Dynamics-inspired Neuromorphic Visual Representation Learning
Cite this paper, related material.
- Download PDF

default search action
- combined dblp search
- author search
- venue search
- publication search


"Dynamics-inspired Neuromorphic Visual Representation Learning."
Details and statistics.
DOI: —

type: Conference or Workshop Paper
metadata version: 2023-08-28

manage site settings
To protect your privacy, all features that rely on external API calls from your browser are turned off by default . You need to opt-in for them to become active. All settings here will be stored as cookies with your web browser. For more information see our F.A.Q.
Unpaywalled article links

load links from unpaywall.org
Privacy notice: By enabling the option above, your browser will contact the API of unpaywall.org to load hyperlinks to open access articles. Although we do not have any reason to believe that your call will be tracked, we do not have any control over how the remote server uses your data. So please proceed with care and consider checking the Unpaywall privacy policy .
Archived links via Wayback Machine

load content from archive.org
Privacy notice: By enabling the option above, your browser will contact the API of archive.org to check for archived content of web pages that are no longer available. Although we do not have any reason to believe that your call will be tracked, we do not have any control over how the remote server uses your data. So please proceed with care and consider checking the Internet Archive privacy policy .
Reference lists

load references from crossref.org and opencitations.net
Privacy notice: By enabling the option above, your browser will contact the APIs of crossref.org , opencitations.net , and semanticscholar.org to load article reference information. Although we do not have any reason to believe that your call will be tracked, we do not have any control over how the remote server uses your data. So please proceed with care and consider checking the Crossref privacy policy and the OpenCitations privacy policy , as well as the AI2 Privacy Policy covering Semantic Scholar.
Citation data
load citations from opencitations.net
Privacy notice: By enabling the option above, your browser will contact the API of opencitations.net and semanticscholar.org to load citation information. Although we do not have any reason to believe that your call will be tracked, we do not have any control over how the remote server uses your data. So please proceed with care and consider checking the OpenCitations privacy policy as well as the AI2 Privacy Policy covering Semantic Scholar.
OpenAlex data

load data from openalex.org
Privacy notice: By enabling the option above, your browser will contact the API of openalex.org to load additional information. Although we do not have any reason to believe that your call will be tracked, we do not have any control over how the remote server uses your data. So please proceed with care and consider checking the information given by OpenAlex .
last updated on 2023-08-28 17:23 CEST by the dblp team

see also: Terms of Use | Privacy Policy | Imprint
dblp was originally created in 1993 at:
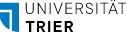
since 2018, dblp has been operated and maintained by:
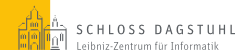
the dblp computer science bibliography is funded and supported by:
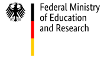
Thank you for visiting nature.com. You are using a browser version with limited support for CSS. To obtain the best experience, we recommend you use a more up to date browser (or turn off compatibility mode in Internet Explorer). In the meantime, to ensure continued support, we are displaying the site without styles and JavaScript.
- View all journals
- My Account Login
- Explore content
- About the journal
- Publish with us
- Sign up for alerts
- Open access
- Published: 01 November 2023
Online dynamical learning and sequence memory with neuromorphic nanowire networks
- Ruomin Zhu ORCID: orcid.org/0000-0002-2310-7762 1 na1 ,
- Sam Lilak 2 na1 ,
- Alon Loeffler 1 ,
- Joseph Lizier ORCID: orcid.org/0000-0002-9910-8972 3 , 4 ,
- Adam Stieg ORCID: orcid.org/0000-0001-7312-9364 5 , 6 ,
- James Gimzewski 2 , 5 , 6 , 7 &
- Zdenka Kuncic ORCID: orcid.org/0000-0002-8866-3073 1 , 4 , 8
Nature Communications volume 14 , Article number: 6697 ( 2023 ) Cite this article
24k Accesses
11 Citations
1002 Altmetric
Metrics details
- Electronic devices
- Information theory and computation
Nanowire Networks (NWNs) belong to an emerging class of neuromorphic systems that exploit the unique physical properties of nanostructured materials. In addition to their neural network-like physical structure, NWNs also exhibit resistive memory switching in response to electrical inputs due to synapse-like changes in conductance at nanowire-nanowire cross-point junctions. Previous studies have demonstrated how the neuromorphic dynamics generated by NWNs can be harnessed for temporal learning tasks. This study extends these findings further by demonstrating online learning from spatiotemporal dynamical features using image classification and sequence memory recall tasks implemented on an NWN device. Applied to the MNIST handwritten digit classification task, online dynamical learning with the NWN device achieves an overall accuracy of 93.4%. Additionally, we find a correlation between the classification accuracy of individual digit classes and mutual information. The sequence memory task reveals how memory patterns embedded in the dynamical features enable online learning and recall of a spatiotemporal sequence pattern. Overall, these results provide proof-of-concept of online learning from spatiotemporal dynamics using NWNs and further elucidate how memory can enhance learning.
Similar content being viewed by others
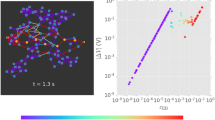
Information dynamics in neuromorphic nanowire networks
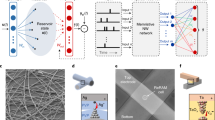
In materia reservoir computing with a fully memristive architecture based on self-organizing nanowire networks
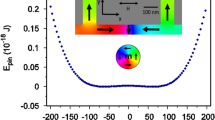
Neuromorphic computation with a single magnetic domain wall
Introduction.
Neuromorphic devices offer the potential for a fundamentally new computing paradigm, one based on a brain-inspired architecture that promises enormous efficiency gains over conventional computing architectures 1 , 2 , 3 , 4 , 5 , 6 , 7 , 8 , 9 , 10 , 11 . A particularly successful neuromorphic computing approach is the implementation of spike-based neural network algorithms in CMOS-based neuromorphic hardware 2 , 12 , 13 , 14 , 15 , 16 , 17 . An alternate neuromorphic computing approach is to exploit brain-like physical properties exhibited by novel nano-scale materials and structures 18 , 19 , 20 , 21 , 22 , including, in particular, the synapse-like dynamics of resistive memory (memristive) switching 4 , 23 , 24 , 25 , 26 , 27 , 28 , 29 , 30 , 31 .
This study focuses on a class of neuromorphic devices based on memristive nanowire networks (NWNs) 32 , 33 . NWNs are comprised of metal-based nanowires that form a heterogeneous network structure similar to a biological neural network 34 , 35 , 36 , 37 , 38 . Additionally, nanowire-nanowire cross-point junctions exhibit memristive switching attributed to the evolution of a metallic nano-filament due to electro-chemical metallisation 39 , 40 , 41 , 42 , 43 . Typically, each NWN contains thousands of nanowires and an even greater number of junctions. In response to electrical input signals, NWNs also exhibit brain-like collective dynamics (e.g., phase transitions, switch synchronisation, avalanche criticality), resulting from the interplay between memristive switching and their recurrent network structure 34 , 37 , 44 , 45 , 46 , 47 , 48 , 49 , 50 .
Recurrent, sparse networks can transform temporal signals into a higher-dimensional dynamical feature space 51 , 52 , which is advantageous for machine learning applications involving dynamically evolving data 53 . Furthermore, the computational burden of training network weights can be circumvented altogether by leveraging Reservoir Computing (RC), which restricts training to a linear output layer, in which only linear weights need to be learned using the rich dynamical features generated by the recurrent network reservoir 54 , 55 , 56 . Physical systems are particularly useful as reservoirs, due to their self-regulating dynamics and physical constraints imposed by conservation laws (e.g., Kirchoff’s laws), in contrast to algorithmic RC, which typically uses a random network with fixed weights and requires manual hyper-parameter optimisation 57 . Previous experimental 36 , 58 , 59 and simulation 36 , 58 , 60 , 61 , 62 , 63 , 64 , 65 studies have demonstrated NWNs exhibit fading memory and can effectively project input signals to a higher-dimensional feature space, thus enabling their use as physical reservoirs in an RC approach to machine learning.
In previous physical RC studies, learning is achieved by training the readout weights after the entire input stream is delivered to the physical system 66 , while the real-time response from the network is not fully reflected in the learning outcome. While such batch-based approaches can be practically limited by memory availability when working with large datasets, an arguably more important consideration is the need to re-train weights when feature distributions evolve 67 . An alternate approach is online training, which has the potential to enhance dynamical learning by allowing the readout weights to adapt to non-stationary dynamical features incrementally 68 , 69 . As is the case for conventional machine learning, online learning approaches are necessary for scaling up neuromorphic computing and ultimately achieving the goal of continual learning 70 , 71 , 72 .
In this study, we use an NWN device to demonstrate online dynamical learning , i.e., learning incrementally from continuous streams of dynamical features. We implement an online training algorithm within an RC framework and use the MNIST handwritten digit database to deliver a stream of spatiotemporal patterns to the NWN device. Dynamical features in the device readouts are then used to train a linear classifier in an online manner, sample by sample, and information-theoretic measures are used to analyse the online learning process. By constructing a numerical sequence pattern using the MNIST database, we then develop and implement a novel sequence memory task that demonstrates the NWN’s ability to generate spatiotemporal memory patterns in a similar manner to the brain, using attractor dynamics. We show how these sequence memory patterns can also be learned in an online manner and then used to recall a target digit presented earlier in the sequence. By comparing recall performance with and without memory patterns, we demonstrate how memory enhances learning.
The first task we performed to test online dynamical learning is the MNIST handwritten digit classification task, which has not previously been experimentally implemented on an NWN device (but has been implemented in NWN simulations 62 , 65 ). A schematic illustration of the experimental setup for the online classification of MNIST handwritten digits using an NWN multi-electrode array (MEA) device is shown in Fig. 1 . MNIST digit images 73 are converted to 1-D temporal voltage pulse streams and delivered consecutively to one electrode. The network’s real-time response is read out from other electrode channels and classification is performed in an external (digital) fully-connected output layer. The weights are learned from the dynamical features and updated after each digit sample using an online iterative algorithm based on recursive least squares (RLS). See “Methods” for full details.
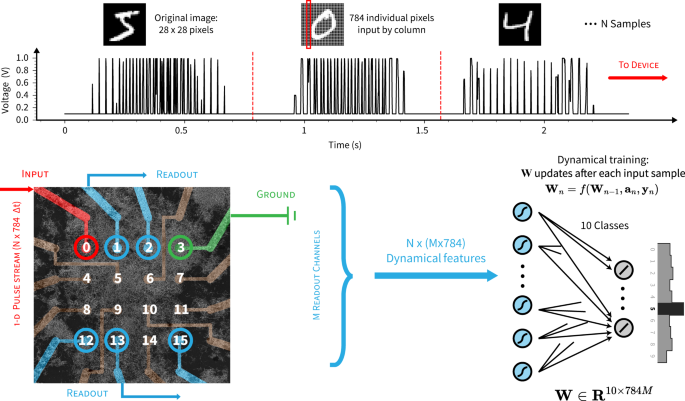
Top: MNIST handwritten digit samples ( N samples × 784 pixel features) are normalised and converted to 1-D temporal voltage pulse streams (each pixel occupies Δ t = 0.001 s ) and delivered consecutively to the nanowire multi-electrode device. Bottom left: scanning electron micrograph image of the 16-electrode device, showing source electrode (channel 0, red), drain electrode (channel 3, green), readout electrodes (channel 1, 2, 12, 13, 15, blue) and other electrodes not used (brown). Bottom right: readout voltages (i.e., N × M × 784 dynamical features) are input into an external linear classifier in which the weight matrix W n for the M × 784 features per digit sample is updated after each sample a n , with corresponding class y n as the target output (digit `5' shown as an example of classification result).
Dynamical feature generation
Figure 2 shows examples of handwritten digit image samples converted to 1-D voltage pulse streams delivered to the allocated source electrode (channel 0) and the corresponding voltage streams read out from other channels (1, 2, 12, 13 and 15) for the setup shown in Fig. 1 (readouts for other channels and digits are shown in Supplementary Figs. S4 and S5) .
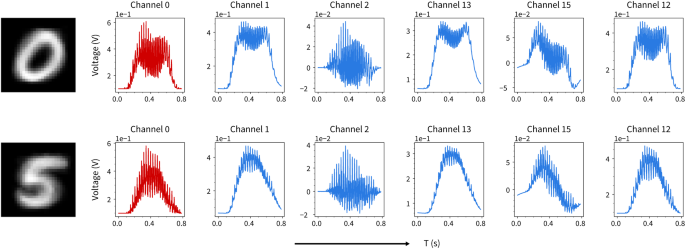
Images of MNIST digits ‘0’ and ‘5’ averaged across 100 samples randomly selected from the training set (column 1), their corresponding input voltage streams (column 2, red) and readout voltages from multiple channels (columns 3–7, blue).
Each row in Fig. 2 shows the averaged image, input and readout data for 100 MNIST samples randomly selected from the training set for the corresponding digit class.
For each class, the readout voltages from each channel (columns 3–7, blue) are distinctly different from the corresponding input voltages and exhibit diverse characteristics across the readout channels. This demonstrates that the NWN nonlinearly maps the input signals into a higher-dimensional space. Rich and diverse dynamical features are embedded into the channel readouts from the spatially distributed electrodes, which are in contact with different parts of the network (see Supplementary Fig. S6 for additional non-equilibrium dynamics under non-adiabatic conditions). We show below how the inter-class distinctiveness of these dynamical features, as well as their intra-class diversity, can be harnessed to perform online classification of the MNIST digits.
Online learning
Table 1 presents the MNIST handwritten digit classification results using the online method (external weights trained by an RLS algorithm). Results are shown for one and five readout channels. For comparison, also shown are the corresponding classification results using the offline batch method (external weights trained by backpropagation with gradient descent). Both classifiers learn from the dynamical features extracted from the NWN, with readouts delivered to the two classifiers separately. For both classifiers, accuracies increase with the number of readout channels, demonstrating the non-linearity embedded by the network in the readout data. For the same number of channels, however, the online method outperforms the batch method. In addition to achieving a higher classification accuracy, the online classifier W requires only a single epoch of 50,000 training samples, compared to 100 training epochs for the batch method using 500 mini-batches of 100 samples and a learning rate η = 0.1. The accuracy of the online classifier becomes comparable to that of the batch classifier when active error correction is not used in the RLS algorithm (see Supplementary Table 1) . A key advantage of the online method is that continuous learning from the streaming input data enables relatively rapid convergence, as shown next.
To better understand how learning is achieved with the NWN device, we investigated further the dependence of classification accuracy on the number of training samples and the device readouts. Figure 3 a shows classification accuracy as a function of the number of digits presented to the classifier during training (See Supplementary Fig. S7 for classification results using different electrode combinations for input/drain/readouts and different voltage ranges). The classification accuracy consistently increases as more readout samples are presented to the classifier to update W and plateaus at ≃ 92% after ≃ 10,000 samples. Classification accuracy also increases with the number of readout channels, corresponding to an increase in the number of dynamical features (i.e., 5 × 784 features per digit for 5 channel readouts, the channels are added following the order 1,2,13,15,12) that become sufficiently distinguishable to improve classification. However, as shown in Fig. 3 b, this increase is not linear, with the largest improvements observed from 1 to 2 channels. Figure 3 c shows the confusion matrix for the classification result using 5 readout channels after learning from 50,000 digit samples. The classification results for 8 digits lie within 1.5 σ (where s.d. is σ = 3%) from the average (93.4%). Digit ‘1’ demonstrates significantly higher accuracy since it has a simpler structure, and ‘5’ is an outlier because of the irregular variances of handwriting and low pixel resolution (See Supplementary Fig. S8 for examples of misclassified digits).
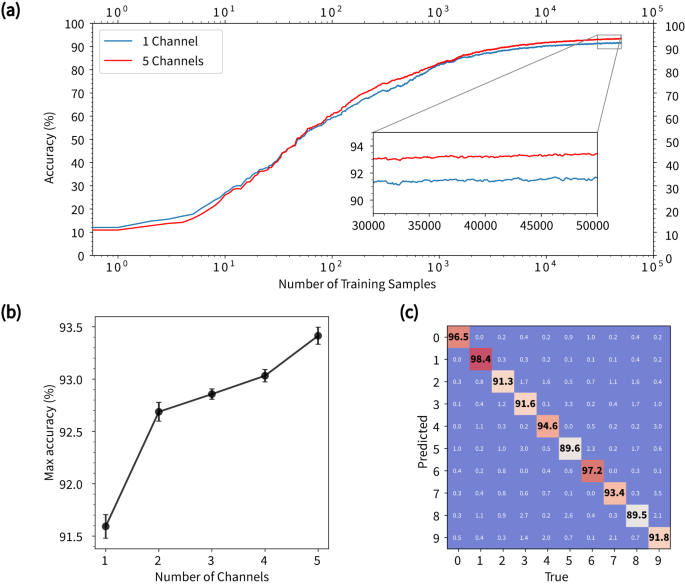
a Testing accuracy as a function of the number of training samples read out from one and five channels. Inset shows a zoom-in of the converged region of the curve. b Maximum testing accuracy achieved after 50,000 training samples with respect to the number of readout channels used by the online linear classifier. Error bars indicate the standard error of the mean of 5 measurements with shuffled training samples. c Confusion matrix for online classification using 5 readout channels.
Mutual information
Mutual information (MI) is an information-theoretic metric that can help uncover the inherent information content within a system and provide a means to assess learning progress during training. Figure 4 a shows the learning curve of the classifier, represented by the mean of the magnitude of the change in the weight matrix, \(\overline{| \Delta {{{{{{{\bf{W}}}}}}}}| }\) , as a function of the number of sample readouts for 5 channels. Learning peaks at ≃ 10 2 − 10 3 samples, after which it declines rapidly and becomes negligible by 10 4 samples. This is reflected in the online classification accuracy (cf. Fig. 3 a), which begins to saturate by ~10 4 samples. The rise and fall of the learning rate profile can be interpreted in terms of maximal dynamical information being extracted by the network. This is indicated by Fig. 4 b, which presents mutual information (MI) between the 10 MNIST digit classes and each of the NWN device readouts used for online classification (cf. Fig. 3 ). The MI values for each channel are calculated by averaging the values across the 784 pixel positions. The coincidence of the saturation in MI with the peak in \(\overline{| \Delta {{{{{{{\bf{W}}}}}}}}| }\) between 10 2 − 10 3 samples demonstrates learning is associated with information dynamics. Note that by ≃ 10 2 samples, the network has received approximately 10 samples for each digit class (on average). It is also noteworthy that MI for the input channel is substantially smaller.
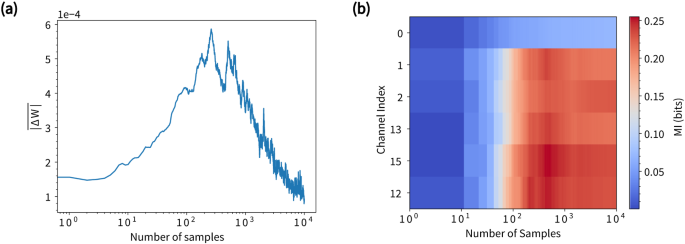
a Mean of the magnitude of changes in the linear weight matrix, \(\overline{| \Delta {{{{{{{\bf{W}}}}}}}}| }\) , as a function of the number of samples learned by the network. b Corresponding Mutual Information (MI) for each of the 5 channels used for online classification (cf. Fig. 3 ) and for input channel 0.
Figure 5 shows MI estimated in a static way, combining all the samples after the whole training dataset is presented to the network. The MI maps are arranged according to the digit classes and averaged within each class. The maps suggest that distinctive information content is extracted when digit samples from different classes are streamed into the network. This is particularly evident when comparing the summed maps for each of the digits (bottom row of Fig. 5 ). Additionally, comparison with the classification confusion matrix shown in Fig. 3 c reveals that the class with the highest total MI value (‘1’) exhibits the highest classification accuracy (98.4%), while the lowest MI classes (‘5’ and ‘8’) exhibit the lowest accuracies (89.6% and 89.5%), although the trend is less evident for intermediate MI values.
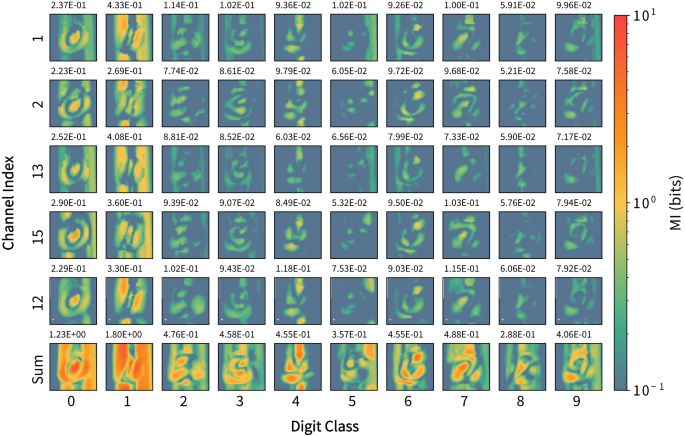
MI maps summed over all channels are shown in the bottom row, and mean MI is shown above each map.
Sequence memory task
As mentioned earlier, RC is most suitable for time-dependent information processing. Here, an RC framework with online learning is used to demonstrate the capacity of NWNs to recall a target digit in a temporal digit sequence constructed from the MNIST database. The sequence memory task is summarised in Fig. 6 . A semi-repetitive sequence of 8 handwritten digits is delivered consecutively into the network in the same way as individual digits were delivered for the MNIST classification task. In addition to readout voltages, the network conductance is calculated from the output current. Using a sliding memory window, the earliest (first) digit is reconstructed from the memory features embedded in the conductance readout of subsequent digits. Figure 6 shows digit ‘7’ reconstructed using the readout features from the network corresponding to the following 3 digits, ‘5’, ‘1’ and ‘4’. See “Methods” for details.
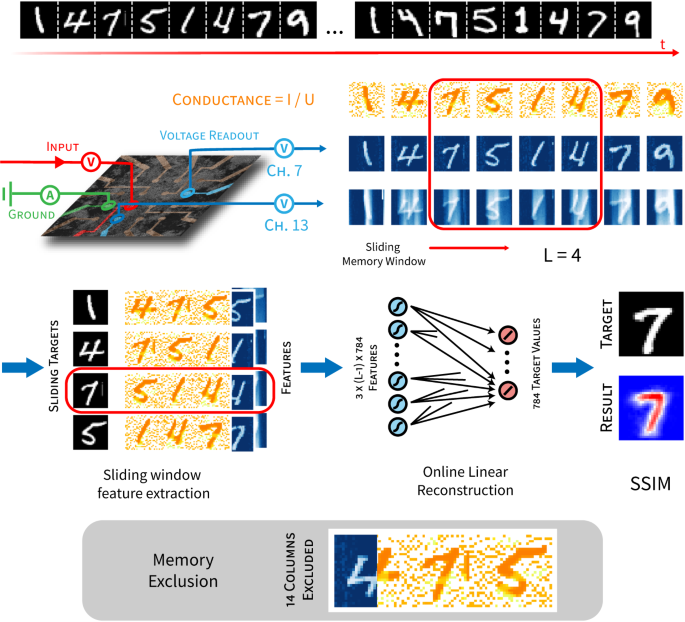
Samples of a semi-repetitive 8-digit sequence (14751479) constructed from the MNIST dataset are temporally streamed into the NWN device through one input channel. A memory window of length L ( L = 4 shown as an example) slides through each digit of the readouts from 2 channels (7 and 13) as well as the network conductance. In each sliding window, the first (earliest) digit is selected for recall as its image is reconstructed from the voltage of one readout channel (channel 7) and memory features embedded in the conductance time series of later L − 1 digits in the memory window. Linear reconstruction weights are trained by the online learning method and reconstruction quality is quantified using the structural similarity index measure (SSIM). The shaded grey box shows an example of the memory exclusion process, in which columns of conductance memory features are replaced by voltage readouts from another channel (channel 13) to demonstrate the memory contribution to image reconstruction (recall) of the target digit.
Figure 7 a shows the network conductance time series and readout voltages for one of the digit sequence samples. The readout voltages exhibit near-instantaneous responses to high pixel intensity inputs, with dynamic ranges that vary distinctively among different channels. The conductance time series also exhibits a large dynamic range (at least 2 orders of magnitude) and, additionally, delayed dynamics. This can be attributed to recurrent loops (i.e., delay lines) in the NWN and to memristive dynamics determined by nano-scale electro-ionic transport. The delay dynamics demonstrate that NWNs retain the memory of previous inputs (see Supplementary Fig. S9 for an example showing the fading memory property of the NWN reservoir). Figure 7 b shows the respective digit images and I − V curves for the sequence sample. The NWN is driven to different internal states as different digits are delivered to the network in sequence. While the dynamics corresponding to digits from the same class show some similar characteristics in the I − V phase space (e.g., digit ‘1’), generally, they exhibit distinctive characteristics due to their sequence position. For example, the first instance of ‘4’ exhibits dynamics that explore more of the phase space than the second instance of ‘4’. This may be attributed to differences in the embedded memory patterns, with the first ‘4’ being preceded by ‘91’ while the second ‘4’ is preceded by ‘51’ and both ‘9’ and ‘5’ have distinctively different phase space characteristics, which are also influenced by their sequence position as well as their uniqueness.
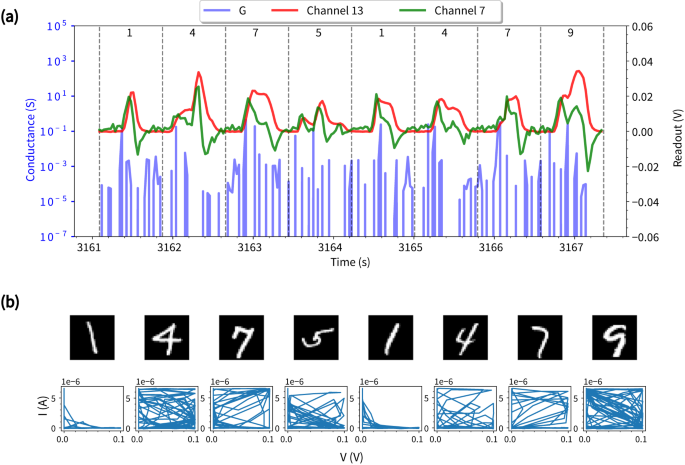
a Conductance time series (G) and readout voltages for one full sequence cycle during the sequence memory task. For better visualisation, voltage readout curves are smoothed by averaging over a moving window of length 0.05 s and values for channel 7 are magnified by ×10. b Corresponding digit images and memory patterns in I − V phase space.
Figure 8 a shows the image reconstruction quality for each digit in the sequence as a function of memory window length. Structural similarity (SSIM) is calculated using a testing group of 500 sets, and the maximum values achieved after learning from 7000 training sets are presented (see Supplementary Fig. S10 for the learning curving for L = 4 and Supplementary Fig. S11 for average SSIM across all digits). The best reconstruction results are achieved for digits ‘1’ and ‘7’, which are repeated digits with relatively simple structures. In contrast, digit ‘4’, which is also repeated, but has a less simple structure, is reconstructed less faithfully. This indicates that the repeat digits produce memory traces that are not completely forgotten before each repetition (i.e., nano-filaments in memristive junctions do not completely decay). On average, the linear reconstructor is able to recall these digits better than the non-repeat digits. For the non-repeat digits (‘5’ and ‘9’), the reconstruction results are more interesting: digit ‘5’ is consistently reconstructed with the lowest SSIM, which correlates with its low classification accuracy (cf. Fig. 3 c), while ‘9’ exhibits a distinctive jump from L = 4 to L = 5 (see also Fig. 8 b). This reflects the contextual information used in the reconstruction: for L = 4, ‘9’ is reconstructed from the sub-sequence ‘147’, which is the same sub-sequence for ‘5’, but for L = 5, ‘9’ is uniquely reconstructed from sub-sequence‘1475’, with a corresponding increase in SSIM. This is not observed for digit ‘5’; upon closer inspection, it appears that the reconstruction of ‘5’ suffers interference from ‘9’ (see Supplementary Fig. S12 ) due to the common sub-sequence ‘147’ and to the larger variance of ‘5’ in the MNIST dataset (which also contributes to its misclassification). A similar jump in SSIM is evident for the repeat digit ‘7’ from L = 2 to L = 3. For L = 3, the first instance of ‘7’ (green curve) is reconstructed from ‘51’, while the second instance (pink curve) is reconstructed from ‘91’, so the jump in SSIM from L = 2 may be attributed to digit ‘7’ leaving more memory traces in digit ‘1’, which has a simpler structure than either ‘9’ or ‘5’.
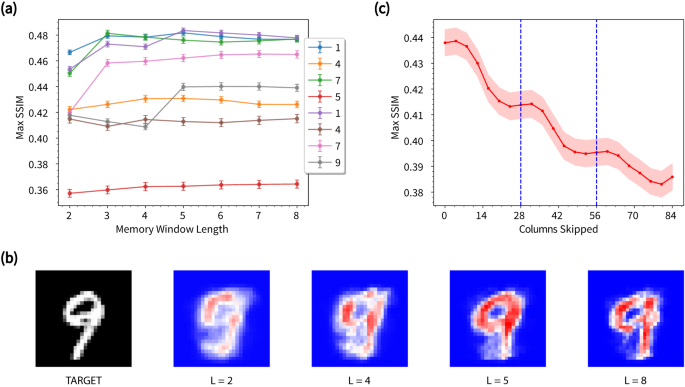
a Maximum SSIM for each digit in the sequence as a function of the memory window length after the network learned from 7000 training sets. The testing set is comprised of 500 sequence samples. Error bars indicate the standard error of the mean across the samples within each digit class. b An example of a target digit from the MNIST dataset and the reconstructed digits using memory windows of different lengths. c Maximum SSIM with respect to the number of columns excluded in the memory feature space. The results are averaged across all testing digits using L = 4, and the standard error of the mean is indicated by the shading. Dashed blue lines indicate when whole digits are excluded.
While the SSIM curves for each individual digit in the sequence increase only gradually with memory window length, their average (shown in Supplementary Fig. S11 ) shows an increase up to L = 5, followed by saturation. This reflects the repetition length of the sequence.
Figure 8 c shows the maximum SSIM, averaged over all reconstructed digits using L = 4 when memory is increasingly excluded from the online reconstruction. SSIM decreases as more columns of conductance features are excluded (replaced with memoryless voltage features). This demonstrates that the memory embedded in the conductance features enhances online learning by the reconstructor. In particular, the maximum SSIM plateaus when ~28 and ~56 columns (corresponding to whole digits) are excluded and decreases significantly when the number of columns excluded is approximately 14, 42 or 70, indicating most of the memory traces are embedded in the central image pixels.
This study is the first to perform the MNIST handwritten digit classification benchmark task using an NWN device. In a previous study, Milano et al. 65 simulated an NWN device and mapped the readouts to a ReRAM cross-point array to perform in materia classification (with a 1-layer neural network) of the MNIST digits, achieving an accuracy of 90.4%. While our experimental implementation is different, readouts from their simulated NWN device also exhibited diverse dynamics and distinct states in response to different digit inputs, similar to that observed in this study. Other studies using memristor cross-bar arrays as physical reservoirs achieved lower MNIST classification accuracies 74 , 75 . In contrast, NWN simulation studies achieved higher classification accuracies of ≃ 98% by either pre-processing the MNIST digits with a convolutional kernel 62 or placing the networks into a deep learning architecture 76 .
In this study, the relatively high classification accuracy achieved with online learning (93.4%) can be largely attributed to the iterative algorithm, which is based on recursive least squares (RLS). Previous RC studies by Jaeger et al. 77 , 78 suggested that RLS converges faster than least mean squares (similar to gradient-based batch methods), which tends to suffer more from numerical roundoff error accumulation, whereas RLS converges in a finite number of steps and uses the remaining training samples for fine-tuning 69 . This is evident in our results showing incremental learning of the weight matrix and is also corroborated by our mutual information analysis. While we performed online classification in an external digital layer, it may be possible to implement the online learning scheme in hardware using, for example, a cross-point array of regular resistors, which exhibit a linear (i.e., Ohmic) response. Such a system would then represent an end-to-end analogue hardware solution for efficient online dynamical learning in edge applications 29 , 79 . An all-analogue RC system was recently demonstrated by Zhong et al. 30 using dynamic resistors as a reservoir and an array of non-volatile memristors in the readout module.
Other studies have exploited the structure of memristor cross-bar arrays to execute matrix–vector multiplication used in conventional machine learning algorithms for MNIST classification, both in experiment 80 , 81 and simulation 82 , 83 , although crosstalk in memristor cross-bars limits the accuracy of classification implemented in this type of hardware 80 .
Beyond physical RC, unconventional physical systems like NWNs could potentially be trained with backpropagation to realise more energy-efficient machine learning than is currently possible with existing software and hardware accelerator approaches 84 . Furthermore, a related study by Loeffler et al. 85 (see also refs. 86 , 87 ) demonstrates how the internal states of NWNs can be controlled by external feedback to harness NWN working memory capacity and enable cognitive tasks to be performed.
Information-theoretic measures like mutual information (MI) have been widely used to assess the intrinsic dynamics in random Boolean networks 88 , 89 , Ising models 90 , and the learning process of echo state networks 91 as well as artificial neural networks (ANNs) 92 . In a previous simulation study 62 , 64 , we found that transfer entropy and active information storage in NWNs reveal that specific parts of the network exhibit richer information dynamics during learning tasks, and we proposed a scheme for optimising task performance accordingly. However, such element-wise calculations are not feasible for physical NWN hardware devices because the number of readouts from the system is limited by the size of the MEA. In this study, we applied a similar approach to that used in Shine et al. 92 to estimate the information content of ANNs at different stages during the MNIST classification task. They found unequal credit assignment, with some image pixels, as well as specific neurons and weights in the ANN, contributing more to learning than others. In our case, by investigating the information content embedded in the NWN readouts, we found that the learning process synchronises with the information provided by the dataset in the temporal domain, while each readout channel provides distinct information about different classes. Interestingly, we also observed some indication of channel preference for a specific digit class, which could potentially be further exploited for channel-wise tuning for other learning tasks.
The sequence memory task introduced in this study is novel and demonstrates both online learning and sequence memory recall from the memory patterns embedded in NWN dynamics. In the brain, memory patterns are linked with network attractor states 93 . The brain’s neural network is able to remember sequence inputs by evolving the internal states to fixed points that define the memory pattern for the sequence 94 . In this study, we also found basins of attraction for the individual digits in the sequence, which allowed us to reconstruct the target digit image as a way of recalling the associated memory pattern. Delayed dynamics similar to that observed in the conductance time series of NWNs were also utilised by Voelker et al. 95 to build spiking recurrent neural networks 96 and implement memory-related tasks. In their studies, the delayed dynamics and memory are implemented in software-based learning algorithms, while NWNs are able to retain memory in hardware due to the memristive junctions and recurrent structure 33 . A similar study by Payvand et al. 97 demonstrated sequence learning using spiking recurrent neural networks implemented in ReRAM to exploit the memory property of this resistive memory hardware. Although their sequence was more repetitive than ours and task performance is measured differently, they demonstrated improved performance when network weights were allowed to self-organise and adapt to changing input, similar to physical NWNs. Future potential applications like natural language processing and image analysis may be envisaged with NWN devices that exploit their capability of learning and memorising dynamic sequences. Future computational applications of NWNs may be realised under new computing paradigms grounded in observations and measurements of physical systems beyond the Turing Machine concept 98 .
In conclusion, we have demonstrated how neuromorphic nanowire network devices can be used to perform tasks in an online manner, learning from the rich spatiotemporal dynamics generated by the physical neural-like network. This is fundamentally different from data-driven statistical machine learning using artificial neural network algorithms. Additionally, our results demonstrate how online learning and recall of streamed sequence patterns are linked to the associated memory patterns embedded in the spatiotemporal dynamics.
Experimental setup
An NWN device, as shown in Fig. 9 , was fabricated and characterised following the procedure developed in our previous studies 34 , 35 , 36 , 49 , 59 . Briefly, a multi-electrode array (MEA) device with 16 electrodes (4x4 grid) was fabricated as the substrate of the device using photolithographically patterned Cr/Ti (5 nm) and Pt (150 nm). Selenium nanowires were first formed by hydrothermal reduction of sodium selenite. Ag 2 Se nanowires were then synthesised by redispersing Se nanowires in a solution of silver nitrate (AgNO 3 ). The resulting nanowire solution was drop-casted over the inner electrodes of the MEA to synthesise the nanowire network (See Supplementary Fig. S1 for SEM images of the NWN without electrodes and Supplementary Fig. S2 for a simulated NWN as well as its corresponding graph representation). A data acquisition device (PXI-6368) was employed to deliver electric signals to the network and simultaneously read out the voltage time series from all electrodes. A source measurement unit (PXI-4141) was used to collect the current time series through the grounded electrode. A switch matrix (TB-2642) was used to modularly route tall signals through the network as desired. All the equipment listed above was from National Instruments and controlled by a custom-made LabView package designed for these applications 49 , 59 , 99 . The readout voltage data exhibited non-uniform phase shifts of 10 − 100Δ t compared to the input stream, so a phase correction method was applied to prepare the readout data for further usage (see details in the following section).
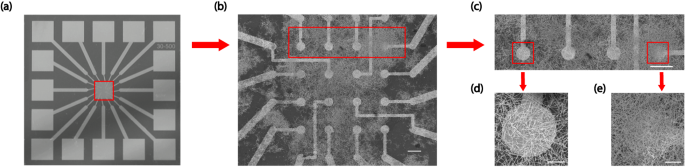
a Optical image of the multi-electrode array. Input/output are enabled by the outer electrodes. b Scanning electron microscopy (SEM) image of the Ag 2 Se Network. 16 inner electrodes are fabricated as a 4 × 4 grid and the nanowires are drop-casted on top of them. Scale bar: 100 μm. c Zoom-in of the SEM image for electrodes 0-3. Scale bar: 100 μm. d Zoom-in for electrode 0. Scale bar: 20 μm. e Zoom-in for electrode 3. Scale bar: 20 μm.
Learning tasks were performed under a reservoir computing (RC) framework 58 , 59 , 62 , 99 . With N digit samples used for training, the respective pixel intensities were normalised to [0.1, 1] V as input voltage values and denoted by U ∈ R N ×784 for future reference. U was then converted to a 1-D temporal voltage pulse stream and delivered to an input channel while another channel was grounded. Each voltage pulse occupied Δ t = 0.001 s in the stream. Voltage features were read simultaneously from M other channels on the device (see Supplementary Fig. S3 for device setups). These temporal readout features were normalised and re-arranged to a 3-D array, V ∈ R N × M ×784 .
The phase of the readout voltage data ( V ) was adjusted per instance in the dataset based on the corresponding input using cross-correlation 100 . For the n -th digit sample, the respective segment in the input pulse stream was denoted as u n ∈ R 784×1 , and the corresponding dynamical features from M readout channels were represented by [ v n ,1 , v n ,2 , … , v n , m ], where v n , m ∈ R 784×1 . The cross-correlation of u n and v n , m is calculated as:
for τ = −783, −782, … 0, … , 783. The phase difference ϕ is determined by:
The 1-D phase adjustment was applied to the readout feature v n , m of the instance based on the phase difference ϕ n , m .
The NWN device readouts embed dynamical features that are linearly separable, so classification can be performed in a linear output layer:
where W is the weight matrix (i.e., classifier), A is the readout feature space and Y contains the sample classes. An online method was implemented based on Greville’s iterative algorithm for computing the pseudoinverse of linear systems 101 . This method is also a special case of the recursive least square (RLS) algorithm 69 , using a uniform sample weighting factor of λ = 1.
The sample feature space was denoted by A = [ a 1 , a 2 , … , a n ], A ∈ R K × N , in which each column ( a n ) represented one sample and every sample was composed of K features ( K = 784 M ). The corresponding classes for each sample were Y = [ y 1 , y 2 , … , y n ], Y ∈ R 10× N . The order of the columns in A and Y are randomly shuffled to smooth the learning curve. During training, a new feature vector of the n -th digit sample a n and its corresponding class vector y n were appended to the right of respective matrices A n and Y n as columns, and the algorithm solved eqn. ( 3 ) for W ( W ∈ R 10× K ) incrementally. The difference between the target y n and the projected result using the previous weight matrix W n −1 was described by:
When ∥ e n ∥ was below a preset threshold \({e}^{{\prime} }=0.1\) , W was updated by:
For the cases when ∥ e n ∥ was above the threshold, an error-correction scheme was applied to optimise the result 68 . In addition, A, Y and θ were initialised at n = 0 by:
with \(\epsilon=\overline{| {{{{{{{\bf{A}}}}}}}}| }\) .
To gain deeper insight into the network’s behaviour and attribute real-time learning to its dynamics, mutual information (MI) between the dynamical features and the corresponding classes was calculated to estimate the information content in a way similar to a previous study on ANNs 92 . All MI results were calculated using the Java Information Dynamics Toolkit (JIDT) 102 . MI was estimated spatially based on the pixel positions from different readout channels and temporally as the feature space expanded when more samples were learned. Among the N digit samples delivered to the network, an ensemble was created using the readout data from channel m at the i -th pixel position: V m , i = [ v 1, m , i , v 2, m , i , … , v N , m , i ], V m , i ∈ R 1× N . Another class vector P ∈ R 1× N was created and mutual information was estimated accordingly by:
where Ω MI stands for the mutual information operator, where the Kraskov estimator was employed 103 .
A 3-D matrix \({{{{{{{\boldsymbol{{{{{{{{\mathcal{M}}}}}}}}}}}}}}}}\in {{{{{{{{\bf{R}}}}}}}}}^{N\times M\times 784}\) was generated after calculating spatial-temporally throughout V . \({{{{{{{\boldsymbol{{{{{{{{\mathcal{M}}}}}}}}}}}}}}}}\) was averaged across the pixel axis (third) to obtain the temporal mutual information per channel. The spatial analysis of mutual information was based on the calculation result for the whole dataset. The class-wise interpretation of \({{{{{{{\boldsymbol{{{{{{{{\mathcal{M}}}}}}}}}}}}}}}}\) was generated by averaging across samples corresponding to each digit class.
A sequence-based memory task was developed to investigate sequence memory and recall. Samples of an 8-digit sequence with a semi-repetitive pattern (14751479) were constructed by randomly sampling the respective digits from the MNIST dataset. Input pixel intensities were normalised to the range [0, 0.1] V, and the samples were streamed into and read out from the NWN in the same way as the classification task, using channels 9, 8 and 7 for input, ground and readout, respectively. In addition to dynamical features from the voltage readouts, memory features were used from the network conductance, calculated pixel-wise by
where \({{{{{{{\boldsymbol{{{{{{{{\mathcal{I}}}}}}}}}}}}}}}}\) is the current leaving the ground channel and U is the input voltage.
To test recall, a digit from the sequence was selected and its image was reconstructed from voltage readouts and memory features in the conductance time series corresponding to digits later in the sequence. A variable memory window of length L ∈ [2, 8] determines the sequence portion used to reconstruct a previous digit image, i.e., from L − 1 subsequent digits. For example, a moving window of length L = 4 reconstructs the first (target) digit from the conductance memory features in the subsequent 3 digits (cf. Fig. 6 ). By placing the target digits and memory features into ensembles, a dataset of 7000 training samples and 500 testing samples was composed using the sliding windows.
To reconstruct each target digit image, the same linear online learning algorithm used for MNIST classification was applied. In this case, Y in eqn. ( 3 ) was composed as Y = [ y 1 , y 2 , . . . , y n ], with Y ∈ R 784× N , and softmax in eqn. ( 4 ) was no longer used. Structural similarity index measure (SSIM) 104 was employed to quantify the reconstruction quality.
To further test that image reconstruction exploits memory features and not just dynamical features associated with the spatial pattern of the sequence (i.e., sequence classification), a memory exclusion test was developed as follows. The conductance features corresponding to a specified number of columns of inputs were replaced by voltage features from channel 13 (voltages are adjusted to the same scale as conductance) so that the memory in conductance is excluded without losing the non-linear features in the readout data (cf. Fig. 6 ). The target digit was then reconstructed for a varying number of columns with memory exclusion.
Data availability
The raw NWN measurement data used in this study are available in the Zenodo database https://zenodo.org/record/7662887 .
Code availability
The code used in this study is available in the Zenodo database https://zenodo.org/record/7662887 .
Mead, C. Neuromorphic electronic systems. Proc. IEEE 78 , 1629–1636 (1990).
Article Google Scholar
Indiveri, G. et al. Neuromorphic silicon neuron circuits. Front. Neurosci. 5 , 73 (2011).
Schuman, C. D. et al. A survey of neuromorphic computing and neural networks in hardware. Preprint at https://arxiv.org/abs/1705.06963 (2017).
Ielmini, D. & Wong, H.-S. P. In-memory computing with resistive switching devices. Nat. Electron. 1 , 333–343 (2018).
Kendall, J. D. & Kumar, S. The building blocks of a brain-inspired computer. Appl. Phys. Rev. 7 , 011305 (2020).
Article ADS CAS Google Scholar
Mehonic, A. et al. Memristors—from in-memory computing, deep learning acceleration, and spiking neural networks to the future of neuromorphic and bio-inspired computing. Adv. Intell. Syst. 2 , 2000085 (2020).
Sebastian, A., Le Gallo, M., Khaddam-Aljameh, R. & Eleftheriou, E. Memory devices and applications for in-memory computing. Nat. Nanotechnol. 15 , 529–544 (2020).
Article ADS CAS PubMed Google Scholar
Zhang, W. et al. Neuro-inspired computing chips. Nat. Electron. 3 , 371–382 (2020).
Article ADS Google Scholar
Zhu, J., Zhang, T., Yang, Y. & Huang, R. A comprehensive review on emerging artificial neuromorphic devices. Appl. Phys. Rev. 7 , 011312 (2020).
Mehonic, A. & Kenyon, A. J. Brain-inspired computing needs a master plan. Nature 604 , 255–260 (2022).
Christensen, D. V. et al. 2022 Roadmap on neuromorphic computing and engineering. Neuromorphic Comput. Eng. 2 , 022501 (2022).
Pfeil, T. et al. Six networks on a universal neuromorphic computing substrate. Front. Neurosci. 7 , 11 (2013).
Merolla, P. A. et al. A million spiking-neuron integrated circuit with a scalable communication network and interface. Science 345 , 668–673 (2014).
Thakur, C. S. et al. Large-scale neuromorphic spiking array processors: a quest to mimic the brain. Front. Neurosci. 12 , 891 (2018).
Bouvier, M. et al. Spiking neural networks hardware implementations and challenges: a survey. ACM J. Emerg. Technol. Comput. Syst. 15 , 22:1–22:35 (2019).
Roy, K., Jaiswal, A. & Panda, P. Towards spike-based machine intelligence with neuromorphic computing. Nature 575 , 607–617 (2019).
Eshraghian, J. K., Wang, X. & Lu, W. D. Memristor-based binarized spiking neural networks: challenges and applications. IEEE Nanotechnol. Mag. 16 , 14–23 (2022).
Bose, S. K. et al. Evolution of a designless nanoparticle network into reconfigurable boolean logic. Nat. Nanotechnol. 10 , 1048–1052 (2015).
Grollier, J. et al. Neuromorphic spintronics. Nat. Electron. 3 , 360–370 (2020).
Sangwan, V. K. & Hersam, M. C. Neuromorphic nanoelectronic materials. Nat. Nanotechnol. 15 , 517–528 (2020).
Tanaka, H. et al. In-materio computing in random networks of carbon nanotubes complexed with chemically dynamic molecules: a review. Neuromorphic Comput. Eng. 2 , 022002 (2022).
Kuncic, Z., Nakayama, T. & Gimzewski, J. Focus on disordered, self-assembled neuromorphic systems. Neuromorphic Comput. Eng. 2 , 040201 (2022).
Waser, R. & Aono, M. Nanoionics-based resistive switching memories. Nat. Mater. 6 , 833–840 (2007).
Ohno, T. et al. Short-term plasticity and long-term potentiation mimicked in single inorganic synapses. Nat. Mater. 10 , 591–595 (2011).
Yang, J. J., Strukov, D. B. & Stewart, D. R. Memristive devices for computing. Nat. Nanotechnol. 8 , 13–24 (2013).
La Barbera, S., Vuillaume, D. & Alibart, F. Filamentary switching: synaptic plasticity through device volatility. ACS Nano 9 , 941–949 (2015).
Article PubMed Google Scholar
Wang, Z. et al. Resistive switching materials for information processing. Nat. Rev. Mater. 5 , 173–195 (2020).
Diaz Schneider, J. I. et al. Resistive switching of self-assembled silver nanowire networks governed by environmental conditions. Adv. Electron. Mater. 8 , 2200631 (2022).
Article CAS Google Scholar
Kumar, P. et al. Hybrid architecture based on two-dimensional memristor crossbar array and CMOS integrated circuit for edge computing. NPJ 2D Mater. Appl. 6 , 1–10 (2022).
Zhong, Y. et al. A memristor-based analogue reservoir computing system for real-time and power-efficient signal processing. Nat. Electron. 5 , 672–681 (2022).
Kotooka, T., Tanaka, Y., Tamukoh, H., Usami, Y. & Tanaka, H. Random network device fabricated using Ag 2 Se nanowires for data augmentation with binarized convolutional neural network. Appl. Phys. Express 16 , 014002 (2023).
Milano, G., Porro, S., Valov, I. & Ricciardi, C. Recent developments and perspectives for memristive devices based on metal oxide nanowires. Adv. Electron. Mater. 5 , 1800909 (2019).
Kuncic, Z. & Nakayama, T. Neuromorphic nanowire networks: principles, progress and future prospects for neuro-inspired information processing. Adv. Phys. X 6 , 1894234 (2021).
Google Scholar
Stieg, A. Z. et al. Emergent criticality in complex turing B-type atomic switch networks. Adv. Mater. 24 , 286–293 (2012).
Article CAS PubMed Google Scholar
Avizienis, A. V. et al. Neuromorphic atomic switch networks. PLoS ONE 7 , e42772 (2012).
Article ADS CAS PubMed PubMed Central Google Scholar
Demis, E. C. et al. Atomic switch networks—nanoarchitectonic design of a complex system for natural computing. Nanotechnology 26 , 204003 (2015).
Diaz-Alvarez, A. et al. Emergent dynamics of neuromorphic nanowire networks. Sci. Rep. 9 , 14920 (2019).
Article ADS PubMed PubMed Central Google Scholar
Loeffler, A. et al. Topological properties of neuromorphic nanowire networks. Front. Neurosci. 14 , 184 (2020).
Article PubMed PubMed Central Google Scholar
Terabe, K., Nakayama, T., Hasegawa, T. & Aono, M. Formation and disappearance of a nanoscale silver cluster realized by solid electrochemical reaction. J. Appl. Phys. 91 , 10110–10114 (2002).
Terabe, K., Hasegawa, T., Nakayama, T. & Aono, M. Quantized conductance atomic switch. Nature 433 , 47–50 (2005).
Schoen, D. T., Xie, C. & Cui, Y. Electrical switching and phase transformation in silver selenide nanowires. J. Am. Chem. Soc. 129 , 4116–4117 (2007).
Menzel, S., Tappertzhofen, S., Waser, R. & Valov, I. Switching kinetics of electrochemical metallization memory cells. Phys. Chem. Chem. Phys. 15 , 6945 (2013).
Kozicki, M. N., Mitkova, M. & Valov, I. Electrochemical metallization memories. Resistive Switching , Ch. 17, 483–514 (Wiley, 2016).
Bellew, A. T., Manning, H. G., Gomes da Rocha, C., Ferreira, M. S. & Boland, J. J. Resistance of single Ag nanowire junctions and their role in the conductivity of nanowire networks. ACS Nano 9 , 11422–11429 (2015).
Manning, H. G. et al. Emergence of winner-takes-all connectivity paths in random nanowire networks. Nat. Commun. 9 , 3219 (2018).
Milano, G. et al. Brain-inspired structural plasticity through reweighting and rewiring in multi-terminal self-organizing memristive nanowire networks. Adv. Intell. Syst. 2 , 2000096 (2020).
Mallinson, J. B. et al. Avalanches and criticality in self-organized nanoscale networks. Sci. Adv. 5 , eaaw8438 (2019).
Hochstetter, J. et al. Avalanches and edge-of-chaos learning in neuromorphic nanowire networks. Nat. Commun. 12 , 4008 (2021).
Dunham, C. S. et al. Nanoscale neuromorphic networks and criticality: a perspective. J. Phys. Complex. 2 , 042001 (2021).
Milano, G., Cultrera, A., Boarino, L., Callegaro, L. & Ricciardi, C. Tomography of memory engrams in self-organizing nanowire connectomes. Nat. Commun. 14 , 5723 (2023).
Medsker, L. & Jain, L. C. Recurrent Neural Networks: Design and Applications (CRC Press, 1999).
Lipton, Z. C., Berkowitz, J. & Elkan, C. A critical review of recurrent neural networks for sequence learning. Preprint at https://arxiv.org/abs/1506.00019 (2015).
Shen, S. et al. Reservoir transformers. Preprint at https://arxiv.org/abs/2012.15045 (2020).
Lukoševičius, M. & Jaeger, H. Reservoir computing approaches to recurrent neural network training. Comput. Sci. Rev. 3 , 127–149 (2009).
Article MATH Google Scholar
Klos, C., Kalle Kossio, Y. F., Goedeke, S., Gilra, A. & Memmesheimer, R.-M. Dynamical learning of dynamics. Phys. Rev. Lett. 125 , 088103 (2020).
Gauthier, D. J., Bollt, E., Griffith, A. & Barbosa, W. A. S. Next generation reservoir computing. Nat. Commun. 12 , 5564 (2021).
Tanaka, G. et al. Recent advances in physical reservoir computing: a review. Neural Netw. 115 , 100–123 (2019).
Sillin, H. O. et al. A theoretical and experimental study of neuromorphic atomic switch networks for reservoir computing. Nanotechnology 24 , 384004 (2013).
Lilak, S. et al. Spoken digit classification by in-materio reservoir computing with neuromorphic atomic switch networks. Front. Nanotechnol. 3 , 675792 (2021).
Fu, K. et al. Reservoir computing with neuromemristive nanowire networks. In Proc. 2020 International Joint Conference on Neural Networks (IJCNN) , 1–8 (2020).
Zhu, R. et al. Harnessing adaptive dynamics in neuro-memristive nanowire networks for transfer learning. In Proc. 2020 International Conference on Rebooting Computing (ICRC) , 102–106 (2020).
Zhu, R. et al. MNIST classification using neuromorphic nanowire networks. In Proc. International Conference on Neuromorphic Systems 2021 (ICONS 2021) , 1–4 (Association for Computing Machinery, 2021).
Loeffler, A. et al. Modularity and multitasking in neuro-memristive reservoir networks. Neuromorphic Comput. Eng. 1 , 014003 (2021).
Zhu, R. et al. Information dynamics in neuromorphic nanowire networks. Sci. Rep. 11 , 13047 (2021).
Milano, G. et al. In materia reservoir computing with a fully memristive architecture based on self-organizing nanowire networks. Nat. Mater. 21 , 195–202 (2022).
Cucchi, M., Abreu, S., Ciccone, G., Brunner, D. & Kleemann, H. Hands-on reservoir computing: a tutorial for practical implementation. Neuromorphic Comput. Eng. 2 , 032002 (2022).
Hoi, S. C. H., Sahoo, D., Lu, J. & Zhao, P. Online learning: a comprehensive survey. Neurocomputing 459 , 249–289 (2021).
Tapson, J. & van Schaik, A. Learning the pseudoinverse solution to network weights. Neural Netw. 45 , 94–100 (2013).
Article CAS PubMed MATH Google Scholar
Farhang-Boroujeny, B. Adaptive Filters: Theory and Applications 2nd edn (Wiley, 2013).
Fontenla-Romero, Ó., Guijarro-Berdiñas, B., Martinez-Rego, D., Pérez-Sánchez, B. & Peteiro-Barral, D. Online machine learning. Efficiency and Scalability Methods for Computational Intellect , 27–54 (IGI Global, 2013).
Gomes, H. M., Read, J., Bifet, A., Barddal, J. P. & Gama, J. Machine learning for streaming data: state of the art, challenges, and opportunities. ACM SIGKDD Explor. Newslett. 21 , 6–22 (2019).
Kudithipudi, D. et al. Biological underpinnings for lifelong learning machines. Nat. Mach. Intell. 4 , 196–210 (2022).
Lecun, Y., Bottou, L., Bengio, Y. & Haffner, P. Gradient-based learning applied to document recognition. Proc. IEEE 86 , 2278–2324 (1998).
Du, C. et al. Reservoir computing using dynamic memristors for temporal information processing. Nat. Commun. 8 , 2204 (2017).
Midya, R. et al. Reservoir computing using diffusive memristors. Adv. Intell. Syst. 1 , 1900084 (2019).
Kendall, J. D., Pantone, R. D. & Nino, J. C. Deep learning in memristive nanowire networks. Preprint at https://arxiv.org/abs/2003.02642 (2020).
Jaeger, H. The “Echo State” Approach to Analysing and Training Recurrent Neural Networks—With an Erratum Note . GMD Technical Report 148 (German National Research Center for Information Technology, 2001).
Jaeger, H. Adaptive nonlinear system identification with echo state networks. In Proc. Neural Inf. Process. Syst. (NIPS 2002) , 609–616 (2003).
Alaba, P. A. et al. Towards a more efficient and cost-sensitive extreme learning machine: a state-of-the-art review of recent trend. Neurocomputing 350 , 70–90 (2019).
Choi, S. et al. A self-rectifying TaOy/nanoporous TaOx memristor synaptic array for learning and energy-efficient neuromorphic systems. NPG Asia Mater. 10 , 1097–1106 (2018).
Yao, P. et al. Fully hardware-implemented memristor convolutional neural network. Nature 577 , 641–646 (2020).
Liu, X. & Zeng, Z. Memristor crossbar architectures for implementing deep neural networks. Complex & Intell. Syst. 8 , 787–802 (2022).
Mao, R., Wen, B., Jiang, M., Chen, J. & Li, C. Experimentally-validated crossbar model for defect-aware training of neural networks. IEEE Trans. Circuits Syst. II Express Briefs 69 , 2468–2472 (2022).
Wright, L. G. et al. Deep physical neural networks trained with backpropagation. Nature 601 , 549–555 (2022).
Loeffler, A. et al. Neuromorphic learning, working memory, and metaplasticity in nanowire networks. Sci. Adv. 9 , eadg3289 (2023).
Diaz-Alvarez, A., Higuchi, R., Li, Q., Shingaya, Y. & Nakayama, T. Associative routing through neuromorphic nanowire networks. AIP Adv. 10 , 025134 (2020).
Li, Q. et al. Dynamic electrical pathway tuning in neuromorphic nanowire networks. Adv. Funct. Mater. 30 , 2003679 (2020).
Lizier, J., Prokopenko, M. & Zomaya, A. The information dynamics of phase transitions in random boolean networks. In Proc. Eleventh International Conference on the Simulation and Synthesis of Living Systems (ALife XI) (2008).
Lizier, J. T., Pritam, S. & Prokopenko, M. Information dynamics in small-world boolean networks. Artif. Life 17 , 293–314 (2011).
Barnett, L., Lizier, J. T., Harré, M., Seth, A. K. & Bossomaier, T. Information flow in a kinetic Ising model peaks in the disordered phase. Phys. Rev. Lett. 111 , 177203 (2013).
Article ADS PubMed Google Scholar
Boedecker, J., Obst, O., Lizier, J. T., Mayer, N. M. & Asada, M. Information processing in echo state networks at the edge of chaos. Theory Biosci. 131 , 205–213 (2012).
Shine, J. M., Li, M., Koyejo, O., Fulcher, B. & Lizier, J. T. Nonlinear reconfiguration of network edges, topology and information content during an artificial learning task. Brain Inform . 8 , 26 (2021).
Khona, M. & Fiete, I. R. Attractor and integrator networks in the brain. Nat. Rev. Neurosci. 23 , 744–766 (2022).
Dayan, P. & Abbott, L. F. Theoretical Neuroscience: Computational and Mathematical Modeling of Neural Systems (Massachusetts Institute of Technology Press, 2001).
Voelker, A., Kajić, I. & Eliasmith, C. Legendre memory units: continuous-time representation in recurrent neural networks. In Proc. 33rd Conference on Neural Information Processing Systems (NeurIPS 2019) ( 2019).
Voelker, A. R. & Eliasmith, C. Improving spiking dynamical networks: accurate delays, higher-order synapses, and time cells. Neural Comput. 30 , 569–609 (2018).
Article MathSciNet PubMed MATH Google Scholar
Payvand, M. et al. Self-organization of an inhomogeneous memristive hardware for sequence learning. Nat. Commun. 13 , 5793 (2022).
Jaeger, H., Noheda, B. & van der Wiel, W. G. Toward a formal theory for computing machines made out of whatever physics offers. Nat. Commun. 14 , 4911 (2023).
Kotooka, T. et al. Ag 2 Se nanowire network as an effective in-materio reservoir computing device. Preprint at ResearchSquare https://doi.org/10.21203/rs.3.rs-322405/v1 (2021).
Ianniello, J. Time delay estimation via cross-correlation in the presence of large estimation errors. IEEE Trans. Acoust. Speech Signal Process. 30 , 998–1003 (1982).
Greville, T. N. E. Some applications of the pseudoinverse of a matrix. SIAM Rev. 2 , 15–22 (1960).
Article ADS MathSciNet MATH Google Scholar
Lizier, J. T. JIDT: an information-theoretic toolkit for studying the dynamics of complex systems. Front. Robot. AI 1 , 11 (2014).
Kraskov, A., Stögbauer, H. & Grassberger, P. Estimating mutual information. Phys. Rev. E 69 , 066138 (2004).
Article ADS MathSciNet Google Scholar
Wang, Z., Bovik, A., Sheikh, H. & Simoncelli, E. Image quality assessment: from error visibility to structural similarity. IEEE Trans. Image Process. 13 , 600–612 (2004).
Download references
Acknowledgements
The authors wish to thank members of the UCLA Nanofabrication Laboratory and the California NanoSystems Institute (CNSI) Nano and Pico Characterization Lab (NPC) for their support of this project. The authors also acknowledge the use of the Artemis High-Performance Computing resource at the Sydney Informatics Hub, a Core Research Facility of the University of Sydney. R.Z. is supported by a Postgraduate Research Excellence Award scholarship from the University of Sydney. A.L. is supported by a Research Training Program scholarship from the University of Sydney. Z.K. acknowledges support from the Australian-American Fulbright Commission.
Author information
These authors contributed equally: Ruomin Zhu, Sam Lilak.
Authors and Affiliations
School of Physics, The University of Sydney, Sydney, NSW, Australia
Ruomin Zhu, Alon Loeffler & Zdenka Kuncic
Department of Chemistry and Biochemistry, University of California, Los Angeles, Los Angeles, CA, US
Sam Lilak & James Gimzewski
School of Computer Science, The University of Sydney, Sydney, NSW, Australia
Joseph Lizier
Centre for Complex Systems, The University of Sydney, Sydney, NSW, Australia
Joseph Lizier & Zdenka Kuncic
California NanoSystems Institute, University of California, Los Angeles, Los Angeles, CA, US
Adam Stieg & James Gimzewski
WPI Center for Materials Nanoarchitectonics (MANA), National Institute for Materials Science (NIMS), Tsukuba, Japan
Research Center for Neuromorphic AI Hardware, Kyutech, Kitakyushu, Japan
James Gimzewski
The University of Sydney Nano Institute, Sydney, NSW, Australia
Zdenka Kuncic
You can also search for this author in PubMed Google Scholar
Contributions
R.Z. and Z.K. conceived and designed the study. S.L., A.Z.S. and J.G. fabricated the device. S.L. performed the experiments with guidance from R.Z., A.Z.S., J.G. and Z.K. R.Z., A.L., J.L. and Z.K. analysed the data. R.Z. wrote the manuscript with consultation from the other authors. Z.K. supervised the project.
Corresponding authors
Correspondence to Ruomin Zhu , Adam Stieg , James Gimzewski or Zdenka Kuncic .

Ethics declarations
Competing interests.
Z.K., A.Z.S. and J.G. are with Emergentia, Inc. The authors declare no other competing interests.
Peer review
Peer review information.
Nature Communications thanks the anonymous reviewers for their contribution to the peer review of this work. A peer review file is available.
Additional information
Publisher’s note Springer Nature remains neutral with regard to jurisdictional claims in published maps and institutional affiliations.
Supplementary information
Supplementary information, peer review file, rights and permissions.
Open Access This article is licensed under a Creative Commons Attribution 4.0 International License, which permits use, sharing, adaptation, distribution and reproduction in any medium or format, as long as you give appropriate credit to the original author(s) and the source, provide a link to the Creative Commons licence, and indicate if changes were made. The images or other third party material in this article are included in the article’s Creative Commons licence, unless indicated otherwise in a credit line to the material. If material is not included in the article’s Creative Commons licence and your intended use is not permitted by statutory regulation or exceeds the permitted use, you will need to obtain permission directly from the copyright holder. To view a copy of this licence, visit http://creativecommons.org/licenses/by/4.0/ .
Reprints and permissions
About this article
Cite this article.
Zhu, R., Lilak, S., Loeffler, A. et al. Online dynamical learning and sequence memory with neuromorphic nanowire networks. Nat Commun 14 , 6697 (2023). https://doi.org/10.1038/s41467-023-42470-5
Download citation
Received : 13 March 2023
Accepted : 11 October 2023
Published : 01 November 2023
DOI : https://doi.org/10.1038/s41467-023-42470-5
Share this article
Anyone you share the following link with will be able to read this content:
Sorry, a shareable link is not currently available for this article.
Provided by the Springer Nature SharedIt content-sharing initiative
Quick links
- Explore articles by subject
- Guide to authors
- Editorial policies
Sign up for the Nature Briefing newsletter — what matters in science, free to your inbox daily.

Main Navigation
- Contact ICML
- Code of Conduct
- Create Profile
- Journal To Conference Track
- Diversity & Inclusion
- Privacy Policy
- Future Meetings
Oral A2 Computer Vision and Efficient ML
Meeting room 313.
Moderator: Jonathon Shlens
Raising the Cost of Malicious AI-Powered Image Editing
Hadi Salman · Alaa Khaddaj · Guillaume Leclerc · Andrew Ilyas · Aleksander Madry
We present an approach to mitigating the risks of malicious image editing posed by large diffusion models. The key idea is to immunize images so as to make them resistant to manipulation by these models. This immunization relies on injection of imperceptible adversarial perturbations designed to disrupt the operation of the targeted diffusion models, forcing them to generate unrealistic images. We provide two methods for crafting such perturbations, and then demonstrate their efficacy. Finally, we discuss a policy component necessary to make our approach fully effective and practical---one that involves the organizations developing diffusion models, rather than individual users, to implement (and support) the immunization process.
Dynamics-inspired Neuromorphic Visual Representation Learning
Zhengqi Pei · Shuhui Wang
This paper investigates the dynamics-inspired neuromorphic architecture for visual representation learning following Hamilton's principle. Our method converts weight-based neural structure to its dynamics-based form that consists of finite sub-models, whose mutual relations measured by computing path integrals amongst their dynamical states are equivalent to the typical neural weights. Based on the entropy reduction process derived from the Euler-Lagrange equations, the feedback signals interpreted as stress forces amongst sub-models push them to move. We first train a dynamics-based neural model from scratch and observe that this model outperforms traditional neural models on MNIST. We then convert several pre-trained neural structures into dynamics-based forms, followed by fine-tuning via entropy reduction to obtain the stabilized dynamical states. We observe consistent improvements in these transformed models over their weight-based counterparts on ImageNet and WebVision in terms of computational complexity, parameter size, testing accuracy, and robustness. Besides, we show the correlation between model performance and structural entropy, providing deeper insight into weight-free neuromorphic learning.
Scaling Vision Transformers to 22 Billion Parameters
Mostafa Dehghani · Josip Djolonga · Basil Mustafa · Piotr Padlewski · Jonathan Heek · Justin Gilmer · Andreas Steiner · Mathilde Caron · Robert Geirhos · Ibrahim Alabdulmohsin · Rodolphe Jenatton · Lucas Beyer · Michael Tschannen · Anurag Arnab · Xiao Wang · Carlos Riquelme · Matthias Minderer · Joan Puigcerver · Utku Evci · Manoj Kumar · Sjoerd van Steenkiste · Gamaleldin Elsayed · Aravindh Mahendran · Fisher Yu · Avital Oliver · Fantine Huot · Jasmijn Bastings · Mark Collier · Alexey Gritsenko · Vighnesh N Birodkar · Cristina Vasconcelos · Yi Tay · Thomas Mensink · Alexander Kolesnikov · Filip Pavetic · Dustin Tran · Thomas Kipf · Mario Lucic · Xiaohua Zhai · Daniel Keysers · Jeremiah Harmsen · Neil Houlsby
The scaling of Transformers has driven breakthrough capabilities for language models. At present, the largest large language models (LLMs) contain upwards of 100B parameters. Vision Transformers (ViT) have introduced the same architecture to image and video modelling, but these have not yet been successfully scaled to nearly the same degree; the largest dense ViT contains 4B parameters (Chen et al., 2022). We present a recipe for highly efficient and stable training of a 22B-parameter ViT (ViT-22B) and perform a wide variety of experiments on the resulting model. When evaluated on downstream tasks (often with a lightweight linear model on frozen features), ViT-22B demonstrates increasing performance with scale. We further observe other interesting benefits of scale, including an improved tradeoff between fairness and performance, state-of-the-art alignment to human visual perception in terms of shape/texture bias, and improved robustness. ViT-22B demonstrates the potential for "LLM-like" scaling in vision, and provides key steps towards getting there.
Facial Expression Recognition with Adaptive Frame Rate based on Multiple Testing Correction
Andrey Savchenko
In this paper, we consider the problem of the high computational complexity of video-based facial expression recognition. A novel sequential procedure is proposed with an adaptive frame rate selection in a short video fragment to speed up decision-making. We automatically adjust the frame rate and process fewer frames with a low frame rate for more straightforward videos and more frames for complex ones. To determine the frame rate at which an inference is sufficiently reliable, the Benjamini-Hochberg procedure from multiple comparisons theory is employed to control the false discovery rate. The main advantages of our method are an improvement of the trustworthiness of decision-making by maintaining only one hyper-parameter (false acceptance rate) and its applicability with arbitrary neural network models used as facial feature extractors without the need to re-train these models. An experimental study on datasets from ABAW and EmotiW challenges proves the superior performance (1.5-40 times faster) of the proposed approach compared to processing all frames and existing techniques with early exiting and adaptive frame selection.
Fourmer: An Efficient Global Modeling Paradigm for Image Restoration
Man Zhou · Jie Huang · Chunle Guo · Chongyi Li
Global modeling-based image restoration frameworks have become popular. However, they often require a high memory footprint and do not consider task-specific degradation. Our work presents an alternative approach to global modeling that is more efficient for image restoration. The key insights which motivate our study are two-fold: 1) Fourier transform is capable of disentangling image degradation and content component to a certain extent, serving as the image degradation prior, and 2) Fourier domain innately embraces global properties, where each pixel in the Fourier space is involved with all spatial pixels. While adhering to the ``spatial interaction + channel evolution'' rule of previous studies, we customize the core designs with Fourier spatial interaction modeling and Fourier channel evolution. Our paradigm, Fourmer, achieves competitive performance on common image restoration tasks such as image de-raining, image enhancement, image dehazing, and guided image super-resolution, while requiring fewer computational resources. The code for Fourmer will be made publicly available.
Learning Signed Distance Functions from Noisy 3D Point Clouds via Noise to Noise Mapping
Baorui Ma · Yushen Liu · Zhizhong Han
Learning signed distance functions (SDFs) from 3D point clouds is an important task in 3D computer vision. However, without ground truth signed distances, point normals or clean point clouds, current methods still struggle from learning SDFs from noisy point clouds. To overcome this challenge, we propose to learn SDFs via a noise to noise mapping, which does not require any clean point cloud or ground truth supervision for training. Our novelty lies in the noise to noise mapping which can infer a highly accurate SDF of a single object or scene from its multiple or even single noisy point cloud observations. Our novel learning manner is supported by modern Lidar systems which capture multiple noisy observations per second. We achieve this by a novel loss which enables statistical reasoning on point clouds and maintains geometric consistency although point clouds are irregular, unordered and have no point correspondence among noisy observations. Our evaluation under the widely used benchmarks demonstrates our superiority over the state-of-the-art methods in surface reconstruction, point cloud denoising and upsampling. Our code, data, and pre-trained models are available at https://github.com/mabaorui/Noise2NoiseMapping/ .
Hiera: A Hierarchical Vision Transformer without the Bells-and-Whistles
Chaitanya Ryali · Yuan-Ting Hu · Daniel Bolya · Chen Wei · Haoqi Fan · Po-Yao Huang · Vaibhav Aggarwal · Arkabandhu Chowdhury · Omid Poursaeed · Judy Hoffman · Jitendra Malik · Yanghao Li · Christoph Feichtenhofer
Modern hierarchical vision transformers have added several vision-specific components in the pursuit of supervised classification performance. While these components lead to effective accuracies and attractive FLOP counts, the added complexity actually makes these transformers slower than their vanilla ViT counterparts. In this paper, we argue that this additional bulk is unnecessary. By pretraining with a strong visual pretext task (MAE), we can strip out all the bells-and-whistles from a state-of-the-art multi-stage vision transformer without losing accuracy. In the process, we create Hiera, an extremely simple hierarchical vision transformer that is more accurate than previous models while being significantly faster both at inference and during training. We evaluate Hiera on a variety of tasks for image and video recognition. Our code and models are available at https://github.com/facebookresearch/hiera.
Rockmate: an Efficient, Fast, Automatic and Generic Tool for Re-materialization in PyTorch
Xunyi Zhao · Théotime Le Hellard · Lionel Eyraud-Dubois · Julia Gusak · Olivier Beaumont
We propose Rockmate to control the memory requirements when training PyTorch DNN models. Rockmate is an automatic tool that starts from the model code and generates an equivalent model, using a predefined amount of memory for activations, at the cost of a few re-computations. Rockmate automatically detects the structure of computational and data dependencies and rewrites the initial model as a sequence of complex blocks. We show that such a structure is widespread and can be found in many models in the literature (Transformer based models, ResNet, RegNets,...). This structure allows us to solve the problem in a fast and efficient way, using an adaptation of Checkmate (too slow on the whole model but general) at the level of individual blocks and an adaptation of Rotor (fast but limited to sequential models) at the level of the sequence itself. We show through experiments on many models that Rockmate is as fast as Rotor and as efficient as Checkmate, and that it allows in many cases to obtain a significantly lower memory consumption for activations (by a factor of 2 to 5) for a rather negligible overhead (of the order of 10% to 20%). Rockmate is open source and available at https://github.com/topal-team/rockmate.
SparseProp: Efficient Sparse Backpropagation for Faster Training of Neural Networks at the Edge
Mahdi Nikdan · Tommaso Pegolotti · Eugenia Iofinova · Eldar Kurtic · Dan Alistarh
We provide an efficient implementation of the backpropagation algorithm, specialized to the case where the weights of the neural network being trained are sparse . Our algorithm is general, as it applies to arbitrary (unstructured) sparsity and common layer types (e.g., convolutional or linear). We provide a fast vectorized implementation on commodity CPUs, and show that it can yield speedups in end-to-end runtime experiments, both in transfer learning using already-sparsified networks, and in training sparse networks from scratch. Thus, our results provide the first support for sparse training on commodity hardware.
Fast Inference from Transformers via Speculative Decoding
Yaniv Leviathan · Matan Kalman · Yossi Matias
Inference from large autoregressive models like Transformers is slow - decoding K tokens takes K serial runs of the model. In this work we introduce speculative decoding - an algorithm to sample from autoregressive models faster without any changes to the outputs, by computing several tokens in parallel. At the heart of our approach lie the observations that (1) hard language-modeling tasks often include easier subtasks that can be approximated well by more efficient models, and (2) using speculative execution and a novel sampling method, we can make exact decoding from the large models faster, by running them in parallel on the outputs of the approximation models, potentially generating several tokens concurrently, and without changing the distribution. Our method can accelerate existing off-the-shelf models without retraining or architecture changes. We demonstrate it on T5-XXL and show a 2X-3X acceleration compared to the standard T5X implementation, with identical outputs.

IMAGES
VIDEO
COMMENTS
TL;DR: We build a dynamics-inspired neural mechanism that outperform the weight-based one on classification tasks. Abstract : This paper investigates the dynamics-inspired neuromorphic architecture for neural representation and learning following Hamilton's principle.
This paper investigates the dynamics-inspired neuromorphic architecture for visual representation learning following Hamilton’s principle. Our method converts weight-based neural structure to its dynamics-based form that consists of finite sub-models, whose mutual relations measured by computing path integrals amongst their dynamical states ...
This paper investigates the dynamics-inspired neu-romorphic architecture for visual representation learning following Hamilton’s principle.
Interpreting arbitrary neural structures as DyN systems. Every tensors-based neural structure (e.g., attention, convolutional layer, FC layer) can be represented by a set of subsystems that deal with time-variant signals. From neural layer to DyN.
NIR supports an unprecedented number of neuromorphic systems, which we demonstrate by reproducing three spiking neural network models of different complexity across 7 neuromorphic simulators...
In our study, we consider the structure and numerical learning from neuromorphic dynamics aspect, inspired by Hebb’s learning rule (Cooper, 2005). The rule states that neural connections between neurons with similar dynamic behaviors tend to be stronger. Rather than constructing a neural structure with a learning mechanism following Hebb’s ...
Bibliographic details on Dynamics-inspired Neuromorphic Visual Representation Learning.
This paper investigates the dynamics-inspired neuromorphic architecture for visual representation learning following Hamilton's principle. Our method converts weight-based neural structure to its dynamics-based form that consists of finite sub-models, whose mutual relations measured by computing path integrals amongst their dynamical states are ...
Nature Communications - Designing efficient neuromorphic systems based on nanowire networks remains a challenge. Here, Zhu et al. demonstrate brain-inspired learning and memory of...
This paper investigates the dynamics-inspired neuromorphic architecture for visual representation learning following Hamilton's principle. Our method converts weight-based neural structure to its dynamics-based form that consists of finite sub-models, whose mutual relations measured by computing path integrals amongst their dynamical states are ...