work and interview each other using narrative practice techniques. He also affirms that narrative practice and narrative medicine could both do more to build alliances with advocacy groups.
We have performed a picture of Narrative Medicine from its origin to today hoping that it will help to promote the power of Narrative Medicine in all three areas becoming increasingly integrated.
Strengths and limitations
The scoping review does not present the results of studies included but objectives, methodology and conclusions/suggestions as it aims to map the evidence related to the Narrative Medicine using a classification defined for the review. This classification had permit to make even clearer the “world” of Narrative Medicine and present a mapping.
English- and Italian-language articles were included because, as seen from the preceding pages, most of the studies were carried out in the United States and Italy.
This could be a limitation, as we may have excluded papers written in other languages. However, the United States and Italy are the countries where Narrative Medicine has developed the most.
The scoping review presents an overview of the literature considering three settings in which Narrative Medicine has emerged from its origins until today highlighting evidence in terms of theory, clinical practice, and education. Currently, a methodology to “measure” Narrative Medicine with indicators, a method assessing the effectiveness and promoting a greater diffusion of Narrative Medicine using objective and measurable indicators, is not available. Furthermore, the literature analysis doesn’t show an integration across three settings. We hope that the review will be a first step towards future projects in which it will be possible to measure Narrative Medicine according to an integrated approach between clinical practice and education/training.
Availability of data and materials
Availability of data and materials: All data generated or analysed during this study are included in this published article.
Abbreviations
- Narrative Medicine
Narrative-Based Medicine
Evidence-Based Medicine
Polvani S. Cura alle stelle. Manuale di salute narrativa. Bulgarini; 2022.
Google Scholar
Polvani S, Sarti A. Medicina narrativa in terapia intensiva. Storie di Malattia e di cura. FrancoAngeli; 2013.
Greenhalgh T, Hurwitz B. Narrative based medicine Why study narrative? BMJ. 1999;318:48–50.
Article CAS PubMed PubMed Central Google Scholar
Greenhalgh T, Hurwitz B. Narrative-Based Medicine: Dialogue and Discourse in Clinical Practice. London: BMJ Books; 1998.
Hurwitz B, Charon R. A narrative future for health care. Lancet. 2013;381:1886–7.
Article PubMed PubMed Central Google Scholar
Ballo P, Milli M, Slater C, Bandini F, Trentanove F, Comper G, Zuppiroli A, Polvani S. Prospective Validation of the Decalogue, a Set of Doctor-Patient Communication Recommendations to Improve Patient Illness Experience and Mood States within a Hospital Cardiologic Ambulatory Setting. Biomed Res Int. 2017. https://doi.org/10.1155/2017/2792131 .
Kleinman A. The Illness Narratives: Suffering, Healing, and the Human Condition. New York: Basic Books; 1988.
Good BJ. Medicine, Rationality, and Experience: An anthropological perspective. Cambridge: Cambridge University Press; 1994.
Book Google Scholar
Istituto Superiore di Sanità, Linee di indirizzo per l’utilizzo della Medicina Narrativa in ambito clinico-assistenziale, per le malattie rare e cronico-degenerative. Sole24Ore Sanità. 2015.
Rui L. Wang L. Global Trends and Hotspots in Narrative Medicine Studies: A Bibliometric Analysis. 2023. https://doi.org/10.21203/rs.3.rs-2816041/v1 .
Article Google Scholar
Charon R. Narrative medicine in the international education of physicians. Presse Med. 2013;42(1):3–5.
Article PubMed Google Scholar
Charon R. Narrative Medicine. A model for empathy, Reflection, profession and Trust. JAMA. 2001;286(15):1897–902.
Article CAS PubMed Google Scholar
Charon R. Narrative Medicine: Caring for the sick is a work of art. JAAPA. 2013;26(12):8.
Charon R. The membranes of care: stories in Narrative Medicine. Acad Med. 2012;87(3):342–7.
Abettan C. From method to hermeneutics: which epistemological framework for narrative medicine? Theor Med Bioeth. 2017;38:179–93.
Cenci C, Fatati G. Conversazioni online per comprendere la malattia e favorire il rapporto medico-paziente. Recenti Prog Med. 2020;111:682–4.
PubMed Google Scholar
Zaharias G. What is narrative-based medicine? Narrative-based medicine 1. Canadian Family Physician|Le Médecin de famille canadien. 2018;64:176–80.
PubMed PubMed Central Google Scholar
Charon R. Form Function, and Ethics. Ann Intern Med. 2001;134:83–7.
Rosti G. Role of narrative-based medicine in proper patient assessment. Support Care Cancer. 2017;25(Suppl 1):3–6.
Greenhalgh T. Narrative based medicine in an evidence-based word. BMJ. 1999;318:323–5.
Lederman M. Social and gendered readings of illness narratives. J Med Humanit. 2016;37:275–88.
Kalitzkus V, Matthiessen PF. Narrative-Based Medicine: Potential, Pitfalls, and Practice. Permanente J. 2009;13(1):80–6.
Lanphier E. Narrative and Medicine premises, practices, pragmatism. Perspective in Biology and Medicine. 2021;64(2):211–34.
Liao HC, Wang YH. Storytelling in Medical Education: Narrative Medicine as a Resource for Interdisciplinary Collaboration. Int J Environ Res Public Health. 2020. https://doi.org/10.3390/ijerph17041135 .
Charon R. Close Reading and Creative Writing in Clinical Education: teaching attention, representation, and affiliation. Acad Med. 2016;91(3):345–50.
O’Mahony S. Against Narrative Medicine. Perspect Biol Med. 2013;56(4):611–9.
Rian J, Hammer R. The Practical Application of Narrative Medicine at Mayo Clinic: Imagining the Scaffold of a Worthy House. Cult Med Psychiatry. 2013;37:670–80.
Zaharias G. Narrative-based medicine and the general practice consultation. Narrative-based medicine 2. Canadian Family Physician|Le Médecin de famille canadien. 2018;64(4):286–90.
Roest B, Milota M, Carlo LC. Developing new ways to listen: the value of narrative approaches in empirical (bio)ethics. BMC Med Ethics. 2021. https://doi.org/10.1186/s12910-021-00691-7 .
Breccia M, Graffigna G, Galimberti S, Iurlo A, Pungolino E, Pizzuti M, Maggi A, et al. Personal history and quality of life in chronic myeloid leukemia patients: a cross-sectional study using narrative medicine and quantitative analysis. Support Care Cancer. 2016;24(11):4487–93.
Cappuccio A, Limonta T, Parodi A, Cristaudo A, Bugliaro F, Cannavò SP, Rossi O. Living with Chronic Spontaneous Urticaria in Italy: A Narrative Medicine Project to Improve the Pathway of Patient Care. Acta Derm Venereol. 2017;97(1):81–5.
Ceccarelli F, Covelli V, Olivieri G, Natalucci F, Conti F. Systemic Lupus Erythematosus before and after COVID-19 Lockdown: How the Perception of Disease Changes through the Lenses of Narrative Medicine. Healthcare (Basel). 2021. https://doi.org/10.3390/healthcare9060726 .
Cenci C, Mecarelli O. Digital narrative medicine for the personalization of epilepsy care pathways. Epilepsy Behav. 2020. https://doi.org/10.1016/j.yebeh.2020.107143 .
Banfi P, Cappuccio A, Latella M, Reale L, Muscianisi E, Marini MG. Narrative medicine to improve the management and quality of life of patients with COPD: the first experience applying parallel chart in Italy. Int J Chron Obstruct Pulmon Dis. 2018;13:287–97.
Cappuccio A, Sanduzzi Zamparelli A, Verga M, Nardini S, Policreti A, Porpiglia PA, Napolitano S, Marini MG. Narrative medicine educational project to improve the care of patients with chronic obstructive pulmonary disease. ERJ Open Res. 2018. https://doi.org/10.1183/23120541.00155-2017 .
Cappuccio A, Napolitano S, Menzella F, Pellegrini G, Policreti A, Pelaia G, Porpiglia PA, Marini MG. Use of narrative medicine to identify key factors for effective doctor-patient relationships in severe asthma. Multidiscip Respir Med. 2019. https://doi.org/10.1186/s40248-019-0190-7 .
Caputo A. Exploring quality of life in Italian patients with rare disease: a computer-aided content analysis of illness stories. Psychol Health Med. 2014;19(2):211–21.
Cepeda MS, Chapman CR, Miranda N, Sanchez R, Rodriguez CH, Restrepo AE, Ferrer LM, Linares RA, Carr DB. Emotional disclosure through patient narrative may improve pain and well-being: results of a randomized controlled trial in patients with cancer pain. J Pain Symptom Manage. 2008;35(6):623–31.
Cercato MC, Colella E, Fabi A, Bertazzi I, Giardina BG, Di Ridolfi P, Mondati M, et al. Narrative medicine: feasibility of a digital narrative diary application in oncology. J Int Med Res. 2022. https://doi.org/10.1177/03000605211045507 .
Cercato MC, Vari S, Maggi G, Faltyn W, Onesti CE, Baldi J, Scotto di Uccio A et al. Narrative Medicine: A Digital Diary in the Management of Bone and Soft Tissue Sarcoma Patients. Preliminary Results of a Multidisciplinary Pilot Study. J Clin Med. 2022. https://doi.org/10.3390/jcm11020406 .
De Vincentis G, Monari F, Baldari S, Salgarello M, Frantellizzi V, Salvi E, Reale L, Napolitano S. Narrative medicine in metastatic prostate cancer reveals ways to improve patient awareness & quality of care. Future Oncol. 2018;14(27):2821–32.
Di Gangi S, Naretto G, Cravero N, Livigni S. A narrative-based study on communication by family members in intensive care unit. J Crit Care. 2013;28(4):483–9.
Donzelli G, Paddeu EM, D’Alessandro F, Nanni CA. The role of narrative medicine in pregnancy after liver transplantation. J Matern Fetal Neonatal Med. 2015;28(2):158–61.
Fox DA, Hauser JM. Exploring perception and usage of narrative medicine by physician specialty: a qualitative analysis. Philos Ethics Humanit Med. 2021. https://doi.org/10.1186/s13010-021-00106-w .
Gargiulo G, Sansone V, Rea T, Artioli G, Botti S, Continisio GI, Ferri P, et al. Narrative Based Medicine as a tool for needs assessment of patients undergoing hematopoietic stem cell transplantation. Acta Biomed. 2017;88:18–24.
Graffigna G, Cecchini I, Breccia M, Capochiani E, Della Seta R, Galimberti S, Melosi A, et al. Recovering from chronic myeloid leukemia: the patients’ perspective seen through the lens of narrative medicine. Qual Life Res. 2017;26(10):2739–54.
Herrington ER, Parker LS. Narrative methods for assessing “quality of life” in hand transplantation: five case studies with bioethical commentary. Med Health Care Philos. 2019;22(3):407–25.
Kvåle K, Haugen DF, Synnes O. Patients’ illness narratives-From being healthy to living with incurable cancer: Encounters with doctors through the disease trajectory. Cancer Rep (Hoboken). 2020. https://doi.org/10.1002/cnr2.1227 .
Lamprell K, Braithwaite J. Reading Between the Lines: A Five-Point Narrative Approach to Online Accounts of Illness. J Med Humanit. 2019;40(4):569–90.
Marini MG, Chesi P, Bruscagnin M, Ceccatelli M, Ruzzon E. Digits and narratives of the experience of Italian families facing premature births. J Matern Fetal Neonatal Med. 2018;31(17):2258–64.
Marini MG, Chesi P, Mazzanti L, Guazzarotti L, Toni TD, Salerno MC, Officioso A, et al. Stories of experiences of care for growth hormone deficiency: the CRESCERE project. Future Sci OA. 2016. https://doi.org/10.4155/fso.15.82 .
Midena E, Varano M, Pilotto E, Staurenghi G, Camparini M, Pece A, Battaglia PM. Real-life patient journey in neovascular age-related macular degeneration: a narrative medicine analysis in the Italian setting. Eye (Lond). 2022;36(1):182–92.
Palandri F, Benevolo G, Iurlo A, Abruzzese E, Carella AM, Paoli C, Palumbo GA, et al. Life for patients with myelofibrosis: the physical, emotional and financial impact, collected using narrative medicine-Results from the Italian “Back to Life” project. Qual Life Res. 2018;27(6):1545–54.
Rushforth A, Ladds E, Wieringa S, Taylor S, Husain L, Greenhalgh T. Long Covid-The illness narratives. Soc Sci Med. 2021. https://doi.org/10.1016/j.socscimed.2021.114326 .
Sansone V, Cancani F, Gagliardi C, Satta T, Cecchetti C, de Ranieri C, Di Nardo M, Rossi A, et al. Narrative diaries in the paediatric intensive care unit: A thematic analysis. Nurs Crit Care. 2022;27(1):45–54.
Scaratti C, Zorzi G, Guastafierro E, Leonardi M, Covelli V, Toppo C, Nardocci N. Long term perceptions of illness and self after Deep Brain Stimulation in pediatric dystonia: A narrative research. Eur J Paediatr Neurol. 2020;26:61–7.
Simonelli F, Sodi A, Falsini B, Bacci G, Iarossi G, Di Iorio V, Giorgio D, et al. Care Pathway of RPE65-Related Inherited Retinal Disorders from Early Symptoms to Genetic Counseling: A Multicenter Narrative Medicine Project in Italy. Clin Ophthalmol. 2021;2(15):4591–605.
Slocum RB, Howard TA, Villano JL. Narrative Medicine perspectives on patient identity and integrative care in neuro-oncology. J Neuroncol. 2017;134(2):417–21.
Slocum RB, Hart AL, Guglin ME. Narrative medicine applications for patient identity and quality of life in ventricular assist device (VAD) patients. Heart Lung. 2019;48(1):18–21.
Suter N, Ardizzone G, Giarelli G, Cadorin L, Gruarin N, Cipolat Mis C, Michilin N, et al. The power of informal cancer caregivers’ writings: results from a thematic and narrative analysis. Support Care Cancer. 2021;29(8):4381–8.
Talarico R, Cannizzo S, Lorenzoni V, Marinello D, Palla I, Pirri S, Ticciati S, et al. RarERN Path: a methodology towards the optimisation of patients’ care pathways in rare and complex diseases developed within the European Reference Networks. Orphanet J Rare Dis. 2021. https://doi.org/10.1186/s13023-021-01778-5 .
Testa M, Cappuccio A, Latella M, Napolitano S, Milli M, Volpe M, Marini MG. The emotional and social burden of heart failure: integrating physicians’, patients’, and caregivers’ perspectives through narrative medicine. BMC Cardiovasc Disord. 2020. https://doi.org/10.1186/s12872-020-01809-2 .
Tonini MC, Fiorencis A, Iannacchero R, Zampolini M, Cappuccio A, Raddino R, Grillo E, et al. Narrative Medicine to integrate patients’, caregivers’ and clinicians ’migraine experiences: the DRONE multicentre project. Neurol Sci. 2021;42:5277–88.
Vanstone M, Toledo F, Clarke F, Boyle A, Giacomini M, Swinton M, Saunders L, et al. Narrative medicine and death in the ICU: word clouds as a visual legacy. BMJ Support Palliat Care. 2016. https://doi.org/10.1136/bmjspcare-2016-001179 .
Volpato E, Centanni S, Banfi P, D’Antonio S, Peterle E, Bugliaro F, Grattagliano I, et al. Narrative Analysis of the Impact of COVID-19 on Patients with Chronic Obstructive Pulmonary Disease, Their Caregivers, and Healthcare Professionals in Italy. Int J Chron Obstruct Pulmon Dis. 2021;16:2181–201.
Zhang Y, Pi B, Xu X, Li Y, Chen X, Yang N. Influence of Narrative Medicine-Based Health Education Combined With An Online Patient Mutual Assistance Group On The Health Of Patients With Inflammatory Bowel Disease and Arthritis. Psychol Res Behav Manag. 2020;7(13):1–10.
Zocher U, Bertazzi I, Colella E, Fabi A, Scarinci V, Franceschini A, Cenci C, et al. Application of narrative medicine in oncological clinical practice: impact on health care professional. Recenti Prog Med. 2020;111(3):154–9.
Chen PJ, Huang CD, Yeh SJ. Impact of a narrative medicine programme on healthcare providers’ empathy scores over time. BMC Med Educ. 2017. https://doi.org/10.1186/s12909-017-0952-x .
Chu SY, Wen CC, Lin CW. A qualitative study of clinical narrative competence of medical personnel. BMC Med Educ. 2020. https://doi.org/10.1186/s12909-020-02336-6 .
Daryazadeh S, Adibi P, Yamani N. The role of narrative medicine program in promoting professional ethics: perceptions of Iranian medical students. J Med Ethics Hist Med. 2021. https://doi.org/10.18502/jmehm.v14i21.8181 .
Karkabi K, Wald HS, Castel OC. The use of abstract paintings and narratives to foster reflective capacity in medical educators: a multinational faculty development workshop. Med Humanit. 2014;40(1):44–8.
Lijoi AF, Tovar AD. Narrative medicine: Re-engaging and re-energizing ourselves through story. Int J Psychiatry Med. 2020;55(5):321–30.
Wallace CL, Trees A, Ohs J, Hinyard L. Narrative Medicine for Healthcare Providers: Improving Practices of Advance Care Planning. Omega (Westport). 2023;87(1):87–102.
Winkel AF, Feldman N, Moss H, Jakalow H, Simon J, Blank S. Narrative Medicine Workshops for Obstetrics and Gynecology Residents and Association with Burnout Measures. Obstet Gynecol. 2016;128(Suppl 1):27–33.
Chou JC, Schepel IRM, Vo AT, Kapetanovic S, Schaff PB. Patient Co-Participation in Narrative Medicine Curricula as a Means of Engaging Patients as Partners in Healthcare: a pilot study involving medical students and patient living with HIV. J Med Humanit. 2021;42(4):641–57.
Lam JA, Feingold-Link M, Noguchi J, Quinn A, Chofay D, Cahill K, Rougas S. My Life, My Story: Integrating a Life Story Narrative Component into Medical Student Curricula. MedEdPORTAL. 2022. https://doi.org/10.15766/mep_2374-8265.11211 .
Arntfield SL, Slesar K, Dickson J, Charon R. Narrative medicine as a means of training medical students toward residency competencies. Patient Educ Couns. 2013;91(3):280–6.
DasGupta S, Charon R. Personal illness narratives: using reflective writing to teach empathy. Acad Med. 2004;79(4):351–6.
Gowda D, Curran T, Khedagi A, Mangold M, Jiwani F, Desai U, Charon R, Balmer D. Implementing an interprofessional narrative medicine program in academic clinics: Feasibility and program evaluation. Perspect Med Educ. 2019;8(1):52–9.
Lemogne C, Buffel du Vaure C, Hoertel N, Catu-Pinault A, Limosin F, Ghasarossian C, Le Jeunne C, Jaury P. Balint groups and narrative medicine compared to a control condition in promoting students’ empathy. BMC Med Educ. 2020. https://doi.org/10.1186/s12909-020-02316-w .
Liao KC, Peng CH, Snell L, Wang X, Huang CD, Saroyan A. Understanding the lived experiences of medical learners in a narrative medicine course: a phenomenological study. BMC Med Educ. 2021. https://doi.org/10.1186/s12909-021-02741-5 .
Lorenz JF, Darok MC, Ho L, Holstrom-Mercader MS, Freiberg AS, Dellasega CA. The Impact of an Unconventional Elective in Narrative Medicine and Pediatric Psycho-oncology on Humanism in Medical Students. J Cancer Educ. 2022;37(6):1798–805.
Miller E, Balmer D, Hermann N, Graham G, Charon R. Sounding narrative medicine: studying students’ professional identity development at Columbia University College of Physicians and Surgeons. Acad Med. 2014;89(2):335–42.
Shaw AC, McQuade JL, Reilley MJ, Nixon B, Baile WF, Epner DE. Integrating Storytelling into a Communication Skills Teaching Program for Medical Oncology Fellows. J Cancer Educ. 2019;34(6):1198–203.
Skelton JR, O’Riordan M, Berenguera Ossȯ A, Beavan J, Weetman K. Learning from patients: trainers’ use of narratives for learning and teaching. BJGP Open. 2017. https://doi.org/10.3399/bjgpopen17X100581 .
Launer J, Wohlmann A. Narrative medicine, narrative practice, and the creation of meaning. Lancet. 2023;401(10371):98–9.
Download references
The work has not been financed.
Author information
Authors and affiliations.
Institute of Management, Scuola Superiore Sant’Anna Pisa, Piazza Martiri della Libertà 33, Pisa, 56127, Italy
Ilaria Palla & Giuseppe Turchetti
SIMeN, Società Italiana Medicina Narrativa, Arezzo, Italy
Stefania Polvani
Azienda USL Toscana Sud Est, Arezzo, Italy
You can also search for this author in PubMed Google Scholar
Contributions
I.P. and S.P. carried out the scoping review, conceived the study, data collection process and drafted the manuscript. G.T. participated in the coordination of the study. All authors read, reviewed and approved the final manuscript.
Corresponding author
Correspondence to Ilaria Palla .
Ethics declarations
Ethics approval and consent to participate.
Not applicable.
Consent for publication
Competing interests.
The authors declare no competing interests.
Additional information
Publisher’s note.
Springer Nature remains neutral with regard to jurisdictional claims in published maps and institutional affiliations.
Supplementary Information
Supplementary material 1., supplementary material 2., supplementary material 3., rights and permissions.
Open Access This article is licensed under a Creative Commons Attribution-NonCommercial-NoDerivatives 4.0 International License, which permits any non-commercial use, sharing, distribution and reproduction in any medium or format, as long as you give appropriate credit to the original author(s) and the source, provide a link to the Creative Commons licence, and indicate if you modified the licensed material. You do not have permission under this licence to share adapted material derived from this article or parts of it. The images or other third party material in this article are included in the article’s Creative Commons licence, unless indicated otherwise in a credit line to the material. If material is not included in the article’s Creative Commons licence and your intended use is not permitted by statutory regulation or exceeds the permitted use, you will need to obtain permission directly from the copyright holder. To view a copy of this licence, visit http://creativecommons.org/licenses/by-nc-nd/4.0/ .
Reprints and permissions
About this article
Cite this article.
Palla, I., Turchetti, G. & Polvani, S. Narrative Medicine: theory, clinical practice and education - a scoping review. BMC Health Serv Res 24 , 1116 (2024). https://doi.org/10.1186/s12913-024-11530-x
Download citation
Received : 01 February 2024
Accepted : 03 September 2024
Published : 27 September 2024
DOI : https://doi.org/10.1186/s12913-024-11530-x
Share this article
Anyone you share the following link with will be able to read this content:
Sorry, a shareable link is not currently available for this article.
Provided by the Springer Nature SharedIt content-sharing initiative
- Healthcare professional
- Scoping review
- Personalized medicine
BMC Health Services Research
ISSN: 1472-6963
- General enquiries: [email protected]
A Systematic Literature Review of Human-Centered, Ethical, and Responsible AI
As Artificial Intelligence (AI) continues to advance rapidly, it becomes increasingly important to consider AI’s ethical and societal implications. In this paper, we present a bottom-up mapping of the current state of research at the intersection of Human-Centered AI, Ethical, and Responsible AI (HCER-AI) by thematically reviewing and analyzing 164 research papers from leading conferences in ethical, social, and human factors of AI: AIES , CHI , CSCW , and FAccT . The ongoing research in HCER-AI places emphasis on governance, fairness, and explainability. These conferences, however, concentrate on specific themes rather than encompassing all aspects. While AIES has fewer papers on HCER-AI, it emphasizes governance and rarely publishes papers about privacy, security, and human flourishing. FAccT publishes more on governance and lacks papers on privacy, security, and human flourishing. CHI and CSCW, as more established conferences, have a broader research portfolio. We find that the current emphasis on governance and fairness in AI research may not adequately address the potential unforeseen and unknown implications of AI. Therefore, we recommend that future research should expand its scope and diversify resources to prepare for these potential consequences. This could involve exploring additional areas such as privacy, security, human flourishing, and explainability.
1. Introduction
Artificial Intelligence (AI) is a rapidly growing field with tremendous potential to transform our lives on an unprecedented scale through a new technological revolution (Kissinger et al . , 2021 ) . It can advance the global economy and contribute to human flourishing (Laker, 2022 ; Lee, 2022 ) . However, like every significant technological revolution, ethical and societal risks such as invasion of privacy or identity theft in social media (Geoffrey A. Fowler, 2021 ; Associated Press, 2021 ) are taking center stage. Risks associated with AI are no exception. If left unattended, AI can negatively impact specific populations, perpetuate historical injustices, or, even worse, amplify them (Baeza-Yates, 2018 ; Leonardo Nicoletti and Dina Bass, 2023 ) . These risks include misclassifying people of color (Buolamwini and Gebru, 2018 ; Leonardo Nicoletti and Dina Bass, 2023 ) ; exacerbating economic disadvantage by denying bank loans through digital redlining (Hertzberg et al . , 2010 ; Kizilaslan and Lookman, 2017 ) ; reducing medical care for economically disadvantaged people based on their prior medical spending (Arthur et al . , 2008 ; Jones et al . , 2020 ; Wilkinson et al . , 2020 ) ; denying bail to Queer, Trans, Black, Indigenous, or People of Color (QTBIPOC) (Silva and Kenney, 2018 ) ; imposing longer prison sentences on QTBIPOC people (Lyn, 2020 ) ; and removing children from QTBIPOC families into foster care (Leckning et al . , 2021 ; Saxena et al . , 2020 ) . In addition to the considerable attention given to biases within data sources, recent work examines human “wrangling” activities necessary to make so-called “raw” data fit-for-purpose. However, these changes to datasets and models during AI development introduce biases as a consequence (Muller and Strohmayer, 2022 ; Pine and Liboiron, 2015 ; Feinberg, 2017 ; Passi and Jackson, 2017 ; Mentis et al . , 2016 ) . These examples remind us that people build AI systems and that social biases and prejudices may be included—intentionally or inadvertently (Aragon et al . , 2022 ; Muller and Strohmayer, 2022 ) .
The scientific community has recently made attempts to address these pressing issues. Many research conferences, journals, and groups have begun to argue for responsible AI development, bringing to the table issues including but not limited to fairness, explainability, and privacy in AI (Google, 2022 ; Microsoft, 2022 ; PwC, 2022 ; Nokia Bell Labs, 2022 ; Barredo Arrieta et al . , 2020 ; The Organisation for Economic Co-operation and Development (2019), OECD ; National Institute of Standards and Technology, 2023 ) , and centering AI around humans (Aragon et al . , 2022 ; Chancellor, 2023 ; Ehsan et al . , 2021a ) . These topics are discussed in research conferences with a long history of advocating for human-centered design, such as the Conference on Human Factors in Computing Systems (CHI) and the Conference on Computer-Supported Cooperative Work and Social Computing (CSCW), and in newer conferences like the Conference on AI, Ethics, and Society (AIES), and the Conference on Fairness, Accountability, and Transparency (FAccT), which were established to cover topics related to the ethics of AI. In response to the large and growing interest in AI, there have been several survey and review papers mapping out the AI research landscape (Capel and Brereton, 2023 ; Wong et al . , 2023b ) , such as those surveying responsible development of AI in healthcare (Siala and Wang, 2022 ) , biases and fairness in AI (Sun et al . , 2019 ; Pessach and Shmueli, 2022 ; Orphanou et al . , 2022 ) , and explainable AI (Abdul et al . , 2018 ; Bertrand et al . , 2022 ; Barredo Arrieta et al . , 2020 ; Angelov et al . , 2021 ) . However, a comprehensive overview at the intersection of human-centered AI , ethical AI , and responsible AI is lacking. The intersection of Human-Centered, Ethical, and Responsible AI (HCER-AI) is significant because it presents a complex challenge of addressing multiple aspects simultaneously (see Section 3 for how we define these terms). Each aspect—human-centeredness, ethical considerations, and responsible AI—cannot be achieved in isolation; they are interdependent. This interdependence underscores the crucial need to thoroughly understand and explore this intersection to create AI systems that are comprehensive and aligned with societal values. We aim to fill this gap by surveying the current state of research in HCER-AI in four leading research conferences on these topics. We formulated four Research Questions (RQs):
What is the state of research in HCER-AI in the four research conferences that cover topics related to HCER-AI?
What research methods are used in HCER-AI studies?
What are the research gaps in HCER-AI?
To further assess the current state of research in HCER-AI and compare it with potential future implementations, as indicated by patents, we conclude with the following question:
What is the landscape of patent applications in HCER-AI?
To answer our RQs, we reviewed and thematically analyzed 164 research papers from the proceedings of AIES, CHI, CSCW, and FAccT related to HCER-AI (Section 3 ). We found that the landscape of HCER-AI covers six primary themes: governance , fairness , explainability , human flourishing , privacy , and security , with a heavy emphasis on the first three themes (Section 5 ). These results echo prior empirical studies with different groups of people, highlighting a gap between public and AI research concerns (Jakesch et al . , 2022 ) . For instance, while there is a critical mass of research on fairness, average users may care more about their safety and privacy (Jakesch et al . , 2022 ) . We also found that conferences have distinct areas of focus within HCER-AI. For example, AIES and FAccT publish more papers on governance and fairness, while CHI and CSCW cover a broader range of topics, including explainability and human flourishing. Our results suggest that future research should take a broader view of AI and diversify resources beyond governance and fairness to prepare for AI’s unexpected and unknown ramifications.
As part of our posteriori analysis, we used AI-assisted summarization to answer our RQs using the abstracts of the papers as input to ChatGPT 4.0, following the recent emerging literature on using AI for qualitative analysis (Byun et al . , 2023 ; Abram et al . , 2020 ) . We compared its findings with our manual analysis (Section 8 ). Although ChatGPT provided an overview of the dataset, it is important to highlight that its classification was not specifically tailored to the research areas currently under investigation. Instead, it provided a broad classification intended for general public understanding, rather than specialized research purposes. In the future, AI-powered research tools like ChatGPT could be a helpful supplementary resource for gaining new insights. Further examination is needed to determine how AI can be effectively utilized in literature reviews.
Materials concerning our classification and analysis will be available on our project’s website, which can be found at social-dynamics.net/RAI-Review .
2. Related Work
To situate our review within the broader human-centered, ethical, and responsible AI literature, we next discuss previous surveys that focused on human-centered AI, specific concerns with AI such as fairness and explainability, and surveys dedicated to specific domains of AI.
Developing AI involves socio-cultural and technical factors, with scholars arguing for developing human-centered AI in the past few years (Aragon et al . , 2022 ; Shneiderman, 2022 ; Bingley et al . , 2023 ; Ehsan et al . , 2021b ) . In particular, there is an emphasis on the challenges of AI integration into socio-technical processes to preserve human autonomy and control, as well as the impacts of AI deployment and applications on society, organizations, and individuals (Boyarskaya et al . , 2020 ; Whittlestone et al . , 2019 ) . Scholars also argue that understanding socio-technical and environmental factors can surface why and how an AI may become human-centered (Shneiderman, 2020 ; Ehsan et al . , 2021a ; Liao and Varshney, 2021 ; Muller et al . , 2022 ) .
Despite its recent surge, bias in AI has been a longstanding topic of interest among academic circles (Caton and Haas, 2020 ) . Several surveys discussed data and model biases across domains, including social data biases that stem from user-generated context and behavioral traces (Olteanu et al . , 2019 ) , gender bias in natural language processing (Sun et al . , 2019 ) , and fairness metrics with potential mitigation strategies (Caton and Haas, 2020 ; Pessach and Shmueli, 2022 ; Orphanou et al . , 2022 ) . Others investigated emerging research trends in responsible AI, such as fair adversarial learning, fair word embeddings, fair recommender systems, and fair visual description (Pessach and Shmueli, 2022 ) .
As AI comes with the promise of advancing our economy and augmenting our lives, it becomes increasingly essential for people to understand AI’s implications and remain in control (Barredo Arrieta et al . , 2020 ) . To this end, much of the previous literature has been dedicated to discussing explainability in AI. For example, a survey of 289 core papers on explanations and explainable systems and more than 12,000 citing papers represents the diverse research landscape of AI, such as algorithmic accountability, interpretable machine learning, context awareness, cognitive psychology, and software learnability (Abdul et al . , 2018 ) . More recently, Angelov et al . ( 2021 ) provided an overview of AI explainability techniques considering recent advancements in machine learning and deep learning. Methods included featured-oriented ones such as SHapley Additive exPlanation (SHAP) (Lundberg and Lee, 2017 ) , and surrogate models such as Local interpretable model-agnostic explanations (LIME) (Dieber and Kirrane, 2020 ) . Another line of work reviewed specific domains (e.g., recommender systems, social networks, and healthcare). These reviews cover notions of fairness specifically tailored for recommender systems (Li et al . , 2022 ) , machine learning fairness in the domain of public and population health (Mhasawade et al . , 2021 ) , and algorithmic fairness in computational medicine (Xu et al . , 2022 ) .
The closest survey to ours is Capel and Brereton ( 2023 ) ’s survey, published at CHI 2023, where the authors focus on the “human-centered AI” as the primary search term. The landscape mapping resulted in four major themes: explainable and interpretable AI, human-centered approaches for designing and evaluating AI, human-AI teaming, and ethical AI. Our survey extends this survey beyond human-centered AI by adding two keywords (ethical AI and responsible AI). Adding these keywords has significance because it presents the complex challenge of addressing multiple aspects simultaneously. Each aspect—human-centeredness, ethical considerations, and responsible AI—cannot be achieved in isolation; they are interdependent. This interdependence underscores the crucial need to thoroughly comprehend and explore this intersection in order to create AI systems that are comprehensive and aligned with societal values. Our work also shows the differences and similarities between the research communities, which are not covered in the prior work.
While previous surveys mapped out parts of research in human-centered AI, ethical AI, and responsible AI, these mappings do not fully cover the intersection of the three, underscoring the need to explore it systematically. We also provide a lens into different research practices across the research communities as a new angle to the prior surveys. Therefore, this review aims to fill this gap and contribute to the ongoing discourse about HCER-AI.
To answer our RQs (§ 1 ), we surveyed the HCER-AI literature in AIES, CHI, CSCW, and FAccT following the PRISMA 2020 statement for conducting systematic literature reviews (Page et al . , 2021 ) . Figure 1 shows the flowchart of our method.

3.1. Positionality Statement
Understanding researcher positionality is essential for demystifying our lens on data collection and analysis (Frluckaj et al . , 2022 ; Havens et al . , 2020 ) . We situate this paper in two Western countries in the 21 st century, writing as authors primarily working as academic and industry researchers. We identify as males from the Middle East, Southern Europe, and North America with diverse ethnic and religious backgrounds. Our shared research backgrounds include human-centered computing, privacy, security, software engineering, AI, social and ubiquitous computing, urbanism, and conducting systematic literature reviews. While we aimed at a bottom-up literature analysis, our results are constructs of our expertise and understanding of the landscape, as well as our positionality and cultural background. At least two authors were involved in all research steps to diversify the biases that attend any humans’ judgments in analyzing the papers and reporting the results.
3.2. Eligibility and Inclusion Criteria
For this systematic review and our scoping, papers qualified for the analysis if their contributions pertained to three main areas: human-centered AI , responsible AI , and ethical AI . By searching for keywords, we relied on the paper authors’ use of terms related to our scoping. Our interpretation of AI is based on the definition of the National Institute of Standards and Technology (NIST), published in 2023: “as an engineered or machine-based system that can, for a given set of objectives, generate outputs such as predictions, recommendations, or decisions influencing real or virtual environments. AI systems are designed to operate with varying levels of autonomy.” (National Institute of Standards and Technology, 2023 ) In the next section (Section 3.3 ), we describe our data collection method.
3.3. Data Collection
We decided to base our review on four research conferences: two well-established venues with a wide net for human-computer interaction research and two recently established venues with a narrow emphasis on AI and its implications (excerpts taken from conferences’ websites):
AIES (established in 2018): “our goal is to encourage talented scholars in these and related fields to submit their best work related to morality, law, policy, psychology, the other social sciences, and AI.”
CHI (established in 1982): “annually brings together researchers and practitioners from all over the world and from diverse cultures, backgrounds, and positionalities, who have as an overarching goal to make the world a better place with interactive digital technologies.”
CSCW (established in 1986): “is the premier international venue for research in the design and use of technologies that affect groups, organizations, communities, and networks.”
FAccT (established in 2019; previously known as FAT*): “a computer science conference with a cross-disciplinary focus that brings together researchers and practitioners interested in fairness, accountability, and transparency in socio-technical systems.”
We collected 228 records from the ACM Digital Library from these four conferences in May 2023. We queried for ‘‘human-centered AI’’ OR ‘‘human-centered artificial intelligence’’ OR ‘‘ethical artificial intelligence’’ OR ‘‘ethical AI’’ OR ‘‘responsible AI’’ OR ‘‘responsible artificial intelligence’’ within anywhere in the proceedings of AIES, CHI, CSCW, and FAccT, including only full research papers using ACM’s filters (excluding other materials such as abstracts, panels, and tutorials). We chose these keywords because human-centered AI, ethical AI, responsible AI are widely used in academia and industry; examples include prior literature (Capel and Brereton, 2023 ; Shneiderman, 2022 ) and guidelines produced by large technology companies (e.g., Google, Microsoft, and IBM use responsible AI to address ethical concerns and risks with AI (Google, 2022 ; Microsoft, 2022 ; PwC, 2022 ; Nokia Bell Labs, 2022 ; Barredo Arrieta et al . , 2020 ; The Organisation for Economic Co-operation and Development (2019), OECD ; Tahaei et al . , 2023 ) ). We define human-centered AI as a paradigm that advocates for including people’s requirements and needs in designing, developing, and deploying AI systems. Ethical AI and responsible AI refer to paradigms that emphasize the inclusion of ethical values in the design, development, and deployment of AI, as well as the responsibility to consider the risks and consequences on individuals and societies. Our review aims to explore the intersection of these research areas. We explore the topics related to the impact of AI to inform the building of AI systems that prioritize the needs and requirements of people and society while mitigating potential harms through a human-centered approach.
The above process resulted in 190 full research papers that two authors read to assess their relevance and eligibility (Section 3.2 ). They examined papers discussing human-centered AI, ethical AI, and responsible AI. They excluded papers that mention the keywords in the references or acknowledgments without discussing them in the main text and papers that did not cover our topics of interest (human-centered AI, ethical AI, and responsible AI). After discussing and resolving disagreements, 26 of these papers were excluded. Therefore, our review and the rest of this paper are based on a final set of 164 research papers from AIES (n=30), CHI (n=68), CSCW (n=34), and FAccT (n=32).
We were also interested in discovering patents related to our keywords. Patents can show the practical applications of an invention and how it propagates in the industry and products (Cao et al . , 2023 ) . HCI research, in particular, significantly impacts patents compared to other computer science research areas (Cao et al . , 2023 ) . Therefore, we ran the same query we executed on ACM on the United States Patent and Trademark Office search tool (United States Patent and Trademark Office, 2023 ) and retrieved 67 results. One author manually reviewed these patents and removed 30 because they were unrelated and only appeared in the results due to a keyword (e.g., patents with “responsible for AI” were also included). This process left us with 37 patents.
3.4. Data Analysis
Following a thematic analysis method (Braun and Clarke, 2006 ; Lazar et al . , 2017a ) , the first two authors split the papers between themselves. After discussions, they decided to open code each paper with the research methods used, primary contributions, human aspects, responsible aspects, and sample description (any information about the population or dataset of the paper). They used the paper authors’ words to code each paper (also known as “in vivo coding” (Lazar et al . , 2017b ) ). When in doubt, they flagged a paper for further discussion (n=27). In a joint session, they used thematic analysis to create themes from the codes and resolved disagreements through discussions. They did not use a set of predefined themes but came up with the results using a bottom-up approach. In our analysis, a paper may appear in multiple codes—codes are not mutually exclusive. Sections 4 and 5 are derived from this analysis. Although our findings are primarily based on this analysis and review, as authors actively engaged in this field, we have also utilized other papers and online resources to enhance our discussion beyond the reviewed papers, especially when addressing future directions. For a comprehensive list of the reviewed papers, refer to Table 4 in the Appendix.
In a joint session, two authors read the title and abstract of the remaining 37 patents and built an affinity diagram. During this process, they removed another 9 patents because they were unrelated and did not discuss our topics of interest as their primary claims. The final set of patents totaled 28 . Section 6 is based on the findings of this analysis.
3.5. Limitations
We acknowledge that our literature search may not be comprehensive and exhaustive. However, covering AIES, CHI, CSCW, and FAccT as prominent venues for research in AI, Human-Computer Interaction (HCI), ethical, and responsible AI gave us insights into the state of the art of HCER-AI. Future research may also build on our work and expand it to other academic and industry literature. We also used keywords instead of reviewing a list of papers and deciding which paper to include or exclude based on our judgment (a typical approach in running systematic literature reviews, for example, Holländer et al . ( 2021 ) and Bergram et al . ( 2022 ) ). Through this approach, we collected papers related to HCER-AI from the authors’ perspective rather than using our own judgment of whether a paper is related to HCER-AI. Future research may take a representative sample of papers from research publications, conduct a bottom-up analysis, and compare results with ours.
4. Results: Research Methods of HCER-AI
In the 164 papers reviewed, 85 were qualitative (e.g., user studies for system design and evaluation, interviews, and workshops), 25 were quantitative (e.g., survey and log analysis), 21 were theoretical (e.g., essays and framework proposal), 20 were mixed-methods (a combination of qualitative and quantitative), 8 were reviews (e.g., review of literature, policies, and guidelines), and 5 were a combination of a review and theory (Figure 2 ). CHI and CSCW are heavily focused on qualitative studies (as expected since they are the premier venues for human-centered studies); however, they lack papers derived from theoretical reviews. AIES and FAccT cover a broader range of research methods with a preference for theoretical papers.

Papers did not have a consistent way of reporting demographics. The typical demographics were gender, job title, age, and prior experience in a particular topic of interest for the research (Table 1 ). Out of the papers that reported the location of research or participants, the primary focus was on North America (n=47), and many did not report demographics (n=28) (Table 2 ).
Demographic | Count of HCER-AI papers |
---|---|
Gender | 59 (57%) |
Age | 48 (47%) |
Job title | 43 (42%) |
Prior experience | 21 (20%) |
Education | 16 (16%) |
Ethnicity | 10 (10%) |
Industry type | 6 (0.6%) |
Organization type | 3 (0.3%) |
Income | 3 (0.3%) |
Continent | Count of HCER-AI papers |
---|---|
North America | 47 (29%) |
Asia | 18 (11%) |
Europe | 15 (9%) |
Africa | 3 (2%) |
Oceania | 1 (0%) |
Not applicable | 69 (42%) |
Not reported | 28 (17%) |
Recommendations for Future Research
Future researchers should consider reporting participant demographics to help with replicability and to understand how generalizable the results are. As discussed, there is a lack of consistency when reporting demographics. The community’s own findings emphasize that building an AI that respects its users is highly dependent on the target population (see Section 5.2 for details). Therefore, we see a need to provide a framework for reporting demographics for future researchers if they want to make their research comparable and replicable, which echoes Sambasivan et al . ( 2021b ) ’s finding of: “ the need for incentivizing data excellence in which academic papers should evolve to offer data documentation, provenance, and ethics as mandatory disclosure. ” (Sambasivan et al . , 2021b )
Furthermore, considering conferences that often suffer from an excessive focus on Western societies (Linxen et al . , 2021 ; van Berkel et al . , 2023 ; Yfantidou et al . , 2023 ; Septiandri et al . , 2023 ) , publishing this information will help with research transparency. 1 1 1 For example, based on the data from CHI papers published between 2016 to 2020, “ 73% of CHI study findings are based on Western participant samples, representing less than 12% of the world’s population. ” (Linxen et al . , 2021 ) It may also create a push for extending the research to other populations. Especially with the current trend and growth in HCER-AI research, more people will be influenced by the research done in this community. Thus, additional care is required to make the research inclusive and ensure that everyone is not lumped into the most powerful group, for instance, by contextualizing the findings. To achieve this, one approach could be by encouraging one of the several proposed methods for reporting datasets, models, and participant demographics (e.g., Data Cards (Pushkarna et al . , 2022 ) , Model Cards (Crisan et al . , 2022 ) , Datasheets for Datasets (Gebru et al . , 2021 ) , FactSheets (Richards et al . , 2019 ) , and Data Statements (Bender and Friedman, 2018 ) ). The outcomes of these methods could serve as input for a visualization tool to support easy and quick probing of transparency and inclusivity.
We also want to highlight the invaluable contribution of a group of researchers that shed light on less studied populations, such as India in this case. Five papers in our set had a sample from India with shared authors. Therefore, the community is interested in accepting papers from less studied countries in the Global South. We encourage more researchers to study marginalized populations.
5. Results: Research Themes in HCER-AI
There has been an exponential growth in HCER-AI research in the past few years (Figure 3 ). Based on our thematic analysis of 164 papers in this area, these efforts fall into six main research themes (Figure 4 ): governance (n=94), fairness (n=71), explainability (n=41), human flourishing (n=23), privacy (n=19), and security (n=10). All conferences had at least one paper in each research theme focused on governance and fairness. While AIES and FAccT are smaller, with a narrow focus on AI and its ethical considerations, CHI and CSCW have a slightly more diverse research portfolio, possibly because they are larger conferences and attract a broader audience (human-computer interaction broadly defined).

We created a pairwise similarity matrix for the pairs of research themes. Based on Figure 5 , we observed that privacy and security, as well as fairness and governance, are the most common pairs (the closer the number gets to 1, the more likely the pair appears together in a paper). On the other hand, many pairs, such as governance with security, privacy, and human flourishing, did not appear together in our reviewed papers. For example, papers on governance often had a more general view of AI, except for some overlapping research with fairness and explainability. Across the four conferences, security had the least number of published papers. One possible explanation for this is the well-established nature of security as a field, particularly within computer science. Prominent conferences covering human factors, such as USENIX Security and IEEE S&P, may attract papers at the intersection of security and responsible AI. Future research may explore similar areas in privacy and security conferences with human subjects.

In the following we discuss each theme, with the number of resources in each theme indicated next to the theme title ( #N ).
5.1. Governance (#94)
All conferences actively publish papers related to AI governance. Aside from general guidelines and critical views on AI governance, papers here fall into two sub-themes: guidelines about how AI should work, be governed, and regulated (Muller and Strohmayer, 2022 ; Langer et al . , 2022 ; Maas, 2018 ; Erdélyi and Erdélyi, 2020 ; Whittlestone et al . , 2019 ; Schiff et al . , 2020 ; Henriksen et al . , 2021 ; Costanza-Chock et al . , 2022 ; Ha, 2022 ; Weidinger et al . , 2022 ; Stapleton et al . , 2022 ; Knowles and Richards, 2021 ; Abdalla and Abdalla, 2021 ; Pushkarna et al . , 2022 ; Smith et al . , 2022 ; Crisan et al . , 2022 ; Sloane and Zakrzewski, 2022 ; Ashurst et al . , 2022 ) ; and tools for auditing AI (Henriksen et al . , 2021 ; Krafft et al . , 2021 ; Kroll, 2021 ; Ramesh et al . , 2022 ; Black et al . , 2022 ; Costanza-Chock et al . , 2022 ; Knowles and Richards, 2021 ) .
5.1.1. AI Guidelines
The three main stakeholders in the AI ecosystem are individuals (e.g., users, developers, and AI experts), organizations (e.g., ACM, companies, and independent institutes), and national and international bodies (e.g., UNICEF and regulators) (Deshpande and Sharp, 2022 ; Whittlestone et al . , 2019 ; Wang et al . , 2023 ) . 2 2 2 Despite the increasing interest in more collectivist views (McNaney et al . , 2018 ; Vitos et al . , 2017 ; Vigil-Hayes et al . , 2017 ; Peters et al . , 2013 ; Reinecke et al . , 2013 ) , these considerations were not present in our AI-centric search results. Several organizations and communities have already started to respond to this urgent need by sharing responsible AI guidelines (Varanasi and Goyal, 2023 ; Wang et al . , 2023 ) . These guidelines are typically used not only for addressing AI’s design challenges but also for education, cross-functional communication, and developing internal resources, as illustrated in a study with Google’s People + AI Guidebook (Yildirim et al . , 2023 ) . From a theoretical standpoint, AI can raise several tensions between ethical values. For instance, improving service quality may require additional data collection from users (Whittlestone et al . , 2019 ) . These trade-offs are further confirmed by people who work for or co-founded a startup where AI entrepreneurs face a dilemma between academic integrity and potentially overrated marketing campaigns (Winecoff and Watkins, 2022 ) . Addressing these trade-offs requires understanding AI’s ethical challenges (Schiff et al . , 2020 ; Eicher et al . , 2018 ) , developing principles for precautionary policy making (Ha, 2022 ; Terzis, 2020 ) , educating students about AI at schools (Lin and Van Brummelen, 2021 ) , and building practical recommendations for the safe and trustworthy deployment of AI (Maas, 2018 ; Eicher et al . , 2018 ; Baughan et al . , 2023 ; Burgess et al . , 2023 ) . Previous actions and strikes by employees in other industries represent one direction that may support promoting ethical values in the AI industry, but they require more commitment from employees than just open letters and petitions (Boag et al . , 2022 ) . As existing legal liability systems fall short in assigning responsibility when potentially harmful conduct occurs from using AI, the need to establish AI schemes that ensure responsible development of AI is further highlighted (Erdélyi and Erdélyi, 2020 ; Bietti, 2020 ) . Considering individual experiences, empirically understanding needs, and breaking traditional abstract views of society to enable research communities to be self-reflective and mindful of the previously mentioned trade-off in AI are directions that we, as researchers, need to take to move toward ethics in AI (Siapka, 2022 ; Washington and Kuo, 2020 ; Stark and Hoey, 2021 ; Rismani et al . , 2023 ; Shahid and Vashistha, 2023 ) .
Funding sources often drive academic research. AI research is no exception; hiring graduate students and researchers requires faculty and universities to attract funding to recruit individuals for research. The AI industry’s indirect influence on the governance of AI may come from these fundings that shape academic research (Abdalla and Abdalla, 2021 ; Young et al . , 2022 ) . In a sample of 149 faculties from four prestigious North American universities, about half of those with known funding sources received money from large technology companies like Google and Microsoft. This type of influence resonates with the tobacco industry’s influence when academic research was exploited to shift the public’s negative opinion toward the benefits and positives of tobacco (Abdalla and Abdalla, 2021 ) . The arbitrary reporting of limitations in AI research exacerbates such concerns. The machine learning research community lacks a single, agreed-upon definition of limitations and does not have a standardized process for disclosing and discussing limitations, in addition to often being non-inclusive (Chi et al . , 2021 ) and lacking model -work documentation (Crisan et al . , 2022 ) or data-work documentation (Sambasivan et al . , 2021b ; Muller and Strohmayer, 2022 ) . On a positive note, recommendations to fill these gaps exist. REAL ML, for instance, provides a set of guided activities to help machine learning researchers recognize, explore, and articulate the limitations of their research (Smith et al . , 2022 ) . Alternatively, frameworks have been proposed to disentangle different components of ethical research in machine learning to allow AI researchers, practitioners, and regulators to systematically analyze existing cultural understandings, histories, and social practices of ethical AI (Sloane and Zakrzewski, 2022 ; Ashurst et al . , 2022 ) , guiding the creation of human-AI teams (Flathmann et al . , 2021 ) .
In addition to the body of research on more organized and private AI companies and research institutes, research on open-source communities shows that the nature of open-source and unrestricted use of code propagates within the AI open-source community as well (Widder et al . , 2022 ) . Working on open-source AI projects creates a sense of neutrality and inevitability toward the technology and its consequences, while there can be serious ramifications to these projects, such as use cases for deep fakes. Similar approaches such as documentation (Miceli et al . , 2022 ) and nudging developers into responsible development are suggested to alleviate the harm (Widder et al . , 2022 ) . However, the community’s adoption of these guidelines and frameworks is still unknown.
5.1.2. Tools for AI Auditing and Research
This category of papers aims to aid experts, activists, and independent bodies in improving the quality and impact of auditing AI (Costanza-Chock et al . , 2022 ; Lam et al . , 2023 ) (especially in the age of large language models (Zhou et al . , 2023 ) ) , to understand accountability as a responsibility put on the shoulders of AI engineers (Henriksen et al . , 2021 ) , to discuss the right of contestability (Lyons et al . , 2021 ; Yurrita et al . , 2022 ) , and to engage with theories from moral philosophy (Nashed et al . , 2021 ) . At the initial stages of a project, design cards (Elsayed-Ali et al . , 2023 ) can help bring knowledge in areas such as creative inspiration, human insights, problem definition, and team building (Hsieh et al . , 2023 ) . Other tools include computational notebooks (Ayobi et al . , 2023 ) to help practitioners explore machine learning models, workflows that encourage designers to explore model behavior and failure patterns early in the design process (Moore et al . , 2023 ) , data probes to help practitioners surface the well-being and positionalities that shape their work strategies (Lee et al . , 2023a ) , and exhibitions to teach critical thinking (Lee et al . , 2023a ) . Another idea for creating a foundation for audit is to apply the tracing and debugging mechanism from software engineering (Kroll, 2021 ; Balayn et al . , 2023 ) . Version control systems keep track of changes in code repositories, and similar ideas could be applied in auditing AI, which requires structure, logs, and wide adoption (Kroll, 2021 ) . Besides technical documentation about how things work and their respective risks (Pushkarna et al . , 2022 ; Weidinger et al . , 2022 ) , many users (average users without technical knowledge and interest in how systems work) are interested in what the system does, how it can affect them, and whether it is fundamentally reasonable to replace the traditional mechanism with AI (Knowles and Richards, 2021 ; Stapleton et al . , 2022 ) .
Only by comprehending the foundations of algorithmic decision-making can we establish legitimate control over algorithmic processes (Burrell et al . , 2019 ) . Recently, scholars have begun to discuss the right to contestability, which entails the ability to contest algorithmic decisions (Lyons et al . , 2021 ; Alfrink et al . , 2023 ; Yurrita et al . , 2022 ) . For example, a study conducted through a series of participatory design workshops found that by designing for contestability, users can actively shape and exert influence over algorithmic decision-making (Vaccaro et al . , 2021 ) . Another study showed that, in high-stakes AI, physicians’ trust in AI was less dependent on their general acceptance of AI but more on their contestable experiences with AI (Verma et al . , 2023 ) . Another large body of work aims to engage AI practitioners with ethical theories. For example, by expanding and defending the Ethical Gravity Thesis, scholars proposed a framework that allows one to situate ethical problems at the appropriate level of abstraction, which can be used to target appropriate interventions (Kasirzadeh and Klein, 2021 ) . Another study provided a mathematical framework to model how much learning is required for an intelligent agent to behave morally with negligible error (Shaw et al . , 2018 ) , while another developed a computational model for building moral autonomous vehicles by learning and generalizing from human moral judgments (Kim et al . , 2018 ) .
Future research could study the specifics of governing other research themes in depth. Most of the current governance research is general except for fairness; therefore, studying the governance of AI with respect to privacy, security, human flourishing, and explainability is needed (Figure 5 ). Furthermore, providing usable documentation and communicating AI’s abilities and implications to the public to create trust in AI is necessary and needs research beyond simple explanations that may cause over- or under-trust issues (Liao and Sundar, 2022 ) . One suggestion is to involve users and communities in helping situate the requirements within the target individuals and groups (Ramesh et al . , 2022 ; Sambasivan et al . , 2021a ) . The rise of “user-driven audits” on social media such as Twitter (i.e., users hypothesizing, collecting data, amplifying, contextualizing, and escalating AI issues and possible harms) can provide a window into people’s expectations and provide a flow of integration of AI by the communities (Wang et al . , 2019b ) .
The industry’s impact on academic integrity is a known issue. In privacy, for example, similar influences have been observed, discussed, and documented (Waldman, 2021 ) . As pointed out in the research methods of HCER-AI (§ 4 ), transparency in data collection, reporting data characteristics, and funding resources with an on-demand visualization tool to provide an overview of these aspects could help. While participatory design approaches have often been used for user-originated or worker-originated critiques of technologies, it is not yet clear whether and how they can be applied to complex algorithmic systems (Delgado et al . , 2022 ; Neuhauser and Kreps, 2011 ; Zytko et al . , 2022 ) . Designers of design cards also need to further extend the scope of these tools to evaluation and later stages of AI projects beyond the current design cards that focus on ideation and initial stages.
5.2. Fairness (#71)
Scholars and practitioners argue that fairness is contextual, cultural, and individually dependent, among many other dimensions; one size does not fit all (Docherty and Biega, 2022 ; Kapania et al . , 2022 ; Wang et al . , 2022c ; Cheng et al . , 2021 ; Lee and Rich, 2021 ; Sambasivan et al . , 2021a ; Kasirzadeh and Smart, 2021 ; Richardson et al . , 2021 ; Ashktorab et al . , 2023 ; Lewicki et al . , 2023 ; Sharma et al . , 2021 ; Aka et al . , 2021 ; Cruz Cortés and Ghosh, 2020 ; Yang et al . , 2022 ; Deng et al . , 2023 ) . Therefore, when designing experiments, contextual considerations should be considered to reduce errors in research design and results (Kapania et al . , 2022 ; Niforatos et al . , 2020 ; Ashktorab et al . , 2023 ) . For example, conclusions about individuals’ perceptions or expectations of fairness may not be replicable when the country, age, or gender group changes (Kapania et al . , 2022 ; Wang et al . , 2022c ; Simons et al . , 2021 ) . Some biases are inherent in practitioners’ (Ashktorab et al . , 2023 ) or data annotators’ (Kapania et al . , 2023 ) backgrounds, and some are also present in the inevitable changes to datasets and models during AI development (Muller and Strohmayer, 2022 ; Muller et al . , 2021 ) . For example, during the data annotation and curation process, when raw data is prepared for the final model, disagreement between annotators may get lost, resulting in the final ground truth being based on the agreement biased towards a certain annotator or group (Muller and Strohmayer, 2022 ; Muller et al . , 2021 ) and questioning its credibility (Chen and Sundar, 2023 ) . This is why scholars proposed new AI data curation approaches grounded in feminist epistemology and informed by critical theories of race and feminist principles (Leavy et al . , 2021 ) . While the field of algorithmic fairness has primarily explored the notion of fairness as treating individuals alike or arguing for social inclusion (Huang and Liem, 2022 ) , there has been a recent debate on the concept of vertical equity —appropriately accounting for relevant differences across individuals—which has also been a central fairness component in many public policy settings (Black et al . , 2022 ) . The empirical notion of fairness from non-experts also shows that people may accept an AI making inferences about portraits when the use case is advertising but do not find it permissible for AI to make inferences about an individual based on their gender, age, or race, demonstrating the complexity of fairness (Engelmann et al . , 2022 ) .
Terminology (e.g., artificial intelligence, algorithm, and robot) also impacts individuals’ perceptions of fair AI and how they trust the system’s decisions. Therefore, when researchers conduct research with human participants and use various terms to refer to an automated decision-making system, participants may have a mixed understanding and expectation of trust and fairness from the system when the terms addressing the studied system change (Langer et al . , 2022 ) . Another example that can impact participants’ perceptions of an AI’s fairness is people’s lived experiences. When a marginalized group has low trust in a human decision-maker for historical reasons (e.g., not trusting a medical doctor’s decision), people have similar expectations of fairness from an AI (e.g., (Lee and Rich, 2021 ; Nakao et al . , 2022 ; Sambasivan et al . , 2021a ) ) .
Conversely, if an individual has a positive historical experience with the human decision-maker (e.g., trusting a medical doctor’s decision), the individual may place higher trust in the AI’s decisions as well (Lee and Rich, 2021 ) . While in other situations, people often consider AI more capable than humans but less morally trustworthy (Tolmeijer et al . , 2022 ) . Overall, human agents are often held responsible in decision-making (Tolmeijer et al . , 2022 ; Lima et al . , 2021 ) . To alleviate the gap between designers and users of AI, designers could bring users and diverse viewpoints into the design stages of AI to create a shared understanding of fairness between different stakeholders (Yildirim et al . , 2022 ; Subramonyam et al . , 2022 ; Park et al . , 2022 ; Raz et al . , 2021 ; To et al . , 2021 ; Choi et al . , 2023 ; Burrell et al . , 2019 ) . To further consider biases in the deployment stages, humans can be integrated into the loop with AI (Cheng et al . , 2022 ; Reitmaier et al . , 2022 ; Raz et al . , 2021 ) , resulting in human-algorithm collaboration (Donahue et al . , 2022 ; Zheng et al . , 2023 ) . For example, in deciding whether a child was maltreated, when AI decisions were combined with a human decision-maker, the number of racially biased decisions was reduced compared to when AI was the only decision-maker. Hence, the human in the loop may improve decisions by taking an overarching assessment and filling AI’s limitations in decision-making (Cheng et al . , 2022 ) .
Future research could study fairness from multiple perspectives (e.g., culture, gender, ethnicity, age) and compare expectations or understandings in various groups ( Lee and Rich , 2021 ; Nakao et al . , 2022 ; Sambasivan et al . , 2021a ) . Multiple studies show the value of diversity in AI development and research teams to uncover potential biases and gaps, a practice that academics and industry need to adopt (Septiandri et al . , 2023 ; Ashktorab et al . , 2023 ) . Furthermore, the studied toolkits provide metrics to evaluate fairness, primarily for AI developers (or experts). Researching how to present these metrics to a non-expert user who is impacted by the system could be a direction to pursue: how to present fairness to the public with minimal knowledge about fairness (e.g., is statistical parity the most understandable way of presenting fairness to a senior adult or a child about the fairness of an AI?). These tools can be helpful for non-expert users to understand AI decisions and make informed decisions, as typically, interactions between people and machines range between two extremes: humans either tend to under-rely on an algorithm by ignoring its recommendations (algorithmic aversion) or over-rely on it by blindly accepting any recommendation (automation bias) (De-Arteaga et al . , 2020 ; He et al . , 2023 ) . Additionally, some scholars have argued that an AI can somehow decide “objectively,” (Houser, 2019 ) . In contrast, others have argued that humans insert their biases into the data they wrangle and the models they build (Muller and Strohmayer, 2022 ) . The former “objectivity” argument could lead to expectations of better treatment by an AI rather than a person. The latter “bias” argument could lead to expectations of equally bad or worse treatment by an AI. While toolkits for audit are focused on fairness, we believe there is space to explore practices and build toolkits for audit and accountability in other aspects like privacy and security. Another aspect to consider is that accountability could provide explainability over decisions but make people feel surveilled (Ehsan et al . , 2021a ) ; therefore, finding a balance between accountability, privacy, and fairness is a challenge that requires further exploration.
5.3. Explainability (#41)
If an AI operates as an opaque box, 3 3 3 Where a decision is generated without explanation or interpretation, preventing users from understanding why and how the decision was made users may have trouble trusting the outputs. Consequently, a recent movement in AI seeks to make these decisions comprehensible to garner users’ trust (Liao et al . , 2020 ; Bertrand et al . , 2022 ; Kim et al . , 2023 ; Yuan et al . , 2023 ) , and explores ways to measure their impact (Cabitza et al . , 2023 ) . In human resource management, decisions made by AI can be further elucidated to gain employees’ trust, helping them understand the rationale behind a decision with clear evaluation criteria (which may instill a sense of fairness) (Park et al . , 2021 , 2022 ) . However, explanations may not always absolve the AI of blame (Lima et al . , 2023 ) . Often, trust issues depend on the personal relevance of the explanations and what is at stake (Yuan et al . , 2023 ) , or on the lack of tools assisting AI practitioners in generating meaningful explanations (Liao et al . , 2023 ) . Paradoxically, explainability can sometimes conflict with privacy and security (Hall et al . , 2022 ; Park et al . , 2022 , 2021 ) . We further discuss this trade-off in Sections 5.5 and 5.6 .
However, explanations need to be human-centered, usable, and contextual (Liao et al . , 2020 ; Bansal et al . , 2021 ; Ehsan et al . , 2021a ; Long et al . , 2022 ; Zhang et al . , 2022 ; Raz et al . , 2021 ; Lee et al . , 2021 ; Toreini et al . , 2020 ) and may even need to be augmented with more than just simple text (Yang et al . , 2023 ) . Otherwise, they could create a false sense of control or be used as a scapegoat for responsibility (Lima et al . , 2022 ) . For example, when explanations were employed in a human-AI team, they did not help improve the accuracy of the final decision (Bansal et al . , 2021 ) . However, when combined with an opaque box model that elucidated what the system does, users’ confusion was reduced (Bell et al . , 2022 ) . In either case, not all explanations are beneficial. To enhance explanations, AI developers could profit from posing how and why not questions to consider users’ expectations and needs (Liao et al . , 2020 ) , and could also utilize participatory design approaches (Lee et al . , 2021 ) . Similar to asking the “how” questions, an alternative framework, Sensible AI (Kaur et al . , 2022 ) —for interpretability was proposed, grounded in Weick’s sensemaking theory (Weick, 1995 ) . From organizational studies, sensemaking describes the individual, environmental, social, and organizational context affecting human understanding. Similarly, Sensible AI translates these properties into the human-machine context. Another way to improve explanations is to add graphics and control sliders to help users understand an AI’s decision, making them feel more in control (Viswanathan et al . , 2022 ) . Explanations can also help improve AI literacy for public and educational purposes, potentially leading to broader adoption and understanding of AI implications and harms (e.g., teaching kids about feature selection in machine learning using visual explanations) (Long et al . , 2022 ) . Another study examined the role of cognition in understanding explanations (Buçinca et al . , 2021 ) , proposing three cognitive forcing interventions to compel people to engage more thoughtfully with the AI-generated explanations.
Future research is still needed to improve the explainability of AI’s inner workings, decisions, and outputs, as there is some criticism about the value of explanations like SHAP for humans (Kumar et al . , 2020 ) . Toolkits for communicating datasets also need to track changes over time, from raw data to the final dataset used in the model, and changes that occur post-deployment, to mitigate the forgettance of data work—as Muller and Strohmayer ( 2022 ) put it: “ Forgettance in data science is when each action tends to push previous actions into the infrastructure, where the action itself and its consequence are easily forgotten. ” (Muller and Strohmayer, 2022 ) One way of documenting datasets is through a standardized process, such as using datasheets (Gebru et al . , 2021 ) , nutrition labels (Chmielinski et al . , 2022 ) , or data statements (Bender and Friedman, 2018 ) . Furthermore, taking a cue from computer hardware and industry safety standards, datasheets for datasets should include dataset provenance, key characteristics, relevant regulations, test results, and also more subjective yet noteworthy information (e.g., the potential bias of a dataset (Gebru et al . , 2021 ) ).
5.4. Human Flourishing (#23)
Sustainable growth and the well-being of humans (also known as human flourishing) using or being affected by AI were discussed in several studies (Wang et al . , 2022c ; Docherty and Biega, 2022 ; Wang et al . , 2022b ; Steiger et al . , 2021 ; Beede et al . , 2020 ; Morrison et al . , 2021 ; Lee et al . , 2021 ) . For example, if an employer wants to increase productivity using AI from a sustainable perspective, employees’ expectations should be considered alongside implementing positive changes, such as monetary compensation (Park et al . , 2022 ) . AI can also facilitate real-time communication for users of augmentative and alternative communication (Valencia et al . , 2023 ) . AI’s impact on children has been deemed crucial due to its potential for long-term effects. Instances of using robots for education at early stages of child development can shape children’s perceptions of robots. Moreover, entertainment systems that collect children’s data may affect their entire life if service providers do not respect their privacy and keep their data indefinitely (Wang et al . , 2022c ) . 4 4 4 Adults are also susceptible to persuasion by, for example, “cute” – but lethal – robots (Kemper and Kolain, 2022 ; Vaisman, 2021 ; Young, 2021 ) . In HCI literature, safety drivers working with Autonomous Vehicles (AV) often comprise an under-explored population impacted by AI. A study found that, as front-line workers, safety drivers are forced to shoulder risks accumulated from the upstream AV industry while confronting restricted self-development prospects when working for AV development (Chu et al . , 2023 ) . Similarly, socio-environmental factors must also be considered for the successful implementation of AI in real-world settings (e.g., a blind person navigating social interactions or the country of post-deployment) (Beede et al . , 2020 ; Morrison et al . , 2021 ) . For example, offering explanations and options for employers to customize an AI-based scheduling system resulted in a smoother transition to AI and less resistance from users (Lee et al . , 2021 ) .
A few papers focused on the labor behind AI (Wang et al . , 2022b ; Steiger et al . , 2021 ; Klinova and Korinek, 2021 ) . AI creates new markets and opportunities which bring wealth to those who will be more in demand (e.g., AI developers) and shrinks the wealth of those who will be less in demand (e.g., customer service agents replaced by an AI chatbot) (Klinova and Korinek, 2021 ) . However, new AI markets may not always be accommodating and lucrative for all. As part of the data creation process, annotators often work long hours with low wages, especially when cost-effective methods of annotating large datasets are in demand, leading AI companies to hire annotators from third-party annotation companies in the Global South for poor wages. In these companies, some annotators start working with the expectation that annotation work will open up opportunities for high-paying jobs in the future and serve as a stepping stone to becoming an engineer. However, this is often a fallacy, and these annotators rarely progress in their careers (Wang et al . , 2022b ) . Similarly, content moderators are sometimes exposed to inappropriate or disturbing content, causing severe stress and trauma. Some of these individuals were not fully aware of the risks associated with the job when they signed up for it due to a lack of transparency in the job description (Steiger et al . , 2021 ) .
Either of these jobs (annotation or content moderation) is often perceived as a “dirty” job and receives minimal attention from companies (Steiger et al . , 2021 ; Wang et al . , 2022b ; Sambasivan et al . , 2021b ) , or as “patchwork,” that is, the human labor that occurs in the space between what AI purports to do and what it actually accomplishes (Fox et al . , 2023 ) . Nevertheless, their contributions to the AI economy are invaluable 5 5 5 “ Without the work and labor poured into the data annotation process, ML efforts are no more than sandcastles. ” (Wang et al . , 2022b ) and foundational (by generating datasets and keeping AI safe from inappropriate content that others do not have to deal with). The following four recommendations can serve as a starting point to help these individuals manage their jobs’ challenges (Gray and Suri, 2019 ; Moreschi et al . , 2020 ; Steiger et al . , 2021 ) : (1) providing a detailed job description outlining its associated risks could give candidates an understanding of whether the job is appropriate for them, (2) limiting the number of contents they view or annotate per day or week, (3) creating a supportive community to help with stress relief, and (4) companies should consider offering employee benefits, including physical and mental health benefits, to these ”ghost” workers.
Future research could study the long-term effects of AI on people, the people who directly use AI (e.g., a person using a fitness tracker with an AI coach (Garcia and Cifor, 2019 ) ), those who are indirectly affected by AI (e.g., a person receiving an AI-assisted loan decision from a credit institute (Hertzberg et al . , 2010 ; Kizilaslan and Lookman, 2017 ) , or the people who may lose their jobs or fear that they may lose their job because of AI (Josie Cox, 2023 ) ), and the people involved in AI’s development and deployment (e.g., annotators and developers). A longer view of the impacts of AI algorithms could include neighborhoods affected by over-policing through AI technologies (Gee, 2021 ) . For instance, as AI in workplaces becomes a technological intervention, which may have different impacts on different groups within that workplace (Park et al . , 2021 , 2022 ) , multiple workplace scenarios can be analyzed through value-sensitive design (Muller and Weisz, 2022 ) . Docherty and Biega ( 2022 ) argue that the tech industry’s focus on user engagement and time spent on the platform is a reductionist view of human well-being. When measuring well-being, an individual’s specific needs and characteristics should be considered. Building on this idea, future researchers could study the well-being of people impacted by AI beyond limiting engagement time and think about human flourishing (i.e., “ the ability to live a good life ” (Health Equity & Policy Lab, 2022 ) ) from a positive computing viewpoint (Calvo and Peters, 2014 ) . Furthermore, although not discussed in the reviewed papers, we see a need to study AI’s environmental effects on natural resources from a nature-centered perspective. For instance, a tool could be designed to remind users about the energy consumption of their AI tools or to provide information about the energy consumption of AI models (including all stages, such as design, development, and deployment) with an easy-to-understand label, like the Energy Star.
5.5. Privacy (#19)
Several papers have touched on the privacy ramifications of AI (Mlynar et al . , 2022 ; Lee and Rich, 2021 ; Zhang et al . , 2022 ; Ehsan et al . , 2021a ; Park et al . , 2022 , 2021 ; Yildirim et al . , 2022 ; Subramonyam et al . , 2022 ; Wang et al . , 2022c ; Hall et al . , 2022 ) . For example, the use of human resource management tools in companies, which bear privacy implications, can make employees uncomfortable. These systems may be as simple as controlling a gate and storing that information for performance measures. However, collecting more data to improve the model’s accuracy can induce a sense of surveillance (Greiffenhagen et al . , 2023 ; Constantinides and Quercia, 2022 ) . Nevertheless, from the viewpoint of those deploying the system, such data collection may be seen as a means of providing a fair assessment of the employee’s performance (Park et al . , 2022 , 2021 ) . Such systems may create conceptual mismatches between normative (or cultural) and legal expectations regarding the use of personal data (Nielsen, 2021 ) . Designers and engineers acknowledge this tension between privacy and collecting more data or sharing datasets between different groups or teams to explore new opportunities. In fact, there is evidence that while people support the sharing of their data to improve technology, they also express concerns over commercial use, associated metadata, and the lack of transparency about the impact of their data (Kamikubo et al . , 2023 ) . Nonetheless, when a company is a large organization (especially when client data is involved), sharing data is difficult because data ownership can be vague and challenging (Yildirim et al . , 2022 ; Subramonyam et al . , 2022 ) . In contrast, smaller companies grapple with several tensions, for example, the trade-off between privacy and ubiquity, resource management and performance optimization, or access and monopolization (Hopkins and Booth, 2021 ) .
A few papers discuss the trade-off between privacy and explainability (Zhang et al . , 2022 ; Ehsan et al . , 2021a ; Hall et al . , 2022 ) . Providing additional information about the model for explainability may compromise privacy or vice versa. For instance, in a study with people’s WiFi data, participants were initially private and sensitive about sharing their WiFi data. However, showing visualizations about their data usage made them feel more comfortable sharing their data because it fostered a sense of trust (Hall et al . , 2022 ) . On the other hand, privacy can become a barrier to providing explanations in image classification systems. For example, when images are obfuscated for privacy reasons, providing explanations for the classification may reveal the identities of people in the images (Zhang et al . , 2022 ) . Other scholars have focused on how privacy legislation is discussed among different sets of relationships (e.g., between companies and investors (Wong et al . , 2023a ) ) through the lens of law and ethics (Benthall and Goldenfein, 2021 ) . For example, a study found that startups with data-sharing partnerships with high-technology firms or prior experience with privacy regulations are more prone to adopting ethical AI principles (Bessen et al . , 2022 ) . Furthermore, they are more inclined to take costly actions, such as eliminating training data or rejecting business opportunities, to adhere to their ethical AI policies.
Future research may explore the trade-off between privacy (and/or security) and explainability, as there is a thin line between what can be monitored and what should be monitored (Constantinides and Quercia, 2022 ) . One perspective is to look at this trade-off from the lens of historian and philosopher Yuval Noah Harari, who posits that the digital platforms, whether powered by AI or not, need to adhere to three basic rules to protect humans from “ digital dictatorships ” (Yuval Noah Harari, 2021 ) . These rules are (1) reasonable use cases for data collection, meaning that any data being collected should be used to assist people rather than manipulate, control, or harm them (e.g., providing Indigenous data sovereignty over culturally-sensitive data (Kukutai et al . , 2020 ; Marley, 2019 ; Tsosie, 2019 ; Walter and Suina, 2019 ) ), (2) surveillance should be bidirectional, implying that if an entity (e.g., an organization or a government) increases surveillance of individuals, accountability on the entity’s side should correspondingly increase, and (3) the elimination of data monopolies, which arise from the concentration of data in a single entity. Additionally, the General Data Protection Regulation (GDPR) (The European Parliament and the Council of the European Union, 2018 ) in Europe argues for limiting the storage time only to legitimate purposes and doing regular reviews to delete unneeded data. Human-centered approaches and design methods can be employed to explore solutions for these problems through committees that oversee data collection and, ideally, ensure that data is in the hands of the individuals.
5.6. Security (#10)
AI security aims to ensure safety and reduce harm to individuals (Wang et al . , 2022c ; Toreini et al . , 2020 ) . It involves enhancing a system’s ability to resist external threats by testing its resilience against vulnerabilities and cyber-attacks, all while safeguarding the integrity and confidentiality of personal data (Fjeld et al . , 2020 ) . However, security may conflict with explainability. For instance, in the case of human resource management systems, disclosing information about models for explainability purposes could pose a security risk. Such openness could harm the organization’s reputation and provide attack opportunities if all model details are publicly available (Park et al . , 2022 , 2021 ) . Furthermore, using AI in security-sensitive decision-making can be questionable. If AI decisions yield many false positives, reliance on them can create unwanted stress, as the operator in charge of making the final decision might feel compelled to act on them. This can be particularly stressful for junior staff who may feel obliged to report every incident (Ehsan et al . , 2021a ) .
Future research may benefit from studying the security aspects of AI from a human-centered perspective. While there is a specific security and privacy track in CHI that publishes papers about the human factor of security and privacy in various technologies such as smartphones, apps, and browsers, and investigates the role of software developers in secure development, we believe the community can benefit from a dedicated focus on what it means to provide human-centered security in AI. This should consider the trade-off between explainability and security, which echoes the classic trade-off between usability and security (Cranor and Garfinkel, 2005 ; Tahaei and Vaniea, 2019 ) . Creating usable security systems is often challenging. In response to this challenge, a dedicated venue, the Symposium on Usable Privacy and Security (SOUPS), was established in 2005. Implementing more complicated security (or privacy) mechanisms can burden users, deter them from using the system, clash with their mental models, and lead to insecure actions. Similarly, providing more explanations for AI outcomes may cause users to overtrust the system. Therefore, finding the right balance between the two calls for further research.
6. Results: Patents of HCER-AI
The current state of patents in HCER-AI is encapsulated by the following themes: explainability (n=13), fairness (n=11), governance (n=6), human flourishing (n=4), privacy (n=4), and security (n=2). The themes in patents and research papers somewhat follow each other, except for governance being the major theme in research, which is expected because research venues cover theoretical, review, and discourse papers, while patents are more on the industry application level (Table 3 ). Also, given that the first academic paper on the subject was published in 2018, it is unsurprising to see a small set of patents in this area. HCI research takes 10.5 years to appear in a patent application, and this lag is increasing over time (Cao et al . , 2023 ) . The exponential rise in research papers in HCER-AI presents an opportunity for early-adopter industries to work on related patents.
Governance | Fairness | Explainability | Human flourishing | Privacy | Security | |
---|---|---|---|---|---|---|
Research papers | 94 (57%) | 71 (43%) | 41 (25%) | 23 (14%) | 19 (12%) | 10 (6%) |
Patents | 6 (21%) | 11 (39%) | 13 (46%) | 4 (14%) | 4 (14%) | 2 (7%) |
7. Mapping the HCER-AI Landscape With the NIST AI Risk Management Framework
We also mapped our constructed HCER-AI research themes onto the U.S. National Institute of Standards and Technology (NIST) Artificial Intelligence Risk Management Framework, published in 2023. We chose NIST because it is a recent framework from a renowned organization for developing frameworks and standards. Alternatives include the Principled Artificial Intelligence from the Berkman Klein Center (Fjeld et al . , 2020 ) , which aligns with the NIST framework. Such mapping provides insights into the different perspectives on the landscape from the viewpoints of a standardization body versus academic research. This approach helps highlight areas needing further attention, potentially directing more funding and focus toward those areas to balance the research portfolio.
Governance (n=94): maps with NIST’s “Govern” function: “ cross-cutting function that is infused throughout AI risk management and enables the other functions of the process ,” “Accountable and Transparent” risk: “ accountability presupposes transparency. Transparency reflects the extent to which information about an AI system and its outputs is available to individuals interacting with such a system – regardless of whether they are even aware that they are doing so ,” and “Valid and Reliable” risk: “ Validity and reliability for deployed AI systems are often assessed by ongoing testing or monitoring that confirms a system is performing as intended .”
Fairness (n=71): maps with NIST’s “Fair – with Harmful Bias Managed” risk: “ includes concerns for equality and equity by addressing issues such as harmful bias and discrimination .”
Explainability (n=41): maps with NIST’s “Explainable and Interpretable” risk: “ refers to a representation of the mechanisms underlying AI systems’ operation, whereas interpretability refers to the meaning of AI systems’ output in the context of their designed functional purposes .”
Human flourishing (n=23): AI should support “ happiness and life satisfaction, meaning and purpose, character and virtue, and close social relationships .” (Willen et al . , 2022 ) This theme also maps loosely with the NIST’s “Safe” risk: “ AI systems should not under defined conditions, lead to a state in which human life, health, property, or the environment is endangered .”
Privacy (n=19): maps with NIST’s “Privacy-Enhanced” risk: “ refers generally to the norms and practices that help to safeguard human autonomy, identity, and dignity .”
Security (n=10): maps with NIST’s “Secure and Resilient” risk: “ AI systems, as well as the ecosystems in which they are deployed, may be resilient if they can withstand unexpected adverse events or changes in their environment or use .”
8. Posteriori Analysis Using ChatGPT 4.0
Motivated by recent work on using AI for qualitative analysis (Byun et al . , 2023 ; Abram et al . , 2020 ; Tahaei et al . , 2020 ) , we explored the possibilities of using ChatGPT in a similar analysis without manual analysis based only on the abstracts (because of input limitations of AI models). We used all the abstracts of the 164 papers as input for ChatGPT to evaluate its ability to produce a similar report to ours. The primary tasks were finding research themes, methods, and recommendations (our RQs). Noting that, we used ChatGPT 4.0, like any other text clustering and classification tool, to probe for topics we might have missed during our research. This process was a complete posthoc procedure after human authors wrote and finalized all the paper sections except this one. All of our prompts and all the content, besides the main headlines and topics, produced by ChatGPT are intentionally left in Appendix B to avoid confusion between our analysis and findings vs. AI-generated analysis and findings.
We first asked for research themes from the abstract following the thematic analysis proposed by Braun and Clarke ( 2006 ) (our approach). ChatGPT came up with the following four research themes: ethical implications of AI, practical applications of AI, understanding and documenting data, and user engagement. We further asked for the research methods used in the abstracts with a caveat that not all papers mention their methods in their abstracts. The answer was more nuanced than the research themes: interviews, participatory design, co-design workshops, prototyping, observation, critical discourse analysis, iterative co-design activities, characterization of collective actions, and case studies. We followed the exploration with research recommendations and future research directions based on the thematic analysis. The following recommendations were generated: user-centric design, greater transparency in AI, AI in marginalized communities, collective action in tech industry, real-world AI evaluations, AI in disaster risk management, effective AI communication, AI ethics integration in education, work-integrated learning for AI, and AI governance practices. We did the same analysis for research themes of patents; the resulting themes were: AI-driven decision making and predictions, transparency, interpretability, and explainability in AI, and machine learning model performance & enhancement.
The research themes produced by ChatGPT (ethical implications of AI, practical applications of AI, understanding and documenting data, and user engagement) could help get a general understanding of the dataset but were not enough to get an in-depth grasp of the data. Our research themes (governance, fairness, explainability, human flourishing, privacy, and security) are also a construct of our positionality (see Section 3.1 ). Despite the differences in both lists, AI governance and AI’s ethical implications are visible. We also recognize that the classification generated by AI does not constitute a compelling taxonomy, with some themes containing others without being of the same level of importance. Possibly, because the AI-generated themes may be meant for general consumption and do not directly correlate with specific research areas of our analysis. Overall, we speculate that AI-assisted research tools like ChatGPT may, in the future, act as a quick secondary point to help researchers with unseen perspectives—considering that it took us less than two hours to produce these results compared to months of work for the rest of the paper. Future research may explore how researchers can better leverage AI to deliver valuable literature reviews by conducting more experiments, comparisons with human-generated analysis, and prompt engineering.
9. Conclusion
We collected 228 records related to HCER-AI from four conference proceedings: AIES, CHI, CSCW, and FAccT, as well as 67 patent applications. We then selected and thematically analyzed 164 research papers and 28 patents from these records. We found that the HCER-AI landscape emphasizes governance, fairness, and explainability. The research community needs to put effort into HCER-AI beyond fairness and create usable tools for non-expert users to audit AI and think about privacy and security from a human-centered viewpoint. While these topics are part of the broader conversation in responsible AI, there is still a need for future research to bring them into practice.
Acknowledgements.
- Abdalla and Abdalla (2021) Mohamed Abdalla and Moustafa Abdalla. 2021. The Grey Hoodie Project: Big Tobacco, Big Tech, and the Threat on Academic Integrity. In Proceedings of the 2021 AAAI/ACM Conference on AI, Ethics, and Society (AIES ’21) . ACM. https://doi.org/10.1145/3461702.3462563
- Abdul et al . (2018) Ashraf Abdul, Jo Vermeulen, Danding Wang, Brian Y. Lim, and Mohan Kankanhalli. 2018. Trends and Trajectories for Explainable, Accountable and Intelligible Systems: An HCI Research Agenda. In Proceedings of the 2018 CHI Conference on Human Factors in Computing Systems (CHI ’18) . ACM. https://doi.org/10.1145/3173574.3174156
- Abram et al . (2020) Marissa D. Abram, Karen T. Mancini, and R. David Parker. 2020. Methods to Integrate Natural Language Processing Into Qualitative Research. International Journal of Qualitative Methods (2020). https://doi.org/10.1177/1609406920984608
- Aka et al . (2021) Osman Aka, Ken Burke, Alex Bauerle, Christina Greer, and Margaret Mitchell. 2021. Measuring Model Biases in the Absence of Ground Truth. In Proceedings of the 2021 AAAI/ACM Conference on AI, Ethics, and Society (AIES ’21) . ACM. https://doi.org/10.1145/3461702.3462557
- Alfrink et al . (2023) Kars Alfrink, Ianus Keller, Neelke Doorn, and Gerd Kortuem. 2023. Contestable Camera Cars: A Speculative Design Exploration of Public AI That Is Open and Responsive to Dispute. In Proceedings of the 2023 CHI Conference on Human Factors in Computing Systems (CHI ’23) . ACM. https://doi.org/10.1145/3544548.3580984
- Angelov et al . (2021) Plamen P. Angelov, Eduardo A. Soares, Richard Jiang, Nicholas I. Arnold, and Peter M. Atkinson. 2021. Explainable artificial intelligence: an analytical review. WIREs Data Mining and Knowledge Discovery (2021). https://doi.org/10.1002/widm.1424
- Aragon et al . (2022) Cecilia Aragon, Shion Guha, Marina Kogan, Michael Muller, and Gina Neff. 2022. Human-centered data science: An introduction . MIT Press. https://mitpress.mit.edu/9780262543217/human-centered-data-science/
- Arthur et al . (2008) Melanie Arthur, Jerris R Hedges, Craig D Newgard, Brain S Diggs, and Richard J Mullins. 2008. Racial disparities in mortality among adults hospitalized after injury. Medical care (2008). https://doi.org/10.1097/MLR.0b013e31815b9d8e
- Ashktorab et al . (2023) Zahra Ashktorab, Benjamin Hoover, Mayank Agarwal, Casey Dugan, Werner Geyer, Hao Bang Yang, and Mikhail Yurochkin. 2023. Fairness Evaluation in Text Classification: Machine Learning Practitioner Perspectives of Individual and Group Fairness. In Proceedings of the 2023 CHI Conference on Human Factors in Computing Systems (CHI ’23) . ACM. https://doi.org/10.1145/3544548.3581227
- Ashurst et al . (2022) Carolyn Ashurst, Solon Barocas, Rosie Campbell, and Deborah Raji. 2022. Disentangling the Components of Ethical Research in Machine Learning. In 2022 ACM Conference on Fairness, Accountability, and Transparency (FAccT ’22) . ACM. https://doi.org/10.1145/3531146.3533781
- Associated Press (2021) Associated Press. 2021. Judge approves $650m settlement of privacy lawsuit against Facebook . Guardian News & Media Limited. Retrieved January 2023 from https://www.theguardian.com/technology/2021/feb/27/facebook-illinois-privacy-lawsuit-settlement
- Ayobi et al . (2023) Amid Ayobi, Jacob Hughes, Christopher J Duckworth, Jakub J Dylag, Sam James, Paul Marshall, Matthew Guy, Anitha Kumaran, Adriane Chapman, Michael Boniface, and Aisling Ann O’Kane. 2023. Computational Notebooks as Co-Design Tools: Engaging Young Adults Living with Diabetes, Family Carers, and Clinicians with Machine Learning Models. In Proceedings of the 2023 CHI Conference on Human Factors in Computing Systems (CHI ’23) . ACM. https://doi.org/10.1145/3544548.3581424
- Baeza-Yates (2018) Ricardo Baeza-Yates. 2018. Bias on the Web. Commun. ACM (May 2018). https://doi.org/10.1145/3209581
- Balayn et al . (2023) Agathe Balayn, Natasa Rikalo, Jie Yang, and Alessandro Bozzon. 2023. Faulty or Ready? Handling Failures in Deep-Learning Computer Vision Models until Deployment: A Study of Practices, Challenges, and Needs. In Proceedings of the 2023 CHI Conference on Human Factors in Computing Systems (CHI ’23) . ACM. https://doi.org/10.1145/3544548.3581555
- Banovic et al . (2023) Nikola Banovic, Zhuoran Yang, Aditya Ramesh, and Alice Liu. 2023. Being Trustworthy is Not Enough: How Untrustworthy Artificial Intelligence (AI) Can Deceive the End-Users and Gain Their Trust. Proc. ACM Hum.-Comput. Interact. (April 2023). https://doi.org/10.1145/3579460
- Bansal et al . (2021) Gagan Bansal, Tongshuang Wu, Joyce Zhou, Raymond Fok, Besmira Nushi, Ece Kamar, Marco Tulio Ribeiro, and Daniel Weld. 2021. Does the Whole Exceed Its Parts? The Effect of AI Explanations on Complementary Team Performance. In Proceedings of the 2021 CHI Conference on Human Factors in Computing Systems (CHI ’21) . ACM. https://doi.org/10.1145/3411764.3445717
- Barredo Arrieta et al . (2020) Alejandro Barredo Arrieta, Natalia Díaz-Rodríguez, Javier Del Ser, Adrien Bennetot, Siham Tabik, Alberto Barbado, Salvador Garcia, Sergio Gil-Lopez, Daniel Molina, Richard Benjamins, Raja Chatila, and Francisco Herrera. 2020. Explainable Artificial Intelligence (XAI): Concepts, taxonomies, opportunities and challenges toward responsible AI. Information Fusion (2020). https://doi.org/10.1016/j.inffus.2019.12.012
- Baughan et al . (2023) Amanda Baughan, Xuezhi Wang, Ariel Liu, Allison Mercurio, Jilin Chen, and Xiao Ma. 2023. A Mixed-Methods Approach to Understanding User Trust after Voice Assistant Failures. In Proceedings of the 2023 CHI Conference on Human Factors in Computing Systems (CHI ’23) . ACM. https://doi.org/10.1145/3544548.3581152
- Bawa et al . (2020) Anshul Bawa, Pranav Khadpe, Pratik Joshi, Kalika Bali, and Monojit Choudhury. 2020. Do Multilingual Users Prefer Chat-Bots That Code-Mix? Let’s Nudge and Find Out! Proc. ACM Hum.-Comput. Interact. (May 2020). https://doi.org/10.1145/3392846
- Beede et al . (2020) Emma Beede, Elizabeth Baylor, Fred Hersch, Anna Iurchenko, Lauren Wilcox, Paisan Ruamviboonsuk, and Laura M. Vardoulakis. 2020. A Human-Centered Evaluation of a Deep Learning System Deployed in Clinics for the Detection of Diabetic Retinopathy. In Proceedings of the 2020 CHI Conference on Human Factors in Computing Systems (CHI ’20) . ACM. https://doi.org/10.1145/3313831.3376718
- Bell et al . (2022) Andrew Bell, Ian Solano-Kamaiko, Oded Nov, and Julia Stoyanovich. 2022. It’s Just Not That Simple: An Empirical Study of the Accuracy-Explainability Trade-off in Machine Learning for Public Policy. In 2022 ACM Conference on Fairness, Accountability, and Transparency (FAccT ’22) . ACM. https://doi.org/10.1145/3531146.3533090
- Bender and Friedman (2018) Emily M. Bender and Batya Friedman. 2018. Data Statements for Natural Language Processing: Toward Mitigating System Bias and Enabling Better Science. Transactions of the Association for Computational Linguistics (2018). https://doi.org/10.1162/tacl_a_00041
- Benthall and Goldenfein (2021) Sebastian Benthall and Jake Goldenfein. 2021. Artificial Intelligence and the Purpose of Social Systems. In Proceedings of the 2021 AAAI/ACM Conference on AI, Ethics, and Society (AIES ’21) . ACM. https://doi.org/10.1145/3461702.3462526
- Bergram et al . (2022) Kristoffer Bergram, Marija Djokovic, Valéry Bezençon, and Adrian Holzer. 2022. The Digital Landscape of Nudging: A Systematic Literature Review of Empirical Research on Digital Nudges. In Proceedings of the 2022 CHI Conference on Human Factors in Computing Systems (CHI ’22) . ACM. https://doi.org/10.1145/3491102.3517638
- Bertrand et al . (2022) Astrid Bertrand, Rafik Belloum, James R. Eagan, and Winston Maxwell. 2022. How Cognitive Biases Affect XAI-Assisted Decision-Making: A Systematic Review. In Proceedings of the 2022 AAAI/ACM Conference on AI, Ethics, and Society (AIES ’22) . ACM. https://doi.org/10.1145/3514094.3534164
- Bessen et al . (2022) James Bessen, Stephen Michael Impink, and Robert Seamans. 2022. The Cost of Ethical AI Development for AI Startups. In Proceedings of the 2022 AAAI/ACM Conference on AI, Ethics, and Society (AIES ’22) . ACM. https://doi.org/10.1145/3514094.3534195
- Bietti (2020) Elettra Bietti. 2020. From Ethics Washing to Ethics Bashing: A View on Tech Ethics from within Moral Philosophy. In Proceedings of the 2020 Conference on Fairness, Accountability, and Transparency (FAT* ’20) . ACM. https://doi.org/10.1145/3351095.3372860
- Bingley et al . (2023) William J. Bingley, Caitlin Curtis, Steven Lockey, Alina Bialkowski, Nicole Gillespie, S. Alexander Haslam, Ryan K.L. Ko, Niklas Steffens, Janet Wiles, and Peter Worthy. 2023. Where is the human in human-centered AI? Insights from developer priorities and user experiences. Computers in Human Behavior (2023). https://doi.org/10.1016/j.chb.2022.107617
- Black et al . (2022) Emily Black, Hadi Elzayn, Alexandra Chouldechova, Jacob Goldin, and Daniel Ho. 2022. Algorithmic Fairness and Vertical Equity: Income Fairness with IRS Tax Audit Models. In 2022 ACM Conference on Fairness, Accountability, and Transparency (FAccT ’22) . ACM. https://doi.org/10.1145/3531146.3533204
- Boag et al . (2022) William Boag, Harini Suresh, Bianca Lepe, and Catherine D’Ignazio. 2022. Tech Worker Organizing for Power and Accountability. In 2022 ACM Conference on Fairness, Accountability, and Transparency (FAccT ’22) . ACM. https://doi.org/10.1145/3531146.3533111
- Boyarskaya et al . (2020) Margarita Boyarskaya, Alexandra Olteanu, and Kate Crawford. 2020. Overcoming Failures of Imagination in AI Infused System Development and Deployment. In In the Navigating the Broader Impacts of AI Research Workshop at NeurIPS 2020 . https://www.microsoft.com/en-us/research/publication/overcoming-failures-of-imagination-in-ai-infused-system-development-and-deployment/
- Braun and Clarke (2006) Virginia Braun and Victoria Clarke. 2006. Using thematic analysis in psychology. Qualitative Research in Psychology (2006). https://doi.org/10.1191/1478088706qp063oa
- Buolamwini and Gebru (2018) Joy Buolamwini and Timnit Gebru. 2018. Gender Shades: Intersectional Accuracy Disparities in Commercial Gender Classification. In Proceedings of the 1st Conference on Fairness, Accountability and Transparency (Proceedings of Machine Learning Research) , Sorelle A. Friedler and Christo Wilson (Eds.). PMLR. https://proceedings.mlr.press/v81/buolamwini18a.html
- Burgess et al . (2023) Eleanor R. Burgess, Ivana Jankovic, Melissa Austin, Nancy Cai, Adela Kapuundefinedcińska, Suzanne Currie, J. Marc Overhage, Erika S Poole, and Jofish Kaye. 2023. Healthcare AI Treatment Decision Support: Design Principles to Enhance Clinician Adoption and Trust. In Proceedings of the 2023 CHI Conference on Human Factors in Computing Systems (CHI ’23) . ACM. https://doi.org/10.1145/3544548.3581251
- Burrell et al . (2019) Jenna Burrell, Zoe Kahn, Anne Jonas, and Daniel Griffin. 2019. When Users Control the Algorithms: Values Expressed in Practices on Twitter. Proc. ACM Hum.-Comput. Interact. (Nov. 2019). https://doi.org/10.1145/3359240
- Buçinca et al . (2021) Zana Buçinca, Maja Barbara Malaya, and Krzysztof Z. Gajos. 2021. To Trust or to Think: Cognitive Forcing Functions Can Reduce Overreliance on AI in AI-Assisted Decision-Making. Proc. ACM Hum.-Comput. Interact. (April 2021). https://doi.org/10.1145/3449287
- Byun et al . (2023) Courtni Byun, Piper Vasicek, and Kevin Seppi. 2023. Dispensing with Humans in Human-Computer Interaction Research. In Extended Abstracts of the 2023 CHI Conference on Human Factors in Computing Systems (CHI EA ’23) . ACM, Article 413, 26 pages. https://doi.org/10.1145/3544549.3582749
- Cabitza et al . (2023) Federico Cabitza, Andrea Campagner, Riccardo Angius, Chiara Natali, and Carlo Reverberi. 2023. AI Shall Have No Dominion: On How to Measure Technology Dominance in AI-Supported Human Decision-Making. In Proceedings of the 2023 CHI Conference on Human Factors in Computing Systems (CHI ’23) . ACM. https://doi.org/10.1145/3544548.3581095
- Cabrera et al . (2021) Ángel Alexander Cabrera, Abraham J. Druck, Jason I. Hong, and Adam Perer. 2021. Discovering and Validating AI Errors With Crowdsourced Failure Reports. Proc. ACM Hum.-Comput. Interact. (Oct. 2021). https://doi.org/10.1145/3479569
- Calvo and Peters (2014) Rafael A Calvo and Dorian Peters. 2014. Positive Computing: Technology for Wellbeing and Human Potential . MIT press. https://mitpress.mit.edu/9780262533706/positive-computing/
- Cao et al . (2023) Hancheng Cao, Yujie Lu, Yuting Deng, Daniel Mcfarland, and Michael S. Bernstein. 2023. Breaking Out of the Ivory Tower: A Large-Scale Analysis of Patent Citations to HCI Research. In Proceedings of the 2023 CHI Conference on Human Factors in Computing Systems (CHI ’23) . ACM. https://doi.org/10.1145/3544548.3581108
- Capel and Brereton (2023) Tara Capel and Margot Brereton. 2023. What is Human-Centered about Human-Centered AI? A Map of the Research Landscape. In Proceedings of the 2023 CHI Conference on Human Factors in Computing Systems (CHI ’23) . ACM, Article 359. https://doi.org/10.1145/3544548.3580959
- Caton and Haas (2020) Simon Caton and Christian Haas. 2020. Fairness in Machine Learning: A Survey. https://doi.org/10.48550/ARXIV.2010.04053
- Chancellor (2023) Stevie Chancellor. 2023. Toward Practices for Human-Centered Machine Learning. Commun. ACM (Feb. 2023). https://doi.org/10.1145/3530987
- Chancellor et al . (2019) Stevie Chancellor, Eric P. S. Baumer, and Munmun De Choudhury. 2019. Who is the ”Human” in Human-Centered Machine Learning: The Case of Predicting Mental Health from Social Media. Proc. ACM Hum.-Comput. Interact. (Nov. 2019). https://doi.org/10.1145/3359249
- Chen and Sundar (2023) Cheng Chen and S. Shyam Sundar. 2023. Is This AI Trained on Credible Data? The Effects of Labeling Quality and Performance Bias on User Trust. In Proceedings of the 2023 CHI Conference on Human Factors in Computing Systems (CHI ’23) . ACM. https://doi.org/10.1145/3544548.3580805
- Cheng et al . (2022) Hao-Fei Cheng, Logan Stapleton, Anna Kawakami, Venkatesh Sivaraman, Yanghuidi Cheng, Diana Qing, Adam Perer, Kenneth Holstein, Zhiwei Steven Wu, and Haiyi Zhu. 2022. How Child Welfare Workers Reduce Racial Disparities in Algorithmic Decisions. In Proceedings of the 2022 CHI Conference on Human Factors in Computing Systems (CHI ’22) . ACM. https://doi.org/10.1145/3491102.3501831
- Cheng et al . (2021) Hao-Fei Cheng, Logan Stapleton, Ruiqi Wang, Paige Bullock, Alexandra Chouldechova, Zhiwei Steven Steven Wu, and Haiyi Zhu. 2021. Soliciting Stakeholders’ Fairness Notions in Child Maltreatment Predictive Systems. In Proceedings of the 2021 CHI Conference on Human Factors in Computing Systems (CHI ’21) . ACM. https://doi.org/10.1145/3411764.3445308
- Chi et al . (2021) Nicole Chi, Emma Lurie, and Deirdre K. Mulligan. 2021. Reconfiguring Diversity and Inclusion for AI Ethics. In Proceedings of the 2021 AAAI/ACM Conference on AI, Ethics, and Society (AIES ’21) . ACM. https://doi.org/10.1145/3461702.3462622
- Chmielinski et al . (2022) Kasia S. Chmielinski, Sarah Newman, Matt Taylor, Josh Joseph, Kemi Thomas, Jessica Yurkofsky, and Yue Chelsea Qiu. 2022. The Dataset Nutrition Label (2nd Gen): Leveraging Context to Mitigate Harms in Artificial Intelligence. https://doi.org/10.48550/ARXIV.2201.03954
- Choi et al . (2023) Yoonseo Choi, Eun Jeong Kang, Min Kyung Lee, and Juho Kim. 2023. Creator-Friendly Algorithms: Behaviors, Challenges, and Design Opportunities in Algorithmic Platforms. In Proceedings of the 2023 CHI Conference on Human Factors in Computing Systems (CHI ’23) . ACM. https://doi.org/10.1145/3544548.3581386
- Chu et al . (2023) Mengdi Chu, Keyu Zong, Xin Shu, Jiangtao Gong, Zhicong Lu, Kaimin Guo, Xinyi Dai, and Guyue Zhou. 2023. Work with AI and Work for AI: Autonomous Vehicle Safety Drivers’ Lived Experiences. In Proceedings of the 2023 CHI Conference on Human Factors in Computing Systems (CHI ’23) . ACM. https://doi.org/10.1145/3544548.3581564
- Constantinides and Quercia (2022) Marios Constantinides and Daniele Quercia. 2022. Good Intentions, Bad Inventions: How Employees Judge Pervasive Technologies in the Workplace. IEEE Pervasive Computing (2022). https://doi.org/10.1109/MPRV.2022.3217408
- Costanza-Chock et al . (2022) Sasha Costanza-Chock, Inioluwa Deborah Raji, and Joy Buolamwini. 2022. Who Audits the Auditors? Recommendations from a Field Scan of the Algorithmic Auditing Ecosystem. In 2022 ACM Conference on Fairness, Accountability, and Transparency (FAccT ’22) . ACM. https://doi.org/10.1145/3531146.3533213
- Cranor and Garfinkel (2005) Lorrie Faith Cranor and Simson Garfinkel. 2005. Security and Usability: Designing Secure Systems That People Can Use . O’Reilly Media, Inc. https://www.oreilly.com/library/view/security-and-usability/0596008279/
- Crisan et al . (2022) Anamaria Crisan, Margaret Drouhard, Jesse Vig, and Nazneen Rajani. 2022. Interactive Model Cards: A Human-Centered Approach to Model Documentation. In 2022 ACM Conference on Fairness, Accountability, and Transparency (FAccT ’22) . ACM. https://doi.org/10.1145/3531146.3533108
- Cruz Cortés and Ghosh (2020) Efrén Cruz Cortés and Debashis Ghosh. 2020. An Invitation to System-Wide Algorithmic Fairness. In Proceedings of the AAAI/ACM Conference on AI, Ethics, and Society (AIES ’20) . ACM. https://doi.org/10.1145/3375627.3375860
- De-Arteaga et al . (2020) Maria De-Arteaga, Riccardo Fogliato, and Alexandra Chouldechova. 2020. A Case for Humans-in-the-Loop: Decisions in the Presence of Erroneous Algorithmic Scores. In Proceedings of the 2020 CHI Conference on Human Factors in Computing Systems (CHI ’20) . ACM. https://doi.org/10.1145/3313831.3376638
- Delgado et al . (2022) Fernando Delgado, Solon Barocas, and Karen Levy. 2022. An Uncommon Task: Participatory Design in Legal AI. Proc. ACM Hum.-Comput. Interact. , Article 51 (April 2022). https://doi.org/10.1145/3512898
- Deng et al . (2023) Wesley Hanwen Deng, Boyuan Guo, Alicia Devrio, Hong Shen, Motahhare Eslami, and Kenneth Holstein. 2023. Understanding Practices, Challenges, and Opportunities for User-Engaged Algorithm Auditing in Industry Practice. In Proceedings of the 2023 CHI Conference on Human Factors in Computing Systems (CHI ’23) . ACM. https://doi.org/10.1145/3544548.3581026
- Deshpande and Sharp (2022) Advait Deshpande and Helen Sharp. 2022. Responsible AI Systems: Who Are the Stakeholders?. In Proceedings of the 2022 AAAI/ACM Conference on AI, Ethics, and Society (AIES ’22) . ACM. https://doi.org/10.1145/3514094.3534187
- Dieber and Kirrane (2020) Jürgen Dieber and Sabrina Kirrane. 2020. Why model why? Assessing the strengths and limitations of LIME. https://doi.org/10.48550/ARXIV.2012.00093
- Docherty and Biega (2022) Niall Docherty and Asia J. Biega. 2022. (Re)Politicizing Digital Well-Being: Beyond User Engagements. In Proceedings of the 2022 CHI Conference on Human Factors in Computing Systems (CHI ’22) . ACM. https://doi.org/10.1145/3491102.3501857
- Donahue et al . (2022) Kate Donahue, Alexandra Chouldechova, and Krishnaram Kenthapadi. 2022. Human-Algorithm Collaboration: Achieving Complementarity and Avoiding Unfairness. In 2022 ACM Conference on Fairness, Accountability, and Transparency (FAccT ’22) . ACM. https://doi.org/10.1145/3531146.3533221
- Ehsan et al . (2021a) Upol Ehsan, Q. Vera Liao, Michael Muller, Mark O. Riedl, and Justin D. Weisz. 2021a. Expanding Explainability: Towards Social Transparency in AI Systems. In Proceedings of the 2021 CHI Conference on Human Factors in Computing Systems (CHI ’21) . ACM. https://doi.org/10.1145/3411764.3445188
- Ehsan et al . (2023) Upol Ehsan, Koustuv Saha, Munmun De Choudhury, and Mark O. Riedl. 2023. Charting the Sociotechnical Gap in Explainable AI: A Framework to Address the Gap in XAI. Proc. ACM Hum.-Comput. Interact. (April 2023). https://doi.org/10.1145/3579467
- Ehsan et al . (2021b) Upol Ehsan, Philipp Wintersberger, Q. Vera Liao, Martina Mara, Marc Streit, Sandra Wachter, Andreas Riener, and Mark O. Riedl. 2021b. Operationalizing Human-Centered Perspectives in Explainable AI. In Extended Abstracts of the 2021 CHI Conference on Human Factors in Computing Systems (CHI EA ’21) . ACM. https://doi.org/10.1145/3411763.3441342
- Eicher et al . (2018) Bobbie Eicher, Lalith Polepeddi, and Ashok Goel. 2018. Jill Watson Doesn’t Care If You’re Pregnant: Grounding AI Ethics in Empirical Studies. In Proceedings of the 2018 AAAI/ACM Conference on AI, Ethics, and Society (AIES ’18) . ACM. https://doi.org/10.1145/3278721.3278760
- Elsayed-Ali et al . (2023) Salma Elsayed-Ali, Sara E Berger, Vagner Figueredo De Santana, and Juana Catalina Becerra Sandoval. 2023. Responsible & Inclusive Cards: An Online Card Tool to Promote Critical Reflection in Technology Industry Work Practices. In Proceedings of the 2023 CHI Conference on Human Factors in Computing Systems (CHI ’23) . ACM. https://doi.org/10.1145/3544548.3580771
- Engelmann et al . (2022) Severin Engelmann, Chiara Ullstein, Orestis Papakyriakopoulos, and Jens Grossklags. 2022. What People Think AI Should Infer From Faces. In 2022 ACM Conference on Fairness, Accountability, and Transparency (FAccT ’22) . ACM. https://doi.org/10.1145/3531146.3533080
- Erdélyi and Erdélyi (2020) Olivia J. Erdélyi and Gábor Erdélyi. 2020. The AI Liability Puzzle and a Fund-Based Work-Around. In Proceedings of the AAAI/ACM Conference on AI, Ethics, and Society (AIES ’20) . ACM. https://doi.org/10.1145/3375627.3375806
- Feinberg (2017) Melanie Feinberg. 2017. A Design Perspective on Data. In Proceedings of the 2017 CHI Conference on Human Factors in Computing Systems (CHI ’17) . ACM. https://doi.org/10.1145/3025453.3025837
- Feuston and Brubaker (2021) Jessica L. Feuston and Jed R. Brubaker. 2021. Putting Tools in Their Place: The Role of Time and Perspective in Human-AI Collaboration for Qualitative Analysis. Proc. ACM Hum.-Comput. Interact. (Oct. 2021). https://doi.org/10.1145/3479856
- Fjeld et al . (2020) Jessica Fjeld, Nele Achten, Hannah Hilligoss, Adam Nagy, and Madhulika Srikumar. 2020. Principled artificial intelligence: Mapping consensus in ethical and rights-based approaches to principles for AI. Berkman Klein Center Research Publication (2020). https://doi.org/10.2139/ssrn.3518482
- Flathmann et al . (2021) Christopher Flathmann, Beau G. Schelble, Rui Zhang, and Nathan J. McNeese. 2021. Modeling and Guiding the Creation of Ethical Human-AI Teams. In Proceedings of the 2021 AAAI/ACM Conference on AI, Ethics, and Society (AIES ’21) . ACM. https://doi.org/10.1145/3461702.3462573
- Fox et al . (2023) Sarah E. Fox, Samantha Shorey, Esther Y. Kang, Dominique Montiel Valle, and Estefania Rodriguez. 2023. Patchwork: The Hidden, Human Labor of AI Integration within Essential Work. Proc. ACM Hum.-Comput. Interact. (April 2023). https://doi.org/10.1145/3579514
- Frluckaj et al . (2022) Hana Frluckaj, Laura Dabbish, David Gray Widder, Huilian Sophie Qiu, and James D. Herbsleb. 2022. Gender and Participation in Open Source Software Development. Proc. ACM Hum.-Comput. Interact. (Nov. 2022). https://doi.org/10.1145/3555190
- Garcia and Cifor (2019) Patricia Garcia and Marika Cifor. 2019. Expanding Our Reflexive Toolbox: Collaborative Possibilities for Examining Socio-Technical Systems Using Duoethnography. Proc. ACM Hum.-Comput. Interact. , Article 190 (Nov. 2019). https://doi.org/10.1145/3359292
- Gebru et al . (2021) Timnit Gebru, Jamie Morgenstern, Briana Vecchione, Jennifer Wortman Vaughan, Hanna Wallach, Hal Daumé III, and Kate Crawford. 2021. Datasheets for Datasets. Commun. ACM (Nov. 2021). https://doi.org/10.1145/3458723
- Gee (2021) Harvey Gee. 2021. Reducing Gun Violence with ShotSpotter Gunshot Detection Technology and Community-Based Plans: What Works? https://scholarsbank.uoregon.edu/xmlui/handle/1794/27170
- Geoffrey A. Fowler (2021) Geoffrey A. Fowler. 2021. There’s no escape from Facebook, even if you don’t use it . The Washington Post. Retrieved January 2023 from https://www.washingtonpost.com/technology/2021/08/29/facebook-privacy-monopoly/
- Google (2022) Google. 2022. Responsible AI practices . Retrieved February 2023 from https://ai.google/responsibilities/responsible-ai-practices/
- Gray and Suri (2019) Mary L Gray and Siddharth Suri. 2019. Ghost Work: How to Stop Silicon Valley from Building a New Global Underclass . Eamon Dolan Books. https://ghostwork.info/
- Greiffenhagen et al . (2023) Christian Greiffenhagen, Xinzhi Xu, and Stuart Reeves. 2023. The Work to Make Facial Recognition Work. Proc. ACM Hum.-Comput. Interact. (April 2023). https://doi.org/10.1145/3579531
- Gu et al . (2021) Hongyan Gu, Jingbin Huang, Lauren Hung, and Xiang ’Anthony’ Chen. 2021. Lessons Learned from Designing an AI-Enabled Diagnosis Tool for Pathologists. Proc. ACM Hum.-Comput. Interact. (April 2021). https://doi.org/10.1145/3449084
- Ha (2022) You Jeen Ha. 2022. South Korean Public Value Coproduction Towards‘AI for Humanity’: A Synergy of Sociocultural Norms and Multistakeholder Deliberation in Bridging the Design and Implementation of National AI Ethics Guidelines. In 2022 ACM Conference on Fairness, Accountability, and Transparency (FAccT ’22) . ACM. https://doi.org/10.1145/3531146.3533091
- Hall et al . (2022) Kaely Hall, Dong Whi Yoo, Wenrui Zhang, Mehrab Bin Morshed, Vedant Das Swain, Gregory D. Abowd, Munmun De Choudhury, Alex Endert, John Stasko, and Jennifer G Kim. 2022. Supporting the Contact Tracing Process with WiFi Location Data: Opportunities and Challenges. In Proceedings of the 2022 CHI Conference on Human Factors in Computing Systems (CHI ’22) . ACM. https://doi.org/10.1145/3491102.3517703
- Havens et al . (2020) Lucy Havens, Melissa Terras, Benjamin Bach, and Beatrice Alex. 2020. Situated Data, Situated Systems: A Methodology to Engage with Power Relations in Natural Language Processing Research. In Proceedings of the Second Workshop on Gender Bias in Natural Language Processing . Association for Computational Linguistics. https://aclanthology.org/2020.gebnlp-1.10
- He et al . (2023) Gaole He, Lucie Kuiper, and Ujwal Gadiraju. 2023. Knowing About Knowing: An Illusion of Human Competence Can Hinder Appropriate Reliance on AI Systems. In Proceedings of the 2023 CHI Conference on Human Factors in Computing Systems (CHI ’23) . ACM. https://doi.org/10.1145/3544548.3581025
- Health Equity & Policy Lab (2022) Health Equity & Policy Lab. 2022. Human Flourishing . University of Pennsylvania. Retrieved January 2023 from https://www.healthequityandpolicylab.com/human-flourishing
- Heger et al . (2022) Amy K. Heger, Liz B. Marquis, Mihaela Vorvoreanu, Hanna Wallach, and Jennifer Wortman Vaughan. 2022. Understanding Machine Learning Practitioners’ Data Documentation Perceptions, Needs, Challenges, and Desiderata. Proc. ACM Hum.-Comput. Interact. (Nov. 2022). https://doi.org/10.1145/3555760
- Henriksen et al . (2021) Anne Henriksen, Simon Enni, and Anja Bechmann. 2021. Situated Accountability: Ethical Principles, Certification Standards, and Explanation Methods in Applied AI. In Proceedings of the 2021 AAAI/ACM Conference on AI, Ethics, and Society (AIES ’21) . ACM. https://doi.org/10.1145/3461702.3462564
- Hertzberg et al . (2010) Andrew Hertzberg, Jose Maria Liberti, and Daniel Paravisini. 2010. Information and incentives inside the firm: Evidence from loan officer rotation. The Journal of Finance (2010). https://doi.org/10.1111/j.1540-6261.2010.01553.x
- Holländer et al . (2021) Kai Holländer, Mark Colley, Enrico Rukzio, and Andreas Butz. 2021. A Taxonomy of Vulnerable Road Users for HCI Based On A Systematic Literature Review. In Proceedings of the 2021 CHI Conference on Human Factors in Computing Systems (CHI ’21) . ACM. https://doi.org/10.1145/3411764.3445480
- Holstein et al . (2023) Kenneth Holstein, Maria De-Arteaga, Lakshmi Tumati, and Yanghuidi Cheng. 2023. Toward Supporting Perceptual Complementarity in Human-AI Collaboration via Reflection on Unobservables. Proc. ACM Hum.-Comput. Interact. (April 2023). https://doi.org/10.1145/3579628
- Hopkins and Booth (2021) Aspen Hopkins and Serena Booth. 2021. Machine Learning Practices Outside Big Tech: How Resource Constraints Challenge Responsible Development. In Proceedings of the 2021 AAAI/ACM Conference on AI, Ethics, and Society (AIES ’21) . ACM. https://doi.org/10.1145/3461702.3462527
- Houser (2019) Kimberly A Houser. 2019. Can AI solve the diversity problem in the tech industry: Mitigating noise and bias in employment decision-making. Stan. Tech. L. Rev. (2019). https://ssrn.com/abstract=3344751
- Hsieh et al . (2023) Gary Hsieh, Brett A. Halperin, Evan Schmitz, Yen Nee Chew, and Yuan-Chi Tseng. 2023. What is in the Cards: Exploring Uses, Patterns, and Trends in Design Cards. In Proceedings of the 2023 CHI Conference on Human Factors in Computing Systems (CHI ’23) . ACM. https://doi.org/10.1145/3544548.3580712
- Huang and Liem (2022) Han-Yin Huang and Cynthia C. S. Liem. 2022. Social Inclusion in Curated Contexts: Insights from Museum Practices. In 2022 ACM Conference on Fairness, Accountability, and Transparency (FAccT ’22) . ACM. https://doi.org/10.1145/3531146.3533095
- Jakesch et al . (2022) Maurice Jakesch, Zana Buçinca, Saleema Amershi, and Alexandra Olteanu. 2022. How Different Groups Prioritize Ethical Values for Responsible AI. In 2022 ACM Conference on Fairness, Accountability, and Transparency (FAccT ’22) . ACM. https://doi.org/10.1145/3531146.3533097
- Jia et al . (2022) Chenyan Jia, Alexander Boltz, Angie Zhang, Anqing Chen, and Min Kyung Lee. 2022. Understanding Effects of Algorithmic vs. Community Label on Perceived Accuracy of Hyper-Partisan Misinformation. Proc. ACM Hum.-Comput. Interact. (Nov. 2022). https://doi.org/10.1145/3555096
- Jones et al . (2020) Bernadette Jones, Paula Toko King, Gabrielle Baker, and Tristram Ingham. 2020. COVID-19, intersectionality, and health equity for indigenous peoples with lived experience of disability. American Indian Culture and Research Journal (2020). https://doi.org/10.17953/aicrj.44.2.jones
- Josie Cox (2023) Josie Cox. 2023. AI anxiety: The workers who fear losing their jobs to artificial intelligence . Retrieved June 2023 from https://www.bbc.com/worklife/article/20230418-ai-anxiety-artificial-intelligence-replace-jobs
- Kamikubo et al . (2023) Rie Kamikubo, Kyungjun Lee, and Hernisa Kacorri. 2023. Contributing to Accessibility Datasets: Reflections on Sharing Study Data by Blind People. In Proceedings of the 2023 CHI Conference on Human Factors in Computing Systems (CHI ’23) . ACM. https://doi.org/10.1145/3544548.3581337
- Kang et al . (2022) Jimoon Kang, June Seop Yoon, and Byungjoo Lee. 2022. How AI-Based Training Affected the Performance of Professional Go Players. In Proceedings of the 2022 CHI Conference on Human Factors in Computing Systems (CHI ’22) . ACM. https://doi.org/10.1145/3491102.3517540
- Kapania et al . (2022) Shivani Kapania, Oliver Siy, Gabe Clapper, Azhagu Meena SP, and Nithya Sambasivan. 2022. ”Because AI is 100% Right and Safe”: User Attitudes and Sources of AI Authority in India. In Proceedings of the 2022 CHI Conference on Human Factors in Computing Systems (CHI ’22) . ACM. https://doi.org/10.1145/3491102.3517533
- Kapania et al . (2023) Shivani Kapania, Alex S Taylor, and Ding Wang. 2023. A Hunt for the Snark: Annotator Diversity in Data Practices. In Proceedings of the 2023 CHI Conference on Human Factors in Computing Systems (CHI ’23) . ACM. https://doi.org/10.1145/3544548.3580645
- Kasirzadeh and Klein (2021) Atoosa Kasirzadeh and Colin Klein. 2021. The Ethical Gravity Thesis: Marrian Levels and the Persistence of Bias in Automated Decision-Making Systems. In Proceedings of the 2021 AAAI/ACM Conference on AI, Ethics, and Society (AIES ’21) . ACM. https://doi.org/10.1145/3461702.3462606
- Kasirzadeh and Smart (2021) Atoosa Kasirzadeh and Andrew Smart. 2021. The Use and Misuse of Counterfactuals in Ethical Machine Learning. In Proceedings of the 2021 ACM Conference on Fairness, Accountability, and Transparency (FAccT ’21) . ACM. https://doi.org/10.1145/3442188.3445886
- Kaur et al . (2022) Harmanpreet Kaur, Eytan Adar, Eric Gilbert, and Cliff Lampe. 2022. Sensible AI: Re-Imagining Interpretability and Explainability Using Sensemaking Theory. In 2022 ACM Conference on Fairness, Accountability, and Transparency (FAccT ’22) . ACM. https://doi.org/10.1145/3531146.3533135
- Kemper and Kolain (2022) Carolin Kemper and Michael Kolain. 2022. K9 Police Robots-Strolling Drones, RoboDogs, or Lethal Weapons?. In WeRobot2022 conference . https://doi.org/10.2139/ssrn.4201692
- Kim et al . (2023) Minji Kim, Kyungjin Lee, Rajesh Balan, and Youngki Lee. 2023. Bubbleu: Exploring Augmented Reality Game Design with Uncertain AI-Based Interaction. In Proceedings of the 2023 CHI Conference on Human Factors in Computing Systems (CHI ’23) . ACM. https://doi.org/10.1145/3544548.3581270
- Kim et al . (2018) Richard Kim, Max Kleiman-Weiner, Andrés Abeliuk, Edmond Awad, Sohan Dsouza, Joshua B. Tenenbaum, and Iyad Rahwan. 2018. A Computational Model of Commonsense Moral Decision Making. In Proceedings of the 2018 AAAI/ACM Conference on AI, Ethics, and Society (AIES ’18) . ACM. https://doi.org/10.1145/3278721.3278770
- Kissinger et al . (2021) Henry Kissinger, Eric Schmidt, and Daniel P Huttenlocher. 2021. The Age of AI: And Our Human Future . John Murray London.
- Kizilaslan and Lookman (2017) Atay Kizilaslan and Aziz A Lookman. 2017. Can Economically Intuitive Factors Improve Ability of Proprietary Algorithms to Predict Defaults of Peer-to-Peer Loans? Available at SSRN 2987613 (2017). https://doi.org/10.2139/ssrn.2987613
- Klinova and Korinek (2021) Katya Klinova and Anton Korinek. 2021. AI and Shared Prosperity. In Proceedings of the 2021 AAAI/ACM Conference on AI, Ethics, and Society (AIES ’21) . ACM. https://doi.org/10.1145/3461702.3462619
- Knowles and Richards (2021) Bran Knowles and John T. Richards. 2021. The Sanction of Authority: Promoting Public Trust in AI. In Proceedings of the 2021 ACM Conference on Fairness, Accountability, and Transparency (FAccT ’21) . ACM. https://doi.org/10.1145/3442188.3445890
- Krafft et al . (2021) P. M. Krafft, Meg Young, Michael Katell, Jennifer E. Lee, Shankar Narayan, Micah Epstein, Dharma Dailey, Bernease Herman, Aaron Tam, Vivian Guetler, Corinne Bintz, Daniella Raz, Pa Ousman Jobe, Franziska Putz, Brian Robick, and Bissan Barghouti. 2021. An Action-Oriented AI Policy Toolkit for Technology Audits by Community Advocates and Activists. In Proceedings of the 2021 ACM Conference on Fairness, Accountability, and Transparency (FAccT ’21) . ACM. https://doi.org/10.1145/3442188.3445938
- Kroll (2021) Joshua A. Kroll. 2021. Outlining Traceability: A Principle for Operationalizing Accountability in Computing Systems. In Proceedings of the 2021 ACM Conference on Fairness, Accountability, and Transparency (FAccT ’21) . ACM. https://doi.org/10.1145/3442188.3445937
- Kukutai et al . (2020) Tahu Kukutai, Stephanie Russo Carroll, and Maggie Walter. 2020. Indigenous data sovereignty. (2020).
- Kumar et al . (2020) I. Elizabeth Kumar, Suresh Venkatasubramanian, Carlos Scheidegger, and Sorelle A. Friedler. 2020. Problems with Shapley-Value-Based Explanations as Feature Importance Measures. In Proceedings of the 37th International Conference on Machine Learning (ICML’20) . JMLR.org. https://doi.org/10.48550/arXiv.2002.11097
- Laker (2022) Benjamin Laker. 2022. Artificial Intelligence Can Help Leaders Drive Global Economy Forward In 2022 . Forbes. Retrieved November 2022 from https://www.forbes.com/sites/benjaminlaker/2022/01/19/artificial-intelligence-can-help-leaders-drive-global-economy-forward-in-2022/
- Lam et al . (2022) Michelle S. Lam, Mitchell L. Gordon, Danaë Metaxa, Jeffrey T. Hancock, James A. Landay, and Michael S. Bernstein. 2022. End-User Audits: A System Empowering Communities to Lead Large-Scale Investigations of Harmful Algorithmic Behavior. Proc. ACM Hum.-Comput. Interact. (Nov. 2022). https://doi.org/10.1145/3555625
- Lam et al . (2023) Michelle S. Lam, Zixian Ma, Anne Li, Izequiel Freitas, Dakuo Wang, James A. Landay, and Michael S. Bernstein. 2023. Model Sketching: Centering Concepts in Early-Stage Machine Learning Model Design. In Proceedings of the 2023 CHI Conference on Human Factors in Computing Systems (CHI ’23) . ACM. https://doi.org/10.1145/3544548.3581290
- Langer et al . (2022) Markus Langer, Tim Hunsicker, Tina Feldkamp, Cornelius J. König, and Nina Grgić-Hlača. 2022. “Look! It’s a Computer Program! It’s an Algorithm! It’s AI!”: Does Terminology Affect Human Perceptions and Evaluations of Algorithmic Decision-Making Systems?. In Proceedings of the 2022 CHI Conference on Human Factors in Computing Systems (CHI ’22) . ACM. https://doi.org/10.1145/3491102.3517527
- Lazar et al . (2017a) Jonathan Lazar, Jinjuan Heidi Feng, and Harry Hochheiser. 2017a. Chapter 11 - Analyzing qualitative data. In Research Methods in Human Computer Interaction (second edition ed.), Jonathan Lazar, Jinjuan Heidi Feng, and Harry Hochheiser (Eds.). Morgan Kaufmann. https://doi.org/10.1016/B978-0-12-805390-4.00011-X
- Lazar et al . (2017b) Jonathan Lazar, Jinjuan Heidi Feng, and Harry Hochheiser. 2017b. Chapter 8 - Interviews and focus groups. In Research Methods in Human Computer Interaction (second edition ed.), Jonathan Lazar, Jinjuan Heidi Feng, and Harry Hochheiser (Eds.). Morgan Kaufmann. https://doi.org/10.1016/B978-0-12-805390-4.00008-X
- Leavy et al . (2021) Susan Leavy, Eugenia Siapera, and Barry O’Sullivan. 2021. Ethical Data Curation for AI: An Approach Based on Feminist Epistemology and Critical Theories of Race. In Proceedings of the 2021 AAAI/ACM Conference on AI, Ethics, and Society (AIES ’21) . ACM. https://doi.org/10.1145/3461702.3462598
- Leckning et al . (2021) Bernard Leckning, Vincent YF He, John R Condon, Tanja Hirvonen, Helen Milroy, and Steven Guthridge. 2021. Patterns of child protection service involvement by Aboriginal children associated with a higher risk of self-harm in adolescence: A retrospective population cohort study using linked administrative data. Child Abuse & Neglect (2021). https://doi.org/10.1016/j.chiabu.2021.104931
- Lee (2022) Kai-Fu Lee. 2022. AI and the human future: Net positive . Economics. Retrieved November 2022 from https://impact.economist.com/projects/innovation-matters/blogs/ai-and-the-human-future-net-positive/
- Lee et al . (2019) Min Kyung Lee, Daniel Kusbit, Anson Kahng, Ji Tae Kim, Xinran Yuan, Allissa Chan, Daniel See, Ritesh Noothigattu, Siheon Lee, Alexandros Psomas, and Ariel D. Procaccia. 2019. WeBuildAI: Participatory Framework for Algorithmic Governance. Proc. ACM Hum.-Comput. Interact. (Nov. 2019). https://doi.org/10.1145/3359283
- Lee et al . (2021) Min Kyung Lee, Ishan Nigam, Angie Zhang, Joel Afriyie, Zhizhen Qin, and Sicun Gao. 2021. Participatory Algorithmic Management: Elicitation Methods for Worker Well-Being Models. In Proceedings of the 2021 AAAI/ACM Conference on AI, Ethics, and Society (AIES ’21) . ACM. https://doi.org/10.1145/3461702.3462628
- Lee and Rich (2021) Min Kyung Lee and Katherine Rich. 2021. Who Is Included in Human Perceptions of AI?: Trust and Perceived Fairness around Healthcare AI and Cultural Mistrust. In Proceedings of the 2021 CHI Conference on Human Factors in Computing Systems (CHI ’21) . ACM, Article 138. https://doi.org/10.1145/3411764.3445570
- Lee et al . (2023b) Patrick Yung Kang Lee, Ning F. Ma, Ig-Jae Kim, and Dongwook Yoon. 2023b. Speculating on Risks of AI Clones to Selfhood and Relationships: Doppelganger-Phobia, Identity Fragmentation, and Living Memories. Proc. ACM Hum.-Comput. Interact. (April 2023). https://doi.org/10.1145/3579524
- Lee et al . (2023a) Sunok Lee, Dasom Choi, Minha Lee, Jonghak Choi, and Sangsu Lee. 2023a. Fostering Youth’s Critical Thinking Competency About AI through Exhibition. In Proceedings of the 2023 CHI Conference on Human Factors in Computing Systems (CHI ’23) . ACM. https://doi.org/10.1145/3544548.3581159
- Leonardo Nicoletti and Dina Bass (2023) Leonardo Nicoletti and Dina Bass. 2023. HUMANS ARE BIASED. GENERATIVE AI IS EVEN WORSE . Retrieved June 2023 from https://www.bloomberg.com/graphics/2023-generative-ai-bias/
- Lewicki et al . (2023) Kornel Lewicki, Michelle Seng Ah Lee, Jennifer Cobbe, and Jatinder Singh. 2023. Out of Context: Investigating the Bias and Fairness Concerns of “Artificial Intelligence as a Service”. In Proceedings of the 2023 CHI Conference on Human Factors in Computing Systems (CHI ’23) . ACM. https://doi.org/10.1145/3544548.3581463
- Li et al . (2023) Rena Li, Sara Kingsley, Chelsea Fan, Proteeti Sinha, Nora Wai, Jaimie Lee, Hong Shen, Motahhare Eslami, and Jason Hong. 2023. Participation and Division of Labor in User-Driven Algorithm Audits: How Do Everyday Users Work Together to Surface Algorithmic Harms?. In Proceedings of the 2023 CHI Conference on Human Factors in Computing Systems (CHI ’23) . ACM. https://doi.org/10.1145/3544548.3582074
- Li et al . (2022) Yunqi Li, Hanxiong Chen, Shuyuan Xu, Yingqiang Ge, Juntao Tan, Shuchang Liu, and Yongfeng Zhang. 2022. Fairness in Recommendation: A Survey. https://doi.org/10.48550/ARXIV.2205.13619
- Liao and Sundar (2022) Q.Vera Liao and S. Shyam Sundar. 2022. Designing for Responsible Trust in AI Systems: A Communication Perspective. In 2022 ACM Conference on Fairness, Accountability, and Transparency (FAccT ’22) . ACM. https://doi.org/10.1145/3531146.3533182
- Liao et al . (2020) Q. Vera Liao, Daniel Gruen, and Sarah Miller. 2020. Questioning the AI: Informing Design Practices for Explainable AI User Experiences. In Proceedings of the 2020 CHI Conference on Human Factors in Computing Systems (CHI ’20) . ACM. https://doi.org/10.1145/3313831.3376590
- Liao et al . (2023) Q. Vera Liao, Hariharan Subramonyam, Jennifer Wang, and Jennifer Wortman Vaughan. 2023. Designerly Understanding: Information Needs for Model Transparency to Support Design Ideation for AI-Powered User Experience. In Proceedings of the 2023 CHI Conference on Human Factors in Computing Systems (CHI ’23) . ACM. https://doi.org/10.1145/3544548.3580652
- Liao and Varshney (2021) Q. Vera Liao and Kush R. Varshney. 2021. Human-Centered Explainable AI (XAI): From Algorithms to User Experiences. (2021). https://doi.org/10.48550/ARXIV.2110.10790
- Lima et al . (2021) Gabriel Lima, Nina Grgić-Hlača, and Meeyoung Cha. 2021. Human Perceptions on Moral Responsibility of AI: A Case Study in AI-Assisted Bail Decision-Making. In Proceedings of the 2021 CHI Conference on Human Factors in Computing Systems (CHI ’21) . ACM. https://doi.org/10.1145/3411764.3445260
- Lima et al . (2023) Gabriel Lima, Nina Grgić-Hlača, and Meeyoung Cha. 2023. Blaming Humans and Machines: What Shapes People’s Reactions to Algorithmic Harm. In Proceedings of the 2023 CHI Conference on Human Factors in Computing Systems (CHI ’23) . ACM. https://doi.org/10.1145/3544548.3580953
- Lima et al . (2022) Gabriel Lima, Nina Grgić-Hlača, Jin Keun Jeong, and Meeyoung Cha. 2022. The Conflict Between Explainable and Accountable Decision-Making Algorithms. In 2022 ACM Conference on Fairness, Accountability, and Transparency (FAccT ’22) . ACM. https://doi.org/10.1145/3531146.3534628
- Lin and Van Brummelen (2021) Phoebe Lin and Jessica Van Brummelen. 2021. Engaging Teachers to Co-Design Integrated AI Curriculum for K-12 Classrooms. In Proceedings of the 2021 CHI Conference on Human Factors in Computing Systems (CHI ’21) . ACM. https://doi.org/10.1145/3411764.3445377
- Linxen et al . (2021) Sebastian Linxen, Christian Sturm, Florian Brühlmann, Vincent Cassau, Klaus Opwis, and Katharina Reinecke. 2021. How WEIRD is CHI?. In Proceedings of the 2021 CHI Conference on Human Factors in Computing Systems (CHI ’21) . ACM. https://doi.org/10.1145/3411764.3445488
- Long et al . (2021) Duri Long, Takeria Blunt, and Brian Magerko. 2021. Co-Designing AI Literacy Exhibits for Informal Learning Spaces. Proc. ACM Hum.-Comput. Interact. (Oct. 2021). https://doi.org/10.1145/3476034
- Long et al . (2022) Duri Long, Anthony Teachey, and Brian Magerko. 2022. Family Learning Talk in AI Literacy Learning Activities. In Proceedings of the 2022 CHI Conference on Human Factors in Computing Systems (CHI ’22) . ACM. https://doi.org/10.1145/3491102.3502091
- Lundberg and Lee (2017) Scott M. Lundberg and Su-In Lee. 2017. A Unified Approach to Interpreting Model Predictions. In Proceedings of the 31st International Conference on Neural Information Processing Systems (NIPS’17) . Curran Associates Inc. https://dl.acm.org/doi/pdf/10.5555/3295222.3295230
- Lyn (2020) Alexandra Lyn. 2020. Risky Business: Artificial Intelligence and Risk Assessments in Sentencing and Bail Procedures in the United States. Available at SSRN 3831441 (2020). https://doi.org/10.2139/ssrn.3831441
- Lyons et al . (2021) Henrietta Lyons, Eduardo Velloso, and Tim Miller. 2021. Conceptualising Contestability: Perspectives on Contesting Algorithmic Decisions. Proc. ACM Hum.-Comput. Interact. (April 2021). https://doi.org/10.1145/3449180
- Maas (2018) Matthijs M. Maas. 2018. Regulating for ’Normal AI Accidents’: Operational Lessons for the Responsible Governance of Artificial Intelligence Deployment. In Proceedings of the 2018 AAAI/ACM Conference on AI, Ethics, and Society (AIES ’18) . ACM. https://doi.org/10.1145/3278721.3278766
- Marley (2019) Tennille L Marley. 2019. Indigenous data sovereignty: University institutional review board policies and guidelines and research with American Indian and Alaska Native communities. American Behavioral Scientist (2019).
- McDonald and Pan (2020) Nora McDonald and Shimei Pan. 2020. Intersectional AI: A Study of How Information Science Students Think about Ethics and Their Impact. Proc. ACM Hum.-Comput. Interact. (Oct. 2020). https://doi.org/10.1145/3415218
- McNaney et al . (2018) Roisin McNaney, John Vines, Andy Dow, Harry Robinson, Heather Robinson, Kate McDonald, Leslie Brown, Peter Santer, Don Murray, Janice Murray, David Green, and Peter Wright. 2018. Enabling the Participation of People with Parkinson’s and Their Caregivers in Co-Inquiry around Collectivist Health Technologies. In Proceedings of the 2018 CHI Conference on Human Factors in Computing Systems (CHI ’18) . ACM. https://doi.org/10.1145/3173574.3174065
- Mentis et al . (2016) Helena M. Mentis, Ahmed Rahim, and Pierre Theodore. 2016. Crafting the Image in Surgical Telemedicine. In Proceedings of the 19th ACM Conference on Computer-Supported Cooperative Work & Social Computing (CSCW ’16) . ACM. https://doi.org/10.1145/2818048.2819978
- Mhasawade et al . (2021) Vishwali Mhasawade, Yuan Zhao, and Rumi Chunara. 2021. Machine learning and algorithmic fairness in public and population health. Nature Machine Intelligence (2021). https://doi.org/10.1038/s42256-021-00373-4
- Miceli et al . (2022) Milagros Miceli, Tianling Yang, Adriana Alvarado Garcia, Julian Posada, Sonja Mei Wang, Marc Pohl, and Alex Hanna. 2022. Documenting Data Production Processes: A Participatory Approach for Data Work. Proc. ACM Hum.-Comput. Interact. (Nov. 2022). https://doi.org/10.1145/3555623
- Microsoft (2022) Microsoft. 2022. Responsible AI . Retrieved February 2023 from https://www.microsoft.com/en-us/ai/responsible-ai
- Mlynar et al . (2022) Jakub Mlynar, Farzaneh Bahrami, André Ourednik, Nico Mutzner, Himanshu Verma, and Hamed Alavi. 2022. AI beyond Deus Ex Machina – Reimagining Intelligence in Future Cities with Urban Experts. In Proceedings of the 2022 CHI Conference on Human Factors in Computing Systems (CHI ’22) . ACM. https://doi.org/10.1145/3491102.3517502
- Moitra et al . (2022) Aparna Moitra, Dennis Wagenaar, Manveer Kalirai, Syed Ishtiaque Ahmed, and Robert Soden. 2022. AI and Disaster Risk: A Practitioner Perspective. Proc. ACM Hum.-Comput. Interact. (Nov. 2022). https://doi.org/10.1145/3555163
- Moore et al . (2023) Steven Moore, Q. Vera Liao, and Hariharan Subramonyam. 2023. FAIlureNotes: Supporting Designers in Understanding the Limits of AI Models for Computer Vision Tasks. In Proceedings of the 2023 CHI Conference on Human Factors in Computing Systems (CHI ’23) . ACM. https://doi.org/10.1145/3544548.3581242
- Moreschi et al . (2020) Bruno Moreschi, Gabriel Pereira, and Fabio G Cozman. 2020. The Brazilian Workers in Amazon Mechanical Turk: dreams and realities of ghost workers. Contracampo (2020). https://doi.org/10.22409/contracampo.v39i1.38252
- Morrison et al . (2021) Cecily Morrison, Edward Cutrell, Martin Grayson, Anja Thieme, Alex Taylor, Geert Roumen, Camilla Longden, Sebastian Tschiatschek, Rita Faia Marques, and Abigail Sellen. 2021. Social Sensemaking with AI: Designing an Open-Ended AI Experience with a Blind Child. In Proceedings of the 2021 CHI Conference on Human Factors in Computing Systems (CHI ’21) . ACM. https://doi.org/10.1145/3411764.3445290
- Muller et al . (2022) Michael Muller, Plamen Agelov, Shion Guha, Marina Kogan, Gina Neff, Nuria Oliver, Manuel Gomez Rodriguez, and Adrian Weller. 2022. NeurIPS 2021 Workshop Proposal: Human Centered AI . Retrieved March 2023 from https://sites.google.com/view/hcai-human-centered-ai-neurips/home
- Muller and Strohmayer (2022) Michael Muller and Angelika Strohmayer. 2022. Forgetting Practices in the Data Sciences. In Proceedings of the 2022 CHI Conference on Human Factors in Computing Systems (CHI ’22) . ACM. https://doi.org/10.1145/3491102.3517644
- Muller and Weisz (2022) Michael Muller and Justin Weisz. 2022. Extending a Human-AI Collaboration Framework with Dynamism and Sociality. In 2022 Symposium on Human-Computer Interaction for Work (CHIWORK 2022) . ACM, Article 10. https://doi.org/10.1145/3533406.3533407
- Muller et al . (2021) Michael Muller, Christine T. Wolf, Josh Andres, Michael Desmond, Narendra Nath Joshi, Zahra Ashktorab, Aabhas Sharma, Kristina Brimijoin, Qian Pan, Evelyn Duesterwald, and Casey Dugan. 2021. Designing Ground Truth and the Social Life of Labels. In Proceedings of the 2021 CHI Conference on Human Factors in Computing Systems (CHI ’21) . ACM, Article 94. https://doi.org/10.1145/3411764.3445402
- Nakao et al . (2022) Yuri Nakao, Simone Stumpf, Subeida Ahmed, Aisha Naseer, and Lorenzo Strappelli. 2022. Toward Involving End-Users in Interactive Human-in-the-Loop AI Fairness. ACM Trans. Interact. Intell. Syst. , Article 18 (July 2022). https://doi.org/10.1145/3514258
- Nashed et al . (2021) Samer Nashed, Justin Svegliato, and Shlomo Zilberstein. 2021. Ethically Compliant Planning within Moral Communities. In Proceedings of the 2021 AAAI/ACM Conference on AI, Ethics, and Society (AIES ’21) . ACM. https://doi.org/10.1145/3461702.3462522
- National Institute of Standards and Technology (2023) National Institute of Standards and Technology. 2023. AI Risk Management Framework . Retrieved February 2023 from https://www.nist.gov/itl/ai-risk-management-framework
- Neuhauser and Kreps (2011) Linda Neuhauser and Gary L Kreps. 2011. Participatory Design and Artificial Intelligence: Strategies to Improve Health Communication for Diverse Audiences. In AAAI Spring Symposium: AI and Health Communication . https://researchers.mq.edu.au/en/publications/participatory-design-and-artificial-intelligence-strategies-to-im
- Nielsen (2021) Aileen Nielsen. 2021. Measuring Lay Reactions to Personal Data Markets. In Proceedings of the 2021 AAAI/ACM Conference on AI, Ethics, and Society (AIES ’21) . ACM. https://doi.org/10.1145/3461702.3462582
- Niforatos et al . (2020) Evangelos Niforatos, Adam Palma, Roman Gluszny, Athanasios Vourvopoulos, and Fotis Liarokapis. 2020. Would You Do It?: Enacting Moral Dilemmas in Virtual Reality for Understanding Ethical Decision-Making. In Proceedings of the 2020 CHI Conference on Human Factors in Computing Systems (CHI ’20) . ACM. https://doi.org/10.1145/3313831.3376788
- Nokia Bell Labs (2022) Nokia Bell Labs. 2022. Responsible AI . Retrieved January 2023 from https://www.bell-labs.com/research-innovation/responsible-ai/
- Olteanu et al . (2019) Alexandra Olteanu, Carlos Castillo, Fernando Diaz, and Emre Kıcıman. 2019. Social Data: Biases, Methodological Pitfalls, and Ethical Boundaries. Frontiers in Big Data (2019). https://doi.org/10.3389/fdata.2019.00013
- Orphanou et al . (2022) Kalia Orphanou, Jahna Otterbacher, Styliani Kleanthous, Khuyagbaatar Batsuren, Fausto Giunchiglia, Veronika Bogina, Avital Shulner Tal, Alan Hartman, and Tsvi Kuflik. 2022. Mitigating Bias in Algorithmic Systems–A Fish-Eye View. ACM Comput. Surv. (Dec. 2022). https://doi.org/10.1145/3527152
- Page et al . (2021) Matthew J Page, Joanne E McKenzie, Patrick M Bossuyt, Isabelle Boutron, Tammy C Hoffmann, Cynthia D Mulrow, Larissa Shamseer, Jennifer M Tetzlaff, Elie A Akl, Sue E Brennan, Roger Chou, Julie Glanville, Jeremy M Grimshaw, Asbjørn Hróbjartsson, Manoj M Lalu, Tianjing Li, Elizabeth W Loder, Evan Mayo-Wilson, Steve McDonald, Luke A McGuinness, Lesley A Stewart, James Thomas, Andrea C Tricco, Vivian A Welch, Penny Whiting, and David Moher. 2021. The PRISMA 2020 statement: an updated guideline for reporting systematic reviews. BMJ (2021). https://doi.org/10.1136/bmj.n71
- Park et al . (2021) Hyanghee Park, Daehwan Ahn, Kartik Hosanagar, and Joonhwan Lee. 2021. Human-AI Interaction in Human Resource Management: Understanding Why Employees Resist Algorithmic Evaluation at Workplaces and How to Mitigate Burdens. In Proceedings of the 2021 CHI Conference on Human Factors in Computing Systems (CHI ’21) . ACM. https://doi.org/10.1145/3411764.3445304
- Park et al . (2022) Hyanghee Park, Daehwan Ahn, Kartik Hosanagar, and Joonhwan Lee. 2022. Designing Fair AI in Human Resource Management: Understanding Tensions Surrounding Algorithmic Evaluation and Envisioning Stakeholder-Centered Solutions. In Proceedings of the 2022 CHI Conference on Human Factors in Computing Systems (CHI ’22) . ACM. https://doi.org/10.1145/3491102.3517672
- Passi and Jackson (2017) Samir Passi and Steven Jackson. 2017. Data Vision: Learning to See Through Algorithmic Abstraction. In Proceedings of the 2017 ACM Conference on Computer Supported Cooperative Work and Social Computing (CSCW ’17) . ACM. https://doi.org/10.1145/2998181.2998331
- Pessach and Shmueli (2022) Dana Pessach and Erez Shmueli. 2022. A Review on Fairness in Machine Learning. ACM Comput. Surv. (Feb. 2022). https://doi.org/10.1145/3494672
- Peters et al . (2013) Anicia Peters, Heike Winschiers-Theophilus, and Brian Mennecke. 2013. Bridging the Digital Divide through Facebook Friendships: A Cross-Cultural Study. In Proceedings of the 2013 Conference on Computer Supported Cooperative Work Companion (CSCW ’13) . ACM. https://doi.org/10.1145/2441955.2442014
- Pine and Liboiron (2015) Kathleen H. Pine and Max Liboiron. 2015. The Politics of Measurement and Action. In Proceedings of the 33rd Annual ACM Conference on Human Factors in Computing Systems (CHI ’15) . ACM. https://doi.org/10.1145/2702123.2702298
- Pushkarna et al . (2022) Mahima Pushkarna, Andrew Zaldivar, and Oddur Kjartansson. 2022. Data Cards: Purposeful and Transparent Dataset Documentation for Responsible AI. In 2022 ACM Conference on Fairness, Accountability, and Transparency (FAccT ’22) . ACM. https://doi.org/10.1145/3531146.3533231
- PwC (2022) PwC. 2022. PwC’s Responsible AI . Retrieved February 2023 from https://www.pwc.com/gx/en/issues/data-and-analytics/artificial-intelligence/what-is-responsible-ai/pwc-responsible-ai.pdf
- Rakova et al . (2021) Bogdana Rakova, Jingying Yang, Henriette Cramer, and Rumman Chowdhury. 2021. Where Responsible AI Meets Reality: Practitioner Perspectives on Enablers for Shifting Organizational Practices. Proc. ACM Hum.-Comput. Interact. (April 2021). https://doi.org/10.1145/3449081
- Ramesh et al . (2022) Divya Ramesh, Vaishnav Kameswaran, Ding Wang, and Nithya Sambasivan. 2022. How Platform-User Power Relations Shape Algorithmic Accountability: A Case Study of Instant Loan Platforms and Financially Stressed Users in India. In 2022 ACM Conference on Fairness, Accountability, and Transparency (FAccT ’22) . ACM. https://doi.org/10.1145/3531146.3533237
- Raz et al . (2021) Daniella Raz, Corinne Bintz, Vivian Guetler, Aaron Tam, Michael Katell, Dharma Dailey, Bernease Herman, P. M. Krafft, and Meg Young. 2021. Face Mis-ID: An Interactive Pedagogical Tool Demonstrating Disparate Accuracy Rates in Facial Recognition. In Proceedings of the 2021 AAAI/ACM Conference on AI, Ethics, and Society (AIES ’21) . ACM. https://doi.org/10.1145/3461702.3462627
- Reinecke et al . (2013) Katharina Reinecke, Minh Khoa Nguyen, Abraham Bernstein, Michael Näf, and Krzysztof Z. Gajos. 2013. Doodle around the World: Online Scheduling Behavior Reflects Cultural Differences in Time Perception and Group Decision-Making. In Proceedings of the 2013 Conference on Computer Supported Cooperative Work (CSCW ’13) . ACM. https://doi.org/10.1145/2441776.2441784
- Reitmaier et al . (2022) Thomas Reitmaier, Electra Wallington, Dani Kalarikalayil Raju, Ondrej Klejch, Jennifer Pearson, Matt Jones, Peter Bell, and Simon Robinson. 2022. Opportunities and Challenges of Automatic Speech Recognition Systems for Low-Resource Language Speakers. In Proceedings of the 2022 CHI Conference on Human Factors in Computing Systems (CHI ’22) . ACM. https://doi.org/10.1145/3491102.3517639
- Richards et al . (2019) John Richards, David Piorkowski, Michael Hind, Stephanie Houde, and Aleksandra Mojsilović. 2019. FactSheets: Increasing trust in AI services through supplier’s declarations of conformity. IBM Journal of Research and Development (2019). https://doi.org/10.1147/JRD.2019.2942288
- Richardson et al . (2021) Brianna Richardson, Jean Garcia-Gathright, Samuel F. Way, Jennifer Thom, and Henriette Cramer. 2021. Towards Fairness in Practice: A Practitioner-Oriented Rubric for Evaluating Fair ML Toolkits. In Proceedings of the 2021 CHI Conference on Human Factors in Computing Systems (CHI ’21) . ACM. https://doi.org/10.1145/3411764.3445604
- Rismani et al . (2023) Shalaleh Rismani, Renee Shelby, Andrew Smart, Edgar Jatho, Joshua Kroll, AJung Moon, and Negar Rostamzadeh. 2023. From Plane Crashes to Algorithmic Harm: Applicability of Safety Engineering Frameworks for Responsible ML. In Proceedings of the 2023 CHI Conference on Human Factors in Computing Systems (CHI ’23) . ACM. https://doi.org/10.1145/3544548.3581407
- Roemmich and Andalibi (2021) Kat Roemmich and Nazanin Andalibi. 2021. Data Subjects’ Conceptualizations of and Attitudes Toward Automatic Emotion Recognition-Enabled Wellbeing Interventions on Social Media. Proc. ACM Hum.-Comput. Interact. (Oct. 2021). https://doi.org/10.1145/3476049
- Sambasivan et al . (2021a) Nithya Sambasivan, Erin Arnesen, Ben Hutchinson, Tulsee Doshi, and Vinodkumar Prabhakaran. 2021a. Re-Imagining Algorithmic Fairness in India and Beyond. In Proceedings of the 2021 ACM Conference on Fairness, Accountability, and Transparency (FAccT ’21) . ACM. https://doi.org/10.1145/3442188.3445896
- Sambasivan et al . (2021b) Nithya Sambasivan, Shivani Kapania, Hannah Highfill, Diana Akrong, Praveen Paritosh, and Lora M Aroyo. 2021b. “Everyone Wants to Do the Model Work, Not the Data Work”: Data Cascades in High-Stakes AI. In Proceedings of the 2021 CHI Conference on Human Factors in Computing Systems (CHI ’21) . ACM. https://doi.org/10.1145/3411764.3445518
- Saxena et al . (2020) Devansh Saxena, Karla Badillo-Urquiola, Pamela J. Wisniewski, and Shion Guha. 2020. A Human-Centered Review of Algorithms Used within the U.S. Child Welfare System. In Proceedings of the 2020 CHI Conference on Human Factors in Computing Systems (CHI ’20) . ACM. https://doi.org/10.1145/3313831.3376229
- Schiff et al . (2020) Daniel Schiff, Justin Biddle, Jason Borenstein, and Kelly Laas. 2020. What’s Next for AI Ethics, Policy, and Governance? A Global Overview. In Proceedings of the AAAI/ACM Conference on AI, Ethics, and Society (AIES ’20) . ACM. https://doi.org/10.1145/3375627.3375804
- Septiandri et al . (2023) Ali Akbar Septiandri, Marios Constantinides, Mohammad Tahaei, and Daniele Quercia. 2023. WEIRD FAccTs: How Western, Educated, Industrialized, Rich, and Democratic is FAccT?. In Proceedings of the 2023 ACM Conference on Fairness, Accountability, and Transparency (FAccT ’23) . ACM. https://doi.org/10.1145/3593013.3593985
- Shahid and Vashistha (2023) Farhana Shahid and Aditya Vashistha. 2023. Decolonizing Content Moderation: Does Uniform Global Community Standard Resemble Utopian Equality or Western Power Hegemony?. In Proceedings of the 2023 CHI Conference on Human Factors in Computing Systems (CHI ’23) . ACM. https://doi.org/10.1145/3544548.3581538
- Sharma et al . (2021) Shubham Sharma, Alan H. Gee, David Paydarfar, and Joydeep Ghosh. 2021. FaiR-N: Fair and Robust Neural Networks for Structured Data. In Proceedings of the 2021 AAAI/ACM Conference on AI, Ethics, and Society (AIES ’21) . ACM. https://doi.org/10.1145/3461702.3462559
- Shaw et al . (2018) Nolan P. Shaw, Andreas Stöckel, Ryan W. Orr, Thomas F. Lidbetter, and Robin Cohen. 2018. Towards Provably Moral AI Agents in Bottom-up Learning Frameworks. In Proceedings of the 2018 AAAI/ACM Conference on AI, Ethics, and Society (AIES ’18) . ACM. https://doi.org/10.1145/3278721.3278728
- Shen et al . (2021) Hong Shen, Alicia DeVos, Motahhare Eslami, and Kenneth Holstein. 2021. Everyday Algorithm Auditing: Understanding the Power of Everyday Users in Surfacing Harmful Algorithmic Behaviors. Proc. ACM Hum.-Comput. Interact. (Oct. 2021). https://doi.org/10.1145/3479577
- Shen et al . (2020) Hong Shen, Haojian Jin, Ángel Alexander Cabrera, Adam Perer, Haiyi Zhu, and Jason I. Hong. 2020. Designing Alternative Representations of Confusion Matrices to Support Non-Expert Public Understanding of Algorithm Performance. Proc. ACM Hum.-Comput. Interact. (Oct. 2020). https://doi.org/10.1145/3415224
- Shneiderman (2020) Ben Shneiderman. 2020. Bridging the Gap Between Ethics and Practice: Guidelines for Reliable, Safe, and Trustworthy Human-Centered AI Systems. ACM Trans. Interact. Intell. Syst. (Oct. 2020). https://doi.org/10.1145/3419764
- Shneiderman (2022) Ben Shneiderman. 2022. Human-centered AI . Oxford University Press.
- Siala and Wang (2022) Haytham Siala and Yichuan Wang. 2022. SHIFTing artificial intelligence to be responsible in healthcare: A systematic review. Social Science & Medicine (2022). https://doi.org/10.1016/j.socscimed.2022.114782
- Siapka (2022) Anastasia Siapka. 2022. Towards a Feminist Metaethics of AI. In Proceedings of the 2022 AAAI/ACM Conference on AI, Ethics, and Society (AIES ’22) . ACM. https://doi.org/10.1145/3514094.3534197
- Silva and Kenney (2018) Selena Silva and Martin Kenney. 2018. Algorithms, platforms, and ethnic bias: An integrative essay. Phylon (1960-) (2018). https://www.jstor.org/stable/26545017
- Simons et al . (2021) Joshua Simons, Sophia Adams Bhatti, and Adrian Weller. 2021. Machine Learning and the Meaning of Equal Treatment. In Proceedings of the 2021 AAAI/ACM Conference on AI, Ethics, and Society (AIES ’21) . ACM. https://doi.org/10.1145/3461702.3462556
- Sloane and Zakrzewski (2022) Mona Sloane and Janina Zakrzewski. 2022. German AI Start-Ups and “AI Ethics”: Using A Social Practice Lens for Assessing and Implementing Socio-Technical Innovation. In 2022 ACM Conference on Fairness, Accountability, and Transparency (FAccT ’22) . ACM. https://doi.org/10.1145/3531146.3533156
- Smith et al . (2022) Jessie J. Smith, Saleema Amershi, Solon Barocas, Hanna Wallach, and Jennifer Wortman Vaughan. 2022. REAL ML: Recognizing, Exploring, and Articulating Limitations of Machine Learning Research. In 2022 ACM Conference on Fairness, Accountability, and Transparency (FAccT ’22) . ACM. https://doi.org/10.1145/3531146.3533122
- Stapleton et al . (2022) Logan Stapleton, Min Hun Lee, Diana Qing, Marya Wright, Alexandra Chouldechova, Ken Holstein, Zhiwei Steven Wu, and Haiyi Zhu. 2022. Imagining New Futures beyond Predictive Systems in Child Welfare: A Qualitative Study with Impacted Stakeholders. In 2022 ACM Conference on Fairness, Accountability, and Transparency (FAccT ’22) . ACM. https://doi.org/10.1145/3531146.3533177
- Stark and Hoey (2021) Luke Stark and Jesse Hoey. 2021. The Ethics of Emotion in Artificial Intelligence Systems. In Proceedings of the 2021 ACM Conference on Fairness, Accountability, and Transparency (FAccT ’21) . ACM. https://doi.org/10.1145/3442188.3445939
- Steiger et al . (2021) Miriah Steiger, Timir J Bharucha, Sukrit Venkatagiri, Martin J. Riedl, and Matthew Lease. 2021. The Psychological Well-Being of Content Moderators: The Emotional Labor of Commercial Moderation and Avenues for Improving Support. In Proceedings of the 2021 CHI Conference on Human Factors in Computing Systems (CHI ’21) . ACM. https://doi.org/10.1145/3411764.3445092
- Subramonyam et al . (2022) Hariharan Subramonyam, Jane Im, Colleen Seifert, and Eytan Adar. 2022. Solving Separation-of-Concerns Problems in Collaborative Design of Human-AI Systems through Leaky Abstractions. In CHI Conference on Human Factors in Computing Systems (CHI ’22) . ACM. https://doi.org/10.1145/3491102.3517537
- Sun et al . (2019) Tony Sun, Andrew Gaut, Shirlyn Tang, Yuxin Huang, Mai ElSherief, Jieyu Zhao, Diba Mirza, Elizabeth Belding, Kai-Wei Chang, and William Yang Wang. 2019. Mitigating Gender Bias in Natural Language Processing: Literature Review. In Proceedings of the 57th Annual Meeting of the Association for Computational Linguistics . Association for Computational Linguistics. https://doi.org/10.18653/v1/P19-1159
- Tahaei et al . (2023) Mohammad Tahaei, Marios Constantinides, Daniele Quercia, Sean Kennedy, Michael Muller, Simone Stumpf, Q. Vera Liao, Ricardo Baeza-Yates, Lora Aroyo, Jess Holbrook, Ewa Luger, Michael Madaio, Ilana Golbin Blumenfeld, Maria De-Arteaga, Jessica Vitak, and Alexandra Olteanu. 2023. Human-Centered Responsible Artificial Intelligence: Current & Future Trends. In Extended Abstracts of the 2023 CHI Conference on Human Factors in Computing Systems (CHI EA ’23) . ACM. https://doi.org/10.1145/3544549.3583178
- Tahaei and Vaniea (2019) Mohammad Tahaei and Kami Vaniea. 2019. A Survey on Developer-Centred Security. In 2019 IEEE European Symposium on Security and Privacy Workshops (EuroS&PW) . IEEE. https://doi.org/10.1109/EuroSPW.2019.00021
- Tahaei et al . (2020) Mohammad Tahaei, Kami Vaniea, and Naomi Saphra. 2020. Understanding Privacy-Related Questions on Stack Overflow. In Proceedings of the 2020 CHI Conference on Human Factors in Computing Systems (CHI ’20) . ACM, 14 pages. https://doi.org/10.1145/3313831.3376768
- Terzis (2020) Petros Terzis. 2020. Onward for the Freedom of Others: Marching beyond the AI Ethics. In Proceedings of the 2020 Conference on Fairness, Accountability, and Transparency (FAT* ’20) . ACM. https://doi.org/10.1145/3351095.3373152
- The European Parliament and the Council of the European Union (2018) The European Parliament and the Council of the European Union. 2018. General Data Protection Regulation (GDPR) . The European Parliament and the Council of the European Union. Retrieved January 2023 from https://eur-lex.europa.eu/legal-content/EN/TXT/PDF/?uri=CELEX:32016R0679
- The Organisation for Economic Co-operation and Development (2019) (OECD)
The Organisation for Economic Co-operation and Development (OECD). 2019. Recommendation of the Council on Artificial Intelligence . Retrieved February 2023 from https://legalinstruments.oecd.org/en/instruments/oecd-legal-0449
- Zytko et al . (2022) Douglas Zytko, Pamela J. Wisniewski, Shion Guha, Eric P. S. Baumer, and Min Kyung Lee. 2022. Participatory Design of AI Systems: Opportunities and Challenges Across Diverse Users, Relationships, and Application Domains. In Extended Abstracts of the 2022 CHI Conference on Human Factors in Computing Systems (CHI EA ’22) . ACM, Article 154. https://doi.org/10.1145/3491101.3516506
Appendix A Appendix: Summary of All Reviewed HCER-AI Research Papers
Citation | Research method | Year | Venue | Explainability | Human flourishing | Privacy | Security | Fairness | Governance |
---|---|---|---|---|---|---|---|---|---|
) | Theory | 2018 | AIES | \tikz\filldraw[gray, thick] (0,0) circle (.5ex); | \tikz\filldraw[gray, thick] (0,0) circle (.5ex); | ||||
( ) | Review & Theory | 2019 | AIES | \tikz\filldraw[gray, thick] (0,0) circle (.5ex); | |||||
( ) | Review & Theory | 2020 | AIES | \tikz\filldraw[gray, thick] (0,0) circle (.5ex); | |||||
) | Review | 2020 | AIES | \tikz\filldraw[gray, thick] (0,0) circle (.5ex); | |||||
) | Qualitative | 2021 | AIES | \tikz\filldraw[gray, thick] (0,0) circle (.5ex); | |||||
( ) | Qualitative | 2021 | AIES | \tikz\filldraw[gray, thick] (0,0) circle (.5ex); | \tikz\filldraw[gray, thick] (0,0) circle (.5ex); | \tikz\filldraw[gray, thick] (0,0) circle (.5ex); | |||
) | Qualitative | 2021 | AIES | \tikz\filldraw[gray, thick] (0,0) circle (.5ex); | |||||
) | Theory | 2021 | AIES | \tikz\filldraw[gray, thick] (0,0) circle (.5ex); | \tikz\filldraw[gray, thick] (0,0) circle (.5ex); | ||||
( ) | Qualitative | 2021 | AIES | \tikz\filldraw[gray, thick] (0,0) circle (.5ex); | \tikz\filldraw[gray, thick] (0,0) circle (.5ex); | ||||
) | Quantitative | 2021 | AIES | \tikz\filldraw[gray, thick] (0,0) circle (.5ex); | |||||
( ) | Qualitative | 2021 | AIES | \tikz\filldraw[gray, thick] (0,0) circle (.5ex); | \tikz\filldraw[gray, thick] (0,0) circle (.5ex); | ||||
( ) | Theory | 2021 | AIES | \tikz\filldraw[gray, thick] (0,0) circle (.5ex); | \tikz\filldraw[gray, thick] (0,0) circle (.5ex); | ||||
) | Review | 2022 | AIES | \tikz\filldraw[gray, thick] (0,0) circle (.5ex); | |||||
( ) | Review | 2022 | AIES | \tikz\filldraw[gray, thick] (0,0) circle (.5ex); | \tikz\filldraw[gray, thick] (0,0) circle (.5ex); | ||||
( ) | Qualitative | 2022 | AIES | \tikz\filldraw[gray, thick] (0,0) circle (.5ex); | |||||
( ) | Quantitative | 2022 | AIES | \tikz\filldraw[gray, thick] (0,0) circle (.5ex); | \tikz\filldraw[gray, thick] (0,0) circle (.5ex); | ||||
( ) | Quantitative | 2021 | AIES | \tikz\filldraw[gray, thick] (0,0) circle (.5ex); | |||||
) | Quantitative | 2021 | AIES | \tikz\filldraw[gray, thick] (0,0) circle (.5ex); | |||||
( ) | Qualitative | 2021 | AIES | \tikz\filldraw[gray, thick] (0,0) circle (.5ex); | |||||
( ) | Qualitative | 2021 | AIES | \tikz\filldraw[gray, thick] (0,0) circle (.5ex); | |||||
) | Quantitative | 2020 | AIES | \tikz\filldraw[gray, thick] (0,0) circle (.5ex); | |||||
) | Theory | 2021 | AIES | \tikz\filldraw[gray, thick] (0,0) circle (.5ex); | \tikz\filldraw[gray, thick] (0,0) circle (.5ex); | ||||
( ) | Theory | 2021 | AIES | \tikz\filldraw[gray, thick] (0,0) circle (.5ex); | |||||
( ) | Quantitative | 2022 | AIES | \tikz\filldraw[gray, thick] (0,0) circle (.5ex); | |||||
( ) | Quantitative | 2018 | AIES | \tikz\filldraw[gray, thick] (0,0) circle (.5ex); | |||||
) | Qualitative | 2021 | AIES | \tikz\filldraw[gray, thick] (0,0) circle (.5ex); | \tikz\filldraw[gray, thick] (0,0) circle (.5ex); | ||||
( ) | Theory | 2021 | AIES | \tikz\filldraw[gray, thick] (0,0) circle (.5ex); | \tikz\filldraw[gray, thick] (0,0) circle (.5ex); | \tikz\filldraw[gray, thick] (0,0) circle (.5ex); | \tikz\filldraw[gray, thick] (0,0) circle (.5ex); | ||
( ) | Quantitative | 2018 | AIES | \tikz\filldraw[gray, thick] (0,0) circle (.5ex); | |||||
( ) | Theory | 2018 | AIES | \tikz\filldraw[gray, thick] (0,0) circle (.5ex); | |||||
) | Theory | 2022 | AIES | \tikz\filldraw[gray, thick] (0,0) circle (.5ex); | |||||
( ) | Qualitative | 2020 | CHI | \tikz\filldraw[gray, thick] (0,0) circle (.5ex); | |||||
( ) | Qualitative | 2020 | CHI | \tikz\filldraw[gray, thick] (0,0) circle (.5ex); | |||||
( ) | Qualitative | 2021 | CHI | \tikz\filldraw[gray, thick] (0,0) circle (.5ex); | \tikz\filldraw[gray, thick] (0,0) circle (.5ex); | ||||
( ) | Qualitative | 2021 | CHI | \tikz\filldraw[gray, thick] (0,0) circle (.5ex); | \tikz\filldraw[gray, thick] (0,0) circle (.5ex); | ||||
( ) | Quantitative | 2021 | CHI | \tikz\filldraw[gray, thick] (0,0) circle (.5ex); | |||||
( ) | Qualitative | 2021 | CHI | \tikz\filldraw[gray, thick] (0,0) circle (.5ex); | \tikz\filldraw[gray, thick] (0,0) circle (.5ex); | \tikz\filldraw[gray, thick] (0,0) circle (.5ex); | |||
( ) | Qualitative | 2021 | CHI | \tikz\filldraw[gray, thick] (0,0) circle (.5ex); | \tikz\filldraw[gray, thick] (0,0) circle (.5ex); | \tikz\filldraw[gray, thick] (0,0) circle (.5ex); | \tikz\filldraw[gray, thick] (0,0) circle (.5ex); | ||
( ) | Mixed | 2021 | CHI | \tikz\filldraw[gray, thick] (0,0) circle (.5ex); | \tikz\filldraw[gray, thick] (0,0) circle (.5ex); | ||||
( ) | Review | 2021 | CHI | \tikz\filldraw[gray, thick] (0,0) circle (.5ex); | |||||
) | Qualitative | 2021 | CHI | \tikz\filldraw[gray, thick] (0,0) circle (.5ex); | |||||
( ) | Theory | 2022 | CHI | \tikz\filldraw[gray, thick] (0,0) circle (.5ex); | \tikz\filldraw[gray, thick] (0,0) circle (.5ex); | \tikz\filldraw[gray, thick] (0,0) circle (.5ex); | |||
) | Quantitative | 2022 | CHI | \tikz\filldraw[gray, thick] (0,0) circle (.5ex); | \tikz\filldraw[gray, thick] (0,0) circle (.5ex); | ||||
( ) | Mixed | 2022 | CHI | \tikz\filldraw[gray, thick] (0,0) circle (.5ex); | \tikz\filldraw[gray, thick] (0,0) circle (.5ex); | ||||
( ) | Qualitative | 2022 | CHI | \tikz\filldraw[gray, thick] (0,0) circle (.5ex); | |||||
( ) | Qualitative | 2022 | CHI | \tikz\filldraw[gray, thick] (0,0) circle (.5ex); | \tikz\filldraw[gray, thick] (0,0) circle (.5ex); | \tikz\filldraw[gray, thick] (0,0) circle (.5ex); | \tikz\filldraw[gray, thick] (0,0) circle (.5ex); | \tikz\filldraw[gray, thick] (0,0) circle (.5ex); | |
( ) | Mixed | 2022 | CHI | \tikz\filldraw[gray, thick] (0,0) circle (.5ex); | |||||
( ) | Review | 2022 | CHI | \tikz\filldraw[gray, thick] (0,0) circle (.5ex); | \tikz\filldraw[gray, thick] (0,0) circle (.5ex); | \tikz\filldraw[gray, thick] (0,0) circle (.5ex); | \tikz\filldraw[gray, thick] (0,0) circle (.5ex); | ||
( ) | Qualitative | 2022 | CHI | \tikz\filldraw[gray, thick] (0,0) circle (.5ex); | |||||
( ) | Mixed | 2022 | CHI | \tikz\filldraw[gray, thick] (0,0) circle (.5ex); | |||||
( ) | Qualitative | 2022 | CHI | \tikz\filldraw[gray, thick] (0,0) circle (.5ex); | \tikz\filldraw[gray, thick] (0,0) circle (.5ex); | ||||
( ) | Qualitative | 2022 | CHI | \tikz\filldraw[gray, thick] (0,0) circle (.5ex); | |||||
( ) | Qualitative | 2022 | CHI | \tikz\filldraw[gray, thick] (0,0) circle (.5ex); | |||||
) | Qualitative | 2022 | CHI | \tikz\filldraw[gray, thick] (0,0) circle (.5ex); | \tikz\filldraw[gray, thick] (0,0) circle (.5ex); | ||||
( ) | Theory | 2022 | CHI | \tikz\filldraw[gray, thick] (0,0) circle (.5ex); | \tikz\filldraw[gray, thick] (0,0) circle (.5ex); | ||||
( ) | Qualitative | 2022 | CHI | \tikz\filldraw[gray, thick] (0,0) circle (.5ex); | |||||
( ) | Mixed | 2022 | CHI | \tikz\filldraw[gray, thick] (0,0) circle (.5ex); | \tikz\filldraw[gray, thick] (0,0) circle (.5ex); | \tikz\filldraw[gray, thick] (0,0) circle (.5ex); | |||
( ) | Review | 2022 | CHI | \tikz\filldraw[gray, thick] (0,0) circle (.5ex); | \tikz\filldraw[gray, thick] (0,0) circle (.5ex); | ||||
( ) | Quantitative | 2022 | CHI | \tikz\filldraw[gray, thick] (0,0) circle (.5ex); | \tikz\filldraw[gray, thick] (0,0) circle (.5ex); | ||||
) | Quantitative | 2020 | CHI | \tikz\filldraw[gray, thick] (0,0) circle (.5ex); | \tikz\filldraw[gray, thick] (0,0) circle (.5ex); | ||||
( ) | Qualitative | 2021 | CHI | \tikz\filldraw[gray, thick] (0,0) circle (.5ex); | |||||
( ) | Mixed | 2021 | CHI | \tikz\filldraw[gray, thick] (0,0) circle (.5ex); | |||||
) | Quantitative | 2021 | CHI | \tikz\filldraw[gray, thick] (0,0) circle (.5ex); | \tikz\filldraw[gray, thick] (0,0) circle (.5ex); | ||||
( ) | Qualitative | 2021 | CHI | \tikz\filldraw[gray, thick] (0,0) circle (.5ex); | |||||
( ) | Qualitative | 2023 | CHI | \tikz\filldraw[gray, thick] (0,0) circle (.5ex); | |||||
( ) | Qualitative | 2023 | CHI | \tikz\filldraw[gray, thick] (0,0) circle (.5ex); | |||||
( ) | Qualitative | 2023 | CHI | \tikz\filldraw[gray, thick] (0,0) circle (.5ex); | \tikz\filldraw[gray, thick] (0,0) circle (.5ex); | \tikz\filldraw[gray, thick] (0,0) circle (.5ex); | |||
( ) | Qualitative | 2023 | CHI | \tikz\filldraw[gray, thick] (0,0) circle (.5ex); | \tikz\filldraw[gray, thick] (0,0) circle (.5ex); | ||||
( ) | Qualitative | 2023 | CHI | \tikz\filldraw[gray, thick] (0,0) circle (.5ex); | \tikz\filldraw[gray, thick] (0,0) circle (.5ex); | ||||
( ) | Mixed | 2023 | CHI | \tikz\filldraw[gray, thick] (0,0) circle (.5ex); | \tikz\filldraw[gray, thick] (0,0) circle (.5ex); | \tikz\filldraw[gray, thick] (0,0) circle (.5ex); | \tikz\filldraw[gray, thick] (0,0) circle (.5ex); | ||
( ) | Qualitative | 2023 | CHI | \tikz\filldraw[gray, thick] (0,0) circle (.5ex); | |||||
( ) | Qualitative | 2023 | CHI | \tikz\filldraw[gray, thick] (0,0) circle (.5ex); | |||||
( ) | Quantitative | 2023 | CHI | \tikz\filldraw[gray, thick] (0,0) circle (.5ex); | \tikz\filldraw[gray, thick] (0,0) circle (.5ex); | ||||
( ) | Quantitative | 2023 | CHI | \tikz\filldraw[gray, thick] (0,0) circle (.5ex); | |||||
) | Qualitative | 2023 | CHI | \tikz\filldraw[gray, thick] (0,0) circle (.5ex); | |||||
( ) | Mixed | 2023 | CHI | \tikz\filldraw[gray, thick] (0,0) circle (.5ex); | \tikz\filldraw[gray, thick] (0,0) circle (.5ex); | ||||
( ) | Qualitative | 2023 | CHI | \tikz\filldraw[gray, thick] (0,0) circle (.5ex); | |||||
( ) | Qualitative | 2023 | CHI | \tikz\filldraw[gray, thick] (0,0) circle (.5ex); | \tikz\filldraw[gray, thick] (0,0) circle (.5ex); | \tikz\filldraw[gray, thick] (0,0) circle (.5ex); | |||
( ) | Qualitative | 2023 | CHI | \tikz\filldraw[gray, thick] (0,0) circle (.5ex); | \tikz\filldraw[gray, thick] (0,0) circle (.5ex); | ||||
( ) | Qualitative | 2023 | CHI | \tikz\filldraw[gray, thick] (0,0) circle (.5ex); | \tikz\filldraw[gray, thick] (0,0) circle (.5ex); | \tikz\filldraw[gray, thick] (0,0) circle (.5ex); | |||
( ) | Mixed | 2023 | CHI | \tikz\filldraw[gray, thick] (0,0) circle (.5ex); | |||||
( ) | Quantitative | 2023 | CHI | \tikz\filldraw[gray, thick] (0,0) circle (.5ex); | |||||
( ) | Qualitative | 2023 | CHI | \tikz\filldraw[gray, thick] (0,0) circle (.5ex); | |||||
( ) | Qualitative | 2023 | CHI | \tikz\filldraw[gray, thick] (0,0) circle (.5ex); | |||||
( ) | Qualitative | 2023 | CHI | \tikz\filldraw[gray, thick] (0,0) circle (.5ex); | |||||
( ) | Mixed | 2023 | CHI | \tikz\filldraw[gray, thick] (0,0) circle (.5ex); | |||||
( ) | Mixed | 2023 | CHI | \tikz\filldraw[gray, thick] (0,0) circle (.5ex); | |||||
( ) | Qualitative | 2023 | CHI | \tikz\filldraw[gray, thick] (0,0) circle (.5ex); | |||||
( ) | Qualitative | 2023 | CHI | \tikz\filldraw[gray, thick] (0,0) circle (.5ex); | |||||
) | Qualitative | 2023 | CHI | \tikz\filldraw[gray, thick] (0,0) circle (.5ex); | |||||
( ) | Mixed | 2023 | CHI | \tikz\filldraw[gray, thick] (0,0) circle (.5ex); | |||||
( ) | Quantitative | 2023 | CHI | \tikz\filldraw[gray, thick] (0,0) circle (.5ex); | \tikz\filldraw[gray, thick] (0,0) circle (.5ex); | \tikz\filldraw[gray, thick] (0,0) circle (.5ex); | |||
( ) | Qualitative | 2023 | CHI | \tikz\filldraw[gray, thick] (0,0) circle (.5ex); | |||||
( ) | Mixed | 2023 | CHI | \tikz\filldraw[gray, thick] (0,0) circle (.5ex); | |||||
( ) | Qualitative | 2023 | CHI | \tikz\filldraw[gray, thick] (0,0) circle (.5ex); | |||||
( ) | Qualitative | 2023 | CHI | \tikz\filldraw[gray, thick] (0,0) circle (.5ex); | \tikz\filldraw[gray, thick] (0,0) circle (.5ex); | ||||
( ) | Qualitative | 2023 | CHI | \tikz\filldraw[gray, thick] (0,0) circle (.5ex); | \tikz\filldraw[gray, thick] (0,0) circle (.5ex); | ||||
( ) | Qualitative | 2023 | CHI | \tikz\filldraw[gray, thick] (0,0) circle (.5ex); | \tikz\filldraw[gray, thick] (0,0) circle (.5ex); | ||||
) | Qualitative | 2023 | CHI | \tikz\filldraw[gray, thick] (0,0) circle (.5ex); | |||||
( ) | Qualitative | 2021 | CSCW | \tikz\filldraw[gray, thick] (0,0) circle (.5ex); | |||||
( ) | Qualitative | 2022 | CSCW | \tikz\filldraw[gray, thick] (0,0) circle (.5ex); | \tikz\filldraw[gray, thick] (0,0) circle (.5ex); | ||||
( ) | Qualitative | 2021 | CSCW | \tikz\filldraw[gray, thick] (0,0) circle (.5ex); | |||||
( ) | Qualitative | 2022 | CSCW | \tikz\filldraw[gray, thick] (0,0) circle (.5ex); | |||||
( ) | Qualitative | 2019 | CSCW | \tikz\filldraw[gray, thick] (0,0) circle (.5ex); | \tikz\filldraw[gray, thick] (0,0) circle (.5ex); | ||||
( ) | Mixed | 2020 | CSCW | \tikz\filldraw[gray, thick] (0,0) circle (.5ex); | \tikz\filldraw[gray, thick] (0,0) circle (.5ex); | ||||
( ) | Mixed | 2020 | CSCW | \tikz\filldraw[gray, thick] (0,0) circle (.5ex); | \tikz\filldraw[gray, thick] (0,0) circle (.5ex); | ||||
( ) | Qualitative | 2023 | CSCW | \tikz\filldraw[gray, thick] (0,0) circle (.5ex); | |||||
( ) | Qualitative | 2019 | CSCW | \tikz\filldraw[gray, thick] (0,0) circle (.5ex); | |||||
( ) | Qualitative | 2023 | CSCW | \tikz\filldraw[gray, thick] (0,0) circle (.5ex); | \tikz\filldraw[gray, thick] (0,0) circle (.5ex); | ||||
) | Qualitative | 2021 | CSCW | \tikz\filldraw[gray, thick] (0,0) circle (.5ex); | \tikz\filldraw[gray, thick] (0,0) circle (.5ex); | \tikz\filldraw[gray, thick] (0,0) circle (.5ex); | |||
( ) | Quantitative | 2023 | CSCW | \tikz\filldraw[gray, thick] (0,0) circle (.5ex); | \tikz\filldraw[gray, thick] (0,0) circle (.5ex); | ||||
) | Qualitative | 2023 | CSCW | \tikz\filldraw[gray, thick] (0,0) circle (.5ex); | |||||
) | Qualitative | 2021 | CSCW | \tikz\filldraw[gray, thick] (0,0) circle (.5ex); | |||||
( ) | Mixed | 2021 | CSCW | \tikz\filldraw[gray, thick] (0,0) circle (.5ex); | |||||
( ) | Qualitative | 2019 | CSCW | \tikz\filldraw[gray, thick] (0,0) circle (.5ex); | |||||
( ) | Qualitative | 2022 | CSCW | \tikz\filldraw[gray, thick] (0,0) circle (.5ex); | |||||
( ) | Qualitative | 2023 | CSCW | \tikz\filldraw[gray, thick] (0,0) circle (.5ex); | |||||
( ) | Qualitative | 2021 | CSCW | \tikz\filldraw[gray, thick] (0,0) circle (.5ex); | |||||
) | Qualitative | 2020 | CSCW | \tikz\filldraw[gray, thick] (0,0) circle (.5ex); | |||||
( ) | Mixed | 2023 | CSCW | \tikz\filldraw[gray, thick] (0,0) circle (.5ex); | |||||
( ) | Qualitative | 2021 | CSCW | \tikz\filldraw[gray, thick] (0,0) circle (.5ex); | |||||
( ) | Qualitative | 2022 | CSCW | \tikz\filldraw[gray, thick] (0,0) circle (.5ex); | \tikz\filldraw[gray, thick] (0,0) circle (.5ex); | \tikz\filldraw[gray, thick] (0,0) circle (.5ex); | \tikz\filldraw[gray, thick] (0,0) circle (.5ex); | ||
( ) | Quantitative | 2022 | CSCW | \tikz\filldraw[gray, thick] (0,0) circle (.5ex); | |||||
( ) | Qualitative | 2021 | CSCW | \tikz\filldraw[gray, thick] (0,0) circle (.5ex); | |||||
( ) | Qualitative | 2023 | CSCW | \tikz\filldraw[gray, thick] (0,0) circle (.5ex); | |||||
( ) | Mixed | 2019 | CSCW | \tikz\filldraw[gray, thick] (0,0) circle (.5ex); | \tikz\filldraw[gray, thick] (0,0) circle (.5ex); | ||||
( ) | Qualitative | 2021 | CSCW | \tikz\filldraw[gray, thick] (0,0) circle (.5ex); | \tikz\filldraw[gray, thick] (0,0) circle (.5ex); | ||||
( ) | Quantitative | 2021 | CSCW | \tikz\filldraw[gray, thick] (0,0) circle (.5ex); | |||||
( ) | Qualitative | 2023 | CSCW | \tikz\filldraw[gray, thick] (0,0) circle (.5ex); | \tikz\filldraw[gray, thick] (0,0) circle (.5ex); | ||||
( ) | Qualitative | 2023 | CSCW | \tikz\filldraw[gray, thick] (0,0) circle (.5ex); | |||||
( ) | Quantitative | 2023 | CSCW | \tikz\filldraw[gray, thick] (0,0) circle (.5ex); | \tikz\filldraw[gray, thick] (0,0) circle (.5ex); | ||||
( ) | Qualitative | 2021 | CSCW | \tikz\filldraw[gray, thick] (0,0) circle (.5ex); | \tikz\filldraw[gray, thick] (0,0) circle (.5ex); | ||||
( ) | Qualitative | 2022 | CSCW | \tikz\filldraw[gray, thick] (0,0) circle (.5ex); | |||||
) | Qualitative | 2021 | FAccT | \tikz\filldraw[gray, thick] (0,0) circle (.5ex); | \tikz\filldraw[gray, thick] (0,0) circle (.5ex); | ||||
( ) | Theory | 2021 | FAccT | \tikz\filldraw[gray, thick] (0,0) circle (.5ex); | |||||
( ) | Qualitative | 2021 | FAccT | \tikz\filldraw[gray, thick] (0,0) circle (.5ex); | \tikz\filldraw[gray, thick] (0,0) circle (.5ex); | ||||
) | Theory | 2021 | FAccT | \tikz\filldraw[gray, thick] (0,0) circle (.5ex); | |||||
( ) | Quantitative | 2022 | FAccT | \tikz\filldraw[gray, thick] (0,0) circle (.5ex); | \tikz\filldraw[gray, thick] (0,0) circle (.5ex); | \tikz\filldraw[gray, thick] (0,0) circle (.5ex); | \tikz\filldraw[gray, thick] (0,0) circle (.5ex); | ||
( ) | Qualitative | 2022 | FAccT | \tikz\filldraw[gray, thick] (0,0) circle (.5ex); | \tikz\filldraw[gray, thick] (0,0) circle (.5ex); | ||||
) | Theory | 2022 | FAccT | \tikz\filldraw[gray, thick] (0,0) circle (.5ex); | |||||
( ) | Qualitative | 2022 | FAccT | \tikz\filldraw[gray, thick] (0,0) circle (.5ex); | \tikz\filldraw[gray, thick] (0,0) circle (.5ex); | \tikz\filldraw[gray, thick] (0,0) circle (.5ex); | |||
( ) | Quantitative | 2022 | FAccT | \tikz\filldraw[gray, thick] (0,0) circle (.5ex); | |||||
( ) | Review | 2022 | FAccT | \tikz\filldraw[gray, thick] (0,0) circle (.5ex); | |||||
( ) | Qualitative | 2022 | FAccT | \tikz\filldraw[gray, thick] (0,0) circle (.5ex); | \tikz\filldraw[gray, thick] (0,0) circle (.5ex); | ||||
) | Qualitative | 2022 | FAccT | \tikz\filldraw[gray, thick] (0,0) circle (.5ex); | |||||
( ) | Qualitative | 2022 | FAccT | \tikz\filldraw[gray, thick] (0,0) circle (.5ex); | |||||
( ) | Mixed | 2022 | FAccT | \tikz\filldraw[gray, thick] (0,0) circle (.5ex); | |||||
( ) | Theory | 2022 | FAccT | \tikz\filldraw[gray, thick] (0,0) circle (.5ex); | \tikz\filldraw[gray, thick] (0,0) circle (.5ex); | ||||
) | Quantitative | 2022 | FAccT | \tikz\filldraw[gray, thick] (0,0) circle (.5ex); | \tikz\filldraw[gray, thick] (0,0) circle (.5ex); | ||||
( ) | Review & Theory | 2022 | FAccT | \tikz\filldraw[gray, thick] (0,0) circle (.5ex); | |||||
) | Qualitative | 2022 | FAccT | \tikz\filldraw[gray, thick] (0,0) circle (.5ex); | |||||
( ) | Theory | 2022 | FAccT | \tikz\filldraw[gray, thick] (0,0) circle (.5ex); | |||||
( ) | Theory | 2022 | FAccT | \tikz\filldraw[gray, thick] (0,0) circle (.5ex); | |||||
( ) | Mixed | 2022 | FAccT | \tikz\filldraw[gray, thick] (0,0) circle (.5ex); | |||||
( ) | Qualitative | 2022 | FAccT | \tikz\filldraw[gray, thick] (0,0) circle (.5ex); | \tikz\filldraw[gray, thick] (0,0) circle (.5ex); | ||||
) | Review | 2021 | FAccT | \tikz\filldraw[gray, thick] (0,0) circle (.5ex); | \tikz\filldraw[gray, thick] (0,0) circle (.5ex); | ||||
( ) | Quantitative | 2022 | FAccT | \tikz\filldraw[gray, thick] (0,0) circle (.5ex); | |||||
) | Theory | 2020 | FAccT | \tikz\filldraw[gray, thick] (0,0) circle (.5ex); | |||||
( ) | Review & Theory | 2020 | FAccT | \tikz\filldraw[gray, thick] (0,0) circle (.5ex); | \tikz\filldraw[gray, thick] (0,0) circle (.5ex); | \tikz\filldraw[gray, thick] (0,0) circle (.5ex); | \tikz\filldraw[gray, thick] (0,0) circle (.5ex); | ||
( ) | Review & Theory | 2022 | FAccT | \tikz\filldraw[gray, thick] (0,0) circle (.5ex); | |||||
) | Theory | 2020 | FAccT | \tikz\filldraw[gray, thick] (0,0) circle (.5ex); | |||||
) | Qualitative | 2020 | FAccT | \tikz\filldraw[gray, thick] (0,0) circle (.5ex); | |||||
( ) | Theory | 2022 | FAccT | \tikz\filldraw[gray, thick] (0,0) circle (.5ex); | \tikz\filldraw[gray, thick] (0,0) circle (.5ex); | ||||
) | Theory | 2021 | FAccT | \tikz\filldraw[gray, thick] (0,0) circle (.5ex); | |||||
( ) | Theory | 2022 | FAccT | \tikz\filldraw[gray, thick] (0,0) circle (.5ex); |
Appendix B Appendix: Interactions With ChatGPT 4.0
We first let ChatGPT 4.0 know that our content is larger than its text limit with the following prompt:
“The total length of the content that I want to send you is too large to send in only one piece. For sending you that content, I will follow this rule: [START PART 1/15] this is the content of the part 1 out of 15 in total [END PART 1/15] Then you just answer: ‘Received part 1/15’
And when I tell you ‘ALL PARTS SENT,’ then you can continue processing the data and answering my requests.”
B.1. Thematic Analysis for Research Papers
ChatGPT generated the following text with minor stylist changes using all the abstracts of the 164 papers in our review. We first asked for “apply thematic analysis to all of the previous 15 prompts based on the method proposed by Braun and Clarke (2008) and generate themes,” which resulted in step-by-step instructions for how to do the thematic analysis. In a follow-up prompt, we asked to “produce a report based on the thematic analysis proposed by Braun and Clarke (2008).” ChatGPT produced the following paragraphs with this prompt:
This approach led to the identification of four main themes, each underscoring key areas of interest and investigation among researchers in the domain of AI ethics. The themes are as follows: Ethical Implications of AI, Practical Applications of AI, Understanding and Documenting Data, and User Engagement.
Theme 1: Ethical Implications of AI
This theme emerges prominently, signaling that ethical considerations are of primary concern in AI research and practice. The studies under this theme scrutinize ethics codes, considering how they may conflate consumers with society and ignore the political aspects of shared resources. The analyses highlight a gulf between the ideals presented in ethics codes and lived experiences, particularly for marginalized communities.
Moreover, this theme reflects the power of collective action among tech workers as a countervailing force against corporate impulses to prioritize growth or profit over ethical considerations. Successful campaigns are discussed, along with frameworks for future action. This suggests a recognition of the potential for internal dynamics within tech companies to act as a transformative lever in the pursuit of ethical AI.
Theme 2: Practical Applications of AI
This theme examines the deployment of AI in real-world contexts, such as healthcare and disaster risk management. AI’s practical applications can offer significant benefits, from improving clinician workflows and patient outcomes in the detection of diabetic eye disease to supporting disaster risk management by making data collection or analysis practices faster, less costly, or more accurate.
However, these studies also acknowledge the potential for negative consequences. In healthcare, socio-environmental factors can impact model performance and patient experience, emphasizing the need for human-centered evaluative research. In disaster risk management, discussions with technical experts reveal the need for careful consideration of potential negative impacts and the identification of mitigation strategies.
Theme 3: Understanding and Documenting Data
This theme addresses the challenges and importance of understanding and documenting the data that fuel AI models. Recognizing that the origins, development, intent, and evolution of datasets influence the resultant models, researchers propose a shift from merely documenting datasets towards documenting data production.
One notable contribution is the proposal of “Data Cards,” structured summaries of essential facts about ML datasets, designed to support transparent, purposeful, and human-centered documentation of datasets. The goal is to make data documentation a user-centric product, providing stakeholders with the critical information necessary for responsible AI development.
Theme 4: User Engagement
The final theme highlights the importance of user engagement in AI systems. A growing interest among researchers and practitioners lies in user-engaged approaches to algorithm auditing. The challenges faced by industry practitioners, such as recruiting and incentivizing user auditors, scaffolding user audits, and deriving actionable insights from user-engaged audit reports, are thoroughly discussed.
Further, the studies suggest a complex relationship between practitioners and user auditors, which represents organizational obstacles to user-engaged auditing. Addressing these obstacles could lead to more robust and user-responsive AI systems, amplifying the potential for effective AI auditing and user-focused AI implementation.
In conclusion, the themes identified through the thematic analysis reveal the multifaceted nature of AI ethics research, which is as concerned with technical aspects as it is with the ethical implications, practical applications, understanding and documenting data, and engaging users in the AI systems lifecycle. The interplay of these themes will undoubtedly continue to shape the discourse in the field.
B.2. Research Methods of Research Papers
Here we asked ChatGPT to about the research methods used in the abstract of the 164 papers using this prompt: “what are the research methods used the all the previous prompts?” Below is the answer:
Interviews: Semi-structured interviews were a common method across several summaries. They were used to gather information about experiences and perspectives from various stakeholders such as data workers, industry practitioners, community organizers, and clinic staff.
Participatory Design and Co-Design Workshops: These methods involve users or stakeholders in the design process to ensure that the resulting system or product meets their needs and is usable in the intended context.
Prototyping: This approach involves creating early samples, models, or releases of a product to test a concept or process.
Observation: Some of the summaries mentioned direct observation as a way to understand current workflows and contexts, such as in the case of observing eye-screening workflows in Thai clinics.
Critical Discourse Analysis: This is a qualitative research method used to analyze written or spoken language within its social context. One study used this method to scrutinize data ethics codes.
Iterative Co-Design Activities: This approach combines co-design, which involves stakeholders in the design process, with iteration, repeating a process to refine and improve the outcome.
Characterization of Collective Actions: This method was used to analyze a collection of documented actions to identify patterns, themes, or trends.
Case Studies: Several summaries reported the use of case studies, which involve in-depth investigations of a single individual, group, or event. This approach can provide a detailed context to understand complex issues.
Prospective Evaluations of Model Accuracy: These evaluations involve testing a model’s performance against a pre-established benchmark or goal.
Convening Technical Experts for Discussion: In one summary, experts were brought together to discuss potential negative impacts of AI in disaster risk management.
B.3. Future Research Recommendations Based on the Research Papers
Here we asked ChatGPT to about the research recommendations and future research directions based on the abstract of the 164 papers using this prompt: “what are some the research recommendation and future research directions based on the thematic analysis ?” Below is the answer:
User-Centric Design: It’s evident that user-focused design needs to be central in the development of AI systems. Future research can focus on understanding the needs of different user groups, developing better tools to engage users in the design process, and exploring ways to make AI systems more transparent and understandable.
Greater Transparency in AI: The need for more transparency in AI systems was highlighted in the data. Future research can explore methods and techniques to improve transparency, including the development of comprehensive documentation practices and standards.
AI in Marginalized Communities: The impact of AI and data technologies on marginalized communities was a key concern. Future work can focus on understanding these impacts in greater detail, developing technologies that support these communities, and exploring methods to ensure that their needs and concerns are considered in the design of AI systems.
Collective Action in Tech Industry: The potential power of collective action within the tech industry to address ethical issues was highlighted. Future research can explore this further, looking at how to support and facilitate such actions and understanding their impacts and effectiveness.
Real-World AI Evaluations: The need for more real-world evaluation of AI systems was evident. Future research can focus on developing methodologies for these evaluations, exploring the impacts of real-world contexts on AI performance, and understanding how AI systems can be effectively integrated into existing workflows.
AI in Disaster Risk Management: AI has potential to assist in disaster risk management, but more research is needed to understand its possible negative consequences. Future work could focus on investigating these consequences, developing strategies to mitigate potential harms, and building frameworks for responsible use of AI in this context.
Effective AI Communication: Developing effective communication strategies for AI, particularly for non-experts, is critical. Future research should investigate how to best communicate about AI, its capabilities and limitations, and the ethical issues it raises.
AI Ethics Integration in Education: The integration of AI ethics into educational programs is a key area for future exploration. Research could focus on developing and evaluating curricula, teaching methods, and assessments that effectively engage students in these topics.
Work-Integrated Learning for AI: Exploring work-integrated learning in the context of AI is a promising area for future research. This could involve studying the effectiveness of different models of work-integrated learning, and how they can be used to prepare students for ethical challenges in the AI industry.
AI Governance Practices: Future work should delve into establishing effective AI governance practices. This includes studying how AI can be regulated, what forms of oversight are most effective, and how organizations can implement ethical AI practices.
B.4. Thematic Analysis for Patents
ChatGPT generated the following text with minor stylist changes using all the abstracts of the 28 patents in our review. We first asked for “produce a report based on the thematic analysis proposed by Braun and Clarke (2008),” ChatGPT produced the following paragraphs with this prompt:
AI-driven decision making and predictions: This theme appeared as a prevalent aspect in many of the patent abstracts. In these cases, AI technologies are being utilized to predict and inform decisions in various areas, such as media content, environmental data, brain state, and security provisioning. For example, one of the inventions predicts moral insight scores from media content using an AI model. This theme demonstrates the value and potential of AI in shaping future decision-making processes in diverse fields.
Transparency, Interpretability, and Explainability in AI: This theme encapsulates the patents that aim to make AI models more understandable and transparent. Given the often ’black-box’ nature of AI systems, efforts are being made to develop systems that can provide explanations for the output they produce, thus increasing their reliability, credibility, and usability. For instance, one patent describes a system that couples interpretable and non-interpretable systems to provide explanations for AI-driven decisions. This theme underlines the importance of accountability and interpretability in the development and application of AI systems.
Machine Learning Model Performance & Enhancement: A subset of patents deal with improving the performance and capabilities of machine learning models, ranging from accuracy and efficiency to robustness and fairness. For instance, one patent discusses using a stochastic process and a novel hypothesis test to compare the performance of black-box models, providing a method to derive global and local explanations. This theme underscores the ongoing innovation in the development and refinement of machine learning models, which is critical to maximizing their utility and application in different fields.

IMAGES
VIDEO
COMMENTS
This paper discusses literature review as a methodology for conducting research and offers an overview of different types of reviews, as well as some guidelines to how to both conduct and evaluate a literature review paper. It also discusses common pitfalls and how to get literature reviews published. 1. Introduction.
Examples of literature reviews. Step 1 - Search for relevant literature. Step 2 - Evaluate and select sources. Step 3 - Identify themes, debates, and gaps. Step 4 - Outline your literature review's structure. Step 5 - Write your literature review.
Definitely, there are many frameworks within the Seven-Step Model, such as steps within steps. Therefore, the CLR is a meta-framework. For example, in Step 1: Exploring Beliefs and Topics, we provide many parts of the belief system, such as worldview, field/discipline-specific beliefs, and topic-specific beliefs.
A literature review can be a part of a research paper or scholarly article, usually falling after the introduction and before the research methods sections. In these cases, the lit review just needs to cover scholarship that is important to the issue you are writing about; sometimes it will also cover key sources that informed your research ...
The literature review can serve various functions in the contexts of education and research. It aids in identifying knowledge gaps, informing research methodology, and developing a theoretical framework during the planning stages of a research study or project, as well as reporting of review findings in the context of the existing literature.
This paper draws input from a study that employed a systematic literature review as its main source of data. A systematic review can be explained as a research method and process for identifying ...
Introduction Researchers and practitioners rely on literature reviews to synthesize large bodies of knowledge. Many types of literature reviews have been developed, each targeting a specific purpose. However, these syntheses are hampered if the review type's paradigmatic roots, methods, and markers of rigor are only vaguely understood. One literature review type whose methodology has yet to ...
A Systematic Literature Review (SLR) is a research methodology to collect, identify, and critically analyze the available research studies (e.g., articles, conference proceedings, books, dissertations) through a systematic procedure [12].An SLR updates the reader with current literature about a subject [6].The goal is to review critical points of current knowledge on a topic about research ...
The systematic literature review (SLR) is one of the important review methodologies which is increasingly becoming popular to synthesize literature in any discipline in general and management in particular. In this article, we explain the SLR methodology and provide guidelines for performing and documenting these studies.
Literature reviews are in great demand in most scientific fields. Their need stems from the ever-increasing output of scientific publications .For example, compared to 1991, in 2008 three, eight, and forty times more papers were indexed in Web of Science on malaria, obesity, and biodiversity, respectively .Given such mountains of papers, scientists cannot be expected to examine in detail every ...
9.3. Types of Review Articles and Brief Illustrations. EHealth researchers have at their disposal a number of approaches and methods for making sense out of existing literature, all with the purpose of casting current research findings into historical contexts or explaining contradictions that might exist among a set of primary research studies conducted on a particular topic.
A literature review is an integrated analysis-- not just a summary-- of scholarly writings and other relevant evidence related directly to your research question.That is, it represents a synthesis of the evidence that provides background information on your topic and shows a association between the evidence and your research question.
Types of Literature Review are as follows: Narrative literature review: This type of review involves a comprehensive summary and critical analysis of the available literature on a particular topic or research question. It is often used as an introductory section of a research paper. Systematic literature review: This is a rigorous and ...
4 Tips for Writing a Literature Review's Intro, Body, and Conclusion | Scribbr. While each review will be unique in its structure--based on both the existing body of both literature and the overall goals of your own paper, dissertation, or research--this video from Scribbr does a good job simplifying the goals of writing a literature review for those who are new to the process.
A literature review may consist of simply a summary of key sources, but in the social sciences, a literature review usually has an organizational pattern and combines both summary and synthesis, often within specific conceptual categories.A summary is a recap of the important information of the source, but a synthesis is a re-organization, or a reshuffling, of that information in a way that ...
Example: Predictors and Outcomes of U.S. Quality Maternity Leave: A Review and Conceptual Framework: 10.1177/08948453211037398 ; Systematic review: "The authors of a systematic review use a specific procedure to search the research literature, select the studies to include in their review, and critically evaluate the studies they find." (p. 139).
Elements of a Literature Review. Summarize subject, issue or theory under consideration, along with objectives of the review; Divide works under review into categories (e.g. those in support of a particular position, those against, those offering alternative theories entirely) Explain how each work is similar to and how it varies from the others
Literature Review is a comprehensive survey of the works published in a particular field of study or line of research, usually over a specific period of time, in the form of an in-depth, critical bibliographic essay or annotated list in which attention is drawn to the most significant works.. Also, we can define a literature review as the collected body of scholarly works related to a topic:
Abstract. Performing a literature review is a critical first step in research to understanding the state-of-the-art and identifying gaps and challenges in the field. A systematic literature review is a method which sets out a series of steps to methodically organize the review. In this paper, we present a guide designed for researchers and in ...
Information providing guidance on starting a literature review, including resources, techniques and approaches to searching the literature and writing the review. ... The methodology section of a research paper, or thesis, enables the reader to critically evaluate the study's validity and reliability by addressing how the data was collected ...
Literature reviews establish the foundation of academic inquires. However, in the planning field, we lack rigorous systematic reviews. In this article, through a systematic search on the methodology of literature review, we categorize a typology of literature reviews, discuss steps in conducting a systematic literature review, and provide suggestions on how to enhance rigor in literature ...
They can also vary in granularity: a literature review in the beginning of an article might only summarize the largest or most influential studies, while an academic literature review will not only describe the research so far but look for common themes, analyze the quality of the research, and explain gaps where further research is needed.
These were excellent papers from her class, but it does not mean they are perfect or contain no errors. Thanks to the students who let us post! Literature Review Sample 1. Literature Review Sample 2. Literature Review Sample 3. Have an exemplary literature review? Have you written a stellar literature review you care to share for teaching purposes?
This method works well if you are trying to identify a sub-topic that has so far been overlooked by other researchers. Methodologically: group your sources by methodology. For example, divide the literature into categories like qualitative versus quantitative, or by population or geographical region, etc.
This research article seeks to build on the work done by Sahakyan et al., by providing an up-to-date survey of XAI techniques relevant to tabular data by thoroughly analyzing previous studies. The method used in this literature review is shown in Figure 2. Initially, the research topic was defined to understand the scope of the review.
Mapping the gap. The purpose of the literature review section of a manuscript is not to report what is known about your topic. The purpose is to identify what remains unknown—what academic writing scholar Janet Giltrow has called the 'knowledge deficit'—thus establishing the need for your research study [].In an earlier Writer's Craft instalment, the Problem-Gap-Hook heuristic was ...
Applying a mixed methodology, this paper uses a bibliometric technique to identify the main topics studied in Digital HR. ... further literature review work is needed on the term Digital HR to ...
Background The origin of Narrative Medicine dates back to more than 20 years ago at an international level. Narrative Medicine is not an alternative to evidence-based medicine, however these two approaches are integrated. Narrative Medicine is a methodology based on specific communication skills where storytelling is a fundamental tool to acquire, understand and integrate several points of ...
In the 164 papers reviewed, 85 were qualitative (e.g., user studies for system design and evaluation, interviews, and workshops), 25 were quantitative (e.g., survey and log analysis), 21 were theoretical (e.g., essays and framework proposal), 20 were mixed-methods (a combination of qualitative and quantitative), 8 were reviews (e.g., review of ...
This systematic review considered articles investigating violence within domestic settings in adults (including women and men), during the COVID-19 outbreak. The search parameters for this review were time-bound, covering the period from January 2020 to March 2021, to ensure a comprehensive retrieval of relevant literature.